mirror of https://github.com/alibaba/EasyCV.git
Feature/paddleocr inference (#148)
* add ocr model and convert weights from paddleocrv3pull/204/head^2
parent
bb68fcbf5c
commit
397ecf2658
|
@ -0,0 +1,85 @@
|
|||
# OCR algorithm
|
||||
## PP-OCRv3
|
||||
We convert [PaddleOCRv3](https://github.com/PaddlePaddle/PaddleOCR) models to pytorch style, and provide end2end interface to recognize text in images, by simplely load exported models.
|
||||
### detection
|
||||
We test on on icdar2015 dataset.
|
||||
|Algorithm|backbone|configs|precison|recall|Hmean|Download|
|
||||
|:---:|:---:|:---:|:---:|:---:|:---:|:---:|
|
||||
|DB|MobileNetv3|[det_model_en.py](configs/ocr/detection/det_model_en.py)|0.7803|0.7250|0.7516|[log](http://pai-vision-data-hz.oss-cn-zhangjiakou.aliyuncs.com/EasyCV/modelzoo/ocr/det/fintune_icdar2015_mobilev3/20220902_140307.log.json)-[model](http://pai-vision-data-hz.oss-cn-zhangjiakou.aliyuncs.com/EasyCV/modelzoo/ocr/det/fintune_icdar2015_mobilev3/epoch_70.pth)|
|
||||
|DB|R50|[det_model_en_r50.py](configs/ocr/detection/det_model_en_r50.py)|0.8622|0.8218|0.8415|[log](http://pai-vision-data-hz.oss-cn-zhangjiakou.aliyuncs.com/EasyCV/modelzoo/ocr/det/fintune_icdar2015_r50/20220906_110252.log.json)-[model](http://pai-vision-data-hz.oss-cn-zhangjiakou.aliyuncs.com/EasyCV/modelzoo/ocr/det/fintune_icdar2015_r50/epoch_1150.pth)|
|
||||
### recognition
|
||||
We test on on [DTRB](https://arxiv.org/abs/1904.01906) dataset.
|
||||
|Algorithm|backbone|configs|acc|Download|
|
||||
|:---:|:---:|:---:|:---:|:---:|
|
||||
|SVTR|MobileNetv1|[rec_model_en.py](configs/ocr/recognition/rec_model_en.py)|0.7536|[log](http://pai-vision-data-hz.oss-cn-zhangjiakou.aliyuncs.com/EasyCV/modelzoo/ocr/rec/fintune_dtrb/20220914_125616.log.json)-[model](http://pai-vision-data-hz.oss-cn-zhangjiakou.aliyuncs.com/EasyCV/modelzoo/ocr/rec/fintune_dtrb/epoch_60.pth)|
|
||||
### predict
|
||||
We provide exported models contain weights and process config for easyly predict, which convert from PaddleOCRv3.
|
||||
#### detection model
|
||||
|language|Download|
|
||||
|---|---|
|
||||
|chinese|[chinese_det.pth](http://pai-vision-data-hz.oss-cn-zhangjiakou.aliyuncs.com/EasyCV/modelzoo/ocr/export_model/det/chinese_det.pth)|
|
||||
|english|[english_det.pth](http://pai-vision-data-hz.oss-cn-zhangjiakou.aliyuncs.com/EasyCV/modelzoo/ocr/export_model/det/english_det.pth)|
|
||||
|multilingual|[multilingual_det.pth](http://pai-vision-data-hz.oss-cn-zhangjiakou.aliyuncs.com/EasyCV/modelzoo/ocr/export_model/det/multilingual_det.pth)|
|
||||
#### recognition model
|
||||
|language|Download|
|
||||
|---|---|
|
||||
|chiese|[chinese_rec.pth](http://pai-vision-data-hz.oss-cn-zhangjiakou.aliyuncs.com/EasyCV/modelzoo/ocr/export_model/rec/chinese_rec.pth)|
|
||||
|english|[english_rec.pth](http://pai-vision-data-hz.oss-cn-zhangjiakou.aliyuncs.com/EasyCV/modelzoo/ocr/export_model/rec/english_rec.pth)|
|
||||
|korean|[korean_rec.pth](http://pai-vision-data-hz.oss-cn-zhangjiakou.aliyuncs.com/EasyCV/modelzoo/ocr/export_model/rec/korean_rec.pth)|
|
||||
|japan|[japan_rec.pth](http://pai-vision-data-hz.oss-cn-zhangjiakou.aliyuncs.com/EasyCV/modelzoo/ocr/export_model/rec/japan_rec.pth)|
|
||||
|chinese_cht|[chinese_cht_rec.pth](http://pai-vision-data-hz.oss-cn-zhangjiakou.aliyuncs.com/EasyCV/modelzoo/ocr/export_model/rec/chinese_cht_rec.pth)|
|
||||
|Telugu|[te_rec.pth](http://pai-vision-data-hz.oss-cn-zhangjiakou.aliyuncs.com/EasyCV/modelzoo/ocr/export_model/rec/te_rec.pth)|
|
||||
|Canada|[ka_rec.pth](http://pai-vision-data-hz.oss-cn-zhangjiakou.aliyuncs.com/EasyCV/modelzoo/ocr/export_model/rec/ka_rec.pth)|
|
||||
|Tamil|[ta_rec.pth](http://pai-vision-data-hz.oss-cn-zhangjiakou.aliyuncs.com/EasyCV/modelzoo/ocr/export_model/rec/ta_rec.pth)|
|
||||
|latin|[latin_rec.pth](http://pai-vision-data-hz.oss-cn-zhangjiakou.aliyuncs.com/EasyCV/modelzoo/ocr/export_model/rec/latin_rec.pth)|
|
||||
|cyrillic|[cyrillic_rec.pth](http://pai-vision-data-hz.oss-cn-zhangjiakou.aliyuncs.com/EasyCV/modelzoo/ocr/export_model/rec/cyrillic_rec.pth)|
|
||||
|devanagari|[devanagari_rec.pth](http://pai-vision-data-hz.oss-cn-zhangjiakou.aliyuncs.com/EasyCV/modelzoo/ocr/export_model/rec/devanagari_rec.pth)|
|
||||
#### direction model
|
||||
|language|Download|
|
||||
|---|---|
|
||||
||[direction.pth](http://pai-vision-data-hz.oss-cn-zhangjiakou.aliyuncs.com/EasyCV/modelzoo/ocr/export_model/cls/direction.pth)|
|
||||
#### usage
|
||||
##### detection
|
||||
```
|
||||
import cv2
|
||||
from easycv.predictors.ocr import OCRDetPredictor
|
||||
predictor = OCRDetPredictor(model_path)
|
||||
out = predictor([img_path]) # out = predictor([img])
|
||||
img = cv2.imread(img_path)
|
||||
out_img = predictor.show_result(out[0], img)
|
||||
cv2.imwrite(out_img_path,out_img)
|
||||
```
|
||||
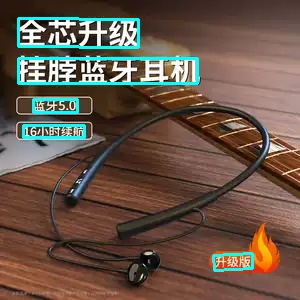
|
||||
##### recognition
|
||||
```
|
||||
import cv2
|
||||
from easycv.predictors.ocr import OCRRecPredictor
|
||||
predictor = OCRRecPredictor(model_path)
|
||||
out = predictor([img_path])
|
||||
print(out)
|
||||
```
|
||||
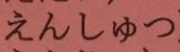<br/>
|
||||

|
||||
##### end2end
|
||||
```
|
||||
import cv2
|
||||
from easycv.predictors.ocr import OCRPredictor
|
||||
! wget http://pai-vision-data-hz.oss-cn-zhangjiakou.aliyuncs.com/EasyCV/modelzoo/ocr/simfang.ttf
|
||||
! wget http://pai-vision-data-hz.oss-cn-zhangjiakou.aliyuncs.com/EasyCV/modelzoo/ocr/ocr_det.jpg
|
||||
predictor = OCRPredictor(
|
||||
det_model_path=path_to_detmodel,
|
||||
rec_model_path=path_to_recmodel,
|
||||
cls_model_path=path_to_clsmodel,
|
||||
use_angle_cls=True)
|
||||
filter_boxes, filter_rec_res = predictor(img_path)
|
||||
img = cv2.imread('ocr_det.jpg')
|
||||
out_img = predictor.show(
|
||||
filter_boxes[0],
|
||||
filter_rec_res[0],
|
||||
img,
|
||||
font_path='simfang.ttf')
|
||||
cv2.imwrite('out_img.jpg', out_img)
|
||||
```
|
||||
There are some ocr results.<br/>
|
||||
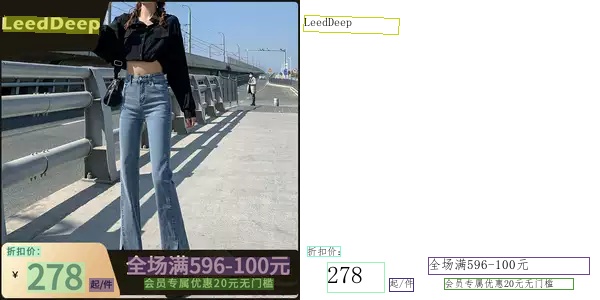
|
||||
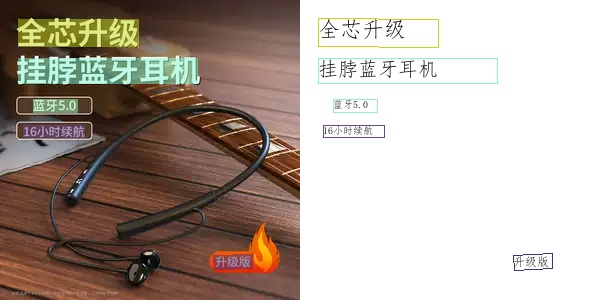
|
|
@ -0,0 +1,148 @@
|
|||
_base_ = ['configs/base.py']
|
||||
|
||||
model = dict(
|
||||
type='DBNet',
|
||||
backbone=dict(
|
||||
type='OCRDetMobileNetV3',
|
||||
scale=0.5,
|
||||
model_name='large',
|
||||
disable_se=True),
|
||||
neck=dict(
|
||||
type='RSEFPN',
|
||||
in_channels=[16, 24, 56, 480],
|
||||
out_channels=96,
|
||||
shortcut=True),
|
||||
head=dict(type='DBHead', in_channels=96, k=50),
|
||||
postprocess=dict(
|
||||
type='DBPostProcess',
|
||||
thresh=0.3,
|
||||
box_thresh=0.6,
|
||||
max_candidates=1000,
|
||||
unclip_ratio=1.5,
|
||||
use_dilation=False,
|
||||
score_mode='fast'),
|
||||
loss=dict(
|
||||
type='DBLoss',
|
||||
balance_loss=True,
|
||||
main_loss_type='DiceLoss',
|
||||
alpha=5,
|
||||
beta=10,
|
||||
ohem_ratio=3),
|
||||
pretrained=
|
||||
'http://pai-vision-data-hz.oss-cn-zhangjiakou.aliyuncs.com/EasyCV/modelzoo/ocr/det/ch_PP-OCRv3_det/student.pth'
|
||||
)
|
||||
|
||||
img_norm_cfg = dict(
|
||||
mean=[123.675, 116.28, 103.53], std=[58.395, 57.12, 57.375], to_rgb=False)
|
||||
|
||||
train_pipeline = [
|
||||
dict(
|
||||
type='IaaAugment',
|
||||
augmenter_args=[{
|
||||
'type': 'Fliplr',
|
||||
'args': {
|
||||
'p': 0.5
|
||||
}
|
||||
}, {
|
||||
'type': 'Affine',
|
||||
'args': {
|
||||
'rotate': [-10, 10]
|
||||
}
|
||||
}, {
|
||||
'type': 'Resize',
|
||||
'args': {
|
||||
'size': [0.5, 3]
|
||||
}
|
||||
}]),
|
||||
dict(
|
||||
type='EastRandomCropData',
|
||||
size=[640, 640],
|
||||
max_tries=50,
|
||||
keep_ratio=True),
|
||||
dict(
|
||||
type='MakeBorderMap', shrink_ratio=0.4, thresh_min=0.3,
|
||||
thresh_max=0.7),
|
||||
dict(type='MakeShrinkMap', shrink_ratio=0.4, min_text_size=8),
|
||||
dict(type='MMNormalize', **img_norm_cfg),
|
||||
dict(
|
||||
type='ImageToTensor',
|
||||
keys=[
|
||||
'img', 'threshold_map', 'threshold_mask', 'shrink_map',
|
||||
'shrink_mask'
|
||||
]),
|
||||
dict(
|
||||
type='Collect',
|
||||
keys=[
|
||||
'img', 'threshold_map', 'threshold_mask', 'shrink_map',
|
||||
'shrink_mask'
|
||||
]),
|
||||
]
|
||||
|
||||
test_pipeline = [
|
||||
dict(type='OCRDetResize', limit_side_len=640, limit_type='min'),
|
||||
dict(type='MMNormalize', **img_norm_cfg),
|
||||
dict(type='ImageToTensor', keys=['img']),
|
||||
dict(
|
||||
type='Collect',
|
||||
keys=['img'],
|
||||
meta_keys=['ori_img_shape', 'polys', 'ignore_tags']),
|
||||
]
|
||||
|
||||
val_pipeline = [
|
||||
dict(type='OCRDetResize', limit_side_len=640, limit_type='min'),
|
||||
dict(type='MMNormalize', **img_norm_cfg),
|
||||
dict(type='ImageToTensor', keys=['img']),
|
||||
dict(
|
||||
type='Collect',
|
||||
keys=['img'],
|
||||
meta_keys=['ori_img_shape', 'polys', 'ignore_tags']),
|
||||
]
|
||||
|
||||
train_dataset = dict(
|
||||
type='OCRDetDataset',
|
||||
data_source=dict(
|
||||
type='OCRPaiDetSource',
|
||||
label_file=[
|
||||
'ocr/det/pai/label_file/train/20191218131226_npx_e2e_train.csv',
|
||||
'ocr/det/pai/label_file/train/20191218131302_social_e2e_train.csv',
|
||||
'ocr/det/pai/label_file/train/20191218122330_book_e2e_train.csv',
|
||||
],
|
||||
data_dir='ocr/det/pai/img/train'),
|
||||
pipeline=train_pipeline)
|
||||
|
||||
val_dataset = dict(
|
||||
type='OCRDetDataset',
|
||||
imgs_per_gpu=1,
|
||||
data_source=dict(
|
||||
type='OCRPaiDetSource',
|
||||
label_file=[
|
||||
'ocr/det/pai/label_file/test/20191218131744_npx_e2e_test.csv',
|
||||
'ocr/det/pai/label_file/test/20191218131817_social_e2e_test.csv'
|
||||
],
|
||||
data_dir='ocr/det/pai/img/test'),
|
||||
pipeline=val_pipeline)
|
||||
|
||||
data = dict(
|
||||
imgs_per_gpu=16, workers_per_gpu=2, train=train_dataset, val=val_dataset)
|
||||
|
||||
total_epochs = 100
|
||||
optimizer = dict(type='Adam', lr=0.001, betas=(0.9, 0.999))
|
||||
|
||||
# learning policy
|
||||
lr_config = dict(policy='fixed')
|
||||
|
||||
checkpoint_config = dict(interval=1)
|
||||
|
||||
log_config = dict(
|
||||
interval=10, hooks=[
|
||||
dict(type='TextLoggerHook'),
|
||||
])
|
||||
|
||||
eval_config = dict(initial=True, interval=1, gpu_collect=False)
|
||||
eval_pipelines = [
|
||||
dict(
|
||||
mode='test',
|
||||
dist_eval=True,
|
||||
evaluators=[dict(type='OCRDetEvaluator')],
|
||||
)
|
||||
]
|
|
@ -0,0 +1,145 @@
|
|||
_base_ = ['configs/base.py']
|
||||
|
||||
model = dict(
|
||||
type='DBNet',
|
||||
backbone=dict(type='OCRDetResNet', in_channels=3, layers=50),
|
||||
neck=dict(
|
||||
type='LKPAN',
|
||||
in_channels=[256, 512, 1024, 2048],
|
||||
out_channels=256,
|
||||
shortcut=True),
|
||||
head=dict(type='DBHead', in_channels=256, kernel_list=[7, 2, 2], k=50),
|
||||
postprocess=dict(
|
||||
type='DBPostProcess',
|
||||
thresh=0.3,
|
||||
box_thresh=0.6,
|
||||
max_candidates=1000,
|
||||
unclip_ratio=1.5,
|
||||
use_dilation=False,
|
||||
score_mode='fast'),
|
||||
loss=dict(
|
||||
type='DBLoss',
|
||||
balance_loss=True,
|
||||
main_loss_type='DiceLoss',
|
||||
alpha=5,
|
||||
beta=10,
|
||||
ohem_ratio=3),
|
||||
pretrained=
|
||||
'http://pai-vision-data-hz.oss-cn-zhangjiakou.aliyuncs.com/EasyCV/modelzoo/ocr/det/ch_PP-OCRv3_det/teacher.pth'
|
||||
)
|
||||
|
||||
img_norm_cfg = dict(
|
||||
mean=[123.675, 116.28, 103.53], std=[58.395, 57.12, 57.375], to_rgb=False)
|
||||
|
||||
train_pipeline = [
|
||||
dict(
|
||||
type='IaaAugment',
|
||||
augmenter_args=[{
|
||||
'type': 'Fliplr',
|
||||
'args': {
|
||||
'p': 0.5
|
||||
}
|
||||
}, {
|
||||
'type': 'Affine',
|
||||
'args': {
|
||||
'rotate': [-10, 10]
|
||||
}
|
||||
}, {
|
||||
'type': 'Resize',
|
||||
'args': {
|
||||
'size': [0.5, 3]
|
||||
}
|
||||
}]),
|
||||
dict(
|
||||
type='EastRandomCropData',
|
||||
size=[640, 640],
|
||||
max_tries=50,
|
||||
keep_ratio=True),
|
||||
dict(
|
||||
type='MakeBorderMap', shrink_ratio=0.4, thresh_min=0.3,
|
||||
thresh_max=0.7),
|
||||
dict(type='MakeShrinkMap', shrink_ratio=0.4, min_text_size=8),
|
||||
dict(type='MMNormalize', **img_norm_cfg),
|
||||
dict(
|
||||
type='ImageToTensor',
|
||||
keys=[
|
||||
'img', 'threshold_map', 'threshold_mask', 'shrink_map',
|
||||
'shrink_mask'
|
||||
]),
|
||||
dict(
|
||||
type='Collect',
|
||||
keys=[
|
||||
'img', 'threshold_map', 'threshold_mask', 'shrink_map',
|
||||
'shrink_mask'
|
||||
]),
|
||||
]
|
||||
|
||||
test_pipeline = [
|
||||
dict(type='OCRDetResize', limit_side_len=960),
|
||||
dict(type='MMNormalize', **img_norm_cfg),
|
||||
dict(type='ImageToTensor', keys=['img']),
|
||||
dict(
|
||||
type='Collect',
|
||||
keys=['img'],
|
||||
meta_keys=['ori_img_shape', 'polys', 'ignore_tags']),
|
||||
]
|
||||
|
||||
val_pipeline = [
|
||||
dict(type='OCRDetResize', limit_side_len=960),
|
||||
dict(type='MMNormalize', **img_norm_cfg),
|
||||
dict(type='ImageToTensor', keys=['img']),
|
||||
dict(
|
||||
type='Collect',
|
||||
keys=['img'],
|
||||
meta_keys=['ori_img_shape', 'polys', 'ignore_tags']),
|
||||
]
|
||||
|
||||
train_dataset = dict(
|
||||
type='OCRDetDataset',
|
||||
data_source=dict(
|
||||
type='OCRPaiDetSource',
|
||||
label_file=[
|
||||
'ocr/det/pai/label_file/train/20191218131226_npx_e2e_train.csv',
|
||||
'ocr/det/pai/label_file/train/20191218131302_social_e2e_train.csv',
|
||||
'ocr/det/pai/label_file/train/20191218122330_book_e2e_train.csv',
|
||||
],
|
||||
data_dir='ocr/det/pai/img/train'),
|
||||
pipeline=train_pipeline)
|
||||
|
||||
val_dataset = dict(
|
||||
type='OCRDetDataset',
|
||||
imgs_per_gpu=1,
|
||||
data_source=dict(
|
||||
type='OCRPaiDetSource',
|
||||
label_file=[
|
||||
'ocr/det/pai/label_file/test/20191218131744_npx_e2e_test.csv',
|
||||
'ocr/det/pai/label_file/test/20191218131817_social_e2e_test.csv'
|
||||
],
|
||||
data_dir='ocr/det/pai/img/test'),
|
||||
pipeline=val_pipeline)
|
||||
|
||||
data = dict(
|
||||
imgs_per_gpu=8, workers_per_gpu=2, train=train_dataset, val=val_dataset)
|
||||
|
||||
total_epochs = 100
|
||||
|
||||
optimizer = dict(type='Adam', lr=0.001, betas=(0.9, 0.999))
|
||||
|
||||
# learning policy
|
||||
lr_config = dict(policy='fixed')
|
||||
|
||||
checkpoint_config = dict(interval=1)
|
||||
|
||||
log_config = dict(
|
||||
interval=10, hooks=[
|
||||
dict(type='TextLoggerHook'),
|
||||
])
|
||||
|
||||
eval_config = dict(initial=True, interval=1, gpu_collect=False)
|
||||
eval_pipelines = [
|
||||
dict(
|
||||
mode='test',
|
||||
dist_eval=True,
|
||||
evaluators=[dict(type='OCRDetEvaluator')],
|
||||
)
|
||||
]
|
|
@ -0,0 +1,154 @@
|
|||
_base_ = ['configs/base.py']
|
||||
|
||||
model = dict(
|
||||
type='DBNet',
|
||||
backbone=dict(
|
||||
type='OCRDetMobileNetV3',
|
||||
scale=0.5,
|
||||
model_name='large',
|
||||
disable_se=True),
|
||||
neck=dict(
|
||||
type='RSEFPN',
|
||||
in_channels=[16, 24, 56, 480],
|
||||
out_channels=96,
|
||||
shortcut=True),
|
||||
head=dict(type='DBHead', in_channels=96, k=50),
|
||||
postprocess=dict(
|
||||
type='DBPostProcess',
|
||||
thresh=0.3,
|
||||
box_thresh=0.6,
|
||||
max_candidates=1000,
|
||||
unclip_ratio=1.5,
|
||||
use_dilation=False,
|
||||
score_mode='fast'),
|
||||
loss=dict(
|
||||
type='DBLoss',
|
||||
balance_loss=True,
|
||||
main_loss_type='DiceLoss',
|
||||
alpha=5,
|
||||
beta=10,
|
||||
ohem_ratio=3),
|
||||
pretrained=
|
||||
'http://pai-vision-data-hz.oss-cn-zhangjiakou.aliyuncs.com/EasyCV/modelzoo/ocr/det/en_PP-OCRv3_det/student.pth'
|
||||
)
|
||||
|
||||
img_norm_cfg = dict(
|
||||
mean=[123.675, 116.28, 103.53], std=[58.395, 57.12, 57.375], to_rgb=False)
|
||||
|
||||
train_pipeline = [
|
||||
dict(
|
||||
type='IaaAugment',
|
||||
augmenter_args=[{
|
||||
'type': 'Fliplr',
|
||||
'args': {
|
||||
'p': 0.5
|
||||
}
|
||||
}, {
|
||||
'type': 'Affine',
|
||||
'args': {
|
||||
'rotate': [-10, 10]
|
||||
}
|
||||
}, {
|
||||
'type': 'Resize',
|
||||
'args': {
|
||||
'size': [0.5, 3]
|
||||
}
|
||||
}]),
|
||||
dict(
|
||||
type='EastRandomCropData',
|
||||
size=[640, 640],
|
||||
max_tries=50,
|
||||
keep_ratio=True),
|
||||
dict(
|
||||
type='MakeBorderMap', shrink_ratio=0.4, thresh_min=0.3,
|
||||
thresh_max=0.7),
|
||||
dict(type='MakeShrinkMap', shrink_ratio=0.4, min_text_size=8),
|
||||
dict(type='MMNormalize', **img_norm_cfg),
|
||||
dict(
|
||||
type='ImageToTensor',
|
||||
keys=[
|
||||
'img', 'threshold_map', 'threshold_mask', 'shrink_map',
|
||||
'shrink_mask'
|
||||
]),
|
||||
dict(
|
||||
type='Collect',
|
||||
keys=[
|
||||
'img', 'threshold_map', 'threshold_mask', 'shrink_map',
|
||||
'shrink_mask'
|
||||
]),
|
||||
]
|
||||
|
||||
# test_pipeline = [
|
||||
# dict(type='MMResize', img_scale=(960, 960)),
|
||||
# dict(type='ResizeDivisor', size_divisor=32),
|
||||
# dict(type='MMNormalize', **img_norm_cfg),
|
||||
# dict(type='ImageToTensor', keys=['img']),
|
||||
# dict(
|
||||
# type='Collect',
|
||||
# keys=['img'],
|
||||
# meta_keys=['ori_img_shape', 'polys', 'ignore_tags']),
|
||||
# ]
|
||||
test_pipeline = [
|
||||
dict(type='OCRDetResize', limit_side_len=640, limit_type='min'),
|
||||
dict(type='MMNormalize', **img_norm_cfg),
|
||||
dict(type='ImageToTensor', keys=['img']),
|
||||
dict(
|
||||
type='Collect',
|
||||
keys=['img'],
|
||||
meta_keys=['ori_img_shape', 'polys', 'ignore_tags']),
|
||||
]
|
||||
|
||||
val_pipeline = [
|
||||
dict(type='OCRDetResize', image_shape=(736, 1280)),
|
||||
dict(type='MMNormalize', **img_norm_cfg),
|
||||
dict(type='ImageToTensor', keys=['img']),
|
||||
dict(
|
||||
type='Collect',
|
||||
keys=['img'],
|
||||
meta_keys=['ori_img_shape', 'polys', 'ignore_tags']),
|
||||
]
|
||||
|
||||
train_dataset = dict(
|
||||
type='OCRDetDataset',
|
||||
data_source=dict(
|
||||
type='OCRDetSource',
|
||||
label_file=
|
||||
'ocr/det/icdar2015/text_localization/train_icdar2015_label.txt',
|
||||
data_dir='ocr/det/icdar2015/text_localization'),
|
||||
pipeline=train_pipeline)
|
||||
|
||||
val_dataset = dict(
|
||||
type='OCRDetDataset',
|
||||
imgs_per_gpu=2,
|
||||
data_source=dict(
|
||||
type='OCRDetSource',
|
||||
label_file=
|
||||
'ocr/det/icdar2015/text_localization/test_icdar2015_label.txt',
|
||||
data_dir='ocr/det/icdar2015/text_localization',
|
||||
test_mode=True),
|
||||
pipeline=val_pipeline)
|
||||
|
||||
data = dict(
|
||||
imgs_per_gpu=16, workers_per_gpu=2, train=train_dataset, val=val_dataset)
|
||||
|
||||
total_epochs = 100
|
||||
optimizer = dict(type='Adam', lr=0.001, betas=(0.9, 0.999))
|
||||
|
||||
# learning policy
|
||||
lr_config = dict(policy='fixed')
|
||||
|
||||
checkpoint_config = dict(interval=1)
|
||||
|
||||
log_config = dict(
|
||||
interval=10, hooks=[
|
||||
dict(type='TextLoggerHook'),
|
||||
])
|
||||
|
||||
eval_config = dict(initial=False, interval=1, gpu_collect=False)
|
||||
eval_pipelines = [
|
||||
dict(
|
||||
mode='test',
|
||||
dist_eval=True,
|
||||
evaluators=[dict(type='OCRDetEvaluator')],
|
||||
)
|
||||
]
|
|
@ -0,0 +1,148 @@
|
|||
_base_ = ['configs/base.py']
|
||||
|
||||
model = dict(
|
||||
type='DBNet',
|
||||
backbone=dict(type='OCRDetResNet', in_channels=3, layers=50),
|
||||
neck=dict(
|
||||
type='LKPAN',
|
||||
in_channels=[256, 512, 1024, 2048],
|
||||
out_channels=256,
|
||||
shortcut=True),
|
||||
head=dict(type='DBHead', in_channels=256, kernel_list=[7, 2, 2], k=50),
|
||||
postprocess=dict(
|
||||
type='DBPostProcess',
|
||||
thresh=0.3,
|
||||
box_thresh=0.6,
|
||||
max_candidates=1000,
|
||||
unclip_ratio=1.5,
|
||||
use_dilation=False,
|
||||
score_mode='fast'),
|
||||
loss=dict(
|
||||
type='DBLoss',
|
||||
balance_loss=True,
|
||||
main_loss_type='DiceLoss',
|
||||
alpha=5,
|
||||
beta=10,
|
||||
ohem_ratio=3),
|
||||
pretrained=
|
||||
'http://pai-vision-data-hz.oss-cn-zhangjiakou.aliyuncs.com/EasyCV/modelzoo/ocr/det/en_PP-OCRv3_det/teacher.pth'
|
||||
)
|
||||
|
||||
img_norm_cfg = dict(
|
||||
mean=[123.675, 116.28, 103.53], std=[58.395, 57.12, 57.375], to_rgb=False)
|
||||
|
||||
train_pipeline = [
|
||||
dict(
|
||||
type='IaaAugment',
|
||||
augmenter_args=[{
|
||||
'type': 'Fliplr',
|
||||
'args': {
|
||||
'p': 0.5
|
||||
}
|
||||
}, {
|
||||
'type': 'Affine',
|
||||
'args': {
|
||||
'rotate': [-10, 10]
|
||||
}
|
||||
}, {
|
||||
'type': 'Resize',
|
||||
'args': {
|
||||
'size': [0.5, 3]
|
||||
}
|
||||
}]),
|
||||
dict(
|
||||
type='EastRandomCropData',
|
||||
size=[640, 640],
|
||||
max_tries=50,
|
||||
keep_ratio=True),
|
||||
dict(
|
||||
type='MakeBorderMap', shrink_ratio=0.4, thresh_min=0.3,
|
||||
thresh_max=0.7),
|
||||
dict(type='MakeShrinkMap', shrink_ratio=0.4, min_text_size=8),
|
||||
dict(type='MMNormalize', **img_norm_cfg),
|
||||
dict(
|
||||
type='ImageToTensor',
|
||||
keys=[
|
||||
'img', 'threshold_map', 'threshold_mask', 'shrink_map',
|
||||
'shrink_mask'
|
||||
]),
|
||||
dict(
|
||||
type='Collect',
|
||||
keys=[
|
||||
'img', 'threshold_map', 'threshold_mask', 'shrink_map',
|
||||
'shrink_mask'
|
||||
]),
|
||||
]
|
||||
|
||||
test_pipeline = [
|
||||
dict(type='MMResize', img_scale=(960, 960)),
|
||||
dict(type='ResizeDivisor', size_divisor=32),
|
||||
dict(type='MMNormalize', **img_norm_cfg),
|
||||
dict(type='ImageToTensor', keys=['img']),
|
||||
dict(
|
||||
type='Collect',
|
||||
keys=['img'],
|
||||
meta_keys=['ori_img_shape', 'polys', 'ignore_tags']),
|
||||
]
|
||||
|
||||
val_pipeline = [
|
||||
dict(type='OCRDetResize', image_shape=(736, 1280)),
|
||||
dict(type='MMNormalize', **img_norm_cfg),
|
||||
dict(type='ImageToTensor', keys=['img']),
|
||||
dict(
|
||||
type='Collect',
|
||||
keys=['img'],
|
||||
meta_keys=['ori_img_shape', 'polys', 'ignore_tags']),
|
||||
]
|
||||
|
||||
train_dataset = dict(
|
||||
type='OCRDetDataset',
|
||||
data_source=dict(
|
||||
type='OCRDetSource',
|
||||
label_file=
|
||||
'ocr/det/icdar2015/text_localization/train_icdar2015_label.txt',
|
||||
data_dir='ocr/det/icdar2015/text_localization'),
|
||||
pipeline=train_pipeline)
|
||||
|
||||
val_dataset = dict(
|
||||
type='OCRDetDataset',
|
||||
imgs_per_gpu=2,
|
||||
data_source=dict(
|
||||
type='OCRDetSource',
|
||||
label_file=
|
||||
'ocr/det/icdar2015/text_localization/test_icdar2015_label.txt',
|
||||
data_dir='ocr/det/icdar2015/text_localization',
|
||||
test_mode=True),
|
||||
pipeline=val_pipeline)
|
||||
|
||||
data = dict(
|
||||
imgs_per_gpu=16, workers_per_gpu=2, train=train_dataset, val=val_dataset)
|
||||
|
||||
total_epochs = 1200
|
||||
optimizer = dict(type='Adam', lr=0.001, weight_decay=1e-4, betas=(0.9, 0.999))
|
||||
|
||||
# learning policy
|
||||
lr_config = dict(
|
||||
policy='CosineAnnealing',
|
||||
min_lr=1e-5,
|
||||
warmup='linear',
|
||||
warmup_iters=5,
|
||||
warmup_ratio=1e-4,
|
||||
warmup_by_epoch=True,
|
||||
by_epoch=False)
|
||||
|
||||
checkpoint_config = dict(interval=10)
|
||||
|
||||
log_config = dict(
|
||||
interval=10, hooks=[
|
||||
dict(type='TextLoggerHook'),
|
||||
])
|
||||
|
||||
eval_config = dict(initial=True, interval=1, gpu_collect=False)
|
||||
eval_pipelines = [
|
||||
dict(
|
||||
mode='test',
|
||||
dist_eval=True,
|
||||
evaluators=[dict(type='OCRDetEvaluator')],
|
||||
)
|
||||
]
|
|
@ -0,0 +1,86 @@
|
|||
_base_ = ['configs/base.py']
|
||||
|
||||
model = dict(
|
||||
type='TextClassifier',
|
||||
backbone=dict(type='OCRRecMobileNetV3', scale=0.35, model_name='small'),
|
||||
head=dict(
|
||||
type='ClsHead',
|
||||
with_avg_pool=True,
|
||||
in_channels=200,
|
||||
num_classes=2,
|
||||
),
|
||||
pretrained=
|
||||
'http://pai-vision-data-hz.oss-cn-zhangjiakou.aliyuncs.com/EasyCV/modelzoo/ocr/cls/ch_ppocr_mobile_v2.0_cls/best_accuracy.pth'
|
||||
)
|
||||
|
||||
train_pipeline = [
|
||||
dict(type='RecAug', use_tia=False),
|
||||
dict(type='ClsResizeImg', img_shape=(3, 48, 192)),
|
||||
dict(type='MMToTensor'),
|
||||
dict(type='Collect', keys=['img', 'label'], meta_keys=['img_path'])
|
||||
]
|
||||
|
||||
val_pipeline = [
|
||||
dict(type='ClsResizeImg', img_shape=(3, 48, 192)),
|
||||
dict(type='MMToTensor'),
|
||||
dict(type='Collect', keys=['img', 'label'], meta_keys=['img_path'])
|
||||
]
|
||||
|
||||
test_pipeline = [
|
||||
dict(type='ClsResizeImg', img_shape=(3, 48, 192)),
|
||||
dict(type='MMToTensor'),
|
||||
dict(type='Collect', keys=['img'], meta_keys=['img_path'])
|
||||
]
|
||||
|
||||
train_dataset = dict(
|
||||
type='OCRClsDataset',
|
||||
data_source=dict(
|
||||
type='OCRClsSource',
|
||||
label_file='ocr/direction/pai/label_file/test_direction.txt',
|
||||
data_dir='ocr/direction/pai/img/test',
|
||||
label_list=['0', '180'],
|
||||
),
|
||||
pipeline=train_pipeline)
|
||||
|
||||
val_dataset = dict(
|
||||
type='OCRClsDataset',
|
||||
data_source=dict(
|
||||
type='OCRClsSource',
|
||||
label_file='ocr/direction/pai/label_file/test_direction.txt',
|
||||
data_dir='ocr/direction/pai/img/test',
|
||||
label_list=['0', '180'],
|
||||
test_mode=True),
|
||||
pipeline=val_pipeline)
|
||||
|
||||
data = dict(
|
||||
imgs_per_gpu=512, workers_per_gpu=8, train=train_dataset, val=val_dataset)
|
||||
|
||||
total_epochs = 100
|
||||
optimizer = dict(type='Adam', lr=0.001, betas=(0.9, 0.999))
|
||||
|
||||
# learning policy
|
||||
lr_config = dict(
|
||||
policy='CosineAnnealing',
|
||||
min_lr=1e-5,
|
||||
warmup='linear',
|
||||
warmup_iters=5,
|
||||
warmup_ratio=1e-4,
|
||||
warmup_by_epoch=True,
|
||||
by_epoch=False)
|
||||
|
||||
checkpoint_config = dict(interval=10)
|
||||
|
||||
log_config = dict(
|
||||
interval=10, hooks=[
|
||||
dict(type='TextLoggerHook'),
|
||||
])
|
||||
|
||||
eval_config = dict(initial=True, interval=1, gpu_collect=False)
|
||||
eval_pipelines = [
|
||||
dict(
|
||||
mode='test',
|
||||
data=data['val'],
|
||||
dist_eval=False,
|
||||
evaluators=[dict(type='ClsEvaluator', topk=(1, ))],
|
||||
)
|
||||
]
|
|
@ -0,0 +1,144 @@
|
|||
_base_ = ['configs/base.py']
|
||||
|
||||
character_dict_path = 'http://pai-vision-data-hz.oss-cn-zhangjiakou.aliyuncs.com/EasyCV/modelzoo/ocr/dict/arabic_dict.txt'
|
||||
|
||||
model = dict(
|
||||
type='OCRRecNet',
|
||||
backbone=dict(
|
||||
type='OCRRecMobileNetV1Enhance',
|
||||
scale=0.5,
|
||||
last_conv_stride=[1, 2],
|
||||
last_pool_type='avg'),
|
||||
# inference
|
||||
# neck=dict(
|
||||
# type='SequenceEncoder',
|
||||
# in_channels=512,
|
||||
# encoder_type='svtr',
|
||||
# dims=64,
|
||||
# depth=2,
|
||||
# hidden_dims=120,
|
||||
# use_guide=True),
|
||||
# head=dict(
|
||||
# type='CTCHead',
|
||||
# in_channels=64,
|
||||
# fc_decay=0.00001),
|
||||
head=dict(
|
||||
type='MultiHead',
|
||||
in_channels=512,
|
||||
out_channels_list=dict(
|
||||
CTCLabelDecode=163,
|
||||
SARLabelDecode=165,
|
||||
),
|
||||
head_list=[
|
||||
dict(
|
||||
type='CTCHead',
|
||||
Neck=dict(
|
||||
type='svtr',
|
||||
dims=64,
|
||||
depth=2,
|
||||
hidden_dims=120,
|
||||
use_guide=True),
|
||||
Head=dict(fc_decay=0.00001, )),
|
||||
dict(type='SARHead', enc_dim=512, max_text_length=25)
|
||||
]),
|
||||
postprocess=dict(
|
||||
type='CTCLabelDecode',
|
||||
character_dict_path=character_dict_path,
|
||||
use_space_char=True),
|
||||
loss=dict(
|
||||
type='MultiLoss',
|
||||
ignore_index=164,
|
||||
loss_config_list=[
|
||||
dict(CTCLoss=None),
|
||||
dict(SARLoss=None),
|
||||
]),
|
||||
pretrained=None)
|
||||
|
||||
train_pipeline = [
|
||||
dict(type='RecConAug', prob=0.5, image_shape=(48, 320, 3)),
|
||||
dict(type='RecAug'),
|
||||
dict(
|
||||
type='MultiLabelEncode',
|
||||
max_text_length=25,
|
||||
use_space_char=True,
|
||||
character_dict_path=character_dict_path,
|
||||
),
|
||||
dict(type='RecResizeImg', image_shape=(3, 48, 320)),
|
||||
dict(type='MMToTensor'),
|
||||
dict(
|
||||
type='Collect',
|
||||
keys=['img', 'label_ctc', 'label_sar', 'length', 'valid_ratio'],
|
||||
meta_keys=['img_path'])
|
||||
]
|
||||
|
||||
val_pipeline = [
|
||||
dict(
|
||||
type='MultiLabelEncode',
|
||||
max_text_length=25,
|
||||
use_space_char=True,
|
||||
character_dict_path=character_dict_path,
|
||||
),
|
||||
dict(type='RecResizeImg', image_shape=(3, 48, 320)),
|
||||
dict(type='MMToTensor'),
|
||||
dict(
|
||||
type='Collect',
|
||||
keys=['img', 'label_ctc', 'label_sar', 'length', 'valid_ratio'],
|
||||
meta_keys=['img_path'])
|
||||
]
|
||||
|
||||
test_pipeline = [
|
||||
dict(type='RecResizeImg', image_shape=(3, 48, 320)),
|
||||
dict(type='MMToTensor'),
|
||||
dict(type='Collect', keys=['img'], meta_keys=['img_path'])
|
||||
]
|
||||
|
||||
train_dataset = dict(
|
||||
type='OCRRecDataset',
|
||||
data_source=dict(
|
||||
type='OCRRecSource',
|
||||
label_file='ocr/rec/pai/label_file/train.txt',
|
||||
data_dir='ocr/rec/pai/img/train',
|
||||
ext_data_num=2,
|
||||
),
|
||||
pipeline=train_pipeline)
|
||||
|
||||
val_dataset = dict(
|
||||
type='OCRRecDataset',
|
||||
data_source=dict(
|
||||
type='OCRRecSource',
|
||||
label_file='ocr/rec/pai/label_file/test.txt',
|
||||
data_dir='ocr/rec/pai/img/test',
|
||||
ext_data_num=0,
|
||||
),
|
||||
pipeline=val_pipeline)
|
||||
|
||||
data = dict(
|
||||
imgs_per_gpu=128, workers_per_gpu=4, train=train_dataset, val=val_dataset)
|
||||
|
||||
total_epochs = 10
|
||||
optimizer = dict(type='Adam', lr=0.001, betas=(0.9, 0.999))
|
||||
|
||||
lr_config = dict(
|
||||
policy='CosineAnnealing',
|
||||
min_lr=1e-5,
|
||||
warmup='linear',
|
||||
warmup_iters=5,
|
||||
warmup_ratio=1e-4,
|
||||
warmup_by_epoch=True,
|
||||
by_epoch=False)
|
||||
|
||||
checkpoint_config = dict(interval=1)
|
||||
|
||||
log_config = dict(
|
||||
interval=10, hooks=[
|
||||
dict(type='TextLoggerHook'),
|
||||
])
|
||||
|
||||
eval_config = dict(initial=True, interval=1, gpu_collect=False)
|
||||
eval_pipelines = [
|
||||
dict(
|
||||
mode='test',
|
||||
dist_eval=False,
|
||||
evaluators=[dict(type='OCRRecEvaluator', ignore_space=False)],
|
||||
)
|
||||
]
|
|
@ -0,0 +1,145 @@
|
|||
_base_ = ['configs/base.py']
|
||||
|
||||
character_dict_path = 'http://pai-vision-data-hz.oss-cn-zhangjiakou.aliyuncs.com/EasyCV/modelzoo/ocr/dict/ppocr_keys_v1.txt'
|
||||
|
||||
model = dict(
|
||||
type='OCRRecNet',
|
||||
backbone=dict(
|
||||
type='OCRRecMobileNetV1Enhance',
|
||||
scale=0.5,
|
||||
last_conv_stride=[1, 2],
|
||||
last_pool_type='avg'),
|
||||
# neck=dict(
|
||||
# type='SequenceEncoder',
|
||||
# in_channels=512,
|
||||
# encoder_type='svtr',
|
||||
# dims=64,
|
||||
# depth=2,
|
||||
# hidden_dims=120,
|
||||
# use_guide=True),
|
||||
# head=dict(
|
||||
# type='CTCHead',
|
||||
# in_channels=64,
|
||||
# fc_decay=0.00001),
|
||||
head=dict(
|
||||
type='MultiHead',
|
||||
in_channels=512,
|
||||
out_channels_list=dict(
|
||||
CTCLabelDecode=6625,
|
||||
SARLabelDecode=6627,
|
||||
),
|
||||
head_list=[
|
||||
dict(
|
||||
type='CTCHead',
|
||||
Neck=dict(
|
||||
type='svtr',
|
||||
dims=64,
|
||||
depth=2,
|
||||
hidden_dims=120,
|
||||
use_guide=True),
|
||||
Head=dict(fc_decay=0.00001, )),
|
||||
dict(type='SARHead', enc_dim=512, max_text_length=25)
|
||||
]),
|
||||
postprocess=dict(
|
||||
type='CTCLabelDecode',
|
||||
character_dict_path=character_dict_path,
|
||||
use_space_char=True),
|
||||
loss=dict(
|
||||
type='MultiLoss',
|
||||
ignore_index=6626,
|
||||
loss_config_list=[
|
||||
dict(CTCLoss=None),
|
||||
dict(SARLoss=None),
|
||||
]),
|
||||
pretrained=
|
||||
'http://pai-vision-data-hz.oss-cn-zhangjiakou.aliyuncs.com/EasyCV/modelzoo/ocr/rec/ch_PP-OCRv3_rec/best_accuracy_student.pth'
|
||||
)
|
||||
|
||||
train_pipeline = [
|
||||
dict(type='RecConAug', prob=0.5, image_shape=(48, 320, 3)),
|
||||
dict(type='RecAug'),
|
||||
dict(
|
||||
type='MultiLabelEncode',
|
||||
max_text_length=25,
|
||||
use_space_char=True,
|
||||
character_dict_path=character_dict_path,
|
||||
),
|
||||
dict(type='RecResizeImg', image_shape=(3, 48, 320)),
|
||||
dict(type='MMToTensor'),
|
||||
dict(
|
||||
type='Collect',
|
||||
keys=['img', 'label_ctc', 'label_sar', 'length', 'valid_ratio'],
|
||||
meta_keys=['img_path'])
|
||||
]
|
||||
|
||||
val_pipeline = [
|
||||
dict(
|
||||
type='MultiLabelEncode',
|
||||
max_text_length=25,
|
||||
use_space_char=True,
|
||||
character_dict_path=character_dict_path,
|
||||
),
|
||||
dict(type='RecResizeImg', image_shape=(3, 48, 320)),
|
||||
dict(type='MMToTensor'),
|
||||
dict(
|
||||
type='Collect',
|
||||
keys=['img', 'label_ctc', 'label_sar', 'length', 'valid_ratio'],
|
||||
meta_keys=['img_path'])
|
||||
]
|
||||
|
||||
test_pipeline = [
|
||||
dict(type='RecResizeImg', image_shape=(3, 48, 320)),
|
||||
dict(type='MMToTensor'),
|
||||
dict(type='Collect', keys=['img'], meta_keys=['img_path'])
|
||||
]
|
||||
|
||||
train_dataset = dict(
|
||||
type='OCRRecDataset',
|
||||
data_source=dict(
|
||||
type='OCRRecSource',
|
||||
label_file='ocr/rec/pai/label_file/train.txt',
|
||||
data_dir='ocr/rec/pai/img/train',
|
||||
ext_data_num=2,
|
||||
),
|
||||
pipeline=train_pipeline)
|
||||
|
||||
val_dataset = dict(
|
||||
type='OCRRecDataset',
|
||||
data_source=dict(
|
||||
type='OCRRecSource',
|
||||
label_file='ocr/rec/pai/label_file/test.txt',
|
||||
data_dir='ocr/rec/pai/img/test',
|
||||
ext_data_num=0,
|
||||
),
|
||||
pipeline=val_pipeline)
|
||||
|
||||
data = dict(
|
||||
imgs_per_gpu=128, workers_per_gpu=4, train=train_dataset, val=val_dataset)
|
||||
|
||||
total_epochs = 10
|
||||
optimizer = dict(type='Adam', lr=0.001, betas=(0.9, 0.999))
|
||||
|
||||
lr_config = dict(
|
||||
policy='CosineAnnealing',
|
||||
min_lr=1e-5,
|
||||
warmup='linear',
|
||||
warmup_iters=5,
|
||||
warmup_ratio=1e-4,
|
||||
warmup_by_epoch=True,
|
||||
by_epoch=False)
|
||||
|
||||
checkpoint_config = dict(interval=1)
|
||||
|
||||
log_config = dict(
|
||||
interval=10, hooks=[
|
||||
dict(type='TextLoggerHook'),
|
||||
])
|
||||
|
||||
eval_config = dict(initial=True, interval=1, gpu_collect=False)
|
||||
eval_pipelines = [
|
||||
dict(
|
||||
mode='test',
|
||||
dist_eval=False,
|
||||
evaluators=[dict(type='OCRRecEvaluator', ignore_space=False)],
|
||||
)
|
||||
]
|
|
@ -0,0 +1,55 @@
|
|||
_base_ = ['configs/ocr/recognition/rec_model_ch.py']
|
||||
|
||||
character_dict_path = 'http://pai-vision-data-hz.oss-cn-zhangjiakou.aliyuncs.com/EasyCV/modelzoo/ocr/dict/chinese_cht_dict.txt'
|
||||
label_length = 8421
|
||||
model = dict(
|
||||
type='OCRRecNet',
|
||||
backbone=dict(
|
||||
type='OCRRecMobileNetV1Enhance',
|
||||
scale=0.5,
|
||||
last_conv_stride=[1, 2],
|
||||
last_pool_type='avg'),
|
||||
# inference
|
||||
# neck=dict(
|
||||
# type='SequenceEncoder',
|
||||
# in_channels=512,
|
||||
# encoder_type='svtr',
|
||||
# dims=64,
|
||||
# depth=2,
|
||||
# hidden_dims=120,
|
||||
# use_guide=True),
|
||||
# head=dict(
|
||||
# type='CTCHead',
|
||||
# in_channels=64,
|
||||
# fc_decay=0.00001),
|
||||
head=dict(
|
||||
type='MultiHead',
|
||||
in_channels=512,
|
||||
out_channels_list=dict(
|
||||
CTCLabelDecode=label_length + 2,
|
||||
SARLabelDecode=label_length + 4,
|
||||
),
|
||||
head_list=[
|
||||
dict(
|
||||
type='CTCHead',
|
||||
Neck=dict(
|
||||
type='svtr',
|
||||
dims=64,
|
||||
depth=2,
|
||||
hidden_dims=120,
|
||||
use_guide=True),
|
||||
Head=dict(fc_decay=0.00001, )),
|
||||
dict(type='SARHead', enc_dim=512, max_text_length=25)
|
||||
]),
|
||||
postprocess=dict(
|
||||
type='CTCLabelDecode',
|
||||
character_dict_path=character_dict_path,
|
||||
use_space_char=True),
|
||||
loss=dict(
|
||||
type='MultiLoss',
|
||||
ignore_index=label_length + 3,
|
||||
loss_config_list=[
|
||||
dict(CTCLoss=None),
|
||||
dict(SARLoss=None),
|
||||
]),
|
||||
pretrained=None)
|
|
@ -0,0 +1,55 @@
|
|||
_base_ = ['configs/ocr/recognition/rec_model_ch.py']
|
||||
|
||||
character_dict_path = 'http://pai-vision-data-hz.oss-cn-zhangjiakou.aliyuncs.com/EasyCV/modelzoo/ocr/dict/cyrillic_dict.txt'
|
||||
label_length = 163
|
||||
model = dict(
|
||||
type='OCRRecNet',
|
||||
backbone=dict(
|
||||
type='OCRRecMobileNetV1Enhance',
|
||||
scale=0.5,
|
||||
last_conv_stride=[1, 2],
|
||||
last_pool_type='avg'),
|
||||
# inference
|
||||
# neck=dict(
|
||||
# type='SequenceEncoder',
|
||||
# in_channels=512,
|
||||
# encoder_type='svtr',
|
||||
# dims=64,
|
||||
# depth=2,
|
||||
# hidden_dims=120,
|
||||
# use_guide=True),
|
||||
# head=dict(
|
||||
# type='CTCHead',
|
||||
# in_channels=64,
|
||||
# fc_decay=0.00001),
|
||||
head=dict(
|
||||
type='MultiHead',
|
||||
in_channels=512,
|
||||
out_channels_list=dict(
|
||||
CTCLabelDecode=label_length + 2,
|
||||
SARLabelDecode=label_length + 4,
|
||||
),
|
||||
head_list=[
|
||||
dict(
|
||||
type='CTCHead',
|
||||
Neck=dict(
|
||||
type='svtr',
|
||||
dims=64,
|
||||
depth=2,
|
||||
hidden_dims=120,
|
||||
use_guide=True),
|
||||
Head=dict(fc_decay=0.00001, )),
|
||||
dict(type='SARHead', enc_dim=512, max_text_length=25)
|
||||
]),
|
||||
postprocess=dict(
|
||||
type='CTCLabelDecode',
|
||||
character_dict_path=character_dict_path,
|
||||
use_space_char=True),
|
||||
loss=dict(
|
||||
type='MultiLoss',
|
||||
ignore_index=label_length + 3,
|
||||
loss_config_list=[
|
||||
dict(CTCLoss=None),
|
||||
dict(SARLoss=None),
|
||||
]),
|
||||
pretrained=None)
|
|
@ -0,0 +1,55 @@
|
|||
_base_ = ['configs/ocr/recognition/rec_model_ch.py']
|
||||
|
||||
character_dict_path = 'http://pai-vision-data-hz.oss-cn-zhangjiakou.aliyuncs.com/EasyCV/modelzoo/ocr/dict/devanagari_dict.txt'
|
||||
label_length = 167
|
||||
model = dict(
|
||||
type='OCRRecNet',
|
||||
backbone=dict(
|
||||
type='OCRRecMobileNetV1Enhance',
|
||||
scale=0.5,
|
||||
last_conv_stride=[1, 2],
|
||||
last_pool_type='avg'),
|
||||
# inference
|
||||
# neck=dict(
|
||||
# type='SequenceEncoder',
|
||||
# in_channels=512,
|
||||
# encoder_type='svtr',
|
||||
# dims=64,
|
||||
# depth=2,
|
||||
# hidden_dims=120,
|
||||
# use_guide=True),
|
||||
# head=dict(
|
||||
# type='CTCHead',
|
||||
# in_channels=64,
|
||||
# fc_decay=0.00001),
|
||||
head=dict(
|
||||
type='MultiHead',
|
||||
in_channels=512,
|
||||
out_channels_list=dict(
|
||||
CTCLabelDecode=label_length + 2,
|
||||
SARLabelDecode=label_length + 4,
|
||||
),
|
||||
head_list=[
|
||||
dict(
|
||||
type='CTCHead',
|
||||
Neck=dict(
|
||||
type='svtr',
|
||||
dims=64,
|
||||
depth=2,
|
||||
hidden_dims=120,
|
||||
use_guide=True),
|
||||
Head=dict(fc_decay=0.00001, )),
|
||||
dict(type='SARHead', enc_dim=512, max_text_length=25)
|
||||
]),
|
||||
postprocess=dict(
|
||||
type='CTCLabelDecode',
|
||||
character_dict_path=character_dict_path,
|
||||
use_space_char=True),
|
||||
loss=dict(
|
||||
type='MultiLoss',
|
||||
ignore_index=label_length + 3,
|
||||
loss_config_list=[
|
||||
dict(CTCLoss=None),
|
||||
dict(SARLoss=None),
|
||||
]),
|
||||
pretrained=None)
|
|
@ -0,0 +1,152 @@
|
|||
_base_ = ['configs/base.py']
|
||||
|
||||
# character_dict_path = 'http://pai-vision-data-hz.oss-cn-zhangjiakou.aliyuncs.com/EasyCV/modelzoo/ocr/dict/ic15_dict.txt'
|
||||
character_dict_path = 'http://pai-vision-data-hz.oss-cn-zhangjiakou.aliyuncs.com/EasyCV/modelzoo/ocr/dict/en_dict.txt'
|
||||
model = dict(
|
||||
type='OCRRecNet',
|
||||
backbone=dict(
|
||||
type='OCRRecMobileNetV1Enhance',
|
||||
scale=0.5,
|
||||
last_conv_stride=[1, 2],
|
||||
last_pool_type='avg'),
|
||||
# neck=dict(
|
||||
# type='SequenceEncoder',
|
||||
# in_channels=512,
|
||||
# encoder_type='svtr',
|
||||
# dims=64,
|
||||
# depth=2,
|
||||
# hidden_dims=120,
|
||||
# use_guide=True),
|
||||
# head=dict(
|
||||
# type='CTCHead',
|
||||
# in_channels=64,
|
||||
# fc_decay=0.00001),
|
||||
head=dict(
|
||||
type='MultiHead',
|
||||
in_channels=512,
|
||||
# out_channels_list=dict(
|
||||
# CTCLabelDecode=37,
|
||||
# SARLabelDecode=39,
|
||||
# ),
|
||||
out_channels_list=dict(
|
||||
CTCLabelDecode=97,
|
||||
SARLabelDecode=99,
|
||||
),
|
||||
head_list=[
|
||||
dict(
|
||||
type='CTCHead',
|
||||
Neck=dict(
|
||||
type='svtr',
|
||||
dims=64,
|
||||
depth=2,
|
||||
hidden_dims=120,
|
||||
use_guide=True),
|
||||
Head=dict(fc_decay=0.00001, )),
|
||||
dict(type='SARHead', enc_dim=512, max_text_length=25)
|
||||
]),
|
||||
postprocess=dict(
|
||||
type='CTCLabelDecode',
|
||||
character_dict_path=character_dict_path,
|
||||
use_space_char=False),
|
||||
loss=dict(
|
||||
type='MultiLoss',
|
||||
# ignore_index=38,
|
||||
ignore_index=98,
|
||||
loss_config_list=[
|
||||
dict(CTCLoss=None),
|
||||
dict(SARLoss=None),
|
||||
]),
|
||||
pretrained=
|
||||
'http://pai-vision-data-hz.oss-cn-zhangjiakou.aliyuncs.com/EasyCV/modelzoo/ocr/rec/en_PP-OCRv3_rec/best_accuracy.pth'
|
||||
)
|
||||
|
||||
train_pipeline = [
|
||||
dict(type='RecConAug', prob=0.5, image_shape=(48, 320, 3)),
|
||||
dict(type='RecAug'),
|
||||
dict(
|
||||
type='MultiLabelEncode',
|
||||
max_text_length=25,
|
||||
use_space_char=False,
|
||||
character_dict_path=character_dict_path),
|
||||
dict(type='RecResizeImg', image_shape=(3, 48, 320)),
|
||||
dict(type='MMToTensor'),
|
||||
dict(
|
||||
type='Collect',
|
||||
keys=['img', 'label_ctc', 'label_sar', 'length', 'valid_ratio'],
|
||||
meta_keys=['img_path'])
|
||||
]
|
||||
|
||||
val_pipeline = [
|
||||
dict(
|
||||
type='MultiLabelEncode',
|
||||
max_text_length=25,
|
||||
use_space_char=False,
|
||||
character_dict_path=character_dict_path),
|
||||
dict(type='RecResizeImg', image_shape=(3, 48, 320)),
|
||||
dict(type='MMToTensor'),
|
||||
dict(
|
||||
type='Collect',
|
||||
keys=['img', 'label_ctc', 'label_sar', 'length', 'valid_ratio'],
|
||||
meta_keys=['img_path'])
|
||||
]
|
||||
# test_pipeline = [
|
||||
# dict(type='OCRResizeNorm', img_shape=(48, 320)),
|
||||
# dict(type='ImageToTensor', keys=['img']),
|
||||
# dict(type='Collect', keys=['img']),
|
||||
# ]
|
||||
test_pipeline = [
|
||||
dict(type='RecResizeImg', image_shape=(3, 48, 320)),
|
||||
dict(type='MMToTensor'),
|
||||
dict(type='Collect', keys=['img'], meta_keys=['img_path'])
|
||||
]
|
||||
|
||||
train_dataset = dict(
|
||||
type='OCRRecDataset',
|
||||
data_source=dict(
|
||||
type='OCRReclmdbSource',
|
||||
data_dir='ocr/rec/DTRB/debug/data_lmdb_release/validation',
|
||||
ext_data_num=2,
|
||||
),
|
||||
pipeline=train_pipeline)
|
||||
|
||||
val_dataset = dict(
|
||||
type='OCRRecDataset',
|
||||
data_source=dict(
|
||||
type='OCRReclmdbSource',
|
||||
data_dir='ocr/rec/DTRB/debug/data_lmdb_release/validation',
|
||||
ext_data_num=0,
|
||||
test_mode=True,
|
||||
),
|
||||
pipeline=val_pipeline)
|
||||
|
||||
data = dict(
|
||||
imgs_per_gpu=256, workers_per_gpu=4, train=train_dataset, val=val_dataset)
|
||||
|
||||
total_epochs = 72
|
||||
|
||||
optimizer = dict(type='Adam', lr=0.0005, betas=(0.9, 0.999), weight_decay=0.0)
|
||||
|
||||
lr_config = dict(
|
||||
policy='CosineAnnealing',
|
||||
min_lr=1e-5,
|
||||
warmup='linear',
|
||||
warmup_iters=5,
|
||||
warmup_ratio=1e-4,
|
||||
warmup_by_epoch=True,
|
||||
by_epoch=False)
|
||||
|
||||
checkpoint_config = dict(interval=5)
|
||||
|
||||
log_config = dict(
|
||||
interval=100, hooks=[
|
||||
dict(type='TextLoggerHook'),
|
||||
])
|
||||
|
||||
eval_config = dict(initial=True, interval=1, gpu_collect=False)
|
||||
eval_pipelines = [
|
||||
dict(
|
||||
mode='test',
|
||||
dist_eval=False,
|
||||
evaluators=[dict(type='OCRRecEvaluator')],
|
||||
)
|
||||
]
|
|
@ -0,0 +1,55 @@
|
|||
_base_ = ['configs/ocr/recognition/rec_model_ch.py']
|
||||
|
||||
character_dict_path = 'http://pai-vision-data-hz.oss-cn-zhangjiakou.aliyuncs.com/EasyCV/modelzoo/ocr/dict/japan_dict.txt'
|
||||
label_length = 4399
|
||||
model = dict(
|
||||
type='OCRRecNet',
|
||||
backbone=dict(
|
||||
type='OCRRecMobileNetV1Enhance',
|
||||
scale=0.5,
|
||||
last_conv_stride=[1, 2],
|
||||
last_pool_type='avg'),
|
||||
# inference
|
||||
# neck=dict(
|
||||
# type='SequenceEncoder',
|
||||
# in_channels=512,
|
||||
# encoder_type='svtr',
|
||||
# dims=64,
|
||||
# depth=2,
|
||||
# hidden_dims=120,
|
||||
# use_guide=True),
|
||||
# head=dict(
|
||||
# type='CTCHead',
|
||||
# in_channels=64,
|
||||
# fc_decay=0.00001),
|
||||
head=dict(
|
||||
type='MultiHead',
|
||||
in_channels=512,
|
||||
out_channels_list=dict(
|
||||
CTCLabelDecode=label_length + 2,
|
||||
SARLabelDecode=label_length + 4,
|
||||
),
|
||||
head_list=[
|
||||
dict(
|
||||
type='CTCHead',
|
||||
Neck=dict(
|
||||
type='svtr',
|
||||
dims=64,
|
||||
depth=2,
|
||||
hidden_dims=120,
|
||||
use_guide=True),
|
||||
Head=dict(fc_decay=0.00001, )),
|
||||
dict(type='SARHead', enc_dim=512, max_text_length=25)
|
||||
]),
|
||||
postprocess=dict(
|
||||
type='CTCLabelDecode',
|
||||
character_dict_path=character_dict_path,
|
||||
use_space_char=True),
|
||||
loss=dict(
|
||||
type='MultiLoss',
|
||||
ignore_index=label_length + 3,
|
||||
loss_config_list=[
|
||||
dict(CTCLoss=None),
|
||||
dict(SARLoss=None),
|
||||
]),
|
||||
pretrained=None)
|
|
@ -0,0 +1,55 @@
|
|||
_base_ = ['configs/ocr/recognition/rec_model_ch.py']
|
||||
|
||||
character_dict_path = 'http://pai-vision-data-hz.oss-cn-zhangjiakou.aliyuncs.com/EasyCV/modelzoo/ocr/dict/ka_dict.txt'
|
||||
label_length = 153
|
||||
model = dict(
|
||||
type='OCRRecNet',
|
||||
backbone=dict(
|
||||
type='OCRRecMobileNetV1Enhance',
|
||||
scale=0.5,
|
||||
last_conv_stride=[1, 2],
|
||||
last_pool_type='avg'),
|
||||
# inference
|
||||
# neck=dict(
|
||||
# type='SequenceEncoder',
|
||||
# in_channels=512,
|
||||
# encoder_type='svtr',
|
||||
# dims=64,
|
||||
# depth=2,
|
||||
# hidden_dims=120,
|
||||
# use_guide=True),
|
||||
# head=dict(
|
||||
# type='CTCHead',
|
||||
# in_channels=64,
|
||||
# fc_decay=0.00001),
|
||||
head=dict(
|
||||
type='MultiHead',
|
||||
in_channels=512,
|
||||
out_channels_list=dict(
|
||||
CTCLabelDecode=label_length + 2,
|
||||
SARLabelDecode=label_length + 4,
|
||||
),
|
||||
head_list=[
|
||||
dict(
|
||||
type='CTCHead',
|
||||
Neck=dict(
|
||||
type='svtr',
|
||||
dims=64,
|
||||
depth=2,
|
||||
hidden_dims=120,
|
||||
use_guide=True),
|
||||
Head=dict(fc_decay=0.00001, )),
|
||||
dict(type='SARHead', enc_dim=512, max_text_length=25)
|
||||
]),
|
||||
postprocess=dict(
|
||||
type='CTCLabelDecode',
|
||||
character_dict_path=character_dict_path,
|
||||
use_space_char=True),
|
||||
loss=dict(
|
||||
type='MultiLoss',
|
||||
ignore_index=label_length + 3,
|
||||
loss_config_list=[
|
||||
dict(CTCLoss=None),
|
||||
dict(SARLoss=None),
|
||||
]),
|
||||
pretrained=None)
|
|
@ -0,0 +1,55 @@
|
|||
_base_ = ['configs/ocr/recognition/rec_model_ch.py']
|
||||
|
||||
character_dict_path = 'http://pai-vision-data-hz.oss-cn-zhangjiakou.aliyuncs.com/EasyCV/modelzoo/ocr/dict/korean_dict.txt'
|
||||
|
||||
model = dict(
|
||||
type='OCRRecNet',
|
||||
backbone=dict(
|
||||
type='OCRRecMobileNetV1Enhance',
|
||||
scale=0.5,
|
||||
last_conv_stride=[1, 2],
|
||||
last_pool_type='avg'),
|
||||
# inference
|
||||
# neck=dict(
|
||||
# type='SequenceEncoder',
|
||||
# in_channels=512,
|
||||
# encoder_type='svtr',
|
||||
# dims=64,
|
||||
# depth=2,
|
||||
# hidden_dims=120,
|
||||
# use_guide=True),
|
||||
# head=dict(
|
||||
# type='CTCHead',
|
||||
# in_channels=64,
|
||||
# fc_decay=0.00001),
|
||||
head=dict(
|
||||
type='MultiHead',
|
||||
in_channels=512,
|
||||
out_channels_list=dict(
|
||||
CTCLabelDecode=3690,
|
||||
SARLabelDecode=3692,
|
||||
),
|
||||
head_list=[
|
||||
dict(
|
||||
type='CTCHead',
|
||||
Neck=dict(
|
||||
type='svtr',
|
||||
dims=64,
|
||||
depth=2,
|
||||
hidden_dims=120,
|
||||
use_guide=True),
|
||||
Head=dict(fc_decay=0.00001, )),
|
||||
dict(type='SARHead', enc_dim=512, max_text_length=25)
|
||||
]),
|
||||
postprocess=dict(
|
||||
type='CTCLabelDecode',
|
||||
character_dict_path=character_dict_path,
|
||||
use_space_char=True),
|
||||
loss=dict(
|
||||
type='MultiLoss',
|
||||
ignore_index=3691,
|
||||
loss_config_list=[
|
||||
dict(CTCLoss=None),
|
||||
dict(SARLoss=None),
|
||||
]),
|
||||
pretrained=None)
|
|
@ -0,0 +1,55 @@
|
|||
_base_ = ['configs/ocr/recognition/rec_model_ch.py']
|
||||
|
||||
character_dict_path = 'http://pai-vision-data-hz.oss-cn-zhangjiakou.aliyuncs.com/EasyCV/modelzoo/ocr/dict/latin_dict.txt'
|
||||
label_length = 185
|
||||
model = dict(
|
||||
type='OCRRecNet',
|
||||
backbone=dict(
|
||||
type='OCRRecMobileNetV1Enhance',
|
||||
scale=0.5,
|
||||
last_conv_stride=[1, 2],
|
||||
last_pool_type='avg'),
|
||||
# inference
|
||||
# neck=dict(
|
||||
# type='SequenceEncoder',
|
||||
# in_channels=512,
|
||||
# encoder_type='svtr',
|
||||
# dims=64,
|
||||
# depth=2,
|
||||
# hidden_dims=120,
|
||||
# use_guide=True),
|
||||
# head=dict(
|
||||
# type='CTCHead',
|
||||
# in_channels=64,
|
||||
# fc_decay=0.00001),
|
||||
head=dict(
|
||||
type='MultiHead',
|
||||
in_channels=512,
|
||||
out_channels_list=dict(
|
||||
CTCLabelDecode=label_length + 2,
|
||||
SARLabelDecode=label_length + 4,
|
||||
),
|
||||
head_list=[
|
||||
dict(
|
||||
type='CTCHead',
|
||||
Neck=dict(
|
||||
type='svtr',
|
||||
dims=64,
|
||||
depth=2,
|
||||
hidden_dims=120,
|
||||
use_guide=True),
|
||||
Head=dict(fc_decay=0.00001, )),
|
||||
dict(type='SARHead', enc_dim=512, max_text_length=25)
|
||||
]),
|
||||
postprocess=dict(
|
||||
type='CTCLabelDecode',
|
||||
character_dict_path=character_dict_path,
|
||||
use_space_char=True),
|
||||
loss=dict(
|
||||
type='MultiLoss',
|
||||
ignore_index=label_length + 3,
|
||||
loss_config_list=[
|
||||
dict(CTCLoss=None),
|
||||
dict(SARLoss=None),
|
||||
]),
|
||||
pretrained=None)
|
|
@ -0,0 +1,55 @@
|
|||
_base_ = ['configs/ocr/recognition/rec_model_ch.py']
|
||||
|
||||
character_dict_path = 'http://pai-vision-data-hz.oss-cn-zhangjiakou.aliyuncs.com/EasyCV/modelzoo/ocr/dict/ta_dict.txt'
|
||||
label_length = 128
|
||||
model = dict(
|
||||
type='OCRRecNet',
|
||||
backbone=dict(
|
||||
type='OCRRecMobileNetV1Enhance',
|
||||
scale=0.5,
|
||||
last_conv_stride=[1, 2],
|
||||
last_pool_type='avg'),
|
||||
# inference
|
||||
# neck=dict(
|
||||
# type='SequenceEncoder',
|
||||
# in_channels=512,
|
||||
# encoder_type='svtr',
|
||||
# dims=64,
|
||||
# depth=2,
|
||||
# hidden_dims=120,
|
||||
# use_guide=True),
|
||||
# head=dict(
|
||||
# type='CTCHead',
|
||||
# in_channels=64,
|
||||
# fc_decay=0.00001),
|
||||
head=dict(
|
||||
type='MultiHead',
|
||||
in_channels=512,
|
||||
out_channels_list=dict(
|
||||
CTCLabelDecode=label_length + 2,
|
||||
SARLabelDecode=label_length + 4,
|
||||
),
|
||||
head_list=[
|
||||
dict(
|
||||
type='CTCHead',
|
||||
Neck=dict(
|
||||
type='svtr',
|
||||
dims=64,
|
||||
depth=2,
|
||||
hidden_dims=120,
|
||||
use_guide=True),
|
||||
Head=dict(fc_decay=0.00001, )),
|
||||
dict(type='SARHead', enc_dim=512, max_text_length=25)
|
||||
]),
|
||||
postprocess=dict(
|
||||
type='CTCLabelDecode',
|
||||
character_dict_path=character_dict_path,
|
||||
use_space_char=True),
|
||||
loss=dict(
|
||||
type='MultiLoss',
|
||||
ignore_index=label_length + 3,
|
||||
loss_config_list=[
|
||||
dict(CTCLoss=None),
|
||||
dict(SARLoss=None),
|
||||
]),
|
||||
pretrained=None)
|
|
@ -0,0 +1,55 @@
|
|||
_base_ = ['configs/ocr/recognition/rec_model_ch.py']
|
||||
|
||||
character_dict_path = 'http://pai-vision-data-hz.oss-cn-zhangjiakou.aliyuncs.com/EasyCV/modelzoo/ocr/dict/te_dict.txt'
|
||||
label_length = 151
|
||||
model = dict(
|
||||
type='OCRRecNet',
|
||||
backbone=dict(
|
||||
type='OCRRecMobileNetV1Enhance',
|
||||
scale=0.5,
|
||||
last_conv_stride=[1, 2],
|
||||
last_pool_type='avg'),
|
||||
# inference
|
||||
# neck=dict(
|
||||
# type='SequenceEncoder',
|
||||
# in_channels=512,
|
||||
# encoder_type='svtr',
|
||||
# dims=64,
|
||||
# depth=2,
|
||||
# hidden_dims=120,
|
||||
# use_guide=True),
|
||||
# head=dict(
|
||||
# type='CTCHead',
|
||||
# in_channels=64,
|
||||
# fc_decay=0.00001),
|
||||
head=dict(
|
||||
type='MultiHead',
|
||||
in_channels=512,
|
||||
out_channels_list=dict(
|
||||
CTCLabelDecode=label_length + 2,
|
||||
SARLabelDecode=label_length + 4,
|
||||
),
|
||||
head_list=[
|
||||
dict(
|
||||
type='CTCHead',
|
||||
Neck=dict(
|
||||
type='svtr',
|
||||
dims=64,
|
||||
depth=2,
|
||||
hidden_dims=120,
|
||||
use_guide=True),
|
||||
Head=dict(fc_decay=0.00001, )),
|
||||
dict(type='SARHead', enc_dim=512, max_text_length=25)
|
||||
]),
|
||||
postprocess=dict(
|
||||
type='CTCLabelDecode',
|
||||
character_dict_path=character_dict_path,
|
||||
use_space_char=True),
|
||||
loss=dict(
|
||||
type='MultiLoss',
|
||||
ignore_index=label_length + 3,
|
||||
loss_config_list=[
|
||||
dict(CTCLoss=None),
|
||||
dict(SARLoss=None),
|
||||
]),
|
||||
pretrained=None)
|
|
@ -7,6 +7,7 @@ from .face_eval import FaceKeypointEvaluator
|
|||
from .faceid_pair_eval import FaceIDPairEvaluator
|
||||
from .keypoint_eval import KeyPointEvaluator
|
||||
from .mse_eval import MSEEvaluator
|
||||
from .ocr_eval import OCRDetEvaluator, OCRRecEvaluator
|
||||
from .retrival_topk_eval import RetrivalTopKEvaluator
|
||||
from .segmentation_eval import SegmentationEvaluator
|
||||
from .top_down_eval import (keypoint_auc, keypoint_epe, keypoint_nme,
|
||||
|
|
|
@ -0,0 +1,272 @@
|
|||
# Modified from https://github.com/PaddlePaddle/PaddleOCR/tree/release/2.6/ppocr/metrics
|
||||
import string
|
||||
from collections import namedtuple
|
||||
|
||||
import numpy as np
|
||||
from rapidfuzz.distance import Levenshtein
|
||||
from shapely.geometry import Polygon
|
||||
|
||||
from .base_evaluator import Evaluator
|
||||
from .builder import EVALUATORS
|
||||
from .metric_registry import METRICS
|
||||
|
||||
|
||||
@EVALUATORS.register_module()
|
||||
class OCRDetEvaluator(Evaluator):
|
||||
|
||||
def __init__(self, dataset_name=None, metric_names=['hmean']):
|
||||
self.iou_constraint = 0.5
|
||||
self.area_precision_constraint = 0.5
|
||||
super().__init__(dataset_name, metric_names)
|
||||
|
||||
def _evaluate_impl(self, gt, pred):
|
||||
|
||||
def get_union(pD, pG):
|
||||
return Polygon(pD).union(Polygon(pG)).area
|
||||
|
||||
def get_intersection_over_union(pD, pG):
|
||||
return get_intersection(pD, pG) / get_union(pD, pG)
|
||||
|
||||
def get_intersection(pD, pG):
|
||||
return Polygon(pD).intersection(Polygon(pG)).area
|
||||
|
||||
def compute_ap(confList, matchList, numGtCare):
|
||||
correct = 0
|
||||
AP = 0
|
||||
if len(confList) > 0:
|
||||
confList = np.array(confList)
|
||||
matchList = np.array(matchList)
|
||||
sorted_ind = np.argsort(-confList)
|
||||
confList = confList[sorted_ind]
|
||||
matchList = matchList[sorted_ind]
|
||||
for n in range(len(confList)):
|
||||
match = matchList[n]
|
||||
if match:
|
||||
correct += 1
|
||||
AP += float(correct) / (n + 1)
|
||||
|
||||
if numGtCare > 0:
|
||||
AP /= numGtCare
|
||||
|
||||
return AP
|
||||
|
||||
perSampleMetrics = {}
|
||||
|
||||
matchedSum = 0
|
||||
|
||||
Rectangle = namedtuple('Rectangle', 'xmin ymin xmax ymax')
|
||||
|
||||
numGlobalCareGt = 0
|
||||
numGlobalCareDet = 0
|
||||
|
||||
arrGlobalConfidences = []
|
||||
arrGlobalMatches = []
|
||||
|
||||
recall = 0
|
||||
precision = 0
|
||||
hmean = 0
|
||||
|
||||
detMatched = 0
|
||||
|
||||
iouMat = np.empty([1, 1])
|
||||
|
||||
gtPols = []
|
||||
detPols = []
|
||||
|
||||
gtPolPoints = []
|
||||
detPolPoints = []
|
||||
|
||||
# Array of Ground Truth Polygons' keys marked as don't Care
|
||||
gtDontCarePolsNum = []
|
||||
# Array of Detected Polygons' matched with a don't Care GT
|
||||
detDontCarePolsNum = []
|
||||
|
||||
pairs = []
|
||||
detMatchedNums = []
|
||||
|
||||
arrSampleConfidences = []
|
||||
arrSampleMatch = []
|
||||
|
||||
evaluationLog = ''
|
||||
|
||||
for n in range(len(gt)):
|
||||
points = gt[n]['points']
|
||||
# transcription = gt[n]['text']
|
||||
dontCare = gt[n]['ignore']
|
||||
# points = Polygon(points)
|
||||
# points = points.buffer(0)
|
||||
if not Polygon(points).is_valid or not Polygon(points).is_simple:
|
||||
continue
|
||||
|
||||
gtPol = points
|
||||
gtPols.append(gtPol)
|
||||
gtPolPoints.append(points)
|
||||
if dontCare:
|
||||
gtDontCarePolsNum.append(len(gtPols) - 1)
|
||||
|
||||
evaluationLog += 'GT polygons: ' + str(len(gtPols)) + (
|
||||
' (' + str(len(gtDontCarePolsNum)) +
|
||||
" don't care)\n" if len(gtDontCarePolsNum) > 0 else '\n')
|
||||
|
||||
for n in range(len(pred)):
|
||||
points = pred[n]['points']
|
||||
# points = Polygon(points)
|
||||
# points = points.buffer(0)
|
||||
if not Polygon(points).is_valid or not Polygon(points).is_simple:
|
||||
continue
|
||||
|
||||
detPol = points
|
||||
detPols.append(detPol)
|
||||
detPolPoints.append(points)
|
||||
if len(gtDontCarePolsNum) > 0:
|
||||
for dontCarePol in gtDontCarePolsNum:
|
||||
dontCarePol = gtPols[dontCarePol]
|
||||
intersected_area = get_intersection(dontCarePol, detPol)
|
||||
pdDimensions = Polygon(detPol).area
|
||||
precision = 0 if pdDimensions == 0 else intersected_area / pdDimensions
|
||||
if (precision > self.area_precision_constraint):
|
||||
detDontCarePolsNum.append(len(detPols) - 1)
|
||||
break
|
||||
|
||||
evaluationLog += 'DET polygons: ' + str(len(detPols)) + (
|
||||
' (' + str(len(detDontCarePolsNum)) +
|
||||
" don't care)\n" if len(detDontCarePolsNum) > 0 else '\n')
|
||||
|
||||
if len(gtPols) > 0 and len(detPols) > 0:
|
||||
# Calculate IoU and precision matrixs
|
||||
outputShape = [len(gtPols), len(detPols)]
|
||||
iouMat = np.empty(outputShape)
|
||||
gtRectMat = np.zeros(len(gtPols), np.int8)
|
||||
detRectMat = np.zeros(len(detPols), np.int8)
|
||||
for gtNum in range(len(gtPols)):
|
||||
for detNum in range(len(detPols)):
|
||||
pG = gtPols[gtNum]
|
||||
pD = detPols[detNum]
|
||||
iouMat[gtNum, detNum] = get_intersection_over_union(pD, pG)
|
||||
|
||||
for gtNum in range(len(gtPols)):
|
||||
for detNum in range(len(detPols)):
|
||||
if gtRectMat[gtNum] == 0 and detRectMat[
|
||||
detNum] == 0 and gtNum not in gtDontCarePolsNum and detNum not in detDontCarePolsNum:
|
||||
if iouMat[gtNum, detNum] > self.iou_constraint:
|
||||
gtRectMat[gtNum] = 1
|
||||
detRectMat[detNum] = 1
|
||||
detMatched += 1
|
||||
pairs.append({'gt': gtNum, 'det': detNum})
|
||||
detMatchedNums.append(detNum)
|
||||
evaluationLog += 'Match GT #' + \
|
||||
str(gtNum) + ' with Det #' + str(detNum) + '\n'
|
||||
|
||||
numGtCare = (len(gtPols) - len(gtDontCarePolsNum))
|
||||
numDetCare = (len(detPols) - len(detDontCarePolsNum))
|
||||
if numGtCare == 0:
|
||||
recall = float(1)
|
||||
precision = float(0) if numDetCare > 0 else float(1)
|
||||
else:
|
||||
recall = float(detMatched) / numGtCare
|
||||
precision = 0 if numDetCare == 0 else float(
|
||||
detMatched) / numDetCare
|
||||
|
||||
hmean = 0 if (precision +
|
||||
recall) == 0 else 2.0 * precision * recall / (
|
||||
precision + recall)
|
||||
|
||||
matchedSum += detMatched
|
||||
numGlobalCareGt += numGtCare
|
||||
numGlobalCareDet += numDetCare
|
||||
|
||||
perSampleMetrics = {
|
||||
'gtCare': numGtCare,
|
||||
'detCare': numDetCare,
|
||||
'detMatched': detMatched,
|
||||
}
|
||||
return perSampleMetrics
|
||||
|
||||
def combine_results(self, results):
|
||||
numGlobalCareGt = 0
|
||||
numGlobalCareDet = 0
|
||||
matchedSum = 0
|
||||
for result in results:
|
||||
numGlobalCareGt += result['gtCare']
|
||||
numGlobalCareDet += result['detCare']
|
||||
matchedSum += result['detMatched']
|
||||
|
||||
methodRecall = 0 if numGlobalCareGt == 0 else float(
|
||||
matchedSum) / numGlobalCareGt
|
||||
methodPrecision = 0 if numGlobalCareDet == 0 else float(
|
||||
matchedSum) / numGlobalCareDet
|
||||
methodHmean = 0 if methodRecall + methodPrecision == 0 else 2 * methodRecall * methodPrecision / (
|
||||
methodRecall + methodPrecision)
|
||||
# print(methodRecall, methodPrecision, methodHmean)
|
||||
# sys.exit(-1)
|
||||
methodMetrics = {
|
||||
'precision': methodPrecision,
|
||||
'recall': methodRecall,
|
||||
'hmean': methodHmean
|
||||
}
|
||||
|
||||
return methodMetrics
|
||||
|
||||
def evaluate(self, preds, gt_polyons_batch, ignore_tags_batch, **kwargs):
|
||||
results = []
|
||||
for pred, gt_polyons, ignore_tags in zip(preds, gt_polyons_batch,
|
||||
ignore_tags_batch):
|
||||
# prepare gt
|
||||
gt_info_list = [{
|
||||
'points': gt_polyon,
|
||||
'text': '',
|
||||
'ignore': ignore_tag
|
||||
} for gt_polyon, ignore_tag in zip(gt_polyons, ignore_tags)]
|
||||
# prepare det
|
||||
det_info_list = [{
|
||||
'points': det_polyon,
|
||||
'text': ''
|
||||
} for det_polyon in pred]
|
||||
result = self._evaluate_impl(gt_info_list, det_info_list)
|
||||
results.append(result)
|
||||
results = self.combine_results(results)
|
||||
return results
|
||||
|
||||
|
||||
@EVALUATORS.register_module()
|
||||
class OCRRecEvaluator(Evaluator):
|
||||
|
||||
def __init__(self,
|
||||
is_filter=False,
|
||||
ignore_space=True,
|
||||
dataset_name=None,
|
||||
metric_names=['acc']):
|
||||
super().__init__(dataset_name, metric_names)
|
||||
self.is_filter = is_filter
|
||||
self.ignore_space = ignore_space
|
||||
self.eps = 1e-5
|
||||
|
||||
def _normalize_text(self, text):
|
||||
text = ''.join(
|
||||
filter(lambda x: x in (string.digits + string.ascii_letters),
|
||||
text))
|
||||
return text.lower()
|
||||
|
||||
def _evaluate_impl(self, preds, labels, **kwargs):
|
||||
correct_num = 0
|
||||
all_num = 0
|
||||
norm_edit_dis = 0.0
|
||||
for (pred, pred_conf), (target, _) in zip(preds, labels):
|
||||
if self.ignore_space:
|
||||
pred = pred.replace(' ', '')
|
||||
target = target.replace(' ', '')
|
||||
if self.is_filter:
|
||||
pred = self._normalize_text(pred)
|
||||
target = self._normalize_text(target)
|
||||
norm_edit_dis += Levenshtein.normalized_distance(pred, target)
|
||||
if pred == target:
|
||||
correct_num += 1
|
||||
all_num += 1
|
||||
return {
|
||||
'acc': correct_num / (all_num + self.eps),
|
||||
'norm_edit_dis': 1 - norm_edit_dis / (all_num + self.eps)
|
||||
}
|
||||
|
||||
|
||||
METRICS.register_default_best_metric(OCRDetEvaluator, 'hmean', 'max')
|
||||
METRICS.register_default_best_metric(OCRRecEvaluator, 'acc', 'max')
|
|
@ -1,6 +1,6 @@
|
|||
# Copyright (c) Alibaba, Inc. and its affiliates.
|
||||
from . import (classification, detection, face, pose, segmentation, selfsup,
|
||||
shared)
|
||||
from . import (classification, detection, face, ocr, pose, segmentation,
|
||||
selfsup, shared)
|
||||
from .builder import build_dali_dataset, build_dataset
|
||||
from .loader import DistributedGroupSampler, GroupSampler, build_dataloader
|
||||
from .registry import DATASETS
|
||||
|
|
|
@ -0,0 +1,5 @@
|
|||
# Copyright (c) Alibaba, Inc. and its affiliates.
|
||||
from . import data_sources, pipelines # pylint: disable=unused-import
|
||||
from .ocr_cls_dataset import OCRClsDataset
|
||||
from .ocr_det_dataset import OCRDetDataset
|
||||
from .ocr_rec_dataset import OCRRecDataset
|
|
@ -0,0 +1,4 @@
|
|||
# Copyright (c) Alibaba, Inc. and its affiliates.
|
||||
from .ocr_cls_datasource import OCRClsSource
|
||||
from .ocr_det_datasource import OCRDetSource, OCRPaiDetSource
|
||||
from .ocr_rec_datasource import OCRReclmdbSource, OCRRecSource
|
|
@ -0,0 +1,39 @@
|
|||
# Copyright (c) Alibaba, Inc. and its affiliates.
|
||||
from easycv.datasets.ocr.data_sources.ocr_det_datasource import OCRDetSource
|
||||
from easycv.datasets.registry import DATASOURCES
|
||||
|
||||
|
||||
@DATASOURCES.register_module()
|
||||
class OCRClsSource(OCRDetSource):
|
||||
"""ocr direction classification data source
|
||||
"""
|
||||
|
||||
def __init__(self,
|
||||
label_file,
|
||||
data_dir='',
|
||||
test_mode=False,
|
||||
delimiter='\t',
|
||||
label_list=['0', '180']):
|
||||
"""
|
||||
|
||||
Args:
|
||||
label_file (str): path of label file
|
||||
data_dir (str, optional): folder of imgge data. Defaults to ''.
|
||||
test_mode (bool, optional): whether train or test. Defaults to False.
|
||||
delimiter (str, optional): delimiter used to separate elements in each row. Defaults to '\t'.
|
||||
label_list (list, optional): Identifiable directional Angle. Defaults to ['0', '180'].
|
||||
"""
|
||||
super(OCRClsSource, self).__init__(
|
||||
label_file,
|
||||
data_dir=data_dir,
|
||||
test_mode=test_mode,
|
||||
delimiter=delimiter)
|
||||
self.label_list = label_list
|
||||
|
||||
def label_encode(self, data):
|
||||
label = data['label']
|
||||
if label not in self.label_list:
|
||||
return None
|
||||
label = self.label_list.index(label)
|
||||
data['label'] = label
|
||||
return data
|
|
@ -0,0 +1,174 @@
|
|||
# Copyright (c) Alibaba, Inc. and its affiliates.
|
||||
import csv
|
||||
import json
|
||||
import logging
|
||||
import os
|
||||
import traceback
|
||||
|
||||
import numpy as np
|
||||
|
||||
from easycv.datasets.registry import DATASOURCES
|
||||
from easycv.file.image import load_image
|
||||
|
||||
IGNORE_TAGS = ['*', '###']
|
||||
|
||||
|
||||
@DATASOURCES.register_module()
|
||||
class OCRDetSource(object):
|
||||
"""ocr det data source
|
||||
"""
|
||||
|
||||
def __init__(self,
|
||||
label_file,
|
||||
data_dir='',
|
||||
test_mode=False,
|
||||
delimiter='\t'):
|
||||
"""
|
||||
|
||||
Args:
|
||||
label_file (str): path of label file
|
||||
data_dir (str, optional): folder of imgge data. Defaults to ''.
|
||||
test_mode (bool, optional): whether train or test. Defaults to False.
|
||||
delimiter (str, optional): delimiter used to separate elements in each row. Defaults to '\t'.
|
||||
"""
|
||||
self.data_dir = data_dir
|
||||
self.delimiter = delimiter
|
||||
self.test_mode = test_mode
|
||||
self.data_lines = self.get_image_info_list(label_file)
|
||||
|
||||
def get_image_info_list(self, label_file):
|
||||
data_lines = []
|
||||
with open(label_file, 'rb') as f:
|
||||
lines = f.readlines()
|
||||
data_lines.extend(lines)
|
||||
return data_lines
|
||||
|
||||
def expand_points_num(self, boxes):
|
||||
max_points_num = 0
|
||||
for box in boxes:
|
||||
if len(box) > max_points_num:
|
||||
max_points_num = len(box)
|
||||
ex_boxes = []
|
||||
for box in boxes:
|
||||
ex_box = box + [box[-1]] * (max_points_num - len(box))
|
||||
ex_boxes.append(ex_box)
|
||||
return ex_boxes
|
||||
|
||||
def label_encode(self, data):
|
||||
label = data['label']
|
||||
label = json.loads(label)
|
||||
nBox = len(label)
|
||||
boxes, txts, txt_tags = [], [], []
|
||||
for bno in range(nBox):
|
||||
box = label[bno]['points']
|
||||
txt = label[bno]['transcription']
|
||||
boxes.append(box)
|
||||
txts.append(txt)
|
||||
if txt in IGNORE_TAGS:
|
||||
txt_tags.append(True)
|
||||
else:
|
||||
txt_tags.append(False)
|
||||
if len(boxes) == 0:
|
||||
return None
|
||||
boxes = self.expand_points_num(boxes)
|
||||
boxes = np.array(boxes, dtype=np.float32)
|
||||
txt_tags = np.array(txt_tags, dtype=np.bool)
|
||||
|
||||
data['polys'] = boxes
|
||||
data['texts'] = txts
|
||||
data['ignore_tags'] = txt_tags
|
||||
return data
|
||||
|
||||
def parse(self, data_line):
|
||||
|
||||
data_line = data_line.decode('utf-8')
|
||||
substr = data_line.strip('\n').split(self.delimiter)
|
||||
file_name = substr[0]
|
||||
label = substr[1]
|
||||
|
||||
return file_name, label
|
||||
|
||||
def __getitem__(self, idx):
|
||||
data_line = self.data_lines[idx]
|
||||
try:
|
||||
file_name, label = self.parse(data_line)
|
||||
img_path = os.path.join(self.data_dir, file_name)
|
||||
data = {'img_path': img_path, 'label': label}
|
||||
if not os.path.exists(img_path):
|
||||
raise Exception('{} does not exist!'.format(img_path))
|
||||
|
||||
img = load_image(img_path, mode='BGR')
|
||||
data['img'] = img.astype(np.float32)
|
||||
data['ori_img_shape'] = img.shape
|
||||
outs = self.label_encode(data)
|
||||
except:
|
||||
logging.error(
|
||||
'When parsing line {}, error happened with msg: {}'.format(
|
||||
data_line, traceback.format_exc()))
|
||||
outs = None
|
||||
if outs is None:
|
||||
rnd_idx = np.random.randint(
|
||||
len(self)) if not self.test_mode else (idx + 1) % len(self)
|
||||
return self[rnd_idx]
|
||||
return outs
|
||||
|
||||
def __len__(self):
|
||||
return len(self.data_lines)
|
||||
|
||||
|
||||
@DATASOURCES.register_module()
|
||||
class OCRPaiDetSource(OCRDetSource):
|
||||
"""ocr det data source for pai format
|
||||
"""
|
||||
|
||||
def __init__(self, label_file, data_dir='', test_mode=False):
|
||||
"""
|
||||
|
||||
Args:
|
||||
label_file (str or list[str]): path of label file
|
||||
data_dir (str, optional): folder of imgge data. Defaults to ''.
|
||||
test_mode (bool, optional): whether train or test. Defaults to False.
|
||||
"""
|
||||
super(OCRPaiDetSource, self).__init__(
|
||||
label_file, data_dir=data_dir, test_mode=test_mode)
|
||||
|
||||
def get_image_info_list(self, label_file):
|
||||
data_lines = []
|
||||
if type(label_file) == list:
|
||||
for file in label_file:
|
||||
data_lines += list(csv.reader(open(file)))[1:]
|
||||
else:
|
||||
data_lines = list(csv.reader(open(label_file)))[1:]
|
||||
return data_lines
|
||||
|
||||
def label_encode(self, data):
|
||||
label = data['label']
|
||||
nBox = len(label)
|
||||
boxes, txts, txt_tags = [], [], []
|
||||
for bno in range(nBox):
|
||||
box = label[bno]['coord']
|
||||
box = [int(float(pos)) for pos in box]
|
||||
box = [box[idx:idx + 2] for idx in range(0, 8, 2)]
|
||||
txt = json.loads(label[bno]['text'])['text']
|
||||
boxes.append(box)
|
||||
txts.append(txt)
|
||||
if txt in IGNORE_TAGS:
|
||||
txt_tags.append(True)
|
||||
else:
|
||||
txt_tags.append(False)
|
||||
if len(boxes) == 0:
|
||||
return None
|
||||
boxes = self.expand_points_num(boxes)
|
||||
boxes = np.array(boxes, dtype=np.float32)
|
||||
txt_tags = np.array(txt_tags, dtype=np.bool)
|
||||
data['polys'] = boxes
|
||||
data['texts'] = txts
|
||||
data['ignore_tags'] = txt_tags
|
||||
return data
|
||||
|
||||
def parse(self, data_line):
|
||||
|
||||
file_name = json.loads(data_line[1])['tfspath'].split('/')[-1]
|
||||
label = json.loads(data_line[2])[0]
|
||||
|
||||
return file_name, label
|
|
@ -0,0 +1,178 @@
|
|||
# Copyright (c) Alibaba, Inc. and its affiliates.
|
||||
import logging
|
||||
import os
|
||||
import traceback
|
||||
|
||||
import cv2
|
||||
import lmdb
|
||||
import numpy as np
|
||||
|
||||
from easycv.datasets.registry import DATASOURCES
|
||||
from easycv.file.image import load_image
|
||||
|
||||
|
||||
@DATASOURCES.register_module()
|
||||
class OCRRecSource(object):
|
||||
"""ocr rec data source
|
||||
"""
|
||||
|
||||
def __init__(self,
|
||||
label_file,
|
||||
data_dir='',
|
||||
ext_data_num=0,
|
||||
test_mode=False,
|
||||
delimiter='\t'):
|
||||
"""
|
||||
|
||||
Args:
|
||||
label_file (str): path of label file
|
||||
data_dir (str, optional): folder of imgge data. Defaults to ''.
|
||||
ext_data_num (int): number of additional data used for augmentation. Defaults to 0.
|
||||
test_mode (bool, optional): whether train or test. Defaults to False.
|
||||
delimiter (str, optional): delimiter used to separate elements in each row. Defaults to '\t'.
|
||||
"""
|
||||
self.data_dir = data_dir
|
||||
self.delimiter = delimiter
|
||||
self.test_mode = test_mode
|
||||
self.ext_data_num = ext_data_num
|
||||
self.data_lines = self.get_image_info_list(label_file)
|
||||
|
||||
def get_image_info_list(self, label_file):
|
||||
data_lines = []
|
||||
with open(label_file, 'rb') as f:
|
||||
lines = f.readlines()
|
||||
data_lines.extend(lines)
|
||||
return data_lines
|
||||
|
||||
def __getitem__(self, idx, get_ext=True):
|
||||
data_line = self.data_lines[idx]
|
||||
try:
|
||||
data_line = data_line.decode('utf-8')
|
||||
substr = data_line.strip('\n').split(self.delimiter)
|
||||
file_name = substr[0]
|
||||
label = substr[1]
|
||||
img_path = os.path.join(self.data_dir, file_name)
|
||||
outs = {'img_path': img_path, 'label': label}
|
||||
if not os.path.exists(img_path):
|
||||
raise Exception('{} does not exist!'.format(img_path))
|
||||
img = load_image(img_path, mode='BGR')
|
||||
outs['img'] = img.astype(np.float32)
|
||||
outs['ori_img_shape'] = img.shape
|
||||
if get_ext:
|
||||
outs['ext_data'] = self.get_ext_data()
|
||||
return outs
|
||||
except:
|
||||
logging.error(
|
||||
'When parsing line {}, error happened with msg: {}'.format(
|
||||
data_line, traceback.format_exc()))
|
||||
outs = None
|
||||
if outs is None:
|
||||
rnd_idx = np.random.randint(self.__len__(
|
||||
)) if not self.test_mode else (idx + 1) % self.__len__()
|
||||
return self.__getitem__(rnd_idx)
|
||||
|
||||
def __len__(self):
|
||||
return len(self.data_lines)
|
||||
|
||||
def get_ext_data(self):
|
||||
ext_data = []
|
||||
|
||||
while len(ext_data) < self.ext_data_num:
|
||||
data = self.__getitem__(
|
||||
np.random.randint(self.__len__()), get_ext=False)
|
||||
ext_data.append(data)
|
||||
return ext_data
|
||||
|
||||
|
||||
@DATASOURCES.register_module(force=True)
|
||||
class OCRReclmdbSource(object):
|
||||
"""ocr rec lmdb data source specific for DTRB dataset
|
||||
"""
|
||||
|
||||
def __init__(self, data_dir='', ext_data_num=0, test_mode=False):
|
||||
self.test_mode = test_mode
|
||||
self.ext_data_num = ext_data_num
|
||||
self.lmdb_sets = self.load_hierarchical_lmdb_dataset(data_dir)
|
||||
logging.info('Initialize indexs of datasets:%s' % data_dir)
|
||||
self.data_idx_order_list = self.dataset_traversal()
|
||||
|
||||
def load_hierarchical_lmdb_dataset(self, data_dir):
|
||||
lmdb_sets = {}
|
||||
dataset_idx = 0
|
||||
for dirpath, dirnames, filenames in os.walk(data_dir + '/'):
|
||||
if not dirnames:
|
||||
env = lmdb.open(
|
||||
dirpath,
|
||||
max_readers=32,
|
||||
readonly=True,
|
||||
lock=False,
|
||||
readahead=False,
|
||||
meminit=False)
|
||||
txn = env.begin(write=False)
|
||||
num_samples = int(txn.get('num-samples'.encode()))
|
||||
lmdb_sets[dataset_idx] = {
|
||||
'dirpath': dirpath,
|
||||
'env': env,
|
||||
'txn': txn,
|
||||
'num_samples': num_samples
|
||||
}
|
||||
dataset_idx += 1
|
||||
return lmdb_sets
|
||||
|
||||
def dataset_traversal(self):
|
||||
lmdb_num = len(self.lmdb_sets)
|
||||
total_sample_num = 0
|
||||
for lno in range(lmdb_num):
|
||||
total_sample_num += self.lmdb_sets[lno]['num_samples']
|
||||
data_idx_order_list = np.zeros((total_sample_num, 2))
|
||||
beg_idx = 0
|
||||
for lno in range(lmdb_num):
|
||||
tmp_sample_num = self.lmdb_sets[lno]['num_samples']
|
||||
end_idx = beg_idx + tmp_sample_num
|
||||
data_idx_order_list[beg_idx:end_idx, 0] = lno
|
||||
data_idx_order_list[beg_idx:end_idx, 1] \
|
||||
= list(range(tmp_sample_num))
|
||||
data_idx_order_list[beg_idx:end_idx, 1] += 1
|
||||
beg_idx = beg_idx + tmp_sample_num
|
||||
return data_idx_order_list
|
||||
|
||||
def get_lmdb_sample_info(self, txn, index):
|
||||
label_key = 'label-%09d'.encode() % index
|
||||
label = txn.get(label_key)
|
||||
if label is None:
|
||||
return None
|
||||
label = label.decode('utf-8')
|
||||
img_key = 'image-%09d'.encode() % index
|
||||
imgbuf = txn.get(img_key)
|
||||
return imgbuf, label
|
||||
|
||||
def __getitem__(self, idx, get_ext=True):
|
||||
lmdb_idx, file_idx = self.data_idx_order_list[idx]
|
||||
lmdb_idx = int(lmdb_idx)
|
||||
file_idx = int(file_idx)
|
||||
sample_info = self.get_lmdb_sample_info(
|
||||
self.lmdb_sets[lmdb_idx]['txn'], file_idx)
|
||||
if sample_info is None:
|
||||
rnd_idx = np.random.randint(self.__len__(
|
||||
)) if not self.test_mode else (idx + 1) % self.__len__()
|
||||
return self.__getitem__(rnd_idx)
|
||||
img, label = sample_info
|
||||
img = cv2.imdecode(np.frombuffer(img, dtype='uint8'), 1)
|
||||
outs = {'img_path': '', 'label': label}
|
||||
outs['img'] = img.astype(np.float32)
|
||||
outs['ori_img_shape'] = img.shape
|
||||
if get_ext:
|
||||
outs['ext_data'] = self.get_ext_data()
|
||||
return outs
|
||||
|
||||
def get_ext_data(self):
|
||||
ext_data = []
|
||||
|
||||
while len(ext_data) < self.ext_data_num:
|
||||
data = self.__getitem__(
|
||||
np.random.randint(self.__len__()), get_ext=False)
|
||||
ext_data.append(data)
|
||||
return ext_data
|
||||
|
||||
def __len__(self):
|
||||
return self.data_idx_order_list.shape[0]
|
|
@ -0,0 +1,21 @@
|
|||
# Copyright (c) Alibaba, Inc. and its affiliates.
|
||||
from easycv.datasets.registry import DATASETS
|
||||
from .ocr_raw_dataset import OCRRawDataset
|
||||
|
||||
|
||||
@DATASETS.register_module(force=True)
|
||||
class OCRClsDataset(OCRRawDataset):
|
||||
"""Dataset for ocr text classification
|
||||
"""
|
||||
|
||||
def __init__(self, data_source, pipeline, profiling=False):
|
||||
super(OCRRawDataset, self).__init__(
|
||||
data_source, pipeline, profiling=profiling)
|
||||
|
||||
def evaluate(self, results, evaluators, logger=None, **kwargs):
|
||||
assert len(evaluators) == 1, \
|
||||
'classification evaluation only support one evaluator'
|
||||
gt_labels = results.pop('label')
|
||||
eval_res = evaluators[0].evaluate(results, gt_labels)
|
||||
|
||||
return eval_res
|
|
@ -0,0 +1,28 @@
|
|||
# Copyright (c) Alibaba, Inc. and its affiliates.
|
||||
import logging
|
||||
import traceback
|
||||
|
||||
import numpy as np
|
||||
|
||||
from easycv.datasets.registry import DATASETS
|
||||
from .ocr_raw_dataset import OCRRawDataset
|
||||
|
||||
|
||||
@DATASETS.register_module()
|
||||
class OCRDetDataset(OCRRawDataset):
|
||||
"""Dataset for ocr text detection
|
||||
"""
|
||||
|
||||
def __init__(self, data_source, pipeline, profiling=False):
|
||||
super(OCRDetDataset, self).__init__(
|
||||
data_source, pipeline, profiling=profiling)
|
||||
|
||||
def evaluate(self, results, evaluators, logger=None, **kwargs):
|
||||
assert len(evaluators) == 1, \
|
||||
'ocrdet evaluation only support one evaluator'
|
||||
points = results.pop('points')
|
||||
ignore_tags = results.pop('ignore_tags')
|
||||
polys = results.pop('polys')
|
||||
eval_res = evaluators[0].evaluate(points, polys, ignore_tags)
|
||||
|
||||
return eval_res
|
|
@ -0,0 +1,36 @@
|
|||
# Copyright (c) Alibaba, Inc. and its affiliates.
|
||||
import logging
|
||||
import traceback
|
||||
|
||||
import numpy as np
|
||||
|
||||
from easycv.datasets.shared.base import BaseDataset
|
||||
|
||||
|
||||
class OCRRawDataset(BaseDataset):
|
||||
"""Dataset for ocr
|
||||
"""
|
||||
|
||||
def __init__(self, data_source, pipeline, profiling=False):
|
||||
super(OCRRawDataset, self).__init__(
|
||||
data_source, pipeline, profiling=profiling)
|
||||
|
||||
def __len__(self):
|
||||
return len(self.data_source)
|
||||
|
||||
def __getitem__(self, idx):
|
||||
try:
|
||||
data_dict = self.data_source[idx]
|
||||
data_dict = self.pipeline(data_dict)
|
||||
except:
|
||||
logging.error(
|
||||
'When parsing line {}, error happened with msg: {}'.format(
|
||||
idx, traceback.format_exc()))
|
||||
data_dict = None
|
||||
if data_dict is None:
|
||||
rnd_idx = np.random.randint(self.__len__())
|
||||
return self.__getitem__(rnd_idx)
|
||||
return data_dict
|
||||
|
||||
def evaluate(self, results, evaluators, logger=None, **kwargs):
|
||||
pass
|
|
@ -0,0 +1,22 @@
|
|||
# Copyright (c) Alibaba, Inc. and its affiliates.
|
||||
from easycv.datasets.registry import DATASETS
|
||||
from .ocr_raw_dataset import OCRRawDataset
|
||||
|
||||
|
||||
@DATASETS.register_module()
|
||||
class OCRRecDataset(OCRRawDataset):
|
||||
"""Dataset for ocr text recognition
|
||||
"""
|
||||
|
||||
def __init__(self, data_source, pipeline, profiling=False):
|
||||
super(OCRRecDataset, self).__init__(
|
||||
data_source, pipeline, profiling=profiling)
|
||||
|
||||
def evaluate(self, results, evaluators, logger=None, **kwargs):
|
||||
assert len(evaluators) == 1, \
|
||||
'ocrrec evaluation only support one evaluator'
|
||||
preds_text = results.pop('preds_text')
|
||||
label_text = results.pop('label_text')
|
||||
eval_res = evaluators[0].evaluate(preds_text, label_text)
|
||||
|
||||
return eval_res
|
|
@ -0,0 +1,5 @@
|
|||
# Copyright (c) Alibaba, Inc. and its affiliates.
|
||||
from .det_transform import (EastRandomCropData, IaaAugment, MakeBorderMap,
|
||||
MakeShrinkMap, OCRDetResize)
|
||||
from .label_ops import CTCLabelEncode, MultiLabelEncode, SARLabelEncode
|
||||
from .rec_transform import ClsResizeImg, RecAug, RecConAug, RecResizeImg
|
|
@ -0,0 +1,624 @@
|
|||
# Modified from https://github.com/PaddlePaddle/PaddleOCR/tree/release/2.6/ppocr/data/imaug
|
||||
import math
|
||||
import random
|
||||
import sys
|
||||
|
||||
import cv2
|
||||
import imgaug
|
||||
import imgaug.augmenters as iaa
|
||||
import numpy as np
|
||||
import pyclipper
|
||||
from shapely.geometry import Polygon
|
||||
|
||||
from easycv.datasets.registry import PIPELINES
|
||||
from easycv.framework.errors import RuntimeError
|
||||
|
||||
|
||||
class AugmenterBuilder(object):
|
||||
|
||||
def __init__(self):
|
||||
pass
|
||||
|
||||
def build(self, args, root=True):
|
||||
if args is None or len(args) == 0:
|
||||
return None
|
||||
elif isinstance(args, list):
|
||||
if root:
|
||||
sequence = [self.build(value, root=False) for value in args]
|
||||
return iaa.Sequential(sequence)
|
||||
else:
|
||||
return getattr(
|
||||
iaa,
|
||||
args[0])(*[self.to_tuple_if_list(a) for a in args[1:]])
|
||||
elif isinstance(args, dict):
|
||||
cls = getattr(iaa, args['type'])
|
||||
return cls(
|
||||
**
|
||||
{k: self.to_tuple_if_list(v)
|
||||
for k, v in args['args'].items()})
|
||||
else:
|
||||
raise RuntimeError('unknown augmenter arg: ' + str(args))
|
||||
|
||||
def to_tuple_if_list(self, obj):
|
||||
if isinstance(obj, list):
|
||||
return tuple(obj)
|
||||
return obj
|
||||
|
||||
|
||||
@PIPELINES.register_module()
|
||||
class IaaAugment():
|
||||
|
||||
def __init__(self, augmenter_args=None, **kwargs):
|
||||
if augmenter_args is None:
|
||||
augmenter_args = [{
|
||||
'type': 'Fliplr',
|
||||
'args': {
|
||||
'p': 0.5
|
||||
}
|
||||
}, {
|
||||
'type': 'Affine',
|
||||
'args': {
|
||||
'rotate': [-10, 10]
|
||||
}
|
||||
}, {
|
||||
'type': 'Resize',
|
||||
'args': {
|
||||
'size': [0.5, 3]
|
||||
}
|
||||
}]
|
||||
self.augmenter = AugmenterBuilder().build(augmenter_args)
|
||||
|
||||
def __call__(self, data):
|
||||
image = data['img']
|
||||
shape = image.shape
|
||||
|
||||
if self.augmenter:
|
||||
aug = self.augmenter.to_deterministic()
|
||||
data['img'] = aug.augment_image(image)
|
||||
data = self.may_augment_annotation(aug, data, shape)
|
||||
return data
|
||||
|
||||
def may_augment_annotation(self, aug, data, shape):
|
||||
if aug is None:
|
||||
return data
|
||||
|
||||
line_polys = []
|
||||
for poly in data['polys']:
|
||||
new_poly = self.may_augment_poly(aug, shape, poly)
|
||||
line_polys.append(new_poly)
|
||||
data['polys'] = np.array(line_polys)
|
||||
return data
|
||||
|
||||
def may_augment_poly(self, aug, img_shape, poly):
|
||||
keypoints = [imgaug.Keypoint(p[0], p[1]) for p in poly]
|
||||
keypoints = aug.augment_keypoints(
|
||||
[imgaug.KeypointsOnImage(keypoints, shape=img_shape)])[0].keypoints
|
||||
poly = [(p.x, p.y) for p in keypoints]
|
||||
return poly
|
||||
|
||||
|
||||
def is_poly_in_rect(poly, x, y, w, h):
|
||||
poly = np.array(poly)
|
||||
if poly[:, 0].min() < x or poly[:, 0].max() > x + w:
|
||||
return False
|
||||
if poly[:, 1].min() < y or poly[:, 1].max() > y + h:
|
||||
return False
|
||||
return True
|
||||
|
||||
|
||||
def is_poly_outside_rect(poly, x, y, w, h):
|
||||
poly = np.array(poly)
|
||||
if poly[:, 0].max() < x or poly[:, 0].min() > x + w:
|
||||
return True
|
||||
if poly[:, 1].max() < y or poly[:, 1].min() > y + h:
|
||||
return True
|
||||
return False
|
||||
|
||||
|
||||
def split_regions(axis):
|
||||
regions = []
|
||||
min_axis = 0
|
||||
for i in range(1, axis.shape[0]):
|
||||
if axis[i] != axis[i - 1] + 1:
|
||||
region = axis[min_axis:i]
|
||||
min_axis = i
|
||||
regions.append(region)
|
||||
return regions
|
||||
|
||||
|
||||
def random_select(axis, max_size):
|
||||
xx = np.random.choice(axis, size=2)
|
||||
xmin = np.min(xx)
|
||||
xmax = np.max(xx)
|
||||
xmin = np.clip(xmin, 0, max_size - 1)
|
||||
xmax = np.clip(xmax, 0, max_size - 1)
|
||||
return xmin, xmax
|
||||
|
||||
|
||||
def region_wise_random_select(regions, max_size):
|
||||
selected_index = list(np.random.choice(len(regions), 2))
|
||||
selected_values = []
|
||||
for index in selected_index:
|
||||
axis = regions[index]
|
||||
xx = int(np.random.choice(axis, size=1))
|
||||
selected_values.append(xx)
|
||||
xmin = min(selected_values)
|
||||
xmax = max(selected_values)
|
||||
return xmin, xmax
|
||||
|
||||
|
||||
def crop_area(im, text_polys, min_crop_side_ratio, max_tries):
|
||||
h, w, _ = im.shape
|
||||
h_array = np.zeros(h, dtype=np.int32)
|
||||
w_array = np.zeros(w, dtype=np.int32)
|
||||
for points in text_polys:
|
||||
points = np.round(points, decimals=0).astype(np.int32)
|
||||
minx = np.min(points[:, 0])
|
||||
maxx = np.max(points[:, 0])
|
||||
w_array[minx:maxx] = 1
|
||||
miny = np.min(points[:, 1])
|
||||
maxy = np.max(points[:, 1])
|
||||
h_array[miny:maxy] = 1
|
||||
# ensure the cropped area not across a text
|
||||
h_axis = np.where(h_array == 0)[0]
|
||||
w_axis = np.where(w_array == 0)[0]
|
||||
|
||||
if len(h_axis) == 0 or len(w_axis) == 0:
|
||||
return 0, 0, w, h
|
||||
|
||||
h_regions = split_regions(h_axis)
|
||||
w_regions = split_regions(w_axis)
|
||||
|
||||
for i in range(max_tries):
|
||||
if len(w_regions) > 1:
|
||||
xmin, xmax = region_wise_random_select(w_regions, w)
|
||||
else:
|
||||
xmin, xmax = random_select(w_axis, w)
|
||||
if len(h_regions) > 1:
|
||||
ymin, ymax = region_wise_random_select(h_regions, h)
|
||||
else:
|
||||
ymin, ymax = random_select(h_axis, h)
|
||||
|
||||
if xmax - xmin < min_crop_side_ratio * w or ymax - ymin < min_crop_side_ratio * h:
|
||||
# area too small
|
||||
continue
|
||||
num_poly_in_rect = 0
|
||||
for poly in text_polys:
|
||||
if not is_poly_outside_rect(poly, xmin, ymin, xmax - xmin,
|
||||
ymax - ymin):
|
||||
num_poly_in_rect += 1
|
||||
break
|
||||
|
||||
if num_poly_in_rect > 0:
|
||||
return xmin, ymin, xmax - xmin, ymax - ymin
|
||||
|
||||
return 0, 0, w, h
|
||||
|
||||
|
||||
@PIPELINES.register_module()
|
||||
class EastRandomCropData(object):
|
||||
"""
|
||||
crop method for ocr detection, ensure the cropped area not across a text,
|
||||
and keep min side larger than min_crop_side_ratio
|
||||
"""
|
||||
|
||||
def __init__(self,
|
||||
size=(640, 640),
|
||||
max_tries=10,
|
||||
min_crop_side_ratio=0.1,
|
||||
keep_ratio=True,
|
||||
**kwargs):
|
||||
"""
|
||||
|
||||
Args:
|
||||
size (tuple, optional): target size to crop. Defaults to (640, 640).
|
||||
max_tries (int, optional): max try times. Defaults to 10.
|
||||
min_crop_side_ratio (float, optional): min side should larger than this. Defaults to 0.1.
|
||||
keep_ratio (bool, optional): whether to keep ratio. Defaults to True.
|
||||
"""
|
||||
self.size = size
|
||||
self.max_tries = max_tries
|
||||
self.min_crop_side_ratio = min_crop_side_ratio
|
||||
self.keep_ratio = keep_ratio
|
||||
|
||||
def __call__(self, data):
|
||||
img = data['img']
|
||||
text_polys = data['polys']
|
||||
ignore_tags = data['ignore_tags']
|
||||
texts = data['texts']
|
||||
all_care_polys = [
|
||||
text_polys[i] for i, tag in enumerate(ignore_tags) if not tag
|
||||
]
|
||||
# compute crop area
|
||||
crop_x, crop_y, crop_w, crop_h = crop_area(img, all_care_polys,
|
||||
self.min_crop_side_ratio,
|
||||
self.max_tries)
|
||||
# crop img
|
||||
scale_w = self.size[0] / crop_w
|
||||
scale_h = self.size[1] / crop_h
|
||||
scale = min(scale_w, scale_h)
|
||||
h = int(crop_h * scale)
|
||||
w = int(crop_w * scale)
|
||||
if self.keep_ratio:
|
||||
padimg = np.zeros((self.size[1], self.size[0], img.shape[2]),
|
||||
img.dtype)
|
||||
padimg[:h, :w] = cv2.resize(
|
||||
img[crop_y:crop_y + crop_h, crop_x:crop_x + crop_w], (w, h))
|
||||
img = padimg
|
||||
else:
|
||||
img = cv2.resize(
|
||||
img[crop_y:crop_y + crop_h, crop_x:crop_x + crop_w],
|
||||
tuple(self.size))
|
||||
# crop text
|
||||
text_polys_crop = []
|
||||
ignore_tags_crop = []
|
||||
texts_crop = []
|
||||
for poly, text, tag in zip(text_polys, texts, ignore_tags):
|
||||
poly = ((poly - (crop_x, crop_y)) * scale).tolist()
|
||||
if not is_poly_outside_rect(poly, 0, 0, w, h):
|
||||
text_polys_crop.append(poly)
|
||||
ignore_tags_crop.append(tag)
|
||||
texts_crop.append(text)
|
||||
data['img'] = img
|
||||
data['polys'] = np.array(text_polys_crop)
|
||||
data['ignore_tags'] = ignore_tags_crop
|
||||
data['texts'] = texts_crop
|
||||
return data
|
||||
|
||||
|
||||
@PIPELINES.register_module()
|
||||
class MakeBorderMap(object):
|
||||
"""
|
||||
Making Border binary mask from DBNet algorithm
|
||||
"""
|
||||
|
||||
def __init__(self,
|
||||
shrink_ratio=0.4,
|
||||
thresh_min=0.3,
|
||||
thresh_max=0.7,
|
||||
**kwargs):
|
||||
self.shrink_ratio = shrink_ratio
|
||||
self.thresh_min = thresh_min
|
||||
self.thresh_max = thresh_max
|
||||
|
||||
def __call__(self, data):
|
||||
|
||||
img = data['img']
|
||||
text_polys = data['polys']
|
||||
ignore_tags = data['ignore_tags']
|
||||
|
||||
canvas = np.zeros(img.shape[:2], dtype=np.float32)
|
||||
mask = np.zeros(img.shape[:2], dtype=np.float32)
|
||||
|
||||
for i in range(len(text_polys)):
|
||||
if ignore_tags[i]:
|
||||
continue
|
||||
self.draw_border_map(text_polys[i], canvas, mask=mask)
|
||||
canvas = canvas * (self.thresh_max - self.thresh_min) + self.thresh_min
|
||||
|
||||
data['threshold_map'] = canvas
|
||||
data['threshold_mask'] = mask
|
||||
return data
|
||||
|
||||
def draw_border_map(self, polygon, canvas, mask):
|
||||
polygon = np.array(polygon)
|
||||
assert polygon.ndim == 2
|
||||
assert polygon.shape[1] == 2
|
||||
|
||||
polygon_shape = Polygon(polygon)
|
||||
if polygon_shape.area <= 0:
|
||||
return
|
||||
distance = polygon_shape.area * (
|
||||
1 - np.power(self.shrink_ratio, 2)) / polygon_shape.length
|
||||
subject = [tuple(l) for l in polygon]
|
||||
padding = pyclipper.PyclipperOffset()
|
||||
padding.AddPath(subject, pyclipper.JT_ROUND,
|
||||
pyclipper.ET_CLOSEDPOLYGON)
|
||||
padded_polygon = np.array(padding.Execute(distance)[0])
|
||||
cv2.fillPoly(mask, [padded_polygon.astype(np.int32)], 1.0)
|
||||
|
||||
xmin = padded_polygon[:, 0].min()
|
||||
xmax = padded_polygon[:, 0].max()
|
||||
ymin = padded_polygon[:, 1].min()
|
||||
ymax = padded_polygon[:, 1].max()
|
||||
width = xmax - xmin + 1
|
||||
height = ymax - ymin + 1
|
||||
|
||||
polygon[:, 0] = polygon[:, 0] - xmin
|
||||
polygon[:, 1] = polygon[:, 1] - ymin
|
||||
|
||||
xs = np.broadcast_to(
|
||||
np.linspace(0, width - 1, num=width).reshape(1, width),
|
||||
(height, width))
|
||||
ys = np.broadcast_to(
|
||||
np.linspace(0, height - 1, num=height).reshape(height, 1),
|
||||
(height, width))
|
||||
|
||||
distance_map = np.zeros((polygon.shape[0], height, width),
|
||||
dtype=np.float32)
|
||||
for i in range(polygon.shape[0]):
|
||||
j = (i + 1) % polygon.shape[0]
|
||||
absolute_distance = self._distance(xs, ys, polygon[i], polygon[j])
|
||||
distance_map[i] = np.clip(absolute_distance / distance, 0, 1)
|
||||
distance_map = distance_map.min(axis=0)
|
||||
|
||||
xmin_valid = min(max(0, xmin), canvas.shape[1] - 1)
|
||||
xmax_valid = min(max(0, xmax), canvas.shape[1] - 1)
|
||||
ymin_valid = min(max(0, ymin), canvas.shape[0] - 1)
|
||||
ymax_valid = min(max(0, ymax), canvas.shape[0] - 1)
|
||||
canvas[ymin_valid:ymax_valid + 1, xmin_valid:xmax_valid + 1] = np.fmax(
|
||||
1 - distance_map[ymin_valid - ymin:ymax_valid - ymax + height,
|
||||
xmin_valid - xmin:xmax_valid - xmax + width],
|
||||
canvas[ymin_valid:ymax_valid + 1, xmin_valid:xmax_valid + 1])
|
||||
|
||||
def _distance(self, xs, ys, point_1, point_2):
|
||||
'''
|
||||
compute the distance from point to a line
|
||||
ys: coordinates in the first axis
|
||||
xs: coordinates in the second axis
|
||||
point_1, point_2: (x, y), the end of the line
|
||||
'''
|
||||
height, width = xs.shape[:2]
|
||||
square_distance_1 = np.square(xs - point_1[0]) + np.square(ys -
|
||||
point_1[1])
|
||||
square_distance_2 = np.square(xs - point_2[0]) + np.square(ys -
|
||||
point_2[1])
|
||||
square_distance = np.square(point_1[0] -
|
||||
point_2[0]) + np.square(point_1[1] -
|
||||
point_2[1])
|
||||
|
||||
cosin = (square_distance - square_distance_1 - square_distance_2) / (
|
||||
2 * np.sqrt(square_distance_1 * square_distance_2))
|
||||
square_sin = 1 - np.square(cosin)
|
||||
square_sin = np.nan_to_num(square_sin)
|
||||
result = np.sqrt(square_distance_1 * square_distance_2 * square_sin /
|
||||
square_distance)
|
||||
|
||||
result[cosin < 0] = np.sqrt(
|
||||
np.fmin(square_distance_1, square_distance_2))[cosin < 0]
|
||||
# self.extend_line(point_1, point_2, result)
|
||||
return result
|
||||
|
||||
def extend_line(self, point_1, point_2, result, shrink_ratio):
|
||||
ex_point_1 = (int(
|
||||
round(point_1[0] + (point_1[0] - point_2[0]) *
|
||||
(1 + shrink_ratio))),
|
||||
int(
|
||||
round(point_1[1] + (point_1[1] - point_2[1]) *
|
||||
(1 + shrink_ratio))))
|
||||
cv2.line(
|
||||
result,
|
||||
tuple(ex_point_1),
|
||||
tuple(point_1),
|
||||
4096.0,
|
||||
1,
|
||||
lineType=cv2.LINE_AA,
|
||||
shift=0)
|
||||
ex_point_2 = (int(
|
||||
round(point_2[0] + (point_2[0] - point_1[0]) *
|
||||
(1 + shrink_ratio))),
|
||||
int(
|
||||
round(point_2[1] + (point_2[1] - point_1[1]) *
|
||||
(1 + shrink_ratio))))
|
||||
cv2.line(
|
||||
result,
|
||||
tuple(ex_point_2),
|
||||
tuple(point_2),
|
||||
4096.0,
|
||||
1,
|
||||
lineType=cv2.LINE_AA,
|
||||
shift=0)
|
||||
return ex_point_1, ex_point_2
|
||||
|
||||
|
||||
@PIPELINES.register_module()
|
||||
class MakeShrinkMap(object):
|
||||
r'''
|
||||
Making binary mask from detection data with ICDAR format.
|
||||
Typically following the process of class `MakeICDARData`.
|
||||
'''
|
||||
|
||||
def __init__(self, min_text_size=8, shrink_ratio=0.4, **kwargs):
|
||||
self.min_text_size = min_text_size
|
||||
self.shrink_ratio = shrink_ratio
|
||||
|
||||
def __call__(self, data):
|
||||
image = data['img']
|
||||
text_polys = data['polys']
|
||||
ignore_tags = data['ignore_tags']
|
||||
|
||||
h, w = image.shape[:2]
|
||||
text_polys, ignore_tags = self.validate_polygons(
|
||||
text_polys, ignore_tags, h, w)
|
||||
gt = np.zeros((h, w), dtype=np.float32)
|
||||
mask = np.ones((h, w), dtype=np.float32)
|
||||
for i in range(len(text_polys)):
|
||||
polygon = text_polys[i]
|
||||
height = max(polygon[:, 1]) - min(polygon[:, 1])
|
||||
width = max(polygon[:, 0]) - min(polygon[:, 0])
|
||||
if ignore_tags[i] or min(height, width) < self.min_text_size:
|
||||
cv2.fillPoly(mask,
|
||||
polygon.astype(np.int32)[np.newaxis, :, :], 0)
|
||||
ignore_tags[i] = True
|
||||
else:
|
||||
polygon_shape = Polygon(polygon)
|
||||
subject = [tuple(l) for l in polygon]
|
||||
padding = pyclipper.PyclipperOffset()
|
||||
padding.AddPath(subject, pyclipper.JT_ROUND,
|
||||
pyclipper.ET_CLOSEDPOLYGON)
|
||||
shrinked = []
|
||||
|
||||
# Increase the shrink ratio every time we get multiple polygon returned back
|
||||
possible_ratios = np.arange(self.shrink_ratio, 1,
|
||||
self.shrink_ratio)
|
||||
np.append(possible_ratios, 1)
|
||||
# print(possible_ratios)
|
||||
for ratio in possible_ratios:
|
||||
# print(f"Change shrink ratio to {ratio}")
|
||||
distance = polygon_shape.area * (
|
||||
1 - np.power(ratio, 2)) / polygon_shape.length
|
||||
shrinked = padding.Execute(-distance)
|
||||
if len(shrinked) == 1:
|
||||
break
|
||||
|
||||
if shrinked == []:
|
||||
cv2.fillPoly(mask,
|
||||
polygon.astype(np.int32)[np.newaxis, :, :], 0)
|
||||
ignore_tags[i] = True
|
||||
continue
|
||||
|
||||
for each_shirnk in shrinked:
|
||||
shirnk = np.array(each_shirnk).reshape(-1, 2)
|
||||
cv2.fillPoly(gt, [shirnk.astype(np.int32)], 1)
|
||||
|
||||
data['shrink_map'] = gt
|
||||
data['shrink_mask'] = mask
|
||||
return data
|
||||
|
||||
def validate_polygons(self, polygons, ignore_tags, h, w):
|
||||
'''
|
||||
polygons (numpy.array, required): of shape (num_instances, num_points, 2)
|
||||
'''
|
||||
if len(polygons) == 0:
|
||||
return polygons, ignore_tags
|
||||
assert len(polygons) == len(ignore_tags)
|
||||
for polygon in polygons:
|
||||
polygon[:, 0] = np.clip(polygon[:, 0], 0, w - 1)
|
||||
polygon[:, 1] = np.clip(polygon[:, 1], 0, h - 1)
|
||||
|
||||
for i in range(len(polygons)):
|
||||
area = self.polygon_area(polygons[i])
|
||||
if abs(area) < 1:
|
||||
ignore_tags[i] = True
|
||||
if area > 0:
|
||||
polygons[i] = polygons[i][::-1, :]
|
||||
return polygons, ignore_tags
|
||||
|
||||
def polygon_area(self, polygon):
|
||||
"""
|
||||
compute polygon area
|
||||
"""
|
||||
area = 0
|
||||
q = polygon[-1]
|
||||
for p in polygon:
|
||||
area += p[0] * q[1] - p[1] * q[0]
|
||||
q = p
|
||||
return area / 2.0
|
||||
|
||||
|
||||
@PIPELINES.register_module()
|
||||
class OCRDetResize(object):
|
||||
"""resize function for ocr det test
|
||||
"""
|
||||
|
||||
def __init__(self, **kwargs):
|
||||
super(OCRDetResize, self).__init__()
|
||||
self.resize_type = 0
|
||||
if 'image_shape' in kwargs:
|
||||
self.image_shape = kwargs['image_shape']
|
||||
self.resize_type = 1
|
||||
elif 'limit_side_len' in kwargs:
|
||||
self.limit_side_len = kwargs['limit_side_len']
|
||||
self.limit_type = kwargs.get('limit_type', 'min')
|
||||
elif 'resize_long' in kwargs:
|
||||
self.resize_type = 2
|
||||
self.resize_long = kwargs.get('resize_long', 960)
|
||||
else:
|
||||
self.limit_side_len = 736
|
||||
self.limit_type = 'min'
|
||||
|
||||
def __call__(self, data):
|
||||
img = data['img']
|
||||
src_h, src_w, _ = img.shape
|
||||
|
||||
if self.resize_type == 0:
|
||||
# img, shape = self.resize_image_type0(img)
|
||||
img, [ratio_h, ratio_w] = self.resize_image_type0(img)
|
||||
elif self.resize_type == 2:
|
||||
img, [ratio_h, ratio_w] = self.resize_image_type2(img)
|
||||
else:
|
||||
# img, shape = self.resize_image_type1(img)
|
||||
img, [ratio_h, ratio_w] = self.resize_image_type1(img)
|
||||
data['img'] = img
|
||||
# data['shape'] = np.array([src_h, src_w, ratio_h, ratio_w])
|
||||
return data
|
||||
|
||||
def resize_image_type1(self, img):
|
||||
resize_h, resize_w = self.image_shape
|
||||
ori_h, ori_w = img.shape[:2] # (h, w, c)
|
||||
ratio_h = float(resize_h) / ori_h
|
||||
ratio_w = float(resize_w) / ori_w
|
||||
img = cv2.resize(img, (int(resize_w), int(resize_h)))
|
||||
return img, [ratio_h, ratio_w]
|
||||
|
||||
def resize_image_type0(self, img):
|
||||
"""
|
||||
resize image to a size multiple of 32 which is required by the network
|
||||
args:
|
||||
img(array): array with shape [h, w, c]
|
||||
return(tuple):
|
||||
img, (ratio_h, ratio_w)
|
||||
"""
|
||||
limit_side_len = self.limit_side_len
|
||||
h, w, c = img.shape
|
||||
|
||||
# limit the max side
|
||||
if self.limit_type == 'max':
|
||||
if max(h, w) > limit_side_len:
|
||||
if h > w:
|
||||
ratio = float(limit_side_len) / h
|
||||
else:
|
||||
ratio = float(limit_side_len) / w
|
||||
else:
|
||||
ratio = 1.
|
||||
elif self.limit_type == 'min':
|
||||
if min(h, w) < limit_side_len:
|
||||
if h < w:
|
||||
ratio = float(limit_side_len) / h
|
||||
else:
|
||||
ratio = float(limit_side_len) / w
|
||||
else:
|
||||
ratio = 1.
|
||||
elif self.limit_type == 'resize_long':
|
||||
ratio = float(limit_side_len) / max(h, w)
|
||||
else:
|
||||
raise Exception('not support limit type, image ')
|
||||
resize_h = int(h * ratio)
|
||||
resize_w = int(w * ratio)
|
||||
|
||||
resize_h = max(int(round(resize_h / 32) * 32), 32)
|
||||
resize_w = max(int(round(resize_w / 32) * 32), 32)
|
||||
|
||||
try:
|
||||
if int(resize_w) <= 0 or int(resize_h) <= 0:
|
||||
return None, (None, None)
|
||||
img = cv2.resize(img, (int(resize_w), int(resize_h)))
|
||||
except:
|
||||
print(img.shape, resize_w, resize_h)
|
||||
sys.exit(0)
|
||||
ratio_h = resize_h / float(h)
|
||||
ratio_w = resize_w / float(w)
|
||||
return img, [ratio_h, ratio_w]
|
||||
|
||||
def resize_image_type2(self, img):
|
||||
h, w, _ = img.shape
|
||||
|
||||
resize_w = w
|
||||
resize_h = h
|
||||
|
||||
if resize_h > resize_w:
|
||||
ratio = float(self.resize_long) / resize_h
|
||||
else:
|
||||
ratio = float(self.resize_long) / resize_w
|
||||
|
||||
resize_h = int(resize_h * ratio)
|
||||
resize_w = int(resize_w * ratio)
|
||||
|
||||
max_stride = 128
|
||||
resize_h = (resize_h + max_stride - 1) // max_stride * max_stride
|
||||
resize_w = (resize_w + max_stride - 1) // max_stride * max_stride
|
||||
img = cv2.resize(img, (int(resize_w), int(resize_h)))
|
||||
ratio_h = resize_h / float(h)
|
||||
ratio_w = resize_w / float(w)
|
||||
|
||||
return img, [ratio_h, ratio_w]
|
|
@ -0,0 +1,215 @@
|
|||
# copyright (c) 2020 PaddlePaddle Authors. All Rights Reserve.
|
||||
#
|
||||
# Licensed under the Apache License, Version 2.0 (the "License");
|
||||
# you may not use this file except in compliance with the License.
|
||||
# You may obtain a copy of the License at
|
||||
#
|
||||
# http://www.apache.org/licenses/LICENSE-2.0
|
||||
#
|
||||
# Unless required by applicable law or agreed to in writing, software
|
||||
# distributed under the License is distributed on an "AS IS" BASIS,
|
||||
# WITHOUT WARRANTIES OR CONDITIONS OF ANY KIND, either express or implied.
|
||||
# See the License for the specific language governing permissions and
|
||||
# limitations under the License.
|
||||
|
||||
import copy
|
||||
import math
|
||||
import os.path as osp
|
||||
|
||||
import cv2
|
||||
import numpy as np
|
||||
import requests
|
||||
|
||||
from easycv.datasets.registry import PIPELINES
|
||||
from easycv.utils.logger import get_root_logger
|
||||
|
||||
|
||||
@PIPELINES.register_module()
|
||||
class BaseRecLabelEncode(object):
|
||||
""" Convert between text-label and text-index """
|
||||
|
||||
def __init__(self,
|
||||
max_text_length,
|
||||
character_dict_path=None,
|
||||
use_space_char=False):
|
||||
|
||||
self.max_text_len = max_text_length
|
||||
self.BEGIN_STR = 'sos'
|
||||
self.END_STR = 'eos'
|
||||
self.lower = False
|
||||
|
||||
if character_dict_path is None:
|
||||
logger = get_root_logger()
|
||||
logger.warning(
|
||||
'The character_dict_path is None, model can only recognize number and lower letters'
|
||||
)
|
||||
self.character_str = '0123456789abcdefghijklmnopqrstuvwxyz'
|
||||
dict_character = list(self.character_str)
|
||||
self.lower = True
|
||||
else:
|
||||
self.character_str = []
|
||||
if character_dict_path.startswith('http'):
|
||||
r = requests.get(character_dict_path)
|
||||
tpath = character_dict_path.split('/')[-1]
|
||||
while not osp.exists(tpath):
|
||||
try:
|
||||
with open(tpath, 'wb') as code:
|
||||
code.write(r.content)
|
||||
except:
|
||||
pass
|
||||
character_dict_path = tpath
|
||||
with open(character_dict_path, 'rb') as fin:
|
||||
lines = fin.readlines()
|
||||
for line in lines:
|
||||
line = line.decode('utf-8').strip('\n').strip('\r\n')
|
||||
self.character_str.append(line)
|
||||
if use_space_char:
|
||||
self.character_str.append(' ')
|
||||
dict_character = list(self.character_str)
|
||||
dict_character = self.add_special_char(dict_character)
|
||||
self.dict = {}
|
||||
for i, char in enumerate(dict_character):
|
||||
self.dict[char] = i
|
||||
self.character = dict_character
|
||||
|
||||
def add_special_char(self, dict_character):
|
||||
return dict_character
|
||||
|
||||
def encode(self, text):
|
||||
"""convert text-label into text-index.
|
||||
input:
|
||||
text: text labels of each image. [batch_size]
|
||||
|
||||
output:
|
||||
text: concatenated text index for CTCLoss.
|
||||
[sum(text_lengths)] = [text_index_0 + text_index_1 + ... + text_index_(n - 1)]
|
||||
length: length of each text. [batch_size]
|
||||
"""
|
||||
if len(text) == 0 or len(text) > self.max_text_len:
|
||||
return None
|
||||
if self.lower:
|
||||
text = text.lower()
|
||||
text_list = []
|
||||
for char in text:
|
||||
if char not in self.dict:
|
||||
# logger = get_logger()
|
||||
# logger.warning('{} is not in dict'.format(char))
|
||||
continue
|
||||
text_list.append(self.dict[char])
|
||||
if len(text_list) == 0:
|
||||
return None
|
||||
return text_list
|
||||
|
||||
|
||||
@PIPELINES.register_module()
|
||||
class CTCLabelEncode(BaseRecLabelEncode):
|
||||
""" Convert between text-label and text-index """
|
||||
|
||||
def __init__(self,
|
||||
max_text_length,
|
||||
character_dict_path=None,
|
||||
use_space_char=False,
|
||||
**kwargs):
|
||||
self.BLANK = ['blank']
|
||||
super(CTCLabelEncode,
|
||||
self).__init__(max_text_length, character_dict_path,
|
||||
use_space_char)
|
||||
|
||||
def __call__(self, data):
|
||||
text = data['label']
|
||||
text = self.encode(text)
|
||||
if text is None:
|
||||
return None
|
||||
data['length'] = np.array(len(text))
|
||||
text = text + [0] * (self.max_text_len - len(text))
|
||||
data['label'] = np.array(text)
|
||||
|
||||
label = [0] * len(self.character)
|
||||
for x in text:
|
||||
label[x] += 1
|
||||
data['label_ace'] = np.array(label)
|
||||
return data
|
||||
|
||||
def add_special_char(self, dict_character):
|
||||
dict_character = self.BLANK + dict_character
|
||||
return dict_character
|
||||
|
||||
|
||||
@PIPELINES.register_module()
|
||||
class SARLabelEncode(BaseRecLabelEncode):
|
||||
""" Convert between text-label and text-index """
|
||||
|
||||
def __init__(self,
|
||||
max_text_length,
|
||||
character_dict_path=None,
|
||||
use_space_char=False,
|
||||
**kwargs):
|
||||
self.BEG_END_STR = '<BOS/EOS>'
|
||||
self.UNKNOWN_STR = '<UKN>'
|
||||
self.PADDING_STR = '<PAD>'
|
||||
super(SARLabelEncode,
|
||||
self).__init__(max_text_length, character_dict_path,
|
||||
use_space_char)
|
||||
|
||||
def add_special_char(self, dict_character):
|
||||
dict_character = dict_character + [self.UNKNOWN_STR]
|
||||
self.unknown_idx = len(dict_character) - 1
|
||||
dict_character = dict_character + [self.BEG_END_STR]
|
||||
self.start_idx = len(dict_character) - 1
|
||||
self.end_idx = len(dict_character) - 1
|
||||
dict_character = dict_character + [self.PADDING_STR]
|
||||
self.padding_idx = len(dict_character) - 1
|
||||
|
||||
return dict_character
|
||||
|
||||
def __call__(self, data):
|
||||
text = data['label']
|
||||
text = self.encode(text)
|
||||
if text is None:
|
||||
return None
|
||||
if len(text) >= self.max_text_len - 1:
|
||||
return None
|
||||
data['length'] = np.array(len(text))
|
||||
target = [self.start_idx] + text + [self.end_idx]
|
||||
padded_text = [self.padding_idx for _ in range(self.max_text_len)]
|
||||
|
||||
padded_text[:len(target)] = target
|
||||
data['label'] = np.array(padded_text)
|
||||
return data
|
||||
|
||||
def get_ignored_tokens(self):
|
||||
return [self.padding_idx]
|
||||
|
||||
|
||||
@PIPELINES.register_module()
|
||||
class MultiLabelEncode(BaseRecLabelEncode):
|
||||
|
||||
def __init__(self,
|
||||
max_text_length,
|
||||
character_dict_path=None,
|
||||
use_space_char=False,
|
||||
**kwargs):
|
||||
super(MultiLabelEncode,
|
||||
self).__init__(max_text_length, character_dict_path,
|
||||
use_space_char)
|
||||
|
||||
self.ctc_encode = CTCLabelEncode(max_text_length, character_dict_path,
|
||||
use_space_char, **kwargs)
|
||||
self.sar_encode = SARLabelEncode(max_text_length, character_dict_path,
|
||||
use_space_char, **kwargs)
|
||||
|
||||
def __call__(self, data):
|
||||
|
||||
data_ctc = copy.deepcopy(data)
|
||||
data_sar = copy.deepcopy(data)
|
||||
data_out = dict()
|
||||
data_out['img_path'] = data.get('img_path', None)
|
||||
data_out['img'] = data['img']
|
||||
ctc = self.ctc_encode(data_ctc)
|
||||
sar = self.sar_encode(data_sar)
|
||||
if ctc is None or sar is None:
|
||||
return None
|
||||
data_out['label_ctc'] = ctc['label']
|
||||
data_out['label_sar'] = sar['label']
|
||||
data_out['length'] = ctc['length']
|
||||
return data_out
|
|
@ -0,0 +1,697 @@
|
|||
# Modified from https://github.com/PaddlePaddle/PaddleOCR/tree/release/2.6/ppocr/data/imaug
|
||||
import math
|
||||
import random
|
||||
import sys
|
||||
|
||||
import cv2
|
||||
import imgaug
|
||||
import imgaug.augmenters as iaa
|
||||
import numpy as np
|
||||
import pyclipper
|
||||
from shapely.geometry import Polygon
|
||||
|
||||
from easycv.datasets.registry import PIPELINES
|
||||
|
||||
|
||||
@PIPELINES.register_module()
|
||||
class RecConAug(object):
|
||||
"""concat multiple texts together for text recognition training
|
||||
"""
|
||||
|
||||
def __init__(self,
|
||||
prob=0.5,
|
||||
image_shape=(32, 320, 3),
|
||||
max_text_length=25,
|
||||
**kwargs):
|
||||
"""
|
||||
|
||||
Args:
|
||||
prob (float, optional): the probability whether do data augmentation. Defaults to 0.5.
|
||||
image_shape (tuple, optional): the output image shape. Defaults to (32, 320, 3).
|
||||
max_text_length (int, optional): the max length of text label. Defaults to 25.
|
||||
"""
|
||||
self.prob = prob
|
||||
self.max_text_length = max_text_length
|
||||
self.image_shape = image_shape
|
||||
self.max_wh_ratio = self.image_shape[1] / self.image_shape[0]
|
||||
|
||||
def merge_ext_data(self, data, ext_data):
|
||||
ori_w = round(data['img'].shape[1] / data['img'].shape[0] *
|
||||
self.image_shape[0])
|
||||
ext_w = round(ext_data['img'].shape[1] / ext_data['img'].shape[0] *
|
||||
self.image_shape[0])
|
||||
data['img'] = cv2.resize(data['img'], (ori_w, self.image_shape[0]))
|
||||
ext_data['img'] = cv2.resize(ext_data['img'],
|
||||
(ext_w, self.image_shape[0]))
|
||||
data['img'] = np.concatenate([data['img'], ext_data['img']], axis=1)
|
||||
data['label'] += ext_data['label']
|
||||
return data
|
||||
|
||||
def __call__(self, data):
|
||||
rnd_num = random.random()
|
||||
if rnd_num > self.prob:
|
||||
return data
|
||||
for idx, ext_data in enumerate(data['ext_data']):
|
||||
if len(data['label']) + len(
|
||||
ext_data['label']) > self.max_text_length:
|
||||
break
|
||||
concat_ratio = data['img'].shape[1] / data['img'].shape[
|
||||
0] + ext_data['img'].shape[1] / ext_data['img'].shape[0]
|
||||
if concat_ratio > self.max_wh_ratio:
|
||||
break
|
||||
data = self.merge_ext_data(data, ext_data)
|
||||
data.pop('ext_data')
|
||||
return data
|
||||
|
||||
|
||||
@PIPELINES.register_module()
|
||||
class RecAug(object):
|
||||
"""data augmentation function for ocr recognition
|
||||
"""
|
||||
|
||||
def __init__(self, use_tia=True, aug_prob=0.4, **kwargs):
|
||||
"""
|
||||
|
||||
Args:
|
||||
use_tia (bool, optional): whether make tia augmentation. Defaults to True.
|
||||
aug_prob (float, optional): the probability were do data augmentation. Defaults to 0.4.
|
||||
"""
|
||||
self.use_tia = use_tia
|
||||
self.aug_prob = aug_prob
|
||||
|
||||
def __call__(self, data):
|
||||
img = data['img']
|
||||
img = warp(img, 10, self.use_tia, self.aug_prob)
|
||||
data['img'] = img
|
||||
return data
|
||||
|
||||
|
||||
def flag():
|
||||
"""
|
||||
flag
|
||||
"""
|
||||
return 1 if random.random() > 0.5000001 else -1
|
||||
|
||||
|
||||
def cvtColor(img):
|
||||
"""
|
||||
cvtColor
|
||||
"""
|
||||
hsv = cv2.cvtColor(img, cv2.COLOR_BGR2HSV)
|
||||
delta = 0.001 * random.random() * flag()
|
||||
hsv[:, :, 2] = hsv[:, :, 2] * (1 + delta)
|
||||
new_img = cv2.cvtColor(hsv, cv2.COLOR_HSV2BGR)
|
||||
return new_img
|
||||
|
||||
|
||||
def blur(img):
|
||||
"""
|
||||
blur
|
||||
"""
|
||||
h, w, _ = img.shape
|
||||
if h > 10 and w > 10:
|
||||
return cv2.GaussianBlur(img, (5, 5), 1)
|
||||
else:
|
||||
return img
|
||||
|
||||
|
||||
def jitter(img):
|
||||
"""
|
||||
jitter
|
||||
"""
|
||||
w, h, _ = img.shape
|
||||
if h > 10 and w > 10:
|
||||
thres = min(w, h)
|
||||
s = int(random.random() * thres * 0.01)
|
||||
src_img = img.copy()
|
||||
for i in range(s):
|
||||
img[i:, i:, :] = src_img[:w - i, :h - i, :]
|
||||
return img
|
||||
else:
|
||||
return img
|
||||
|
||||
|
||||
def add_gasuss_noise(image, mean=0, var=0.1):
|
||||
"""
|
||||
Gasuss noise
|
||||
"""
|
||||
|
||||
noise = np.random.normal(mean, var**0.5, image.shape)
|
||||
out = image + 0.5 * noise
|
||||
out = np.clip(out, 0, 255)
|
||||
out = np.uint8(out)
|
||||
return out
|
||||
|
||||
|
||||
def get_crop(image):
|
||||
"""
|
||||
random crop
|
||||
"""
|
||||
h, w, _ = image.shape
|
||||
top_min = 1
|
||||
top_max = 8
|
||||
top_crop = int(random.randint(top_min, top_max))
|
||||
top_crop = min(top_crop, h - 1)
|
||||
crop_img = image.copy()
|
||||
ratio = random.randint(0, 1)
|
||||
if ratio:
|
||||
crop_img = crop_img[top_crop:h, :, :]
|
||||
else:
|
||||
crop_img = crop_img[0:h - top_crop, :, :]
|
||||
return crop_img
|
||||
|
||||
|
||||
class Config:
|
||||
"""
|
||||
Config
|
||||
"""
|
||||
|
||||
def __init__(self, use_tia):
|
||||
self.anglex = random.random() * 30
|
||||
self.angley = random.random() * 15
|
||||
self.anglez = random.random() * 10
|
||||
self.fov = 42
|
||||
self.r = 0
|
||||
self.shearx = random.random() * 0.3
|
||||
self.sheary = random.random() * 0.05
|
||||
self.borderMode = cv2.BORDER_REPLICATE
|
||||
self.use_tia = use_tia
|
||||
|
||||
def make(self, w, h, ang):
|
||||
"""
|
||||
make
|
||||
"""
|
||||
self.anglex = random.random() * 5 * flag()
|
||||
self.angley = random.random() * 5 * flag()
|
||||
self.anglez = -1 * random.random() * int(ang) * flag()
|
||||
self.fov = 42
|
||||
self.r = 0
|
||||
self.shearx = 0
|
||||
self.sheary = 0
|
||||
self.borderMode = cv2.BORDER_REPLICATE
|
||||
self.w = w
|
||||
self.h = h
|
||||
|
||||
self.perspective = self.use_tia
|
||||
self.stretch = self.use_tia
|
||||
self.distort = self.use_tia
|
||||
|
||||
self.crop = True
|
||||
self.affine = False
|
||||
self.reverse = True
|
||||
self.noise = True
|
||||
self.jitter = True
|
||||
self.blur = True
|
||||
self.color = True
|
||||
|
||||
|
||||
def rad(x):
|
||||
"""
|
||||
rad
|
||||
"""
|
||||
return x * np.pi / 180
|
||||
|
||||
|
||||
def get_warpR(config):
|
||||
"""
|
||||
get_warpR
|
||||
"""
|
||||
anglex, angley, anglez, fov, w, h, r = \
|
||||
config.anglex, config.angley, config.anglez, config.fov, config.w, config.h, config.r
|
||||
if w > 69 and w < 112:
|
||||
anglex = anglex * 1.5
|
||||
|
||||
z = np.sqrt(w**2 + h**2) / 2 / np.tan(rad(fov / 2))
|
||||
# Homogeneous coordinate transformation matrix
|
||||
rx = np.array(
|
||||
[[1, 0, 0, 0], [0, np.cos(rad(anglex)), -np.sin(rad(anglex)), 0],
|
||||
[0, -np.sin(rad(anglex)),
|
||||
np.cos(rad(anglex)), 0], [0, 0, 0, 1]], np.float32)
|
||||
ry = np.array([[np.cos(rad(angley)), 0,
|
||||
np.sin(rad(angley)), 0], [0, 1, 0, 0],
|
||||
[
|
||||
-np.sin(rad(angley)),
|
||||
0,
|
||||
np.cos(rad(angley)),
|
||||
0,
|
||||
], [0, 0, 0, 1]], np.float32)
|
||||
rz = np.array(
|
||||
[[np.cos(rad(anglez)), np.sin(rad(anglez)), 0, 0],
|
||||
[-np.sin(rad(anglez)),
|
||||
np.cos(rad(anglez)), 0, 0], [0, 0, 1, 0], [0, 0, 0, 1]], np.float32)
|
||||
r = rx.dot(ry).dot(rz)
|
||||
# generate 4 points
|
||||
pcenter = np.array([h / 2, w / 2, 0, 0], np.float32)
|
||||
p1 = np.array([0, 0, 0, 0], np.float32) - pcenter
|
||||
p2 = np.array([w, 0, 0, 0], np.float32) - pcenter
|
||||
p3 = np.array([0, h, 0, 0], np.float32) - pcenter
|
||||
p4 = np.array([w, h, 0, 0], np.float32) - pcenter
|
||||
dst1 = r.dot(p1)
|
||||
dst2 = r.dot(p2)
|
||||
dst3 = r.dot(p3)
|
||||
dst4 = r.dot(p4)
|
||||
list_dst = np.array([dst1, dst2, dst3, dst4])
|
||||
org = np.array([[0, 0], [w, 0], [0, h], [w, h]], np.float32)
|
||||
dst = np.zeros((4, 2), np.float32)
|
||||
# Project onto the image plane
|
||||
dst[:, 0] = list_dst[:, 0] * z / (z - list_dst[:, 2]) + pcenter[0]
|
||||
dst[:, 1] = list_dst[:, 1] * z / (z - list_dst[:, 2]) + pcenter[1]
|
||||
|
||||
warpR = cv2.getPerspectiveTransform(org, dst)
|
||||
|
||||
dst1, dst2, dst3, dst4 = dst
|
||||
r1 = int(min(dst1[1], dst2[1]))
|
||||
r2 = int(max(dst3[1], dst4[1]))
|
||||
c1 = int(min(dst1[0], dst3[0]))
|
||||
c2 = int(max(dst2[0], dst4[0]))
|
||||
|
||||
try:
|
||||
ratio = min(1.0 * h / (r2 - r1), 1.0 * w / (c2 - c1))
|
||||
|
||||
dx = -c1
|
||||
dy = -r1
|
||||
T1 = np.float32([[1., 0, dx], [0, 1., dy], [0, 0, 1.0 / ratio]])
|
||||
ret = T1.dot(warpR)
|
||||
except:
|
||||
ratio = 1.0
|
||||
T1 = np.float32([[1., 0, 0], [0, 1., 0], [0, 0, 1.]])
|
||||
ret = T1
|
||||
return ret, (-r1, -c1), ratio, dst
|
||||
|
||||
|
||||
def get_warpAffine(config):
|
||||
"""
|
||||
get_warpAffine
|
||||
"""
|
||||
anglez = config.anglez
|
||||
rz = np.array(
|
||||
[[np.cos(rad(anglez)), np.sin(rad(anglez)), 0],
|
||||
[-np.sin(rad(anglez)), np.cos(rad(anglez)), 0]], np.float32)
|
||||
return rz
|
||||
|
||||
|
||||
def warp(img, ang, use_tia=True, prob=0.4):
|
||||
"""
|
||||
warp
|
||||
"""
|
||||
h, w, _ = img.shape
|
||||
config = Config(use_tia=use_tia)
|
||||
config.make(w, h, ang)
|
||||
new_img = img
|
||||
|
||||
if config.distort:
|
||||
img_height, img_width = img.shape[0:2]
|
||||
if random.random() <= prob and img_height >= 20 and img_width >= 20:
|
||||
new_img = tia_distort(new_img, random.randint(3, 6))
|
||||
|
||||
if config.stretch:
|
||||
img_height, img_width = img.shape[0:2]
|
||||
if random.random() <= prob and img_height >= 20 and img_width >= 20:
|
||||
new_img = tia_stretch(new_img, random.randint(3, 6))
|
||||
|
||||
if config.perspective:
|
||||
if random.random() <= prob:
|
||||
new_img = tia_perspective(new_img)
|
||||
|
||||
if config.crop:
|
||||
img_height, img_width = img.shape[0:2]
|
||||
if random.random() <= prob and img_height >= 20 and img_width >= 20:
|
||||
new_img = get_crop(new_img)
|
||||
|
||||
if config.blur:
|
||||
if random.random() <= prob:
|
||||
new_img = blur(new_img)
|
||||
if config.color:
|
||||
if random.random() <= prob:
|
||||
new_img = cvtColor(new_img)
|
||||
if config.jitter:
|
||||
new_img = jitter(new_img)
|
||||
if config.noise:
|
||||
if random.random() <= prob:
|
||||
new_img = add_gasuss_noise(new_img)
|
||||
if config.reverse:
|
||||
if random.random() <= prob:
|
||||
new_img = 255 - new_img
|
||||
return new_img
|
||||
|
||||
|
||||
class WarpMLS:
|
||||
|
||||
def __init__(self, src, src_pts, dst_pts, dst_w, dst_h, trans_ratio=1.):
|
||||
self.src = src
|
||||
self.src_pts = src_pts
|
||||
self.dst_pts = dst_pts
|
||||
self.pt_count = len(self.dst_pts)
|
||||
self.dst_w = dst_w
|
||||
self.dst_h = dst_h
|
||||
self.trans_ratio = trans_ratio
|
||||
self.grid_size = 100
|
||||
self.rdx = np.zeros((self.dst_h, self.dst_w))
|
||||
self.rdy = np.zeros((self.dst_h, self.dst_w))
|
||||
|
||||
@staticmethod
|
||||
def __bilinear_interp(x, y, v11, v12, v21, v22):
|
||||
return (v11 * (1 - y) + v12 * y) * (1 - x) + (v21 *
|
||||
(1 - y) + v22 * y) * x
|
||||
|
||||
def generate(self):
|
||||
self.calc_delta()
|
||||
return self.gen_img()
|
||||
|
||||
def calc_delta(self):
|
||||
w = np.zeros(self.pt_count, dtype=np.float32)
|
||||
|
||||
if self.pt_count < 2:
|
||||
return
|
||||
|
||||
i = 0
|
||||
while 1:
|
||||
if self.dst_w <= i < self.dst_w + self.grid_size - 1:
|
||||
i = self.dst_w - 1
|
||||
elif i >= self.dst_w:
|
||||
break
|
||||
|
||||
j = 0
|
||||
while 1:
|
||||
if self.dst_h <= j < self.dst_h + self.grid_size - 1:
|
||||
j = self.dst_h - 1
|
||||
elif j >= self.dst_h:
|
||||
break
|
||||
|
||||
sw = 0
|
||||
swp = np.zeros(2, dtype=np.float32)
|
||||
swq = np.zeros(2, dtype=np.float32)
|
||||
new_pt = np.zeros(2, dtype=np.float32)
|
||||
cur_pt = np.array([i, j], dtype=np.float32)
|
||||
|
||||
k = 0
|
||||
for k in range(self.pt_count):
|
||||
if i == self.dst_pts[k][0] and j == self.dst_pts[k][1]:
|
||||
break
|
||||
|
||||
w[k] = 1. / ((i - self.dst_pts[k][0]) *
|
||||
(i - self.dst_pts[k][0]) +
|
||||
(j - self.dst_pts[k][1]) *
|
||||
(j - self.dst_pts[k][1]))
|
||||
|
||||
sw += w[k]
|
||||
swp = swp + w[k] * np.array(self.dst_pts[k])
|
||||
swq = swq + w[k] * np.array(self.src_pts[k])
|
||||
|
||||
if k == self.pt_count - 1:
|
||||
pstar = 1 / sw * swp
|
||||
qstar = 1 / sw * swq
|
||||
|
||||
miu_s = 0
|
||||
for k in range(self.pt_count):
|
||||
if i == self.dst_pts[k][0] and j == self.dst_pts[k][1]:
|
||||
continue
|
||||
pt_i = self.dst_pts[k] - pstar
|
||||
miu_s += w[k] * np.sum(pt_i * pt_i)
|
||||
|
||||
cur_pt -= pstar
|
||||
cur_pt_j = np.array([-cur_pt[1], cur_pt[0]])
|
||||
|
||||
for k in range(self.pt_count):
|
||||
if i == self.dst_pts[k][0] and j == self.dst_pts[k][1]:
|
||||
continue
|
||||
|
||||
pt_i = self.dst_pts[k] - pstar
|
||||
pt_j = np.array([-pt_i[1], pt_i[0]])
|
||||
|
||||
tmp_pt = np.zeros(2, dtype=np.float32)
|
||||
tmp_pt[0] = np.sum(
|
||||
pt_i * cur_pt) * self.src_pts[k][0] - np.sum(
|
||||
pt_j * cur_pt) * self.src_pts[k][1]
|
||||
tmp_pt[1] = -np.sum(pt_i * cur_pt_j) * self.src_pts[k][
|
||||
0] + np.sum(pt_j * cur_pt_j) * self.src_pts[k][1]
|
||||
tmp_pt *= (w[k] / miu_s)
|
||||
new_pt += tmp_pt
|
||||
|
||||
new_pt += qstar
|
||||
else:
|
||||
new_pt = self.src_pts[k]
|
||||
|
||||
self.rdx[j, i] = new_pt[0] - i
|
||||
self.rdy[j, i] = new_pt[1] - j
|
||||
|
||||
j += self.grid_size
|
||||
i += self.grid_size
|
||||
|
||||
def gen_img(self):
|
||||
src_h, src_w = self.src.shape[:2]
|
||||
dst = np.zeros_like(self.src, dtype=np.float32)
|
||||
|
||||
for i in np.arange(0, self.dst_h, self.grid_size):
|
||||
for j in np.arange(0, self.dst_w, self.grid_size):
|
||||
ni = i + self.grid_size
|
||||
nj = j + self.grid_size
|
||||
w = h = self.grid_size
|
||||
if ni >= self.dst_h:
|
||||
ni = self.dst_h - 1
|
||||
h = ni - i + 1
|
||||
if nj >= self.dst_w:
|
||||
nj = self.dst_w - 1
|
||||
w = nj - j + 1
|
||||
|
||||
di = np.reshape(np.arange(h), (-1, 1))
|
||||
dj = np.reshape(np.arange(w), (1, -1))
|
||||
delta_x = self.__bilinear_interp(di / h, dj / w,
|
||||
self.rdx[i, j], self.rdx[i,
|
||||
nj],
|
||||
self.rdx[ni, j], self.rdx[ni,
|
||||
nj])
|
||||
delta_y = self.__bilinear_interp(di / h, dj / w,
|
||||
self.rdy[i, j], self.rdy[i,
|
||||
nj],
|
||||
self.rdy[ni, j], self.rdy[ni,
|
||||
nj])
|
||||
nx = j + dj + delta_x * self.trans_ratio
|
||||
ny = i + di + delta_y * self.trans_ratio
|
||||
nx = np.clip(nx, 0, src_w - 1)
|
||||
ny = np.clip(ny, 0, src_h - 1)
|
||||
nxi = np.array(np.floor(nx), dtype=np.int32)
|
||||
nyi = np.array(np.floor(ny), dtype=np.int32)
|
||||
nxi1 = np.array(np.ceil(nx), dtype=np.int32)
|
||||
nyi1 = np.array(np.ceil(ny), dtype=np.int32)
|
||||
|
||||
if len(self.src.shape) == 3:
|
||||
x = np.tile(np.expand_dims(ny - nyi, axis=-1), (1, 1, 3))
|
||||
y = np.tile(np.expand_dims(nx - nxi, axis=-1), (1, 1, 3))
|
||||
else:
|
||||
x = ny - nyi
|
||||
y = nx - nxi
|
||||
dst[i:i + h,
|
||||
j:j + w] = self.__bilinear_interp(x, y, self.src[nyi, nxi],
|
||||
self.src[nyi, nxi1],
|
||||
self.src[nyi1, nxi],
|
||||
self.src[nyi1, nxi1])
|
||||
|
||||
dst = np.clip(dst, 0, 255)
|
||||
dst = np.array(dst, dtype=np.uint8)
|
||||
|
||||
return dst
|
||||
|
||||
|
||||
def tia_distort(src, segment=4):
|
||||
img_h, img_w = src.shape[:2]
|
||||
|
||||
cut = img_w // segment
|
||||
thresh = cut // 3
|
||||
|
||||
src_pts = list()
|
||||
dst_pts = list()
|
||||
|
||||
src_pts.append([0, 0])
|
||||
src_pts.append([img_w, 0])
|
||||
src_pts.append([img_w, img_h])
|
||||
src_pts.append([0, img_h])
|
||||
|
||||
dst_pts.append([np.random.randint(thresh), np.random.randint(thresh)])
|
||||
dst_pts.append(
|
||||
[img_w - np.random.randint(thresh),
|
||||
np.random.randint(thresh)])
|
||||
dst_pts.append(
|
||||
[img_w - np.random.randint(thresh), img_h - np.random.randint(thresh)])
|
||||
dst_pts.append(
|
||||
[np.random.randint(thresh), img_h - np.random.randint(thresh)])
|
||||
|
||||
half_thresh = thresh * 0.5
|
||||
|
||||
for cut_idx in np.arange(1, segment, 1):
|
||||
src_pts.append([cut * cut_idx, 0])
|
||||
src_pts.append([cut * cut_idx, img_h])
|
||||
dst_pts.append([
|
||||
cut * cut_idx + np.random.randint(thresh) - half_thresh,
|
||||
np.random.randint(thresh) - half_thresh
|
||||
])
|
||||
dst_pts.append([
|
||||
cut * cut_idx + np.random.randint(thresh) - half_thresh,
|
||||
img_h + np.random.randint(thresh) - half_thresh
|
||||
])
|
||||
|
||||
trans = WarpMLS(src, src_pts, dst_pts, img_w, img_h)
|
||||
dst = trans.generate()
|
||||
|
||||
return dst
|
||||
|
||||
|
||||
def tia_stretch(src, segment=4):
|
||||
img_h, img_w = src.shape[:2]
|
||||
|
||||
cut = img_w // segment
|
||||
thresh = cut * 4 // 5
|
||||
|
||||
src_pts = list()
|
||||
dst_pts = list()
|
||||
|
||||
src_pts.append([0, 0])
|
||||
src_pts.append([img_w, 0])
|
||||
src_pts.append([img_w, img_h])
|
||||
src_pts.append([0, img_h])
|
||||
|
||||
dst_pts.append([0, 0])
|
||||
dst_pts.append([img_w, 0])
|
||||
dst_pts.append([img_w, img_h])
|
||||
dst_pts.append([0, img_h])
|
||||
|
||||
half_thresh = thresh * 0.5
|
||||
|
||||
for cut_idx in np.arange(1, segment, 1):
|
||||
move = np.random.randint(thresh) - half_thresh
|
||||
src_pts.append([cut * cut_idx, 0])
|
||||
src_pts.append([cut * cut_idx, img_h])
|
||||
dst_pts.append([cut * cut_idx + move, 0])
|
||||
dst_pts.append([cut * cut_idx + move, img_h])
|
||||
|
||||
trans = WarpMLS(src, src_pts, dst_pts, img_w, img_h)
|
||||
dst = trans.generate()
|
||||
|
||||
return dst
|
||||
|
||||
|
||||
def tia_perspective(src):
|
||||
img_h, img_w = src.shape[:2]
|
||||
|
||||
thresh = img_h // 2
|
||||
|
||||
src_pts = list()
|
||||
dst_pts = list()
|
||||
|
||||
src_pts.append([0, 0])
|
||||
src_pts.append([img_w, 0])
|
||||
src_pts.append([img_w, img_h])
|
||||
src_pts.append([0, img_h])
|
||||
|
||||
dst_pts.append([0, np.random.randint(thresh)])
|
||||
dst_pts.append([img_w, np.random.randint(thresh)])
|
||||
dst_pts.append([img_w, img_h - np.random.randint(thresh)])
|
||||
dst_pts.append([0, img_h - np.random.randint(thresh)])
|
||||
|
||||
trans = WarpMLS(src, src_pts, dst_pts, img_w, img_h)
|
||||
dst = trans.generate()
|
||||
|
||||
return dst
|
||||
|
||||
|
||||
@PIPELINES.register_module()
|
||||
class RecResizeImg(object):
|
||||
|
||||
def __init__(
|
||||
self,
|
||||
image_shape,
|
||||
infer_mode=False,
|
||||
character_dict_path='./easycv/datasets/ocr/dict/ppocr_keys_v1.txt',
|
||||
padding=True,
|
||||
**kwargs):
|
||||
self.image_shape = image_shape
|
||||
self.infer_mode = infer_mode
|
||||
self.character_dict_path = character_dict_path
|
||||
self.padding = padding
|
||||
|
||||
def __call__(self, data):
|
||||
img = data['img']
|
||||
if self.infer_mode and self.character_dict_path is not None:
|
||||
norm_img, valid_ratio = resize_norm_img_chinese(
|
||||
img, self.image_shape)
|
||||
else:
|
||||
norm_img, valid_ratio = resize_norm_img(img, self.image_shape,
|
||||
self.padding)
|
||||
data['img'] = norm_img
|
||||
data['valid_ratio'] = valid_ratio
|
||||
return data
|
||||
|
||||
|
||||
def resize_norm_img(img, image_shape, padding=True):
|
||||
imgC, imgH, imgW = image_shape
|
||||
h = img.shape[0]
|
||||
w = img.shape[1]
|
||||
if not padding:
|
||||
resized_image = cv2.resize(
|
||||
img, (imgW, imgH), interpolation=cv2.INTER_LINEAR)
|
||||
resized_w = imgW
|
||||
else:
|
||||
ratio = w / float(h)
|
||||
if math.ceil(imgH * ratio) > imgW:
|
||||
resized_w = imgW
|
||||
else:
|
||||
resized_w = int(math.ceil(imgH * ratio))
|
||||
resized_image = cv2.resize(img, (resized_w, imgH))
|
||||
resized_image = resized_image.astype('float32')
|
||||
if image_shape[0] == 1:
|
||||
resized_image = resized_image / 255
|
||||
resized_image = resized_image[np.newaxis, :]
|
||||
else:
|
||||
# resized_image = resized_image.transpose((2, 0, 1)) / 255
|
||||
resized_image = resized_image / 255
|
||||
resized_image -= 0.5
|
||||
resized_image /= 0.5
|
||||
# padding_im = np.zeros((imgC, imgH, imgW), dtype=np.float32)
|
||||
# padding_im[:, :, 0:resized_w] = resized_image
|
||||
padding_im = np.zeros((imgH, imgW, imgC), dtype=np.float32)
|
||||
padding_im[:, 0:resized_w, :] = resized_image
|
||||
valid_ratio = min(1.0, float(resized_w / imgW))
|
||||
return padding_im, valid_ratio
|
||||
|
||||
|
||||
def resize_norm_img_chinese(img, image_shape):
|
||||
imgC, imgH, imgW = image_shape
|
||||
# todo: change to 0 and modified image shape
|
||||
max_wh_ratio = imgW * 1.0 / imgH
|
||||
h, w = img.shape[0], img.shape[1]
|
||||
ratio = w * 1.0 / h
|
||||
max_wh_ratio = max(max_wh_ratio, ratio)
|
||||
imgW = int(imgH * max_wh_ratio)
|
||||
if math.ceil(imgH * ratio) > imgW:
|
||||
resized_w = imgW
|
||||
else:
|
||||
resized_w = int(math.ceil(imgH * ratio))
|
||||
resized_image = cv2.resize(img, (resized_w, imgH))
|
||||
resized_image = resized_image.astype('float32')
|
||||
if image_shape[0] == 1:
|
||||
resized_image = resized_image / 255
|
||||
resized_image = resized_image[np.newaxis, :]
|
||||
else:
|
||||
# resized_image = resized_image.transpose((2, 0, 1)) / 255
|
||||
resized_image = resized_image / 255
|
||||
resized_image -= 0.5
|
||||
resized_image /= 0.5
|
||||
# padding_im = np.zeros((imgC, imgH, imgW), dtype=np.float32)
|
||||
# padding_im[:, :, 0:resized_w] = resized_image
|
||||
padding_im = np.zeros((imgH, imgW, imgC), dtype=np.float32)
|
||||
padding_im[:, 0:resized_w, :] = resized_image
|
||||
valid_ratio = min(1.0, float(resized_w / imgW))
|
||||
return padding_im, valid_ratio
|
||||
|
||||
|
||||
@PIPELINES.register_module()
|
||||
class ClsResizeImg(object):
|
||||
|
||||
def __init__(self, img_shape, **kwargs):
|
||||
self.img_shape = img_shape
|
||||
|
||||
def __call__(self, data):
|
||||
img = data['img']
|
||||
norm_img, _ = resize_norm_img(img, self.img_shape)
|
||||
data['img'] = norm_img
|
||||
return data
|
|
@ -6,6 +6,7 @@ from .detection import *
|
|||
from .face import *
|
||||
from .heads import *
|
||||
from .loss import *
|
||||
from .ocr import *
|
||||
from .pose import TopDown
|
||||
from .registry import BACKBONES, HEADS, LOSSES, MODELS, NECKS
|
||||
from .segmentation import *
|
||||
|
|
|
@ -1,9 +1,11 @@
|
|||
# Copyright (c) Alibaba, Inc. and its affiliates.
|
||||
from .cross_entropy_loss import CrossEntropyLoss
|
||||
from .det_db_loss import DBLoss
|
||||
from .face_keypoint_loss import FacePoseLoss, WingLossWithPose
|
||||
from .focal_loss import FocalLoss, VarifocalLoss
|
||||
from .iou_loss import GIoULoss, IoULoss, YOLOX_IOULoss
|
||||
from .mse_loss import JointsMSELoss
|
||||
from .ocr_rec_multi_loss import MultiLoss
|
||||
from .pytorch_metric_learning import *
|
||||
from .set_criterion import (CDNCriterion, DNCriterion, HungarianMatcher,
|
||||
SetCriterion)
|
||||
|
|
|
@ -0,0 +1,208 @@
|
|||
# Modified from https://github.com/PaddlePaddle/PaddleOCR/blob/release/2.6/ppocr/losses/det_db_loss.py
|
||||
import torch
|
||||
import torch.nn as nn
|
||||
import torch.nn.functional as F
|
||||
|
||||
from easycv.models.builder import LOSSES
|
||||
|
||||
|
||||
class BalanceLoss(nn.Module):
|
||||
|
||||
def __init__(self,
|
||||
balance_loss=True,
|
||||
main_loss_type='DiceLoss',
|
||||
negative_ratio=3,
|
||||
return_origin=False,
|
||||
eps=1e-6,
|
||||
**kwargs):
|
||||
"""
|
||||
The BalanceLoss for Differentiable Binarization text detection
|
||||
args:
|
||||
balance_loss (bool): whether balance loss or not, default is True
|
||||
main_loss_type (str): can only be one of ['CrossEntropy','DiceLoss',
|
||||
'Euclidean','BCELoss', 'MaskL1Loss'], default is 'DiceLoss'.
|
||||
negative_ratio (int|float): float, default is 3.
|
||||
return_origin (bool): whether return unbalanced loss or not, default is False.
|
||||
eps (float): default is 1e-6.
|
||||
"""
|
||||
super(BalanceLoss, self).__init__()
|
||||
self.balance_loss = balance_loss
|
||||
self.main_loss_type = main_loss_type
|
||||
self.negative_ratio = negative_ratio
|
||||
self.return_origin = return_origin
|
||||
self.eps = eps
|
||||
|
||||
if self.main_loss_type == 'CrossEntropy':
|
||||
self.loss = nn.CrossEntropyLoss()
|
||||
elif self.main_loss_type == 'Euclidean':
|
||||
self.loss = nn.MSELoss()
|
||||
elif self.main_loss_type == 'DiceLoss':
|
||||
self.loss = DiceLoss(self.eps)
|
||||
elif self.main_loss_type == 'BCELoss':
|
||||
self.loss = BCELoss(reduction='none')
|
||||
elif self.main_loss_type == 'MaskL1Loss':
|
||||
self.loss = MaskL1Loss(self.eps)
|
||||
else:
|
||||
loss_type = [
|
||||
'CrossEntropy', 'DiceLoss', 'Euclidean', 'BCELoss',
|
||||
'MaskL1Loss'
|
||||
]
|
||||
raise Exception(
|
||||
'main_loss_type in BalanceLoss() can only be one of {}'.format(
|
||||
loss_type))
|
||||
|
||||
def forward(self, pred, gt, mask=None):
|
||||
"""
|
||||
The BalanceLoss for Differentiable Binarization text detection
|
||||
args:
|
||||
pred (variable): predicted feature maps.
|
||||
gt (variable): ground truth feature maps.
|
||||
mask (variable): masked maps.
|
||||
return: (variable) balanced loss
|
||||
"""
|
||||
positive = gt * mask
|
||||
negative = (1 - gt) * mask
|
||||
|
||||
positive_count = int(positive.sum())
|
||||
negative_count = int(
|
||||
min(negative.sum(), positive_count * self.negative_ratio))
|
||||
loss = self.loss(pred, gt, mask=mask)
|
||||
|
||||
if not self.balance_loss:
|
||||
return loss
|
||||
|
||||
positive_loss = positive * loss
|
||||
negative_loss = negative * loss
|
||||
negative_loss = torch.reshape(negative_loss, shape=[-1])
|
||||
if negative_count > 0:
|
||||
sort_loss, _ = negative_loss.sort(descending=True)
|
||||
negative_loss = sort_loss[:negative_count]
|
||||
# negative_loss, _ = paddle.topk(negative_loss, k=negative_count_int)
|
||||
balance_loss = (positive_loss.sum() + negative_loss.sum()) / (
|
||||
positive_count + negative_count + self.eps)
|
||||
else:
|
||||
balance_loss = positive_loss.sum() / (positive_count + self.eps)
|
||||
if self.return_origin:
|
||||
return balance_loss, loss
|
||||
|
||||
return balance_loss
|
||||
|
||||
|
||||
class DiceLoss(nn.Module):
|
||||
'''
|
||||
Loss function from https://arxiv.org/abs/1707.03237,
|
||||
where iou computation is introduced heatmap manner to measure the
|
||||
diversity bwtween tow heatmaps.
|
||||
'''
|
||||
|
||||
def __init__(self, eps=1e-6):
|
||||
super(DiceLoss, self).__init__()
|
||||
self.eps = eps
|
||||
|
||||
def forward(self, pred: torch.Tensor, gt, mask, weights=None):
|
||||
'''
|
||||
pred: one or two heatmaps of shape (N, 1, H, W),
|
||||
the losses of tow heatmaps are added together.
|
||||
gt: (N, 1, H, W)
|
||||
mask: (N, H, W)
|
||||
'''
|
||||
return self._compute(pred, gt, mask, weights)
|
||||
|
||||
def _compute(self, pred, gt, mask, weights):
|
||||
if pred.dim() == 4:
|
||||
pred = pred[:, 0, :, :]
|
||||
gt = gt[:, 0, :, :]
|
||||
assert pred.shape == gt.shape
|
||||
assert pred.shape == mask.shape
|
||||
if weights is not None:
|
||||
assert weights.shape == mask.shape
|
||||
mask = weights * mask
|
||||
intersection = (pred * gt * mask).sum()
|
||||
|
||||
union = (pred * mask).sum() + (gt * mask).sum() + self.eps
|
||||
loss = 1 - 2.0 * intersection / union
|
||||
assert loss <= 1
|
||||
return loss
|
||||
|
||||
|
||||
class MaskL1Loss(nn.Module):
|
||||
|
||||
def __init__(self, eps=1e-6):
|
||||
super(MaskL1Loss, self).__init__()
|
||||
self.eps = eps
|
||||
|
||||
def forward(self, pred: torch.Tensor, gt, mask):
|
||||
loss = (torch.abs(pred - gt) * mask).sum() / (mask.sum() + self.eps)
|
||||
return loss
|
||||
|
||||
|
||||
class BCELoss(nn.Module):
|
||||
|
||||
def __init__(self, reduction='mean'):
|
||||
super(BCELoss, self).__init__()
|
||||
self.reduction = reduction
|
||||
|
||||
def forward(self, input, label, mask=None, weight=None, name=None):
|
||||
loss = F.binary_cross_entropy(input, label, reduction=self.reduction)
|
||||
return loss
|
||||
|
||||
|
||||
@LOSSES.register_module()
|
||||
class DBLoss(nn.Module):
|
||||
"""
|
||||
Differentiable Binarization (DB) Loss Function
|
||||
args:
|
||||
parm (dict): the super paramter for DB Loss
|
||||
"""
|
||||
|
||||
def __init__(self,
|
||||
balance_loss=True,
|
||||
main_loss_type='DiceLoss',
|
||||
alpha=5,
|
||||
beta=10,
|
||||
ohem_ratio=3,
|
||||
eps=1e-6,
|
||||
**kwargs):
|
||||
super(DBLoss, self).__init__()
|
||||
self.alpha = alpha
|
||||
self.beta = beta
|
||||
self.dice_loss = DiceLoss(eps=eps)
|
||||
self.l1_loss = MaskL1Loss(eps=eps)
|
||||
self.bce_loss = BalanceLoss(
|
||||
balance_loss=balance_loss,
|
||||
main_loss_type=main_loss_type,
|
||||
negative_ratio=ohem_ratio)
|
||||
|
||||
def forward(self, predicts, labels):
|
||||
predict_maps = predicts['maps']
|
||||
# label_threshold_map, label_threshold_mask, label_shrink_map, label_shrink_mask = labels[
|
||||
# 1:]
|
||||
label_threshold_map, label_threshold_mask, label_shrink_map, label_shrink_mask = labels[
|
||||
'threshold_map'], labels['threshold_mask'], labels[
|
||||
'shrink_map'], labels['shrink_mask']
|
||||
if len(label_threshold_map.shape) == 4:
|
||||
label_threshold_map = label_threshold_map.squeeze(1)
|
||||
label_threshold_mask = label_threshold_mask.squeeze(1)
|
||||
label_shrink_map = label_shrink_map.squeeze(1)
|
||||
label_shrink_mask = label_shrink_mask.squeeze(1)
|
||||
shrink_maps = predict_maps[:, 0, :, :]
|
||||
threshold_maps = predict_maps[:, 1, :, :]
|
||||
binary_maps = predict_maps[:, 2, :, :]
|
||||
|
||||
loss_shrink_maps = self.bce_loss(shrink_maps, label_shrink_map,
|
||||
label_shrink_mask)
|
||||
loss_threshold_maps = self.l1_loss(threshold_maps, label_threshold_map,
|
||||
label_threshold_mask)
|
||||
loss_binary_maps = self.dice_loss(binary_maps, label_shrink_map,
|
||||
label_shrink_mask)
|
||||
loss_shrink_maps = self.alpha * loss_shrink_maps
|
||||
loss_threshold_maps = self.beta * loss_threshold_maps
|
||||
|
||||
# loss_all = loss_shrink_maps + loss_threshold_maps \
|
||||
# + loss_binary_maps
|
||||
losses = {
|
||||
'loss_shrink_maps': loss_shrink_maps,
|
||||
'loss_threshold_maps': loss_threshold_maps,
|
||||
'loss_binary_maps': loss_binary_maps
|
||||
}
|
||||
return losses
|
|
@ -0,0 +1,102 @@
|
|||
# Modified from https://github.com/PaddlePaddle/PaddleOCR/tree/release/2.6/ppocr/losses
|
||||
import torch
|
||||
from torch import nn
|
||||
|
||||
from easycv.models.builder import LOSSES
|
||||
|
||||
|
||||
@LOSSES.register_module()
|
||||
class CTCLoss(nn.Module):
|
||||
|
||||
def __init__(self, use_focal_loss=False, **kwargs):
|
||||
super(CTCLoss, self).__init__()
|
||||
self.loss_func = nn.CTCLoss(blank=0, reduction='none')
|
||||
self.use_focal_loss = use_focal_loss
|
||||
|
||||
def forward(self, predicts, labels, label_lengths):
|
||||
if isinstance(predicts, (list, tuple)):
|
||||
predicts = predicts[-1]
|
||||
# predicts = predicts.transpose(1, 0, 2)
|
||||
predicts = predicts.permute(1, 0, 2).contiguous()
|
||||
predicts = predicts.log_softmax(2)
|
||||
N, B, _ = predicts.shape
|
||||
preds_lengths = torch.tensor([N] * B, dtype=torch.int32)
|
||||
labels = labels.type(torch.int32)
|
||||
label_lengths = label_lengths.type(torch.int64)
|
||||
|
||||
loss = self.loss_func(predicts, labels, preds_lengths, label_lengths)
|
||||
if self.use_focal_loss:
|
||||
weight = torch.exp(-loss)
|
||||
weight = torch.subtract(torch.tensor([1.0]), weight)
|
||||
weight = torch.square(weight)
|
||||
loss = torch.multiply(loss, weight)
|
||||
loss = loss.mean()
|
||||
return {'loss': loss}
|
||||
|
||||
|
||||
@LOSSES.register_module()
|
||||
class SARLoss(nn.Module):
|
||||
|
||||
def __init__(self, **kwargs):
|
||||
super(SARLoss, self).__init__()
|
||||
ignore_index = kwargs.get('ignore_index', 92) # 6626
|
||||
self.loss_func = torch.nn.CrossEntropyLoss(
|
||||
reduction='mean', ignore_index=ignore_index)
|
||||
|
||||
def forward(self, predicts, label):
|
||||
predict = predicts[:, :
|
||||
-1, :] # ignore last index of outputs to be in same seq_len with targets
|
||||
label = label.type(
|
||||
torch.int64
|
||||
)[:, 1:] # ignore first index of target in loss calculation
|
||||
batch_size, num_steps, num_classes = predict.shape[0], predict.shape[
|
||||
1], predict.shape[2]
|
||||
assert len(label.shape) == len(list(predict.shape)) - 1, \
|
||||
"The target's shape and inputs's shape is [N, d] and [N, num_steps]"
|
||||
|
||||
inputs = torch.reshape(predict, [-1, num_classes])
|
||||
targets = torch.reshape(label, [-1])
|
||||
loss = self.loss_func(inputs, targets)
|
||||
return {'loss': loss}
|
||||
|
||||
|
||||
@LOSSES.register_module()
|
||||
class MultiLoss(nn.Module):
|
||||
|
||||
def __init__(self,
|
||||
loss_config_list,
|
||||
weight_1=1.0,
|
||||
weight_2=1.0,
|
||||
gtc_loss='sar',
|
||||
**kwargs):
|
||||
super().__init__()
|
||||
self.loss_funcs = {}
|
||||
self.loss_list = loss_config_list
|
||||
self.weight_1 = weight_1
|
||||
self.weight_2 = weight_2
|
||||
self.gtc_loss = gtc_loss
|
||||
for loss_info in self.loss_list:
|
||||
for name, param in loss_info.items():
|
||||
if param is not None:
|
||||
kwargs.update(param)
|
||||
loss = eval(name)(**kwargs)
|
||||
self.loss_funcs[name] = loss
|
||||
|
||||
def forward(self, predicts, label_ctc=None, label_sar=None, length=None):
|
||||
self.total_loss = {}
|
||||
total_loss = 0.0
|
||||
# batch [image, label_ctc, label_sar, length, valid_ratio]
|
||||
for name, loss_func in self.loss_funcs.items():
|
||||
if name == 'CTCLoss':
|
||||
loss = loss_func(predicts['ctc'], label_ctc,
|
||||
length)['loss'] * self.weight_1
|
||||
elif name == 'SARLoss':
|
||||
loss = loss_func(predicts['sar'],
|
||||
label_sar)['loss'] * self.weight_2
|
||||
else:
|
||||
raise NotImplementedError(
|
||||
'{} is not supported in MultiLoss yet'.format(name))
|
||||
self.total_loss[name] = loss
|
||||
total_loss += loss
|
||||
self.total_loss['loss'] = total_loss
|
||||
return self.total_loss
|
|
@ -0,0 +1,7 @@
|
|||
# Copyright (c) Alibaba, Inc. and its affiliates.
|
||||
from . import backbones
|
||||
from .cls import TextClassifier
|
||||
from .det import DBNet
|
||||
from .heads import CTCHead, DBHead
|
||||
from .necks import DBFPN, LKPAN, RSEFPN, SequenceEncoder
|
||||
from .rec import OCRRecNet
|
|
@ -0,0 +1,6 @@
|
|||
# Copyright (c) Alibaba, Inc. and its affiliates.
|
||||
from .det_mobilenet_v3 import OCRDetMobileNetV3
|
||||
from .det_resnet_vd import OCRDetResNet
|
||||
from .rec_mobilenet_v3 import OCRRecMobileNetV3
|
||||
from .rec_mv1_enhance import OCRRecMobileNetV1Enhance
|
||||
from .rec_svtrnet import SVTRNet
|
|
@ -0,0 +1,337 @@
|
|||
# Modified from https://github.com/PaddlePaddle/PaddleOCR/blob/release/2.6/ppocr/modeling/backbones/det_mobilenet_v3.py
|
||||
import torch
|
||||
import torch.nn as nn
|
||||
import torch.nn.functional as F
|
||||
|
||||
from easycv.models.registry import BACKBONES
|
||||
|
||||
|
||||
class Hswish(nn.Module):
|
||||
|
||||
def __init__(self, inplace=True):
|
||||
super(Hswish, self).__init__()
|
||||
self.inplace = inplace
|
||||
|
||||
def forward(self, x):
|
||||
return x * F.relu6(x + 3., inplace=self.inplace) / 6.
|
||||
|
||||
|
||||
# out = max(0, min(1, slop*x+offset))
|
||||
# paddle.fluid.layers.hard_sigmoid(x, slope=0.2, offset=0.5, name=None)
|
||||
class Hsigmoid(nn.Module):
|
||||
|
||||
def __init__(self, inplace=True):
|
||||
super(Hsigmoid, self).__init__()
|
||||
self.inplace = inplace
|
||||
|
||||
def forward(self, x):
|
||||
# torch: F.relu6(x + 3., inplace=self.inplace) / 6.
|
||||
# paddle: F.relu6(1.2 * x + 3., inplace=self.inplace) / 6.
|
||||
return F.relu6(1.2 * x + 3., inplace=self.inplace) / 6.
|
||||
|
||||
|
||||
class GELU(nn.Module):
|
||||
|
||||
def __init__(self, inplace=True):
|
||||
super(GELU, self).__init__()
|
||||
self.inplace = inplace
|
||||
|
||||
def forward(self, x):
|
||||
return torch.nn.functional.gelu(x)
|
||||
|
||||
|
||||
class Swish(nn.Module):
|
||||
|
||||
def __init__(self, inplace=True):
|
||||
super(Swish, self).__init__()
|
||||
self.inplace = inplace
|
||||
|
||||
def forward(self, x):
|
||||
if self.inplace:
|
||||
x.mul_(torch.sigmoid(x))
|
||||
return x
|
||||
else:
|
||||
return x * torch.sigmoid(x)
|
||||
|
||||
|
||||
class Activation(nn.Module):
|
||||
|
||||
def __init__(self, act_type, inplace=True):
|
||||
super(Activation, self).__init__()
|
||||
act_type = act_type.lower()
|
||||
if act_type == 'relu':
|
||||
self.act = nn.ReLU(inplace=inplace)
|
||||
elif act_type == 'relu6':
|
||||
self.act = nn.ReLU6(inplace=inplace)
|
||||
elif act_type == 'sigmoid':
|
||||
raise NotImplementedError
|
||||
elif act_type == 'hard_sigmoid':
|
||||
self.act = Hsigmoid(inplace)
|
||||
elif act_type == 'hard_swish':
|
||||
self.act = Hswish(inplace=inplace)
|
||||
elif act_type == 'leakyrelu':
|
||||
self.act = nn.LeakyReLU(inplace=inplace)
|
||||
elif act_type == 'gelu':
|
||||
self.act = GELU(inplace=inplace)
|
||||
elif act_type == 'swish':
|
||||
self.act = Swish(inplace=inplace)
|
||||
else:
|
||||
raise NotImplementedError
|
||||
|
||||
def forward(self, inputs):
|
||||
return self.act(inputs)
|
||||
|
||||
|
||||
def make_divisible(v, divisor=8, min_value=None):
|
||||
if min_value is None:
|
||||
min_value = divisor
|
||||
new_v = max(min_value, int(v + divisor / 2) // divisor * divisor)
|
||||
if new_v < 0.9 * v:
|
||||
new_v += divisor
|
||||
return new_v
|
||||
|
||||
|
||||
class ConvBNLayer(nn.Module):
|
||||
|
||||
def __init__(self,
|
||||
in_channels,
|
||||
out_channels,
|
||||
kernel_size,
|
||||
stride,
|
||||
padding,
|
||||
groups=1,
|
||||
if_act=True,
|
||||
act=None,
|
||||
name=None):
|
||||
super(ConvBNLayer, self).__init__()
|
||||
self.if_act = if_act
|
||||
self.conv = nn.Conv2d(
|
||||
in_channels=in_channels,
|
||||
out_channels=out_channels,
|
||||
kernel_size=kernel_size,
|
||||
stride=stride,
|
||||
padding=padding,
|
||||
groups=groups,
|
||||
bias=False)
|
||||
|
||||
self.bn = nn.BatchNorm2d(out_channels, )
|
||||
if self.if_act:
|
||||
self.act = Activation(act_type=act, inplace=True)
|
||||
|
||||
def forward(self, x):
|
||||
x = self.conv(x)
|
||||
x = self.bn(x)
|
||||
if self.if_act:
|
||||
x = self.act(x)
|
||||
return x
|
||||
|
||||
|
||||
class SEModule(nn.Module):
|
||||
|
||||
def __init__(self, in_channels, reduction=4, name=''):
|
||||
super(SEModule, self).__init__()
|
||||
self.avg_pool = nn.AdaptiveAvgPool2d(1)
|
||||
self.conv1 = nn.Conv2d(
|
||||
in_channels=in_channels,
|
||||
out_channels=in_channels // reduction,
|
||||
kernel_size=1,
|
||||
stride=1,
|
||||
padding=0,
|
||||
bias=True)
|
||||
self.relu1 = Activation(act_type='relu', inplace=True)
|
||||
self.conv2 = nn.Conv2d(
|
||||
in_channels=in_channels // reduction,
|
||||
out_channels=in_channels,
|
||||
kernel_size=1,
|
||||
stride=1,
|
||||
padding=0,
|
||||
bias=True)
|
||||
self.hard_sigmoid = Activation(act_type='hard_sigmoid', inplace=True)
|
||||
|
||||
def forward(self, inputs):
|
||||
outputs = self.avg_pool(inputs)
|
||||
outputs = self.conv1(outputs)
|
||||
outputs = self.relu1(outputs)
|
||||
outputs = self.conv2(outputs)
|
||||
outputs = self.hard_sigmoid(outputs)
|
||||
outputs = inputs * outputs
|
||||
return outputs
|
||||
|
||||
|
||||
class ResidualUnit(nn.Module):
|
||||
|
||||
def __init__(self,
|
||||
in_channels,
|
||||
mid_channels,
|
||||
out_channels,
|
||||
kernel_size,
|
||||
stride,
|
||||
use_se,
|
||||
act=None,
|
||||
name=''):
|
||||
super(ResidualUnit, self).__init__()
|
||||
self.if_shortcut = stride == 1 and in_channels == out_channels
|
||||
self.if_se = use_se
|
||||
|
||||
self.expand_conv = ConvBNLayer(
|
||||
in_channels=in_channels,
|
||||
out_channels=mid_channels,
|
||||
kernel_size=1,
|
||||
stride=1,
|
||||
padding=0,
|
||||
if_act=True,
|
||||
act=act,
|
||||
name=name + '_expand')
|
||||
self.bottleneck_conv = ConvBNLayer(
|
||||
in_channels=mid_channels,
|
||||
out_channels=mid_channels,
|
||||
kernel_size=kernel_size,
|
||||
stride=stride,
|
||||
padding=int((kernel_size - 1) // 2),
|
||||
groups=mid_channels,
|
||||
if_act=True,
|
||||
act=act,
|
||||
name=name + '_depthwise')
|
||||
if self.if_se:
|
||||
self.mid_se = SEModule(mid_channels, name=name + '_se')
|
||||
self.linear_conv = ConvBNLayer(
|
||||
in_channels=mid_channels,
|
||||
out_channels=out_channels,
|
||||
kernel_size=1,
|
||||
stride=1,
|
||||
padding=0,
|
||||
if_act=False,
|
||||
act=None,
|
||||
name=name + '_linear')
|
||||
|
||||
def forward(self, inputs):
|
||||
x = self.expand_conv(inputs)
|
||||
x = self.bottleneck_conv(x)
|
||||
if self.if_se:
|
||||
x = self.mid_se(x)
|
||||
x = self.linear_conv(x)
|
||||
if self.if_shortcut:
|
||||
x = inputs + x
|
||||
return x
|
||||
|
||||
|
||||
@BACKBONES.register_module()
|
||||
class OCRDetMobileNetV3(nn.Module):
|
||||
|
||||
def __init__(self,
|
||||
in_channels=3,
|
||||
model_name='large',
|
||||
scale=0.5,
|
||||
disable_se=False,
|
||||
**kwargs):
|
||||
"""
|
||||
the MobilenetV3 backbone network for detection module.
|
||||
Args:
|
||||
params(dict): the super parameters for build network
|
||||
"""
|
||||
super(OCRDetMobileNetV3, self).__init__()
|
||||
|
||||
self.disable_se = disable_se
|
||||
|
||||
if model_name == 'large':
|
||||
cfg = [
|
||||
# k, exp, c, se, nl, s,
|
||||
[3, 16, 16, False, 'relu', 1],
|
||||
[3, 64, 24, False, 'relu', 2],
|
||||
[3, 72, 24, False, 'relu', 1],
|
||||
[5, 72, 40, True, 'relu', 2],
|
||||
[5, 120, 40, True, 'relu', 1],
|
||||
[5, 120, 40, True, 'relu', 1],
|
||||
[3, 240, 80, False, 'hard_swish', 2],
|
||||
[3, 200, 80, False, 'hard_swish', 1],
|
||||
[3, 184, 80, False, 'hard_swish', 1],
|
||||
[3, 184, 80, False, 'hard_swish', 1],
|
||||
[3, 480, 112, True, 'hard_swish', 1],
|
||||
[3, 672, 112, True, 'hard_swish', 1],
|
||||
[5, 672, 160, True, 'hard_swish', 2],
|
||||
[5, 960, 160, True, 'hard_swish', 1],
|
||||
[5, 960, 160, True, 'hard_swish', 1],
|
||||
]
|
||||
cls_ch_squeeze = 960
|
||||
elif model_name == 'small':
|
||||
cfg = [
|
||||
# k, exp, c, se, nl, s,
|
||||
[3, 16, 16, True, 'relu', 2],
|
||||
[3, 72, 24, False, 'relu', 2],
|
||||
[3, 88, 24, False, 'relu', 1],
|
||||
[5, 96, 40, True, 'hard_swish', 2],
|
||||
[5, 240, 40, True, 'hard_swish', 1],
|
||||
[5, 240, 40, True, 'hard_swish', 1],
|
||||
[5, 120, 48, True, 'hard_swish', 1],
|
||||
[5, 144, 48, True, 'hard_swish', 1],
|
||||
[5, 288, 96, True, 'hard_swish', 2],
|
||||
[5, 576, 96, True, 'hard_swish', 1],
|
||||
[5, 576, 96, True, 'hard_swish', 1],
|
||||
]
|
||||
cls_ch_squeeze = 576
|
||||
else:
|
||||
raise NotImplementedError('mode[' + model_name +
|
||||
'_model] is not implemented!')
|
||||
|
||||
supported_scale = [0.35, 0.5, 0.75, 1.0, 1.25]
|
||||
assert scale in supported_scale, \
|
||||
'supported scale are {} but input scale is {}'.format(supported_scale, scale)
|
||||
inplanes = 16
|
||||
# conv1
|
||||
self.conv = ConvBNLayer(
|
||||
in_channels=in_channels,
|
||||
out_channels=make_divisible(inplanes * scale),
|
||||
kernel_size=3,
|
||||
stride=2,
|
||||
padding=1,
|
||||
groups=1,
|
||||
if_act=True,
|
||||
act='hard_swish',
|
||||
name='conv1')
|
||||
|
||||
self.stages = nn.ModuleList()
|
||||
self.out_channels = []
|
||||
block_list = []
|
||||
i = 0
|
||||
inplanes = make_divisible(inplanes * scale)
|
||||
for (k, exp, c, se, nl, s) in cfg:
|
||||
se = se and not self.disable_se
|
||||
if s == 2 and i > 2:
|
||||
self.out_channels.append(inplanes)
|
||||
self.stages.append(nn.Sequential(*block_list))
|
||||
block_list = []
|
||||
block_list.append(
|
||||
ResidualUnit(
|
||||
in_channels=inplanes,
|
||||
mid_channels=make_divisible(scale * exp),
|
||||
out_channels=make_divisible(scale * c),
|
||||
kernel_size=k,
|
||||
stride=s,
|
||||
use_se=se,
|
||||
act=nl,
|
||||
name='conv' + str(i + 2)))
|
||||
inplanes = make_divisible(scale * c)
|
||||
i += 1
|
||||
block_list.append(
|
||||
ConvBNLayer(
|
||||
in_channels=inplanes,
|
||||
out_channels=make_divisible(scale * cls_ch_squeeze),
|
||||
kernel_size=1,
|
||||
stride=1,
|
||||
padding=0,
|
||||
groups=1,
|
||||
if_act=True,
|
||||
act='hard_swish',
|
||||
name='conv_last'))
|
||||
self.stages.append(nn.Sequential(*block_list))
|
||||
self.out_channels.append(make_divisible(scale * cls_ch_squeeze))
|
||||
# for i, stage in enumerate(self.stages):
|
||||
# self.add_sublayer(sublayer=stage, name="stage{}".format(i))
|
||||
|
||||
def forward(self, x):
|
||||
x = self.conv(x)
|
||||
out_list = []
|
||||
for stage in self.stages:
|
||||
x = stage(x)
|
||||
out_list.append(x)
|
||||
return out_list
|
|
@ -0,0 +1,276 @@
|
|||
# Modified from https://github.com/PaddlePaddle/PaddleOCR/blob/release/2.6/ppocr/modeling/backbones/det_resnet_vd.py
|
||||
import torch
|
||||
import torch.nn as nn
|
||||
import torch.nn.functional as F
|
||||
|
||||
from easycv.models.registry import BACKBONES
|
||||
from .det_mobilenet_v3 import Activation
|
||||
|
||||
|
||||
class ConvBNLayer(nn.Module):
|
||||
|
||||
def __init__(
|
||||
self,
|
||||
in_channels,
|
||||
out_channels,
|
||||
kernel_size,
|
||||
stride=1,
|
||||
groups=1,
|
||||
is_vd_mode=False,
|
||||
act=None,
|
||||
name=None,
|
||||
):
|
||||
super(ConvBNLayer, self).__init__()
|
||||
|
||||
self.is_vd_mode = is_vd_mode
|
||||
self.act = act
|
||||
self._pool2d_avg = nn.AvgPool2d(
|
||||
kernel_size=2, stride=2, padding=0, ceil_mode=True)
|
||||
self._conv = nn.Conv2d(
|
||||
in_channels=in_channels,
|
||||
out_channels=out_channels,
|
||||
kernel_size=kernel_size,
|
||||
stride=stride,
|
||||
padding=(kernel_size - 1) // 2,
|
||||
groups=groups,
|
||||
bias=False)
|
||||
if name == 'conv1':
|
||||
bn_name = 'bn_' + name
|
||||
else:
|
||||
bn_name = 'bn' + name[3:]
|
||||
self._batch_norm = nn.BatchNorm2d(
|
||||
out_channels,
|
||||
track_running_stats=True,
|
||||
)
|
||||
|
||||
if act is not None:
|
||||
self._act = Activation(act_type=act, inplace=True)
|
||||
|
||||
def forward(self, inputs):
|
||||
if self.is_vd_mode:
|
||||
inputs = self._pool2d_avg(inputs)
|
||||
y = self._conv(inputs)
|
||||
y = self._batch_norm(y)
|
||||
if self.act is not None:
|
||||
y = self._act(y)
|
||||
return y
|
||||
|
||||
|
||||
class BottleneckBlock(nn.Module):
|
||||
|
||||
def __init__(self,
|
||||
in_channels,
|
||||
out_channels,
|
||||
stride,
|
||||
shortcut=True,
|
||||
if_first=False,
|
||||
name=None):
|
||||
super(BottleneckBlock, self).__init__()
|
||||
|
||||
self.conv0 = ConvBNLayer(
|
||||
in_channels=in_channels,
|
||||
out_channels=out_channels,
|
||||
kernel_size=1,
|
||||
act='relu',
|
||||
name=name + '_branch2a')
|
||||
self.conv1 = ConvBNLayer(
|
||||
in_channels=out_channels,
|
||||
out_channels=out_channels,
|
||||
kernel_size=3,
|
||||
stride=stride,
|
||||
act='relu',
|
||||
name=name + '_branch2b')
|
||||
self.conv2 = ConvBNLayer(
|
||||
in_channels=out_channels,
|
||||
out_channels=out_channels * 4,
|
||||
kernel_size=1,
|
||||
act=None,
|
||||
name=name + '_branch2c')
|
||||
|
||||
if not shortcut:
|
||||
self.short = ConvBNLayer(
|
||||
in_channels=in_channels,
|
||||
out_channels=out_channels * 4,
|
||||
kernel_size=1,
|
||||
stride=1,
|
||||
is_vd_mode=False if if_first else True,
|
||||
name=name + '_branch1')
|
||||
|
||||
self.shortcut = shortcut
|
||||
|
||||
def forward(self, inputs):
|
||||
y = self.conv0(inputs)
|
||||
conv1 = self.conv1(y)
|
||||
conv2 = self.conv2(conv1)
|
||||
|
||||
if self.shortcut:
|
||||
short = inputs
|
||||
else:
|
||||
short = self.short(inputs)
|
||||
y = torch.add(short, conv2)
|
||||
y = F.relu(y)
|
||||
return y
|
||||
|
||||
|
||||
class BasicBlock(nn.Module):
|
||||
|
||||
def __init__(self,
|
||||
in_channels,
|
||||
out_channels,
|
||||
stride,
|
||||
shortcut=True,
|
||||
if_first=False,
|
||||
name=None):
|
||||
super(BasicBlock, self).__init__()
|
||||
self.stride = stride
|
||||
self.conv0 = ConvBNLayer(
|
||||
in_channels=in_channels,
|
||||
out_channels=out_channels,
|
||||
kernel_size=3,
|
||||
stride=stride,
|
||||
act='relu',
|
||||
name=name + '_branch2a')
|
||||
self.conv1 = ConvBNLayer(
|
||||
in_channels=out_channels,
|
||||
out_channels=out_channels,
|
||||
kernel_size=3,
|
||||
act=None,
|
||||
name=name + '_branch2b')
|
||||
|
||||
if not shortcut:
|
||||
self.short = ConvBNLayer(
|
||||
in_channels=in_channels,
|
||||
out_channels=out_channels,
|
||||
kernel_size=1,
|
||||
stride=1,
|
||||
is_vd_mode=False if if_first else True,
|
||||
name=name + '_branch1')
|
||||
|
||||
self.shortcut = shortcut
|
||||
|
||||
def forward(self, inputs):
|
||||
y = self.conv0(inputs)
|
||||
conv1 = self.conv1(y)
|
||||
|
||||
if self.shortcut:
|
||||
short = inputs
|
||||
else:
|
||||
short = self.short(inputs)
|
||||
y = short + conv1
|
||||
y = F.relu(y)
|
||||
return y
|
||||
|
||||
|
||||
@BACKBONES.register_module()
|
||||
class OCRDetResNet(nn.Module):
|
||||
|
||||
def __init__(self, in_channels=3, layers=50, **kwargs):
|
||||
super(OCRDetResNet, self).__init__()
|
||||
|
||||
self.layers = layers
|
||||
supported_layers = [18, 34, 50, 101, 152, 200]
|
||||
assert layers in supported_layers, \
|
||||
'supported layers are {} but input layer is {}'.format(
|
||||
supported_layers, layers)
|
||||
|
||||
if layers == 18:
|
||||
depth = [2, 2, 2, 2]
|
||||
elif layers == 34 or layers == 50:
|
||||
depth = [3, 4, 6, 3]
|
||||
elif layers == 101:
|
||||
depth = [3, 4, 23, 3]
|
||||
elif layers == 152:
|
||||
depth = [3, 8, 36, 3]
|
||||
elif layers == 200:
|
||||
depth = [3, 12, 48, 3]
|
||||
num_channels = [64, 256, 512, 1024
|
||||
] if layers >= 50 else [64, 64, 128, 256]
|
||||
num_filters = [64, 128, 256, 512]
|
||||
|
||||
self.conv1_1 = ConvBNLayer(
|
||||
in_channels=in_channels,
|
||||
out_channels=32,
|
||||
kernel_size=3,
|
||||
stride=2,
|
||||
act='relu',
|
||||
name='conv1_1')
|
||||
self.conv1_2 = ConvBNLayer(
|
||||
in_channels=32,
|
||||
out_channels=32,
|
||||
kernel_size=3,
|
||||
stride=1,
|
||||
act='relu',
|
||||
name='conv1_2')
|
||||
self.conv1_3 = ConvBNLayer(
|
||||
in_channels=32,
|
||||
out_channels=64,
|
||||
kernel_size=3,
|
||||
stride=1,
|
||||
act='relu',
|
||||
name='conv1_3')
|
||||
self.pool2d_max = nn.MaxPool2d(kernel_size=3, stride=2, padding=1)
|
||||
|
||||
self.stages = nn.ModuleList()
|
||||
self.out_channels = []
|
||||
if layers >= 50:
|
||||
for block in range(len(depth)):
|
||||
# block_list = []
|
||||
block_list = nn.Sequential()
|
||||
shortcut = False
|
||||
for i in range(depth[block]):
|
||||
if layers in [101, 152] and block == 2:
|
||||
if i == 0:
|
||||
conv_name = 'res' + str(block + 2) + 'a'
|
||||
else:
|
||||
conv_name = 'res' + str(block + 2) + 'b' + str(i)
|
||||
else:
|
||||
conv_name = 'res' + str(block + 2) + chr(97 + i)
|
||||
bottleneck_block = BottleneckBlock(
|
||||
in_channels=num_channels[block]
|
||||
if i == 0 else num_filters[block] * 4,
|
||||
out_channels=num_filters[block],
|
||||
stride=2 if i == 0 and block != 0 else 1,
|
||||
shortcut=shortcut,
|
||||
if_first=block == i == 0,
|
||||
name=conv_name)
|
||||
|
||||
shortcut = True
|
||||
block_list.add_module('bb_%d_%d' % (block, i),
|
||||
bottleneck_block)
|
||||
self.out_channels.append(num_filters[block] * 4)
|
||||
# self.stages.append(nn.Sequential(*block_list))
|
||||
self.stages.append(block_list)
|
||||
else:
|
||||
for block in range(len(depth)):
|
||||
# block_list = []
|
||||
block_list = nn.Sequential()
|
||||
shortcut = False
|
||||
for i in range(depth[block]):
|
||||
conv_name = 'res' + str(block + 2) + chr(97 + i)
|
||||
basic_block = BasicBlock(
|
||||
in_channels=num_channels[block]
|
||||
if i == 0 else num_filters[block],
|
||||
out_channels=num_filters[block],
|
||||
stride=2 if i == 0 and block != 0 else 1,
|
||||
shortcut=shortcut,
|
||||
if_first=block == i == 0,
|
||||
name=conv_name)
|
||||
|
||||
shortcut = True
|
||||
block_list.add_module('bb_%d_%d' % (block, i), basic_block)
|
||||
# block_list.append(basic_block)
|
||||
self.out_channels.append(num_filters[block])
|
||||
self.stages.append(block_list)
|
||||
|
||||
# self.stages.append(nn.Sequential(*block_list))
|
||||
|
||||
def forward(self, inputs):
|
||||
y = self.conv1_1(inputs)
|
||||
y = self.conv1_2(y)
|
||||
y = self.conv1_3(y)
|
||||
y = self.pool2d_max(y)
|
||||
out = []
|
||||
for block in self.stages:
|
||||
y = block(y)
|
||||
out.append(y)
|
||||
return out
|
|
@ -0,0 +1,129 @@
|
|||
# Modified from https://github.com/PaddlePaddle/PaddleOCR/blob/release/2.6/ppocr/modeling/backbones/rec_mobilenet_v3.py
|
||||
import torch.nn as nn
|
||||
|
||||
from easycv.models.registry import BACKBONES
|
||||
from .det_mobilenet_v3 import (Activation, ConvBNLayer, ResidualUnit,
|
||||
make_divisible)
|
||||
|
||||
|
||||
@BACKBONES.register_module()
|
||||
class OCRRecMobileNetV3(nn.Module):
|
||||
"""mobilenetv3 backbone for ocr recognition
|
||||
"""
|
||||
|
||||
def __init__(self,
|
||||
in_channels=3,
|
||||
model_name='small',
|
||||
scale=0.5,
|
||||
large_stride=None,
|
||||
small_stride=None,
|
||||
**kwargs):
|
||||
super(OCRRecMobileNetV3, self).__init__()
|
||||
if small_stride is None:
|
||||
small_stride = [2, 2, 2, 2]
|
||||
if large_stride is None:
|
||||
large_stride = [1, 2, 2, 2]
|
||||
|
||||
assert isinstance(large_stride, list), 'large_stride type must ' \
|
||||
'be list but got {}'.format(type(large_stride))
|
||||
assert isinstance(small_stride, list), 'small_stride type must ' \
|
||||
'be list but got {}'.format(type(small_stride))
|
||||
assert len(large_stride) == 4, 'large_stride length must be ' \
|
||||
'4 but got {}'.format(len(large_stride))
|
||||
assert len(small_stride) == 4, 'small_stride length must be ' \
|
||||
'4 but got {}'.format(len(small_stride))
|
||||
|
||||
if model_name == 'large':
|
||||
cfg = [
|
||||
# k, exp, c, se, nl, s,
|
||||
[3, 16, 16, False, 'relu', large_stride[0]],
|
||||
[3, 64, 24, False, 'relu', (large_stride[1], 1)],
|
||||
[3, 72, 24, False, 'relu', 1],
|
||||
[5, 72, 40, True, 'relu', (large_stride[2], 1)],
|
||||
[5, 120, 40, True, 'relu', 1],
|
||||
[5, 120, 40, True, 'relu', 1],
|
||||
[3, 240, 80, False, 'hard_swish', 1],
|
||||
[3, 200, 80, False, 'hard_swish', 1],
|
||||
[3, 184, 80, False, 'hard_swish', 1],
|
||||
[3, 184, 80, False, 'hard_swish', 1],
|
||||
[3, 480, 112, True, 'hard_swish', 1],
|
||||
[3, 672, 112, True, 'hard_swish', 1],
|
||||
[5, 672, 160, True, 'hard_swish', (large_stride[3], 1)],
|
||||
[5, 960, 160, True, 'hard_swish', 1],
|
||||
[5, 960, 160, True, 'hard_swish', 1],
|
||||
]
|
||||
cls_ch_squeeze = 960
|
||||
elif model_name == 'small':
|
||||
cfg = [
|
||||
# k, exp, c, se, nl, s,
|
||||
[3, 16, 16, True, 'relu', (small_stride[0], 1)],
|
||||
[3, 72, 24, False, 'relu', (small_stride[1], 1)],
|
||||
[3, 88, 24, False, 'relu', 1],
|
||||
[5, 96, 40, True, 'hard_swish', (small_stride[2], 1)],
|
||||
[5, 240, 40, True, 'hard_swish', 1],
|
||||
[5, 240, 40, True, 'hard_swish', 1],
|
||||
[5, 120, 48, True, 'hard_swish', 1],
|
||||
[5, 144, 48, True, 'hard_swish', 1],
|
||||
[5, 288, 96, True, 'hard_swish', (small_stride[3], 1)],
|
||||
[5, 576, 96, True, 'hard_swish', 1],
|
||||
[5, 576, 96, True, 'hard_swish', 1],
|
||||
]
|
||||
cls_ch_squeeze = 576
|
||||
else:
|
||||
raise NotImplementedError('mode[' + model_name +
|
||||
'_model] is not implemented!')
|
||||
|
||||
supported_scale = [0.35, 0.5, 0.75, 1.0, 1.25]
|
||||
assert scale in supported_scale, \
|
||||
'supported scales are {} but input scale is {}'.format(supported_scale, scale)
|
||||
|
||||
inplanes = 16
|
||||
# conv1
|
||||
self.conv1 = ConvBNLayer(
|
||||
in_channels=in_channels,
|
||||
out_channels=make_divisible(inplanes * scale),
|
||||
kernel_size=3,
|
||||
stride=2,
|
||||
padding=1,
|
||||
groups=1,
|
||||
if_act=True,
|
||||
act='hard_swish',
|
||||
name='conv1')
|
||||
i = 0
|
||||
block_list = []
|
||||
inplanes = make_divisible(inplanes * scale)
|
||||
for (k, exp, c, se, nl, s) in cfg:
|
||||
block_list.append(
|
||||
ResidualUnit(
|
||||
in_channels=inplanes,
|
||||
mid_channels=make_divisible(scale * exp),
|
||||
out_channels=make_divisible(scale * c),
|
||||
kernel_size=k,
|
||||
stride=s,
|
||||
use_se=se,
|
||||
act=nl,
|
||||
name='conv' + str(i + 2)))
|
||||
inplanes = make_divisible(scale * c)
|
||||
i += 1
|
||||
self.blocks = nn.Sequential(*block_list)
|
||||
|
||||
self.conv2 = ConvBNLayer(
|
||||
in_channels=inplanes,
|
||||
out_channels=make_divisible(scale * cls_ch_squeeze),
|
||||
kernel_size=1,
|
||||
stride=1,
|
||||
padding=0,
|
||||
groups=1,
|
||||
if_act=True,
|
||||
act='hard_swish',
|
||||
name='conv_last')
|
||||
|
||||
self.pool = nn.MaxPool2d(kernel_size=2, stride=2, padding=0)
|
||||
self.out_channels = make_divisible(scale * cls_ch_squeeze)
|
||||
|
||||
def forward(self, x):
|
||||
x = self.conv1(x)
|
||||
x = self.blocks(x)
|
||||
x = self.conv2(x)
|
||||
x = self.pool(x)
|
||||
return x
|
|
@ -0,0 +1,240 @@
|
|||
# Modified from https://github.com/PaddlePaddle/PaddleOCR/blob/release/2.6/ppocr/modeling/backbones/rec_mv1_enhance.py
|
||||
import torch
|
||||
import torch.nn as nn
|
||||
import torch.nn.functional as F
|
||||
|
||||
from easycv.models.registry import BACKBONES
|
||||
from .det_mobilenet_v3 import Activation
|
||||
|
||||
|
||||
class ConvBNLayer(nn.Module):
|
||||
|
||||
def __init__(self,
|
||||
num_channels,
|
||||
filter_size,
|
||||
num_filters,
|
||||
stride,
|
||||
padding,
|
||||
channels=None,
|
||||
num_groups=1,
|
||||
act='hard_swish'):
|
||||
super(ConvBNLayer, self).__init__()
|
||||
self.act = act
|
||||
self._conv = nn.Conv2d(
|
||||
in_channels=num_channels,
|
||||
out_channels=num_filters,
|
||||
kernel_size=filter_size,
|
||||
stride=stride,
|
||||
padding=padding,
|
||||
groups=num_groups,
|
||||
bias=False)
|
||||
|
||||
self._batch_norm = nn.BatchNorm2d(num_filters, )
|
||||
if self.act is not None:
|
||||
self._act = Activation(act_type=act, inplace=True)
|
||||
|
||||
def forward(self, inputs):
|
||||
y = self._conv(inputs)
|
||||
y = self._batch_norm(y)
|
||||
if self.act is not None:
|
||||
y = self._act(y)
|
||||
return y
|
||||
|
||||
|
||||
class DepthwiseSeparable(nn.Module):
|
||||
|
||||
def __init__(self,
|
||||
num_channels,
|
||||
num_filters1,
|
||||
num_filters2,
|
||||
num_groups,
|
||||
stride,
|
||||
scale,
|
||||
dw_size=3,
|
||||
padding=1,
|
||||
use_se=False):
|
||||
super(DepthwiseSeparable, self).__init__()
|
||||
self.use_se = use_se
|
||||
self._depthwise_conv = ConvBNLayer(
|
||||
num_channels=num_channels,
|
||||
num_filters=int(num_filters1 * scale),
|
||||
filter_size=dw_size,
|
||||
stride=stride,
|
||||
padding=padding,
|
||||
num_groups=int(num_groups * scale))
|
||||
if use_se:
|
||||
self._se = SEModule(int(num_filters1 * scale))
|
||||
self._pointwise_conv = ConvBNLayer(
|
||||
num_channels=int(num_filters1 * scale),
|
||||
filter_size=1,
|
||||
num_filters=int(num_filters2 * scale),
|
||||
stride=1,
|
||||
padding=0)
|
||||
|
||||
def forward(self, inputs):
|
||||
y = self._depthwise_conv(inputs)
|
||||
if self.use_se:
|
||||
y = self._se(y)
|
||||
y = self._pointwise_conv(y)
|
||||
return y
|
||||
|
||||
|
||||
@BACKBONES.register_module()
|
||||
class OCRRecMobileNetV1Enhance(nn.Module):
|
||||
|
||||
def __init__(self,
|
||||
in_channels=3,
|
||||
scale=0.5,
|
||||
last_conv_stride=1,
|
||||
last_pool_type='max',
|
||||
**kwargs):
|
||||
super().__init__()
|
||||
self.scale = scale
|
||||
self.block_list = []
|
||||
|
||||
self.conv1 = ConvBNLayer(
|
||||
num_channels=in_channels,
|
||||
filter_size=3,
|
||||
channels=3,
|
||||
num_filters=int(32 * scale),
|
||||
stride=2,
|
||||
padding=1)
|
||||
|
||||
conv2_1 = DepthwiseSeparable(
|
||||
num_channels=int(32 * scale),
|
||||
num_filters1=32,
|
||||
num_filters2=64,
|
||||
num_groups=32,
|
||||
stride=1,
|
||||
scale=scale)
|
||||
self.block_list.append(conv2_1)
|
||||
|
||||
conv2_2 = DepthwiseSeparable(
|
||||
num_channels=int(64 * scale),
|
||||
num_filters1=64,
|
||||
num_filters2=128,
|
||||
num_groups=64,
|
||||
stride=1,
|
||||
scale=scale)
|
||||
self.block_list.append(conv2_2)
|
||||
|
||||
conv3_1 = DepthwiseSeparable(
|
||||
num_channels=int(128 * scale),
|
||||
num_filters1=128,
|
||||
num_filters2=128,
|
||||
num_groups=128,
|
||||
stride=1,
|
||||
scale=scale)
|
||||
self.block_list.append(conv3_1)
|
||||
|
||||
conv3_2 = DepthwiseSeparable(
|
||||
num_channels=int(128 * scale),
|
||||
num_filters1=128,
|
||||
num_filters2=256,
|
||||
num_groups=128,
|
||||
stride=(2, 1),
|
||||
scale=scale)
|
||||
self.block_list.append(conv3_2)
|
||||
|
||||
conv4_1 = DepthwiseSeparable(
|
||||
num_channels=int(256 * scale),
|
||||
num_filters1=256,
|
||||
num_filters2=256,
|
||||
num_groups=256,
|
||||
stride=1,
|
||||
scale=scale)
|
||||
self.block_list.append(conv4_1)
|
||||
|
||||
conv4_2 = DepthwiseSeparable(
|
||||
num_channels=int(256 * scale),
|
||||
num_filters1=256,
|
||||
num_filters2=512,
|
||||
num_groups=256,
|
||||
stride=(2, 1),
|
||||
scale=scale)
|
||||
self.block_list.append(conv4_2)
|
||||
|
||||
for _ in range(5):
|
||||
conv5 = DepthwiseSeparable(
|
||||
num_channels=int(512 * scale),
|
||||
num_filters1=512,
|
||||
num_filters2=512,
|
||||
num_groups=512,
|
||||
stride=1,
|
||||
dw_size=5,
|
||||
padding=2,
|
||||
scale=scale,
|
||||
use_se=False)
|
||||
self.block_list.append(conv5)
|
||||
|
||||
conv5_6 = DepthwiseSeparable(
|
||||
num_channels=int(512 * scale),
|
||||
num_filters1=512,
|
||||
num_filters2=1024,
|
||||
num_groups=512,
|
||||
stride=(2, 1),
|
||||
dw_size=5,
|
||||
padding=2,
|
||||
scale=scale,
|
||||
use_se=True)
|
||||
self.block_list.append(conv5_6)
|
||||
|
||||
conv6 = DepthwiseSeparable(
|
||||
num_channels=int(1024 * scale),
|
||||
num_filters1=1024,
|
||||
num_filters2=1024,
|
||||
num_groups=1024,
|
||||
stride=last_conv_stride,
|
||||
dw_size=5,
|
||||
padding=2,
|
||||
use_se=True,
|
||||
scale=scale)
|
||||
self.block_list.append(conv6)
|
||||
|
||||
self.block_list = nn.Sequential(*self.block_list)
|
||||
if last_pool_type == 'avg':
|
||||
self.pool = nn.AvgPool2d(kernel_size=2, stride=2, padding=0)
|
||||
else:
|
||||
self.pool = nn.MaxPool2d(kernel_size=2, stride=2, padding=0)
|
||||
self.out_channels = int(1024 * scale)
|
||||
|
||||
def forward(self, inputs):
|
||||
y = self.conv1(inputs)
|
||||
y = self.block_list(y)
|
||||
y = self.pool(y)
|
||||
return y
|
||||
|
||||
|
||||
def hardsigmoid(x):
|
||||
return F.relu6(x + 3., inplace=True) / 6.
|
||||
|
||||
|
||||
class SEModule(nn.Module):
|
||||
|
||||
def __init__(self, channel, reduction=4):
|
||||
super(SEModule, self).__init__()
|
||||
self.avg_pool = nn.AdaptiveAvgPool2d(1)
|
||||
self.conv1 = nn.Conv2d(
|
||||
in_channels=channel,
|
||||
out_channels=channel // reduction,
|
||||
kernel_size=1,
|
||||
stride=1,
|
||||
padding=0,
|
||||
bias=True)
|
||||
self.conv2 = nn.Conv2d(
|
||||
in_channels=channel // reduction,
|
||||
out_channels=channel,
|
||||
kernel_size=1,
|
||||
stride=1,
|
||||
padding=0,
|
||||
bias=True)
|
||||
|
||||
def forward(self, inputs):
|
||||
outputs = self.avg_pool(inputs)
|
||||
outputs = self.conv1(outputs)
|
||||
outputs = F.relu(outputs)
|
||||
outputs = self.conv2(outputs)
|
||||
outputs = hardsigmoid(outputs)
|
||||
x = torch.mul(inputs, outputs)
|
||||
|
||||
return x
|
|
@ -0,0 +1,569 @@
|
|||
# Modified from https://github.com/PaddlePaddle/PaddleOCR/blob/release/2.6/ppocr/modeling/backbones/rec_svtrnet.py
|
||||
import numpy as np
|
||||
import torch
|
||||
import torch.nn as nn
|
||||
|
||||
from easycv.models.registry import BACKBONES
|
||||
from easycv.models.utils.transformer import DropPath
|
||||
from .det_mobilenet_v3 import Activation
|
||||
|
||||
|
||||
class ConvBNLayer(nn.Module):
|
||||
|
||||
def __init__(self,
|
||||
in_channels,
|
||||
out_channels,
|
||||
kernel_size=3,
|
||||
stride=1,
|
||||
padding=0,
|
||||
bias_attr=False,
|
||||
groups=1,
|
||||
act='gelu'):
|
||||
super().__init__()
|
||||
self.conv = nn.Conv2d(
|
||||
in_channels=in_channels,
|
||||
out_channels=out_channels,
|
||||
kernel_size=kernel_size,
|
||||
stride=stride,
|
||||
padding=padding,
|
||||
groups=groups,
|
||||
bias=bias_attr)
|
||||
self.norm = nn.BatchNorm2d(out_channels)
|
||||
self.act = Activation(act_type=act, inplace=True)
|
||||
|
||||
def forward(self, inputs):
|
||||
out = self.conv(inputs)
|
||||
out = self.norm(out)
|
||||
out = self.act(out)
|
||||
return out
|
||||
|
||||
|
||||
class Identity(nn.Module):
|
||||
|
||||
def __init__(self):
|
||||
super(Identity, self).__init__()
|
||||
|
||||
def forward(self, input):
|
||||
return input
|
||||
|
||||
|
||||
class Mlp(nn.Module):
|
||||
|
||||
def __init__(self,
|
||||
in_features,
|
||||
hidden_features=None,
|
||||
out_features=None,
|
||||
act_layer='gelu',
|
||||
drop=0.):
|
||||
super().__init__()
|
||||
out_features = out_features or in_features
|
||||
hidden_features = hidden_features or in_features
|
||||
self.fc1 = nn.Linear(in_features, hidden_features)
|
||||
self.act = Activation(act_type=act_layer, inplace=True)
|
||||
self.fc2 = nn.Linear(hidden_features, out_features)
|
||||
self.drop = nn.Dropout(drop)
|
||||
|
||||
def forward(self, x):
|
||||
x = self.fc1(x)
|
||||
x = self.act(x)
|
||||
x = self.drop(x)
|
||||
x = self.fc2(x)
|
||||
x = self.drop(x)
|
||||
return x
|
||||
|
||||
|
||||
class ConvMixer(nn.Module):
|
||||
|
||||
def __init__(
|
||||
self,
|
||||
dim,
|
||||
num_heads=8,
|
||||
HW=[8, 25],
|
||||
local_k=[3, 3],
|
||||
):
|
||||
super().__init__()
|
||||
self.HW = HW
|
||||
self.dim = dim
|
||||
self.local_mixer = nn.Conv2d(
|
||||
dim,
|
||||
dim,
|
||||
local_k,
|
||||
1,
|
||||
[local_k[0] // 2, local_k[1] // 2],
|
||||
groups=num_heads,
|
||||
)
|
||||
|
||||
def forward(self, x):
|
||||
h = self.HW[0]
|
||||
w = self.HW[1]
|
||||
x = x.transpose([0, 2, 1]).reshape([0, self.dim, h, w])
|
||||
x = self.local_mixer(x)
|
||||
x = x.flatten(2).permute(0, 2, 1)
|
||||
return x
|
||||
|
||||
|
||||
class Attention(nn.Module):
|
||||
|
||||
def __init__(self,
|
||||
dim,
|
||||
num_heads=8,
|
||||
mixer='Global',
|
||||
HW=[8, 25],
|
||||
local_k=[7, 11],
|
||||
qkv_bias=False,
|
||||
qk_scale=None,
|
||||
attn_drop=0.,
|
||||
proj_drop=0.):
|
||||
super().__init__()
|
||||
self.num_heads = num_heads
|
||||
head_dim = dim // num_heads
|
||||
self.scale = qk_scale or head_dim**-0.5
|
||||
self.qkv = nn.Linear(dim, dim * 3, bias=qkv_bias)
|
||||
self.attn_drop = nn.Dropout(attn_drop)
|
||||
self.proj = nn.Linear(dim, dim)
|
||||
self.proj_drop = nn.Dropout(proj_drop)
|
||||
self.HW = HW
|
||||
if HW is not None:
|
||||
H = HW[0]
|
||||
W = HW[1]
|
||||
self.N = H * W
|
||||
self.C = dim
|
||||
if mixer == 'Local' and HW is not None:
|
||||
hk = local_k[0]
|
||||
wk = local_k[1]
|
||||
mask = torch.ones(
|
||||
H * W, H + hk - 1, W + wk - 1, dtype=torch.float32)
|
||||
for h in range(0, H):
|
||||
for w in range(0, W):
|
||||
mask[h * W + w, h:h + hk, w:w + wk] = 0.
|
||||
mask_paddle = mask[:, hk // 2:H + hk // 2,
|
||||
wk // 2:W + wk // 2].flatten(1)
|
||||
mask_inf = torch.full([H * W, H * W],
|
||||
fill_value=float('-Inf'),
|
||||
dtype=torch.float32)
|
||||
mask = torch.where(mask_paddle < 1, mask_paddle, mask_inf)
|
||||
self.mask = mask.unsqueeze(1).unsqueeze(0)
|
||||
# self.mask = mask[None, None, :]
|
||||
self.mixer = mixer
|
||||
|
||||
def forward(self, x):
|
||||
if self.HW is not None:
|
||||
N = self.N
|
||||
C = self.C
|
||||
else:
|
||||
_, N, C = x.shape
|
||||
|
||||
qkv = self.qkv(x).reshape(
|
||||
(-1, N, 3, self.num_heads,
|
||||
C // self.num_heads)).permute(2, 0, 3, 1, 4)
|
||||
q, k, v = qkv[0] * self.scale, qkv[1], qkv[2]
|
||||
|
||||
attn = (q.matmul(k.permute(0, 1, 3, 2)))
|
||||
if self.mixer == 'Local':
|
||||
attn += self.mask
|
||||
attn = nn.functional.softmax(attn, dim=-1)
|
||||
attn = self.attn_drop(attn)
|
||||
|
||||
x = (attn.matmul(v)).permute(0, 2, 1, 3).reshape((-1, N, C))
|
||||
x = self.proj(x)
|
||||
x = self.proj_drop(x)
|
||||
return x
|
||||
|
||||
|
||||
class Block(nn.Module):
|
||||
|
||||
def __init__(self,
|
||||
dim,
|
||||
num_heads,
|
||||
mixer='Global',
|
||||
local_mixer=[7, 11],
|
||||
HW=[8, 25],
|
||||
mlp_ratio=4.,
|
||||
qkv_bias=False,
|
||||
qk_scale=None,
|
||||
drop=0.,
|
||||
attn_drop=0.,
|
||||
drop_path=0.,
|
||||
act_layer='gelu',
|
||||
norm_layer='nn.LayerNorm',
|
||||
epsilon=1e-6,
|
||||
prenorm=True):
|
||||
super().__init__()
|
||||
if isinstance(norm_layer, str):
|
||||
self.norm1 = eval(norm_layer)(dim, eps=epsilon)
|
||||
else:
|
||||
self.norm1 = norm_layer(dim)
|
||||
if mixer == 'Global' or mixer == 'Local':
|
||||
self.mixer = Attention(
|
||||
dim,
|
||||
num_heads=num_heads,
|
||||
mixer=mixer,
|
||||
HW=HW,
|
||||
local_k=local_mixer,
|
||||
qkv_bias=qkv_bias,
|
||||
qk_scale=qk_scale,
|
||||
attn_drop=attn_drop,
|
||||
proj_drop=drop)
|
||||
elif mixer == 'Conv':
|
||||
self.mixer = ConvMixer(
|
||||
dim, num_heads=num_heads, HW=HW, local_k=local_mixer)
|
||||
else:
|
||||
raise TypeError('The mixer must be one of [Global, Local, Conv]')
|
||||
|
||||
self.drop_path = DropPath(drop_path) if drop_path > 0. else Identity()
|
||||
if isinstance(norm_layer, str):
|
||||
self.norm2 = eval(norm_layer)(dim, eps=epsilon)
|
||||
else:
|
||||
self.norm2 = norm_layer(dim)
|
||||
mlp_hidden_dim = int(dim * mlp_ratio)
|
||||
self.mlp_ratio = mlp_ratio
|
||||
self.mlp = Mlp(
|
||||
in_features=dim,
|
||||
hidden_features=mlp_hidden_dim,
|
||||
act_layer=act_layer,
|
||||
drop=drop)
|
||||
self.prenorm = prenorm
|
||||
|
||||
def forward(self, x):
|
||||
if self.prenorm:
|
||||
x = self.norm1(x + self.drop_path(self.mixer(x)))
|
||||
x = self.norm2(x + self.drop_path(self.mlp(x)))
|
||||
else:
|
||||
x = x + self.drop_path(self.mixer(self.norm1(x)))
|
||||
x = x + self.drop_path(self.mlp(self.norm2(x)))
|
||||
return x
|
||||
|
||||
|
||||
class PatchEmbed(nn.Module):
|
||||
""" Image to Patch Embedding
|
||||
"""
|
||||
|
||||
def __init__(self,
|
||||
img_size=[32, 100],
|
||||
in_channels=3,
|
||||
embed_dim=768,
|
||||
sub_num=2):
|
||||
super().__init__()
|
||||
num_patches = (img_size[1] // (2 ** sub_num)) * \
|
||||
(img_size[0] // (2 ** sub_num))
|
||||
self.img_size = img_size
|
||||
self.num_patches = num_patches
|
||||
self.embed_dim = embed_dim
|
||||
self.norm = None
|
||||
if sub_num == 2:
|
||||
self.proj = nn.Sequential(
|
||||
ConvBNLayer(
|
||||
in_channels=in_channels,
|
||||
out_channels=embed_dim // 2,
|
||||
kernel_size=3,
|
||||
stride=2,
|
||||
padding=1,
|
||||
act='gelu',
|
||||
bias_attr=True),
|
||||
ConvBNLayer(
|
||||
in_channels=embed_dim // 2,
|
||||
out_channels=embed_dim,
|
||||
kernel_size=3,
|
||||
stride=2,
|
||||
padding=1,
|
||||
act='gelu',
|
||||
bias_attr=True))
|
||||
if sub_num == 3:
|
||||
self.proj = nn.Sequential(
|
||||
ConvBNLayer(
|
||||
in_channels=in_channels,
|
||||
out_channels=embed_dim // 4,
|
||||
kernel_size=3,
|
||||
stride=2,
|
||||
padding=1,
|
||||
act='gelu',
|
||||
bias_attr=True),
|
||||
ConvBNLayer(
|
||||
in_channels=embed_dim // 4,
|
||||
out_channels=embed_dim // 2,
|
||||
kernel_size=3,
|
||||
stride=2,
|
||||
padding=1,
|
||||
act='gelu',
|
||||
bias_attr=True),
|
||||
ConvBNLayer(
|
||||
in_channels=embed_dim // 2,
|
||||
out_channels=embed_dim,
|
||||
kernel_size=3,
|
||||
stride=2,
|
||||
padding=1,
|
||||
act='gelu',
|
||||
bias_attr=True))
|
||||
|
||||
def forward(self, x):
|
||||
B, C, H, W = x.shape
|
||||
assert H == self.img_size[0] and W == self.img_size[1], \
|
||||
"Input image size ({}*{}) doesn't match model ({}*{}).".format(
|
||||
H, W, self.img_size[0], self.img_size[1]
|
||||
)
|
||||
x = self.proj(x).flatten(2).permute(0, 2, 1)
|
||||
return x
|
||||
|
||||
|
||||
class SubSample(nn.Module):
|
||||
|
||||
def __init__(self,
|
||||
in_channels,
|
||||
out_channels,
|
||||
types='Pool',
|
||||
stride=[2, 1],
|
||||
sub_norm='nn.LayerNorm',
|
||||
act=None):
|
||||
super().__init__()
|
||||
self.types = types
|
||||
if types == 'Pool':
|
||||
self.avgpool = nn.AvgPool2d(
|
||||
kernel_size=[3, 5], stride=stride, padding=[1, 2])
|
||||
self.maxpool = nn.MaxPool2d(
|
||||
kernel_size=[3, 5], stride=stride, padding=[1, 2])
|
||||
self.proj = nn.Linear(in_channels, out_channels)
|
||||
else:
|
||||
self.conv = nn.Conv2d(
|
||||
in_channels,
|
||||
out_channels,
|
||||
kernel_size=3,
|
||||
stride=stride,
|
||||
padding=1,
|
||||
)
|
||||
self.norm = eval(sub_norm)(out_channels)
|
||||
if act is not None:
|
||||
self.act = act()
|
||||
else:
|
||||
self.act = None
|
||||
|
||||
def forward(self, x):
|
||||
|
||||
if self.types == 'Pool':
|
||||
x1 = self.avgpool(x)
|
||||
x2 = self.maxpool(x)
|
||||
x = (x1 + x2) * 0.5
|
||||
out = self.proj(x.flatten(2).permute(0, 2, 1))
|
||||
else:
|
||||
x = self.conv(x)
|
||||
out = x.flatten(2).permute(0, 2, 1)
|
||||
out = self.norm(out)
|
||||
if self.act is not None:
|
||||
out = self.act(out)
|
||||
|
||||
return out
|
||||
|
||||
|
||||
@BACKBONES.register_module()
|
||||
class SVTRNet(nn.Module):
|
||||
|
||||
def __init__(
|
||||
self,
|
||||
img_size=[32, 100],
|
||||
in_channels=3,
|
||||
embed_dim=[64, 128, 256],
|
||||
depth=[3, 6, 3],
|
||||
num_heads=[2, 4, 8],
|
||||
mixer=['Local'] * 6 +
|
||||
['Global'] * 6, # Local atten, Global atten, Conv
|
||||
local_mixer=[[7, 11], [7, 11], [7, 11]],
|
||||
patch_merging='Conv', # Conv, Pool, None
|
||||
mlp_ratio=4,
|
||||
qkv_bias=True,
|
||||
qk_scale=None,
|
||||
drop_rate=0.,
|
||||
last_drop=0.0,
|
||||
attn_drop_rate=0.,
|
||||
drop_path_rate=0.1,
|
||||
norm_layer='nn.LayerNorm',
|
||||
sub_norm='nn.LayerNorm',
|
||||
epsilon=1e-6,
|
||||
out_channels=192,
|
||||
out_char_num=25,
|
||||
block_unit='Block',
|
||||
act='nn.GELU',
|
||||
last_stage=True,
|
||||
sub_num=2,
|
||||
prenorm=True,
|
||||
use_lenhead=False,
|
||||
**kwargs):
|
||||
super().__init__()
|
||||
self.img_size = img_size
|
||||
self.embed_dim = embed_dim
|
||||
self.out_channels = out_channels
|
||||
self.prenorm = prenorm
|
||||
patch_merging = None if patch_merging != 'Conv' and patch_merging != 'Pool' else patch_merging
|
||||
self.patch_embed = PatchEmbed(
|
||||
img_size=img_size,
|
||||
in_channels=in_channels,
|
||||
embed_dim=embed_dim[0],
|
||||
sub_num=sub_num)
|
||||
num_patches = self.patch_embed.num_patches
|
||||
self.HW = [img_size[0] // (2**sub_num), img_size[1] // (2**sub_num)]
|
||||
self.pos_embed = nn.Parameter(
|
||||
torch.zeros(1, num_patches, embed_dim[0]))
|
||||
self.pos_drop = nn.Dropout(p=drop_rate)
|
||||
Block_unit = eval(block_unit)
|
||||
|
||||
dpr = np.linspace(0, drop_path_rate, sum(depth))
|
||||
self.blocks1 = nn.ModuleList([
|
||||
Block_unit(
|
||||
dim=embed_dim[0],
|
||||
num_heads=num_heads[0],
|
||||
mixer=mixer[0:depth[0]][i],
|
||||
HW=self.HW,
|
||||
local_mixer=local_mixer[0],
|
||||
mlp_ratio=mlp_ratio,
|
||||
qkv_bias=qkv_bias,
|
||||
qk_scale=qk_scale,
|
||||
drop=drop_rate,
|
||||
act_layer=eval(act),
|
||||
attn_drop=attn_drop_rate,
|
||||
drop_path=dpr[0:depth[0]][i],
|
||||
norm_layer=norm_layer,
|
||||
epsilon=epsilon,
|
||||
prenorm=prenorm) for i in range(depth[0])
|
||||
])
|
||||
if patch_merging is not None:
|
||||
self.sub_sample1 = SubSample(
|
||||
embed_dim[0],
|
||||
embed_dim[1],
|
||||
sub_norm=sub_norm,
|
||||
stride=[2, 1],
|
||||
types=patch_merging)
|
||||
HW = [self.HW[0] // 2, self.HW[1]]
|
||||
else:
|
||||
HW = self.HW
|
||||
self.patch_merging = patch_merging
|
||||
self.blocks2 = nn.ModuleList([
|
||||
Block_unit(
|
||||
dim=embed_dim[1],
|
||||
num_heads=num_heads[1],
|
||||
mixer=mixer[depth[0]:depth[0] + depth[1]][i],
|
||||
HW=HW,
|
||||
local_mixer=local_mixer[1],
|
||||
mlp_ratio=mlp_ratio,
|
||||
qkv_bias=qkv_bias,
|
||||
qk_scale=qk_scale,
|
||||
drop=drop_rate,
|
||||
act_layer=eval(act),
|
||||
attn_drop=attn_drop_rate,
|
||||
drop_path=dpr[depth[0]:depth[0] + depth[1]][i],
|
||||
norm_layer=norm_layer,
|
||||
epsilon=epsilon,
|
||||
prenorm=prenorm) for i in range(depth[1])
|
||||
])
|
||||
if patch_merging is not None:
|
||||
self.sub_sample2 = SubSample(
|
||||
embed_dim[1],
|
||||
embed_dim[2],
|
||||
sub_norm=sub_norm,
|
||||
stride=[2, 1],
|
||||
types=patch_merging)
|
||||
HW = [self.HW[0] // 4, self.HW[1]]
|
||||
else:
|
||||
HW = self.HW
|
||||
self.blocks3 = nn.ModuleList([
|
||||
Block_unit(
|
||||
dim=embed_dim[2],
|
||||
num_heads=num_heads[2],
|
||||
mixer=mixer[depth[0] + depth[1]:][i],
|
||||
HW=HW,
|
||||
local_mixer=local_mixer[2],
|
||||
mlp_ratio=mlp_ratio,
|
||||
qkv_bias=qkv_bias,
|
||||
qk_scale=qk_scale,
|
||||
drop=drop_rate,
|
||||
act_layer=eval(act),
|
||||
attn_drop=attn_drop_rate,
|
||||
drop_path=dpr[depth[0] + depth[1]:][i],
|
||||
norm_layer=norm_layer,
|
||||
epsilon=epsilon,
|
||||
prenorm=prenorm) for i in range(depth[2])
|
||||
])
|
||||
self.last_stage = last_stage
|
||||
if last_stage:
|
||||
self.avg_pool = nn.AdaptiveAvgPool2d([1, out_char_num])
|
||||
self.last_conv = nn.Conv2d(
|
||||
in_channels=embed_dim[2],
|
||||
out_channels=self.out_channels,
|
||||
kernel_size=1,
|
||||
stride=1,
|
||||
padding=0,
|
||||
bias=False)
|
||||
self.hardswish = Activation('hard_swish', inplace=True)
|
||||
# self.dropout = nn.Dropout(p=last_drop, mode="downscale_in_infer")
|
||||
self.dropout = nn.Dropout(p=last_drop)
|
||||
if not prenorm:
|
||||
self.norm = eval(norm_layer)(embed_dim[-1], eps=epsilon)
|
||||
self.use_lenhead = use_lenhead
|
||||
if use_lenhead:
|
||||
self.len_conv = nn.Linear(embed_dim[2], self.out_channels)
|
||||
self.hardswish_len = Activation(
|
||||
'hard_swish', inplace=True) # nn.Hardswish()
|
||||
self.dropout_len = nn.Dropout(p=last_drop)
|
||||
|
||||
torch.nn.init.xavier_normal_(self.pos_embed)
|
||||
self.apply(self._init_weights)
|
||||
|
||||
def _init_weights(self, m):
|
||||
# weight initialization
|
||||
if isinstance(m, nn.Conv2d):
|
||||
nn.init.kaiming_normal_(m.weight, mode='fan_out')
|
||||
if m.bias is not None:
|
||||
nn.init.zeros_(m.bias)
|
||||
elif isinstance(m, nn.BatchNorm2d):
|
||||
nn.init.ones_(m.weight)
|
||||
nn.init.zeros_(m.bias)
|
||||
elif isinstance(m, nn.Linear):
|
||||
nn.init.normal_(m.weight, 0, 0.01)
|
||||
if m.bias is not None:
|
||||
nn.init.zeros_(m.bias)
|
||||
elif isinstance(m, nn.ConvTranspose2d):
|
||||
nn.init.kaiming_normal_(m.weight, mode='fan_out')
|
||||
if m.bias is not None:
|
||||
nn.init.zeros_(m.bias)
|
||||
elif isinstance(m, nn.LayerNorm):
|
||||
nn.init.ones_(m.weight)
|
||||
nn.init.zeros_(m.bias)
|
||||
|
||||
def forward_features(self, x):
|
||||
x = self.patch_embed(x)
|
||||
x = x + self.pos_embed
|
||||
x = self.pos_drop(x)
|
||||
for blk in self.blocks1:
|
||||
x = blk(x)
|
||||
if self.patch_merging is not None:
|
||||
x = self.sub_sample1(
|
||||
x.permute(0, 2, 1).reshape(
|
||||
[-1, self.embed_dim[0], self.HW[0], self.HW[1]]))
|
||||
for blk in self.blocks2:
|
||||
x = blk(x)
|
||||
if self.patch_merging is not None:
|
||||
x = self.sub_sample2(
|
||||
x.permute(0, 2, 1).reshape(
|
||||
[-1, self.embed_dim[1], self.HW[0] // 2, self.HW[1]]))
|
||||
for blk in self.blocks3:
|
||||
x = blk(x)
|
||||
if not self.prenorm:
|
||||
x = self.norm(x)
|
||||
return x
|
||||
|
||||
def forward(self, x):
|
||||
x = self.forward_features(x)
|
||||
if self.use_lenhead:
|
||||
len_x = self.len_conv(x.mean(1))
|
||||
len_x = self.dropout_len(self.hardswish_len(len_x))
|
||||
if self.last_stage:
|
||||
if self.patch_merging is not None:
|
||||
h = self.HW[0] // 4
|
||||
else:
|
||||
h = self.HW[0]
|
||||
x = self.avg_pool(
|
||||
x.permute(0, 2,
|
||||
1).reshape([-1, self.embed_dim[2], h, self.HW[1]]))
|
||||
x = self.last_conv(x)
|
||||
x = self.hardswish(x)
|
||||
x = self.dropout(x)
|
||||
if self.use_lenhead:
|
||||
return x, len_x
|
||||
return x
|
|
@ -0,0 +1,2 @@
|
|||
# Copyright (c) Alibaba, Inc. and its affiliates.
|
||||
from .text_classifier import TextClassifier
|
|
@ -0,0 +1,86 @@
|
|||
# Copyright (c) Alibaba, Inc. and its affiliates.
|
||||
import numpy as np
|
||||
import torch
|
||||
import torch.nn as nn
|
||||
import torch.nn.functional as F
|
||||
|
||||
from easycv.models import builder
|
||||
from easycv.models.base import BaseModel
|
||||
from easycv.models.builder import MODELS
|
||||
from easycv.utils.checkpoint import load_checkpoint
|
||||
from easycv.utils.logger import get_root_logger
|
||||
|
||||
|
||||
@MODELS.register_module()
|
||||
class TextClassifier(BaseModel):
|
||||
"""for text classification
|
||||
"""
|
||||
|
||||
def __init__(
|
||||
self,
|
||||
backbone,
|
||||
head,
|
||||
neck=None,
|
||||
loss=None,
|
||||
pretrained=None,
|
||||
**kwargs,
|
||||
):
|
||||
super(TextClassifier, self).__init__()
|
||||
|
||||
self.pretrained = pretrained
|
||||
|
||||
self.backbone = builder.build_backbone(backbone)
|
||||
self.neck = builder.build_neck(neck) if neck else None
|
||||
self.head = builder.build_head(head)
|
||||
self.loss = nn.CrossEntropyLoss()
|
||||
self.init_weights()
|
||||
|
||||
def init_weights(self):
|
||||
logger = get_root_logger()
|
||||
if self.pretrained:
|
||||
load_checkpoint(self, self.pretrained, strict=False, logger=logger)
|
||||
else:
|
||||
# weight initialization
|
||||
for m in self.modules():
|
||||
if isinstance(m, nn.Conv2d) or isinstance(
|
||||
m, nn.ConvTranspose2d):
|
||||
nn.init.kaiming_normal_(m.weight, mode='fan_out')
|
||||
if m.bias is not None:
|
||||
nn.init.zeros_(m.bias)
|
||||
elif isinstance(m, nn.BatchNorm2d):
|
||||
nn.init.ones_(m.weight)
|
||||
if m.bias is not None:
|
||||
nn.init.zeros_(m.bias)
|
||||
elif isinstance(m, nn.Linear):
|
||||
nn.init.normal_(m.weight, 0, 0.01)
|
||||
if m.bias is not None:
|
||||
nn.init.zeros_(m.bias)
|
||||
|
||||
def extract_feat(self, x):
|
||||
y = dict()
|
||||
x = self.backbone(x)
|
||||
y['backbone_out'] = x
|
||||
if self.neck:
|
||||
x = self.neck(x)
|
||||
y['neck_out'] = x
|
||||
# convert to list in order to fit easycv cls head
|
||||
x = self.head([x])[0]
|
||||
x = F.softmax(x, dim=1)
|
||||
y['head_out'] = x
|
||||
return y
|
||||
|
||||
def forward_train(self, img, label, **kwargs):
|
||||
out = {}
|
||||
preds = self.extract_feat(img)
|
||||
out['loss'] = self.loss(preds['head_out'], label)
|
||||
return out
|
||||
|
||||
def forward_test(self, img, **kwargs):
|
||||
label = kwargs.get('label', None)
|
||||
result = {}
|
||||
preds = self.extract_feat(img)
|
||||
if label != None:
|
||||
result['label'] = label.cpu()
|
||||
result['neck'] = preds['head_out'].cpu()
|
||||
result['class'] = torch.argmax(preds['head_out'], dim=1).cpu()
|
||||
return result
|
|
@ -0,0 +1,2 @@
|
|||
# Copyright (c) Alibaba, Inc. and its affiliates.
|
||||
from .db_net import DBNet
|
|
@ -0,0 +1,145 @@
|
|||
# Copyright (c) Alibaba, Inc. and its affiliates.
|
||||
import numpy as np
|
||||
import torch
|
||||
import torch.nn as nn
|
||||
import torch.nn.functional as F
|
||||
|
||||
from easycv.models import builder
|
||||
from easycv.models.base import BaseModel
|
||||
from easycv.models.builder import MODELS
|
||||
from easycv.models.ocr.postprocess.db_postprocess import DBPostProcess
|
||||
from easycv.utils.checkpoint import load_checkpoint
|
||||
from easycv.utils.logger import get_root_logger
|
||||
|
||||
|
||||
@MODELS.register_module()
|
||||
class DBNet(BaseModel):
|
||||
"""DBNet for text detection
|
||||
"""
|
||||
|
||||
def __init__(
|
||||
self,
|
||||
backbone,
|
||||
neck,
|
||||
head,
|
||||
postprocess,
|
||||
loss=None,
|
||||
pretrained=None,
|
||||
**kwargs,
|
||||
):
|
||||
super(DBNet, self).__init__()
|
||||
|
||||
self.pretrained = pretrained
|
||||
|
||||
self.backbone = builder.build_backbone(backbone)
|
||||
self.neck = builder.build_neck(neck)
|
||||
self.head = builder.build_head(head)
|
||||
self.loss = builder.build_loss(loss) if loss else None
|
||||
self.postprocess_op = DBPostProcess(**postprocess)
|
||||
self.init_weights()
|
||||
|
||||
def init_weights(self):
|
||||
logger = get_root_logger()
|
||||
if self.pretrained:
|
||||
load_checkpoint(self, self.pretrained, strict=False, logger=logger)
|
||||
else:
|
||||
# weight initialization
|
||||
for m in self.modules():
|
||||
if isinstance(m, nn.Conv2d) or isinstance(
|
||||
m, nn.ConvTranspose2d):
|
||||
nn.init.kaiming_normal_(m.weight, mode='fan_out')
|
||||
if m.bias is not None:
|
||||
nn.init.zeros_(m.bias)
|
||||
elif isinstance(m, nn.BatchNorm2d):
|
||||
nn.init.ones_(m.weight)
|
||||
if m.bias is not None:
|
||||
nn.init.zeros_(m.bias)
|
||||
elif isinstance(m, nn.Linear):
|
||||
nn.init.normal_(m.weight, 0, 0.01)
|
||||
if m.bias is not None:
|
||||
nn.init.zeros_(m.bias)
|
||||
|
||||
def extract_feat(self, x):
|
||||
x = self.backbone(x)
|
||||
# y["backbone_out"] = x
|
||||
x = self.neck(x)
|
||||
# y["neck_out"] = x
|
||||
x = self.head(x)
|
||||
return x
|
||||
|
||||
def forward_train(self, img, **kwargs):
|
||||
predicts = self.extract_feat(img)
|
||||
loss = self.loss(predicts, kwargs)
|
||||
return loss
|
||||
|
||||
def forward_test(self, img, **kwargs):
|
||||
shape_list = [
|
||||
img_meta['ori_img_shape'] for img_meta in kwargs['img_metas']
|
||||
]
|
||||
with torch.no_grad():
|
||||
preds = self.extract_feat(img)
|
||||
post_results = self.postprocess_op(preds, shape_list)
|
||||
if 'ignore_tags' in kwargs['img_metas'][0]:
|
||||
ignore_tags = [
|
||||
img_meta['ignore_tags'] for img_meta in kwargs['img_metas']
|
||||
]
|
||||
post_results['ignore_tags'] = ignore_tags
|
||||
if 'polys' in kwargs['img_metas'][0]:
|
||||
polys = [img_meta['polys'] for img_meta in kwargs['img_metas']]
|
||||
post_results['polys'] = polys
|
||||
return post_results
|
||||
|
||||
def postprocess(self, preds, shape_list):
|
||||
|
||||
post_results = self.postprocess_op(preds, shape_list)
|
||||
points_results = post_results['points']
|
||||
dt_boxes = []
|
||||
for idx in range(len(points_results)):
|
||||
dt_box = points_results[idx]
|
||||
dt_box = self.filter_tag_det_res(dt_box, shape_list[idx])
|
||||
dt_boxes.append(dt_box)
|
||||
return dt_boxes
|
||||
|
||||
def filter_tag_det_res(self, dt_boxes, image_shape):
|
||||
img_height, img_width = image_shape[0:2]
|
||||
dt_boxes_new = []
|
||||
for box in dt_boxes:
|
||||
box = self.order_points_clockwise(box)
|
||||
box = self.clip_det_res(box, img_height, img_width)
|
||||
rect_width = int(np.linalg.norm(box[0] - box[1]))
|
||||
rect_height = int(np.linalg.norm(box[0] - box[3]))
|
||||
if rect_width <= 3 or rect_height <= 3:
|
||||
continue
|
||||
dt_boxes_new.append(box)
|
||||
dt_boxes = np.array(dt_boxes_new)
|
||||
return dt_boxes
|
||||
|
||||
def order_points_clockwise(self, pts):
|
||||
"""
|
||||
reference from: https://github.com/jrosebr1/imutils/blob/master/imutils/perspective.py
|
||||
# sort the points based on their x-coordinates
|
||||
"""
|
||||
xSorted = pts[np.argsort(pts[:, 0]), :]
|
||||
|
||||
# grab the left-most and right-most points from the sorted
|
||||
# x-roodinate points
|
||||
leftMost = xSorted[:2, :]
|
||||
rightMost = xSorted[2:, :]
|
||||
|
||||
# now, sort the left-most coordinates according to their
|
||||
# y-coordinates so we can grab the top-left and bottom-left
|
||||
# points, respectively
|
||||
leftMost = leftMost[np.argsort(leftMost[:, 1]), :]
|
||||
(tl, bl) = leftMost
|
||||
|
||||
rightMost = rightMost[np.argsort(rightMost[:, 1]), :]
|
||||
(tr, br) = rightMost
|
||||
|
||||
rect = np.array([tl, tr, br, bl], dtype='float32')
|
||||
return rect
|
||||
|
||||
def clip_det_res(self, points, img_height, img_width):
|
||||
for pno in range(points.shape[0]):
|
||||
points[pno, 0] = int(min(max(points[pno, 0], 0), img_width - 1))
|
||||
points[pno, 1] = int(min(max(points[pno, 1], 0), img_height - 1))
|
||||
return points
|
|
@ -0,0 +1,3 @@
|
|||
# Copyright (c) Alibaba, Inc. and its affiliates.
|
||||
from .db_head import DBHead
|
||||
from .rec_head import CTCHead
|
|
@ -0,0 +1,82 @@
|
|||
# Modified from https://github.com/PaddlePaddle/PaddleOCR/blob/release/2.6/ppocr/modeling/heads/det_db_head.py
|
||||
import torch
|
||||
import torch.nn as nn
|
||||
import torch.nn.functional as F
|
||||
|
||||
from easycv.models.builder import HEADS
|
||||
|
||||
|
||||
class DBBaseHead(nn.Module):
|
||||
|
||||
def __init__(self, in_channels, kernel_list=[3, 2, 2], **kwargs):
|
||||
super(DBBaseHead, self).__init__()
|
||||
self.conv1 = nn.Conv2d(
|
||||
in_channels=in_channels,
|
||||
out_channels=in_channels // 4,
|
||||
kernel_size=kernel_list[0],
|
||||
padding=int(kernel_list[0] // 2),
|
||||
bias=False)
|
||||
self.conv_bn1 = nn.BatchNorm2d(in_channels // 4)
|
||||
self.relu1 = nn.ReLU(inplace=True)
|
||||
|
||||
self.conv2 = nn.ConvTranspose2d(
|
||||
in_channels=in_channels // 4,
|
||||
out_channels=in_channels // 4,
|
||||
kernel_size=kernel_list[1],
|
||||
stride=2)
|
||||
self.conv_bn2 = nn.BatchNorm2d(in_channels // 4)
|
||||
self.relu2 = nn.ReLU(inplace=True)
|
||||
|
||||
self.conv3 = nn.ConvTranspose2d(
|
||||
in_channels=in_channels // 4,
|
||||
out_channels=1,
|
||||
kernel_size=kernel_list[2],
|
||||
stride=2)
|
||||
|
||||
def forward(self, x):
|
||||
x = self.conv1(x)
|
||||
x = self.conv_bn1(x)
|
||||
x = self.relu1(x)
|
||||
x = self.conv2(x)
|
||||
x = self.conv_bn2(x)
|
||||
x = self.relu2(x)
|
||||
x = self.conv3(x)
|
||||
x = torch.sigmoid(x)
|
||||
return x
|
||||
|
||||
|
||||
@HEADS.register_module()
|
||||
class DBHead(nn.Module):
|
||||
"""
|
||||
Differentiable Binarization (DB) for text detection:
|
||||
see https://arxiv.org/abs/1911.08947
|
||||
args:
|
||||
params(dict): super parameters for build DB network
|
||||
"""
|
||||
|
||||
def __init__(self, in_channels, k=50, **kwargs):
|
||||
super(DBHead, self).__init__()
|
||||
self.k = k
|
||||
binarize_name_list = [
|
||||
'conv2d_56', 'batch_norm_47', 'conv2d_transpose_0',
|
||||
'batch_norm_48', 'conv2d_transpose_1', 'binarize'
|
||||
]
|
||||
thresh_name_list = [
|
||||
'conv2d_57', 'batch_norm_49', 'conv2d_transpose_2',
|
||||
'batch_norm_50', 'conv2d_transpose_3', 'thresh'
|
||||
]
|
||||
self.binarize = DBBaseHead(in_channels, **kwargs)
|
||||
self.thresh = DBBaseHead(in_channels, **kwargs)
|
||||
|
||||
def step_function(self, x, y):
|
||||
return torch.reciprocal(1 + torch.exp(-self.k * (x - y)))
|
||||
|
||||
def forward(self, x):
|
||||
shrink_maps = self.binarize(x)
|
||||
if not self.training:
|
||||
return {'maps': shrink_maps}
|
||||
|
||||
threshold_maps = self.thresh(x)
|
||||
binary_maps = self.step_function(shrink_maps, threshold_maps)
|
||||
y = torch.cat([shrink_maps, threshold_maps, binary_maps], dim=1)
|
||||
return {'maps': y}
|
|
@ -0,0 +1,482 @@
|
|||
# Modified from https://github.com/PaddlePaddle/PaddleOCR/tree/release/2.6/ppocr/modeling/heads
|
||||
import math
|
||||
|
||||
import torch
|
||||
import torch.nn as nn
|
||||
import torch.nn.functional as F
|
||||
|
||||
from easycv.models.builder import HEADS
|
||||
from ..necks.squence_encoder import Im2Seq, SequenceEncoder
|
||||
|
||||
|
||||
class SAREncoder(nn.Module):
|
||||
"""
|
||||
Args:
|
||||
enc_bi_rnn (bool): If True, use bidirectional RNN in encoder.
|
||||
enc_drop_rnn (float): Dropout probability of RNN layer in encoder.
|
||||
enc_gru (bool): If True, use GRU, else LSTM in encoder.
|
||||
d_model (int): Dim of channels from backbone.
|
||||
d_enc (int): Dim of encoder RNN layer.
|
||||
mask (bool): If True, mask padding in RNN sequence.
|
||||
"""
|
||||
|
||||
def __init__(self,
|
||||
enc_bi_rnn=False,
|
||||
enc_drop_rnn=0.1,
|
||||
enc_gru=False,
|
||||
d_model=512,
|
||||
d_enc=512,
|
||||
mask=True,
|
||||
**kwargs):
|
||||
super().__init__()
|
||||
assert isinstance(enc_bi_rnn, bool)
|
||||
assert isinstance(enc_drop_rnn, (int, float))
|
||||
assert 0 <= enc_drop_rnn < 1.0
|
||||
assert isinstance(enc_gru, bool)
|
||||
assert isinstance(d_model, int)
|
||||
assert isinstance(d_enc, int)
|
||||
assert isinstance(mask, bool)
|
||||
|
||||
self.enc_bi_rnn = enc_bi_rnn
|
||||
self.enc_drop_rnn = enc_drop_rnn
|
||||
self.mask = mask
|
||||
|
||||
# LSTM Encoder
|
||||
kwargs = dict(
|
||||
input_size=d_model,
|
||||
hidden_size=d_enc,
|
||||
num_layers=2,
|
||||
batch_first=True,
|
||||
dropout=enc_drop_rnn,
|
||||
bidirectional=enc_bi_rnn)
|
||||
|
||||
if enc_gru:
|
||||
self.rnn_encoder = nn.GRU(**kwargs)
|
||||
else:
|
||||
self.rnn_encoder = nn.LSTM(**kwargs)
|
||||
|
||||
# global feature transformation
|
||||
encoder_rnn_out_size = d_enc * (int(enc_bi_rnn) + 1)
|
||||
self.linear = nn.Linear(encoder_rnn_out_size, encoder_rnn_out_size)
|
||||
|
||||
def forward(self, feat, valid_ratios=None):
|
||||
|
||||
h_feat = feat.shape[2] # bsz c h w
|
||||
feat_v = F.max_pool2d(
|
||||
feat, kernel_size=(h_feat, 1), stride=1, padding=0)
|
||||
feat_v = feat_v.squeeze(2) # bsz * C * W
|
||||
feat_v = feat_v.permute(0, 2, 1).contiguous() # bsz * W * C
|
||||
holistic_feat = self.rnn_encoder(feat_v)[0] # bsz * T * C
|
||||
|
||||
if valid_ratios is not None:
|
||||
valid_hf = []
|
||||
T = holistic_feat.size(1)
|
||||
for i, valid_ratio in enumerate(valid_ratios):
|
||||
valid_step = min(T, math.ceil(T * valid_ratio)) - 1
|
||||
# for i in range(valid_ratios.size(0)):
|
||||
# valid_step = torch.min(T, torch.ceil(T * valid_ratios[i])) - 1
|
||||
valid_hf.append(holistic_feat[i, valid_step, :])
|
||||
valid_hf = torch.stack(valid_hf, dim=0)
|
||||
else:
|
||||
valid_hf = holistic_feat[:, -1, :] # bsz * C
|
||||
holistic_feat = self.linear(valid_hf) # bsz * C
|
||||
|
||||
return holistic_feat
|
||||
|
||||
|
||||
class BaseDecoder(nn.Module):
|
||||
|
||||
def __init__(self, **kwargs):
|
||||
super().__init__()
|
||||
|
||||
def forward_train(self, feat, out_enc, targets, valid_ratios):
|
||||
raise NotImplementedError
|
||||
|
||||
def forward_test(self, feat, out_enc, valid_ratios):
|
||||
raise NotImplementedError
|
||||
|
||||
def forward(self,
|
||||
feat,
|
||||
out_enc,
|
||||
label=None,
|
||||
valid_ratios=None,
|
||||
train_mode=True):
|
||||
self.train_mode = train_mode
|
||||
|
||||
if train_mode:
|
||||
return self.forward_train(feat, out_enc, label, valid_ratios)
|
||||
return self.forward_test(feat, out_enc, valid_ratios)
|
||||
|
||||
|
||||
class ParallelSARDecoder(BaseDecoder):
|
||||
"""
|
||||
Args:
|
||||
out_channels (int): Output class number.
|
||||
enc_bi_rnn (bool): If True, use bidirectional RNN in encoder.
|
||||
dec_bi_rnn (bool): If True, use bidirectional RNN in decoder.
|
||||
dec_drop_rnn (float): Dropout of RNN layer in decoder.
|
||||
dec_gru (bool): If True, use GRU, else LSTM in decoder.
|
||||
d_model (int): Dim of channels from backbone.
|
||||
d_enc (int): Dim of encoder RNN layer.
|
||||
d_k (int): Dim of channels of attention module.
|
||||
pred_dropout (float): Dropout probability of prediction layer.
|
||||
max_seq_len (int): Maximum sequence length for decoding.
|
||||
mask (bool): If True, mask padding in feature map.
|
||||
start_idx (int): Index of start token.
|
||||
padding_idx (int): Index of padding token.
|
||||
pred_concat (bool): If True, concat glimpse feature from
|
||||
attention with holistic feature and hidden state.
|
||||
"""
|
||||
|
||||
def __init__(
|
||||
self,
|
||||
out_channels, # 90 + unknown + start + padding
|
||||
enc_bi_rnn=False,
|
||||
dec_bi_rnn=False,
|
||||
dec_drop_rnn=0.0,
|
||||
dec_gru=False,
|
||||
d_model=512,
|
||||
d_enc=512,
|
||||
d_k=64,
|
||||
pred_dropout=0.1,
|
||||
max_text_length=30,
|
||||
mask=True,
|
||||
pred_concat=True,
|
||||
**kwargs):
|
||||
super().__init__()
|
||||
|
||||
self.num_classes = out_channels
|
||||
self.enc_bi_rnn = enc_bi_rnn
|
||||
self.d_k = d_k
|
||||
self.start_idx = out_channels - 2
|
||||
self.padding_idx = out_channels - 1
|
||||
self.max_seq_len = max_text_length
|
||||
self.mask = mask
|
||||
self.pred_concat = pred_concat
|
||||
|
||||
encoder_rnn_out_size = d_enc * (int(enc_bi_rnn) + 1)
|
||||
decoder_rnn_out_size = encoder_rnn_out_size * (int(dec_bi_rnn) + 1)
|
||||
|
||||
# 2D attention layer
|
||||
self.conv1x1_1 = nn.Linear(decoder_rnn_out_size, d_k)
|
||||
self.conv3x3_1 = nn.Conv2d(
|
||||
d_model, d_k, kernel_size=3, stride=1, padding=1)
|
||||
self.conv1x1_2 = nn.Linear(d_k, 1)
|
||||
|
||||
# Decoder RNN layer
|
||||
|
||||
kwargs = dict(
|
||||
input_size=encoder_rnn_out_size,
|
||||
hidden_size=encoder_rnn_out_size,
|
||||
num_layers=2,
|
||||
batch_first=True,
|
||||
dropout=dec_drop_rnn,
|
||||
bidirectional=dec_bi_rnn)
|
||||
if dec_gru:
|
||||
self.rnn_decoder = nn.GRU(**kwargs)
|
||||
else:
|
||||
self.rnn_decoder = nn.LSTM(**kwargs)
|
||||
|
||||
# Decoder input embedding
|
||||
self.embedding = nn.Embedding(
|
||||
self.num_classes,
|
||||
encoder_rnn_out_size,
|
||||
padding_idx=self.padding_idx)
|
||||
|
||||
# Prediction layer
|
||||
self.pred_dropout = nn.Dropout(pred_dropout)
|
||||
pred_num_classes = self.num_classes - 1
|
||||
if pred_concat:
|
||||
fc_in_channel = decoder_rnn_out_size + d_model + encoder_rnn_out_size
|
||||
else:
|
||||
fc_in_channel = d_model
|
||||
self.prediction = nn.Linear(fc_in_channel, pred_num_classes)
|
||||
|
||||
def _2d_attention(self,
|
||||
decoder_input,
|
||||
feat,
|
||||
holistic_feat,
|
||||
valid_ratios=None):
|
||||
|
||||
y = self.rnn_decoder(decoder_input)[0]
|
||||
# y: bsz * (seq_len + 1) * hidden_size
|
||||
|
||||
attn_query = self.conv1x1_1(y) # bsz * (seq_len + 1) * attn_size
|
||||
bsz, seq_len, attn_size = attn_query.shape
|
||||
attn_query = attn_query.view(bsz, seq_len, attn_size, 1, 1)
|
||||
# (bsz, seq_len + 1, attn_size, 1, 1)
|
||||
|
||||
attn_key = self.conv3x3_1(feat)
|
||||
# bsz * attn_size * h * w
|
||||
attn_key = attn_key.unsqueeze(1)
|
||||
# bsz * 1 * attn_size * h * w
|
||||
|
||||
attn_weight = torch.tanh(torch.add(attn_key, attn_query, alpha=1))
|
||||
|
||||
# bsz * (seq_len + 1) * attn_size * h * w
|
||||
attn_weight = attn_weight.permute(0, 1, 3, 4, 2).contiguous()
|
||||
# bsz * (seq_len + 1) * h * w * attn_size
|
||||
attn_weight = self.conv1x1_2(attn_weight)
|
||||
# bsz * (seq_len + 1) * h * w * 1
|
||||
bsz, T, h, w, c = attn_weight.size()
|
||||
assert c == 1
|
||||
|
||||
if valid_ratios is not None:
|
||||
# cal mask of attention weight
|
||||
attn_mask = torch.zeros_like(attn_weight)
|
||||
for i, valid_ratio in enumerate(valid_ratios):
|
||||
valid_width = min(w, math.ceil(w * valid_ratio))
|
||||
attn_mask[i, :, :, valid_width:, :] = 1
|
||||
attn_weight = attn_weight.masked_fill(attn_mask.bool(),
|
||||
float('-inf'))
|
||||
# if valid_ratios is not None:
|
||||
# # cal mask of attention weight
|
||||
# for i in range(valid_ratios.size(0)):
|
||||
# valid_width = torch.min(w, torch.ceil(w * valid_ratios[i]))
|
||||
# # valid_width = paddle.minimum(
|
||||
# # w, paddle.ceil(valid_ratios[i] * w).astype("int32"))
|
||||
# if valid_width < w:
|
||||
# attn_weight[i, :, :, valid_width:, :] = float('-inf')
|
||||
|
||||
attn_weight = attn_weight.view(bsz, T, -1)
|
||||
attn_weight = F.softmax(attn_weight, dim=-1)
|
||||
|
||||
attn_weight = attn_weight.view(bsz, T, h, w,
|
||||
c).permute(0, 1, 4, 2, 3).contiguous()
|
||||
# attn_weight: bsz * T * c * h * w
|
||||
# feat: bsz * c * h * w
|
||||
attn_feat = torch.sum(
|
||||
torch.mul(feat.unsqueeze(1), attn_weight), (3, 4), keepdim=False)
|
||||
# bsz * (seq_len + 1) * C
|
||||
|
||||
# Linear transformation
|
||||
if self.pred_concat:
|
||||
hf_c = holistic_feat.size(-1)
|
||||
holistic_feat = holistic_feat.expand(bsz, seq_len, hf_c)
|
||||
y = self.prediction(torch.cat((y, attn_feat, holistic_feat), 2))
|
||||
else:
|
||||
y = self.prediction(attn_feat)
|
||||
# bsz * (seq_len + 1) * num_classes
|
||||
if self.train_mode:
|
||||
y = self.pred_dropout(y)
|
||||
|
||||
return y
|
||||
|
||||
def forward_train(self, feat, out_enc, label, valid_ratios=None):
|
||||
|
||||
lab_embedding = self.embedding(label)
|
||||
# bsz * seq_len * emb_dim
|
||||
out_enc = out_enc.unsqueeze(1)
|
||||
# bsz * 1 * emb_dim
|
||||
in_dec = torch.cat((out_enc, lab_embedding), dim=1)
|
||||
# bsz * (seq_len + 1) * C
|
||||
out_dec = self._2d_attention(
|
||||
in_dec, feat, out_enc, valid_ratios=valid_ratios)
|
||||
|
||||
return out_dec[:, 1:, :] # bsz * seq_len * num_classes
|
||||
|
||||
def forward_test(self, feat, out_enc, valid_ratios=None):
|
||||
|
||||
seq_len = self.max_seq_len
|
||||
bsz = feat.shape[0]
|
||||
start_token = torch.full((bsz, ),
|
||||
self.start_idx,
|
||||
device=feat.device,
|
||||
dtype=torch.long)
|
||||
# bsz
|
||||
start_token = self.embedding(start_token)
|
||||
# bsz * emb_dim
|
||||
emb_dim = start_token.shape[1]
|
||||
start_token = start_token.unsqueeze(1)
|
||||
start_token = start_token.unsqueeze(1).expand(-1, seq_len, -1)
|
||||
# bsz * seq_len * emb_dim
|
||||
out_enc = out_enc.unsqueeze(1)
|
||||
# bsz * 1 * emb_dim
|
||||
decoder_input = torch.cat((out_enc, start_token), dim=1)
|
||||
# bsz * (seq_len + 1) * emb_dim
|
||||
|
||||
outputs = []
|
||||
for i in range(1, seq_len + 1):
|
||||
decoder_output = self._2d_attention(
|
||||
decoder_input, feat, out_enc, valid_ratios=valid_ratios)
|
||||
char_output = decoder_output[:, i, :] # bsz * num_classes
|
||||
char_output = F.softmax(char_output, -1)
|
||||
outputs.append(char_output)
|
||||
_, max_idx = torch.max(char_output, dim=1, keepdim=False)
|
||||
char_embedding = self.embedding(max_idx) # bsz * emb_dim
|
||||
if i < seq_len:
|
||||
decoder_input[:, i + 1, :] = char_embedding
|
||||
|
||||
outputs = torch.stack(outputs, 1) # bsz * seq_len * num_classes
|
||||
|
||||
return outputs
|
||||
|
||||
|
||||
@HEADS.register_module()
|
||||
class SARHead(nn.Module):
|
||||
|
||||
def __init__(self,
|
||||
in_channels,
|
||||
out_channels,
|
||||
enc_dim=512,
|
||||
max_text_length=30,
|
||||
enc_bi_rnn=False,
|
||||
enc_drop_rnn=0.1,
|
||||
enc_gru=False,
|
||||
dec_bi_rnn=False,
|
||||
dec_drop_rnn=0.0,
|
||||
dec_gru=False,
|
||||
d_k=512,
|
||||
pred_dropout=0.1,
|
||||
pred_concat=True,
|
||||
**kwargs):
|
||||
super(SARHead, self).__init__()
|
||||
|
||||
# encoder module
|
||||
self.encoder = SAREncoder(
|
||||
enc_bi_rnn=enc_bi_rnn,
|
||||
enc_drop_rnn=enc_drop_rnn,
|
||||
enc_gru=enc_gru,
|
||||
d_model=in_channels,
|
||||
d_enc=enc_dim)
|
||||
|
||||
# decoder module
|
||||
self.decoder = ParallelSARDecoder(
|
||||
out_channels=out_channels,
|
||||
enc_bi_rnn=enc_bi_rnn,
|
||||
dec_bi_rnn=dec_bi_rnn,
|
||||
dec_drop_rnn=dec_drop_rnn,
|
||||
dec_gru=dec_gru,
|
||||
d_model=in_channels,
|
||||
d_enc=enc_dim,
|
||||
d_k=d_k,
|
||||
pred_dropout=pred_dropout,
|
||||
max_text_length=max_text_length,
|
||||
pred_concat=pred_concat)
|
||||
|
||||
def forward(self, feat, label, valid_ratios=None):
|
||||
'''
|
||||
img_metas: [label, valid_ratio]
|
||||
'''
|
||||
holistic_feat = self.encoder(feat, valid_ratios) # bsz c
|
||||
|
||||
if self.training:
|
||||
final_out = self.decoder(
|
||||
feat, holistic_feat, label, valid_ratios=valid_ratios)
|
||||
else:
|
||||
final_out = self.decoder(
|
||||
feat,
|
||||
holistic_feat,
|
||||
label=None,
|
||||
valid_ratios=valid_ratios,
|
||||
train_mode=False)
|
||||
|
||||
return final_out
|
||||
|
||||
|
||||
@HEADS.register_module()
|
||||
class CTCHead(nn.Module):
|
||||
|
||||
def __init__(self,
|
||||
in_channels,
|
||||
out_channels=6625,
|
||||
fc_decay=0.0004,
|
||||
mid_channels=None,
|
||||
return_feats=False,
|
||||
**kwargs):
|
||||
super(CTCHead, self).__init__()
|
||||
if mid_channels is None:
|
||||
self.fc = nn.Linear(
|
||||
in_channels,
|
||||
out_channels,
|
||||
bias=True,
|
||||
)
|
||||
else:
|
||||
self.fc1 = nn.Linear(
|
||||
in_channels,
|
||||
mid_channels,
|
||||
bias=True,
|
||||
)
|
||||
self.fc2 = nn.Linear(
|
||||
mid_channels,
|
||||
out_channels,
|
||||
bias=True,
|
||||
)
|
||||
|
||||
self.out_channels = out_channels
|
||||
self.mid_channels = mid_channels
|
||||
self.return_feats = return_feats
|
||||
|
||||
def forward(self, x, labels=None):
|
||||
if self.mid_channels is None:
|
||||
predicts = self.fc(x)
|
||||
else:
|
||||
x = self.fc1(x)
|
||||
predicts = self.fc2(x)
|
||||
|
||||
if self.return_feats:
|
||||
result = (x, predicts)
|
||||
else:
|
||||
result = predicts
|
||||
|
||||
if not self.training:
|
||||
predicts = F.softmax(predicts, dim=2)
|
||||
result = predicts
|
||||
|
||||
return result
|
||||
|
||||
|
||||
@HEADS.register_module()
|
||||
class MultiHead(nn.Module):
|
||||
|
||||
def __init__(self, in_channels, out_channels_list, **kwargs):
|
||||
super().__init__()
|
||||
self.head_list = kwargs.pop('head_list')
|
||||
head_name = [head.type for head in self.head_list]
|
||||
self.gtc_head = 'sar' if 'SARHead' in head_name else 'ctc'
|
||||
# assert len(self.head_list) >= 2
|
||||
for idx, head_name in enumerate(self.head_list):
|
||||
name = head_name.type
|
||||
if name == 'SARHead':
|
||||
# sar head
|
||||
sar_args = self.head_list[idx]
|
||||
self.sar_head = eval(name)(
|
||||
in_channels=in_channels,
|
||||
out_channels=out_channels_list['SARLabelDecode'],
|
||||
**sar_args)
|
||||
elif name == 'CTCHead':
|
||||
# ctc neck
|
||||
self.encoder_reshape = Im2Seq(in_channels)
|
||||
neck_args = self.head_list[idx].Neck
|
||||
# encoder_type = neck_args.pop('type')
|
||||
encoder_type = neck_args.get('type')
|
||||
self.encoder = encoder_type
|
||||
self.ctc_encoder = SequenceEncoder(
|
||||
in_channels=in_channels,
|
||||
encoder_type=encoder_type,
|
||||
**neck_args)
|
||||
# ctc head
|
||||
head_args = self.head_list[idx].Head
|
||||
self.ctc_head = eval(name)(
|
||||
in_channels=self.ctc_encoder.out_channels,
|
||||
out_channels=out_channels_list['CTCLabelDecode'],
|
||||
**head_args)
|
||||
else:
|
||||
raise NotImplementedError(
|
||||
'{} is not supported in MultiHead yet'.format(name))
|
||||
|
||||
def forward(self, x, label=None, valid_ratios=None):
|
||||
ctc_encoder = self.ctc_encoder(x)
|
||||
ctc_out = self.ctc_head(ctc_encoder)
|
||||
head_out = dict()
|
||||
head_out['ctc'] = ctc_out
|
||||
head_out['ctc_neck'] = ctc_encoder
|
||||
# eval mode
|
||||
if not self.training:
|
||||
return ctc_out
|
||||
if self.gtc_head == 'sar':
|
||||
sar_out = self.sar_head(x, label, valid_ratios)
|
||||
head_out['sar'] = sar_out
|
||||
return head_out
|
||||
else:
|
||||
return head_out
|
|
@ -0,0 +1,3 @@
|
|||
# Copyright (c) Alibaba, Inc. and its affiliates.
|
||||
from .db_fpn import DBFPN, LKPAN, RSEFPN
|
||||
from .squence_encoder import SequenceEncoder
|
|
@ -0,0 +1,348 @@
|
|||
# Modified from https://github.com/PaddlePaddle/PaddleOCR/blob/release/2.6/ppocr/modeling/necks/db_fpn.py
|
||||
from tkinter import N
|
||||
|
||||
import torch
|
||||
import torch.nn as nn
|
||||
import torch.nn.functional as F
|
||||
|
||||
from easycv.models.registry import NECKS
|
||||
from ..backbones.det_mobilenet_v3 import SEModule
|
||||
|
||||
|
||||
def hard_swish(x, inplace=True):
|
||||
return x * F.relu6(x + 3., inplace=inplace) / 6.
|
||||
|
||||
|
||||
class DSConv(nn.Module):
|
||||
|
||||
def __init__(self,
|
||||
in_channels,
|
||||
out_channels,
|
||||
kernel_size,
|
||||
padding,
|
||||
stride=1,
|
||||
groups=None,
|
||||
if_act=True,
|
||||
act='relu',
|
||||
**kwargs):
|
||||
super(DSConv, self).__init__()
|
||||
if groups == None:
|
||||
groups = in_channels
|
||||
self.if_act = if_act
|
||||
self.act = act
|
||||
self.conv1 = nn.Conv2d(
|
||||
in_channels=in_channels,
|
||||
out_channels=in_channels,
|
||||
kernel_size=kernel_size,
|
||||
stride=stride,
|
||||
padding=padding,
|
||||
groups=groups,
|
||||
bias=False)
|
||||
|
||||
self.bn1 = nn.BatchNorm2d(in_channels)
|
||||
|
||||
self.conv2 = nn.Conv2d(
|
||||
in_channels=in_channels,
|
||||
out_channels=int(in_channels * 4),
|
||||
kernel_size=1,
|
||||
stride=1,
|
||||
bias=False)
|
||||
|
||||
self.bn2 = nn.BatchNorm2d(int(in_channels * 4))
|
||||
|
||||
self.conv3 = nn.Conv2d(
|
||||
in_channels=int(in_channels * 4),
|
||||
out_channels=out_channels,
|
||||
kernel_size=1,
|
||||
stride=1,
|
||||
bias=False)
|
||||
self._c = [in_channels, out_channels]
|
||||
if in_channels != out_channels:
|
||||
self.conv_end = nn.Conv2d(
|
||||
in_channels=in_channels,
|
||||
out_channels=out_channels,
|
||||
kernel_size=1,
|
||||
stride=1,
|
||||
bias=False)
|
||||
|
||||
def forward(self, inputs):
|
||||
|
||||
x = self.conv1(inputs)
|
||||
x = self.bn1(x)
|
||||
|
||||
x = self.conv2(x)
|
||||
x = self.bn2(x)
|
||||
if self.if_act:
|
||||
if self.act == 'relu':
|
||||
x = F.relu(x)
|
||||
elif self.act == 'hardswish':
|
||||
x = hard_swish(x)
|
||||
else:
|
||||
print('The activation function({}) is selected incorrectly.'.
|
||||
format(self.act))
|
||||
exit()
|
||||
|
||||
x = self.conv3(x)
|
||||
if self._c[0] != self._c[1]:
|
||||
x = x + self.conv_end(inputs)
|
||||
return x
|
||||
|
||||
|
||||
@NECKS.register_module()
|
||||
class DBFPN(nn.Module):
|
||||
|
||||
def __init__(self, in_channels, out_channels, **kwargs):
|
||||
super(DBFPN, self).__init__()
|
||||
self.out_channels = out_channels
|
||||
|
||||
self.in2_conv = nn.Conv2d(
|
||||
in_channels=in_channels[0],
|
||||
out_channels=self.out_channels,
|
||||
kernel_size=1,
|
||||
bias=False)
|
||||
self.in3_conv = nn.Conv2d(
|
||||
in_channels=in_channels[1],
|
||||
out_channels=self.out_channels,
|
||||
kernel_size=1,
|
||||
bias=False)
|
||||
self.in4_conv = nn.Conv2d(
|
||||
in_channels=in_channels[2],
|
||||
out_channels=self.out_channels,
|
||||
kernel_size=1,
|
||||
bias=False)
|
||||
self.in5_conv = nn.Conv2d(
|
||||
in_channels=in_channels[3],
|
||||
out_channels=self.out_channels,
|
||||
kernel_size=1,
|
||||
bias=False)
|
||||
self.p5_conv = nn.Conv2d(
|
||||
in_channels=self.out_channels,
|
||||
out_channels=self.out_channels // 4,
|
||||
kernel_size=3,
|
||||
padding=1,
|
||||
bias=False)
|
||||
self.p4_conv = nn.Conv2d(
|
||||
in_channels=self.out_channels,
|
||||
out_channels=self.out_channels // 4,
|
||||
kernel_size=3,
|
||||
padding=1,
|
||||
bias=False)
|
||||
self.p3_conv = nn.Conv2d(
|
||||
in_channels=self.out_channels,
|
||||
out_channels=self.out_channels // 4,
|
||||
kernel_size=3,
|
||||
padding=1,
|
||||
bias=False)
|
||||
self.p2_conv = nn.Conv2d(
|
||||
in_channels=self.out_channels,
|
||||
out_channels=self.out_channels // 4,
|
||||
kernel_size=3,
|
||||
padding=1,
|
||||
bias=False)
|
||||
|
||||
def forward(self, x):
|
||||
c2, c3, c4, c5 = x
|
||||
|
||||
in5 = self.in5_conv(c5)
|
||||
in4 = self.in4_conv(c4)
|
||||
in3 = self.in3_conv(c3)
|
||||
in2 = self.in2_conv(c2)
|
||||
|
||||
out4 = in4 + F.interpolate(
|
||||
in5,
|
||||
scale_factor=2,
|
||||
mode='nearest',
|
||||
)
|
||||
out3 = in3 + F.interpolate(
|
||||
out4,
|
||||
scale_factor=2,
|
||||
mode='nearest',
|
||||
)
|
||||
out2 = in2 + F.interpolate(
|
||||
out3,
|
||||
scale_factor=2,
|
||||
mode='nearest',
|
||||
)
|
||||
|
||||
p5 = self.p5_conv(in5)
|
||||
p4 = self.p4_conv(out4)
|
||||
p3 = self.p3_conv(out3)
|
||||
p2 = self.p2_conv(out2)
|
||||
p5 = F.interpolate(
|
||||
p5,
|
||||
scale_factor=8,
|
||||
mode='nearest',
|
||||
)
|
||||
p4 = F.interpolate(
|
||||
p4,
|
||||
scale_factor=4,
|
||||
mode='nearest',
|
||||
)
|
||||
p3 = F.interpolate(
|
||||
p3,
|
||||
scale_factor=2,
|
||||
mode='nearest',
|
||||
)
|
||||
|
||||
fuse = torch.cat([p5, p4, p3, p2], dim=1)
|
||||
return fuse
|
||||
|
||||
|
||||
class RSELayer(nn.Module):
|
||||
|
||||
def __init__(self, in_channels, out_channels, kernel_size, shortcut=True):
|
||||
super(RSELayer, self).__init__()
|
||||
self.out_channels = out_channels
|
||||
self.in_conv = nn.Conv2d(
|
||||
in_channels=in_channels,
|
||||
out_channels=self.out_channels,
|
||||
kernel_size=kernel_size,
|
||||
padding=int(kernel_size // 2),
|
||||
bias=False)
|
||||
self.se_block = SEModule(self.out_channels)
|
||||
self.shortcut = shortcut
|
||||
|
||||
def forward(self, ins):
|
||||
x = self.in_conv(ins)
|
||||
if self.shortcut:
|
||||
out = x + self.se_block(x)
|
||||
else:
|
||||
out = self.se_block(x)
|
||||
return out
|
||||
|
||||
|
||||
@NECKS.register_module()
|
||||
class RSEFPN(nn.Module):
|
||||
|
||||
def __init__(self, in_channels, out_channels, shortcut=True, **kwargs):
|
||||
super(RSEFPN, self).__init__()
|
||||
self.out_channels = out_channels
|
||||
self.ins_conv = nn.ModuleList()
|
||||
self.inp_conv = nn.ModuleList()
|
||||
|
||||
for i in range(len(in_channels)):
|
||||
self.ins_conv.append(
|
||||
RSELayer(
|
||||
in_channels[i],
|
||||
out_channels,
|
||||
kernel_size=1,
|
||||
shortcut=shortcut))
|
||||
self.inp_conv.append(
|
||||
RSELayer(
|
||||
out_channels,
|
||||
out_channels // 4,
|
||||
kernel_size=3,
|
||||
shortcut=shortcut))
|
||||
|
||||
def forward(self, x):
|
||||
c2, c3, c4, c5 = x
|
||||
|
||||
in5 = self.ins_conv[3](c5)
|
||||
in4 = self.ins_conv[2](c4)
|
||||
in3 = self.ins_conv[1](c3)
|
||||
in2 = self.ins_conv[0](c2)
|
||||
|
||||
out4 = in4 + F.upsample(in5, scale_factor=2, mode='nearest') # 1/16
|
||||
out3 = in3 + F.upsample(out4, scale_factor=2, mode='nearest') # 1/8
|
||||
out2 = in2 + F.upsample(out3, scale_factor=2, mode='nearest') # 1/4
|
||||
|
||||
p5 = self.inp_conv[3](in5)
|
||||
p4 = self.inp_conv[2](out4)
|
||||
p3 = self.inp_conv[1](out3)
|
||||
p2 = self.inp_conv[0](out2)
|
||||
|
||||
p5 = F.upsample(p5, scale_factor=8, mode='nearest')
|
||||
p4 = F.upsample(p4, scale_factor=4, mode='nearest')
|
||||
p3 = F.upsample(p3, scale_factor=2, mode='nearest')
|
||||
|
||||
fuse = torch.cat([p5, p4, p3, p2], dim=1)
|
||||
return fuse
|
||||
|
||||
|
||||
@NECKS.register_module()
|
||||
class LKPAN(nn.Module):
|
||||
|
||||
def __init__(self, in_channels, out_channels, mode='large', **kwargs):
|
||||
super(LKPAN, self).__init__()
|
||||
self.out_channels = out_channels
|
||||
|
||||
self.ins_conv = nn.ModuleList()
|
||||
self.inp_conv = nn.ModuleList()
|
||||
# pan head
|
||||
self.pan_head_conv = nn.ModuleList()
|
||||
self.pan_lat_conv = nn.ModuleList()
|
||||
|
||||
if mode.lower() == 'lite':
|
||||
p_layer = DSConv
|
||||
elif mode.lower() == 'large':
|
||||
p_layer = nn.Conv2d
|
||||
else:
|
||||
raise ValueError(
|
||||
"mode can only be one of ['lite', 'large'], but received {}".
|
||||
format(mode))
|
||||
|
||||
for i in range(len(in_channels)):
|
||||
self.ins_conv.append(
|
||||
nn.Conv2d(
|
||||
in_channels=in_channels[i],
|
||||
out_channels=self.out_channels,
|
||||
kernel_size=1,
|
||||
bias=False))
|
||||
|
||||
self.inp_conv.append(
|
||||
p_layer(
|
||||
in_channels=self.out_channels,
|
||||
out_channels=self.out_channels // 4,
|
||||
kernel_size=9,
|
||||
padding=4,
|
||||
bias=False))
|
||||
|
||||
if i > 0:
|
||||
self.pan_head_conv.append(
|
||||
nn.Conv2d(
|
||||
in_channels=self.out_channels // 4,
|
||||
out_channels=self.out_channels // 4,
|
||||
kernel_size=3,
|
||||
padding=1,
|
||||
stride=2,
|
||||
bias=False))
|
||||
self.pan_lat_conv.append(
|
||||
p_layer(
|
||||
in_channels=self.out_channels // 4,
|
||||
out_channels=self.out_channels // 4,
|
||||
kernel_size=9,
|
||||
padding=4,
|
||||
bias=False))
|
||||
|
||||
def forward(self, x):
|
||||
c2, c3, c4, c5 = x
|
||||
|
||||
in5 = self.ins_conv[3](c5)
|
||||
in4 = self.ins_conv[2](c4)
|
||||
in3 = self.ins_conv[1](c3)
|
||||
in2 = self.ins_conv[0](c2)
|
||||
|
||||
out4 = in4 + F.upsample(in5, scale_factor=2, mode='nearest') # 1/16
|
||||
out3 = in3 + F.upsample(out4, scale_factor=2, mode='nearest') # 1/8
|
||||
out2 = in2 + F.upsample(out3, scale_factor=2, mode='nearest') # 1/4
|
||||
|
||||
f5 = self.inp_conv[3](in5)
|
||||
f4 = self.inp_conv[2](out4)
|
||||
f3 = self.inp_conv[1](out3)
|
||||
f2 = self.inp_conv[0](out2)
|
||||
|
||||
pan3 = f3 + self.pan_head_conv[0](f2)
|
||||
pan4 = f4 + self.pan_head_conv[1](pan3)
|
||||
pan5 = f5 + self.pan_head_conv[2](pan4)
|
||||
|
||||
p2 = self.pan_lat_conv[0](f2)
|
||||
p3 = self.pan_lat_conv[1](pan3)
|
||||
p4 = self.pan_lat_conv[2](pan4)
|
||||
p5 = self.pan_lat_conv[3](pan5)
|
||||
|
||||
p5 = F.upsample(p5, scale_factor=8, mode='nearest')
|
||||
p4 = F.upsample(p4, scale_factor=4, mode='nearest')
|
||||
p3 = F.upsample(p3, scale_factor=2, mode='nearest')
|
||||
|
||||
fuse = torch.cat([p5, p4, p3, p2], dim=1)
|
||||
return fuse
|
|
@ -0,0 +1,225 @@
|
|||
# Modified from https://github.com/PaddlePaddle/PaddleOCR/tree/release/2.6/ppocr/modeling/necks
|
||||
import torch
|
||||
import torch.nn as nn
|
||||
|
||||
from easycv.models.registry import NECKS
|
||||
from ..backbones.rec_svtrnet import Block, ConvBNLayer
|
||||
|
||||
|
||||
class Im2Seq(nn.Module):
|
||||
|
||||
def __init__(self, in_channels, **kwargs):
|
||||
super().__init__()
|
||||
self.out_channels = in_channels
|
||||
|
||||
def forward(self, x):
|
||||
B, C, H, W = x.shape
|
||||
# assert H == 1
|
||||
x = x.squeeze(dim=2)
|
||||
# x = x.transpose([0, 2, 1]) # paddle (NTC)(batch, width, channels)
|
||||
x = x.permute(0, 2, 1)
|
||||
return x
|
||||
|
||||
|
||||
class EncoderWithRNN_(nn.Module):
|
||||
|
||||
def __init__(self, in_channels, hidden_size):
|
||||
super(EncoderWithRNN_, self).__init__()
|
||||
self.out_channels = hidden_size * 2
|
||||
self.rnn1 = nn.LSTM(
|
||||
in_channels,
|
||||
hidden_size,
|
||||
bidirectional=False,
|
||||
batch_first=True,
|
||||
num_layers=2)
|
||||
self.rnn2 = nn.LSTM(
|
||||
in_channels,
|
||||
hidden_size,
|
||||
bidirectional=False,
|
||||
batch_first=True,
|
||||
num_layers=2)
|
||||
|
||||
def forward(self, x):
|
||||
self.rnn1.flatten_parameters()
|
||||
self.rnn2.flatten_parameters()
|
||||
out1, h1 = self.rnn1(x)
|
||||
out2, h2 = self.rnn2(torch.flip(x, [1]))
|
||||
return torch.cat([out1, torch.flip(out2, [1])], 2)
|
||||
|
||||
|
||||
class EncoderWithRNN(nn.Module):
|
||||
|
||||
def __init__(self, in_channels, hidden_size):
|
||||
super(EncoderWithRNN, self).__init__()
|
||||
self.out_channels = hidden_size * 2
|
||||
self.lstm = nn.LSTM(
|
||||
in_channels,
|
||||
hidden_size,
|
||||
num_layers=2,
|
||||
batch_first=True,
|
||||
bidirectional=True) # batch_first:=True
|
||||
|
||||
def forward(self, x):
|
||||
x, _ = self.lstm(x)
|
||||
return x
|
||||
|
||||
|
||||
class EncoderWithFC(nn.Module):
|
||||
|
||||
def __init__(self, in_channels, hidden_size):
|
||||
super(EncoderWithFC, self).__init__()
|
||||
self.out_channels = hidden_size
|
||||
self.fc = nn.Linear(
|
||||
in_channels,
|
||||
hidden_size,
|
||||
bias=True,
|
||||
)
|
||||
|
||||
def forward(self, x):
|
||||
x = self.fc(x)
|
||||
return x
|
||||
|
||||
|
||||
class EncoderWithSVTR(nn.Module):
|
||||
|
||||
def __init__(
|
||||
self,
|
||||
in_channels,
|
||||
dims=64, # XS
|
||||
depth=2,
|
||||
hidden_dims=120,
|
||||
use_guide=False,
|
||||
num_heads=8,
|
||||
qkv_bias=True,
|
||||
mlp_ratio=2.0,
|
||||
drop_rate=0.1,
|
||||
attn_drop_rate=0.1,
|
||||
drop_path=0.,
|
||||
qk_scale=None,
|
||||
**kwargs):
|
||||
super(EncoderWithSVTR, self).__init__()
|
||||
self.depth = depth
|
||||
self.use_guide = use_guide
|
||||
self.conv1 = ConvBNLayer(
|
||||
in_channels, in_channels // 8, padding=1, act='swish')
|
||||
self.conv2 = ConvBNLayer(
|
||||
in_channels // 8, hidden_dims, kernel_size=1, act='swish')
|
||||
|
||||
self.svtr_block = nn.ModuleList([
|
||||
Block(
|
||||
dim=hidden_dims,
|
||||
num_heads=num_heads,
|
||||
mixer='Global',
|
||||
HW=None,
|
||||
mlp_ratio=mlp_ratio,
|
||||
qkv_bias=qkv_bias,
|
||||
qk_scale=qk_scale,
|
||||
drop=drop_rate,
|
||||
act_layer='swish',
|
||||
attn_drop=attn_drop_rate,
|
||||
drop_path=drop_path,
|
||||
norm_layer='nn.LayerNorm',
|
||||
epsilon=1e-05,
|
||||
prenorm=False) for i in range(depth)
|
||||
])
|
||||
self.norm = nn.LayerNorm(hidden_dims, eps=1e-6)
|
||||
self.conv3 = ConvBNLayer(
|
||||
hidden_dims, in_channels, kernel_size=1, act='swish')
|
||||
# last conv-nxn, the input is concat of input tensor and conv3 output tensor
|
||||
self.conv4 = ConvBNLayer(
|
||||
2 * in_channels, in_channels // 8, padding=1, act='swish')
|
||||
|
||||
self.conv1x1 = ConvBNLayer(
|
||||
in_channels // 8, dims, kernel_size=1, act='swish')
|
||||
self.out_channels = dims
|
||||
self.apply(self._init_weights)
|
||||
|
||||
def _init_weights(self, m):
|
||||
# weight initialization
|
||||
if isinstance(m, nn.Conv2d):
|
||||
nn.init.kaiming_normal_(m.weight, mode='fan_out')
|
||||
if m.bias is not None:
|
||||
nn.init.zeros_(m.bias)
|
||||
elif isinstance(m, nn.BatchNorm2d):
|
||||
nn.init.ones_(m.weight)
|
||||
nn.init.zeros_(m.bias)
|
||||
elif isinstance(m, nn.Linear):
|
||||
nn.init.normal_(m.weight, 0, 0.01)
|
||||
if m.bias is not None:
|
||||
nn.init.zeros_(m.bias)
|
||||
elif isinstance(m, nn.ConvTranspose2d):
|
||||
nn.init.kaiming_normal_(m.weight, mode='fan_out')
|
||||
if m.bias is not None:
|
||||
nn.init.zeros_(m.bias)
|
||||
elif isinstance(m, nn.LayerNorm):
|
||||
nn.init.ones_(m.weight)
|
||||
nn.init.zeros_(m.bias)
|
||||
|
||||
def forward(self, x):
|
||||
# for use guide
|
||||
if self.use_guide:
|
||||
z = x.clone()
|
||||
z.stop_gradient = True
|
||||
else:
|
||||
z = x
|
||||
# for short cut
|
||||
h = z
|
||||
# reduce dim
|
||||
z = self.conv1(z)
|
||||
z = self.conv2(z)
|
||||
# SVTR global block
|
||||
B, C, H, W = z.shape
|
||||
z = z.flatten(2).permute(0, 2, 1)
|
||||
|
||||
for blk in self.svtr_block:
|
||||
z = blk(z)
|
||||
|
||||
z = self.norm(z)
|
||||
# last stage
|
||||
z = z.reshape([-1, H, W, C]).permute(0, 3, 1, 2)
|
||||
z = self.conv3(z)
|
||||
z = torch.cat((h, z), dim=1)
|
||||
z = self.conv1x1(self.conv4(z))
|
||||
|
||||
return z
|
||||
|
||||
|
||||
@NECKS.register_module()
|
||||
class SequenceEncoder(nn.Module):
|
||||
|
||||
def __init__(self, in_channels, encoder_type, hidden_size=48, **kwargs):
|
||||
super(SequenceEncoder, self).__init__()
|
||||
self.encoder_reshape = Im2Seq(in_channels)
|
||||
self.out_channels = self.encoder_reshape.out_channels
|
||||
self.encoder_type = encoder_type
|
||||
if encoder_type == 'reshape':
|
||||
self.only_reshape = True
|
||||
else:
|
||||
support_encoder_dict = {
|
||||
'reshape': Im2Seq,
|
||||
'fc': EncoderWithFC,
|
||||
'rnn': EncoderWithRNN,
|
||||
'svtr': EncoderWithSVTR,
|
||||
}
|
||||
assert encoder_type in support_encoder_dict, '{} must in {}'.format(
|
||||
encoder_type, support_encoder_dict.keys())
|
||||
|
||||
if encoder_type == 'svtr':
|
||||
self.encoder = support_encoder_dict[encoder_type](
|
||||
self.encoder_reshape.out_channels, **kwargs)
|
||||
else:
|
||||
self.encoder = support_encoder_dict[encoder_type](
|
||||
self.encoder_reshape.out_channels, hidden_size)
|
||||
self.out_channels = self.encoder.out_channels
|
||||
self.only_reshape = False
|
||||
|
||||
def forward(self, x):
|
||||
if self.encoder_type != 'svtr':
|
||||
x = self.encoder_reshape(x)
|
||||
if not self.only_reshape:
|
||||
x = self.encoder(x)
|
||||
return x
|
||||
else:
|
||||
x = self.encoder(x)
|
||||
x = self.encoder_reshape(x)
|
||||
return x
|
|
@ -0,0 +1 @@
|
|||
# Copyright (c) Alibaba, Inc. and its affiliates.
|
|
@ -0,0 +1,192 @@
|
|||
# copyright (c) 2020 PaddlePaddle Authors. All Rights Reserve.
|
||||
#
|
||||
# Licensed under the Apache License, Version 2.0 (the "License");
|
||||
# you may not use this file except in compliance with the License.
|
||||
# You may obtain a copy of the License at
|
||||
#
|
||||
# http://www.apache.org/licenses/LICENSE-2.0
|
||||
#
|
||||
# Unless required by applicable law or agreed to in writing, software
|
||||
# distributed under the License is distributed on an "AS IS" BASIS,
|
||||
# WITHOUT WARRANTIES OR CONDITIONS OF ANY KIND, either express or implied.
|
||||
# See the License for the specific language governing permissions and
|
||||
# limitations under the License.
|
||||
# Modified from https://github.com/PaddlePaddle/PaddleOCR/blob/release/2.6/ppocr/postprocess/db_postprocess.py
|
||||
"""
|
||||
This code is refered from:
|
||||
https://github.com/WenmuZhou/DBNet.pytorch/blob/master/post_processing/seg_detector_representer.py
|
||||
"""
|
||||
import cv2
|
||||
import numpy as np
|
||||
import pyclipper
|
||||
import torch
|
||||
from shapely.geometry import Polygon
|
||||
|
||||
|
||||
class DBPostProcess(object):
|
||||
"""
|
||||
The post process for Differentiable Binarization (DB).
|
||||
"""
|
||||
|
||||
def __init__(self,
|
||||
thresh=0.3,
|
||||
box_thresh=0.7,
|
||||
max_candidates=1000,
|
||||
unclip_ratio=2.0,
|
||||
use_dilation=False,
|
||||
score_mode='fast',
|
||||
**kwargs):
|
||||
self.thresh = thresh
|
||||
self.box_thresh = box_thresh
|
||||
self.max_candidates = max_candidates
|
||||
self.unclip_ratio = unclip_ratio
|
||||
self.min_size = 3
|
||||
self.score_mode = score_mode
|
||||
assert score_mode in [
|
||||
'slow', 'fast'
|
||||
], 'Score mode must be in [slow, fast] but got: {}'.format(score_mode)
|
||||
|
||||
self.dilation_kernel = None if not use_dilation else np.array([[1, 1],
|
||||
[1, 1]])
|
||||
|
||||
def boxes_from_bitmap(self, pred, _bitmap, dest_width, dest_height):
|
||||
'''
|
||||
_bitmap: single map with shape (1, H, W),
|
||||
whose values are binarized as {0, 1}
|
||||
'''
|
||||
|
||||
bitmap = _bitmap
|
||||
# cv2.imwrite('mask.jpg',(bitmap * 255).astype(np.uint8))
|
||||
height, width = bitmap.shape
|
||||
|
||||
outs = cv2.findContours((bitmap * 255).astype(np.uint8), cv2.RETR_LIST,
|
||||
cv2.CHAIN_APPROX_SIMPLE)
|
||||
if len(outs) == 3:
|
||||
img, contours, _ = outs[0], outs[1], outs[2]
|
||||
elif len(outs) == 2:
|
||||
contours, _ = outs[0], outs[1]
|
||||
|
||||
num_contours = min(len(contours), self.max_candidates)
|
||||
|
||||
boxes = []
|
||||
scores = []
|
||||
for index in range(num_contours):
|
||||
contour = contours[index]
|
||||
points, sside = self.get_mini_boxes(contour)
|
||||
if sside < self.min_size:
|
||||
continue
|
||||
points = np.array(points)
|
||||
if self.score_mode == 'fast':
|
||||
score = self.box_score_fast(pred, points.reshape(-1, 2))
|
||||
else:
|
||||
score = self.box_score_slow(pred, contour)
|
||||
if self.box_thresh > score:
|
||||
continue
|
||||
|
||||
box = self.unclip(points).reshape(-1, 1, 2)
|
||||
box, sside = self.get_mini_boxes(box)
|
||||
if sside < self.min_size + 2:
|
||||
continue
|
||||
box = np.array(box)
|
||||
|
||||
box[:, 0] = np.clip(
|
||||
np.round(box[:, 0] / width * dest_width), 0, dest_width)
|
||||
box[:, 1] = np.clip(
|
||||
np.round(box[:, 1] / height * dest_height), 0, dest_height)
|
||||
boxes.append(box.astype(np.int16))
|
||||
scores.append(score)
|
||||
return np.array(boxes, dtype=np.int16), scores
|
||||
|
||||
def unclip(self, box):
|
||||
unclip_ratio = self.unclip_ratio
|
||||
poly = Polygon(box)
|
||||
distance = poly.area * unclip_ratio / poly.length
|
||||
offset = pyclipper.PyclipperOffset()
|
||||
offset.AddPath(box, pyclipper.JT_ROUND, pyclipper.ET_CLOSEDPOLYGON)
|
||||
expanded = np.array(offset.Execute(distance))
|
||||
return expanded
|
||||
|
||||
def get_mini_boxes(self, contour):
|
||||
bounding_box = cv2.minAreaRect(contour)
|
||||
points = sorted(list(cv2.boxPoints(bounding_box)), key=lambda x: x[0])
|
||||
|
||||
index_1, index_2, index_3, index_4 = 0, 1, 2, 3
|
||||
if points[1][1] > points[0][1]:
|
||||
index_1 = 0
|
||||
index_4 = 1
|
||||
else:
|
||||
index_1 = 1
|
||||
index_4 = 0
|
||||
if points[3][1] > points[2][1]:
|
||||
index_2 = 2
|
||||
index_3 = 3
|
||||
else:
|
||||
index_2 = 3
|
||||
index_3 = 2
|
||||
|
||||
box = [
|
||||
points[index_1], points[index_2], points[index_3], points[index_4]
|
||||
]
|
||||
return box, min(bounding_box[1])
|
||||
|
||||
def box_score_fast(self, bitmap, _box):
|
||||
'''
|
||||
box_score_fast: use bbox mean score as the mean score
|
||||
'''
|
||||
h, w = bitmap.shape[:2]
|
||||
box = _box.copy()
|
||||
xmin = np.clip(np.floor(box[:, 0].min()).astype(np.int), 0, w - 1)
|
||||
xmax = np.clip(np.ceil(box[:, 0].max()).astype(np.int), 0, w - 1)
|
||||
ymin = np.clip(np.floor(box[:, 1].min()).astype(np.int), 0, h - 1)
|
||||
ymax = np.clip(np.ceil(box[:, 1].max()).astype(np.int), 0, h - 1)
|
||||
|
||||
mask = np.zeros((ymax - ymin + 1, xmax - xmin + 1), dtype=np.uint8)
|
||||
box[:, 0] = box[:, 0] - xmin
|
||||
box[:, 1] = box[:, 1] - ymin
|
||||
cv2.fillPoly(mask, box.reshape(1, -1, 2).astype(np.int32), 1)
|
||||
return cv2.mean(bitmap[ymin:ymax + 1, xmin:xmax + 1], mask)[0]
|
||||
|
||||
def box_score_slow(self, bitmap, contour):
|
||||
'''
|
||||
box_score_slow: use polyon mean score as the mean score
|
||||
'''
|
||||
h, w = bitmap.shape[:2]
|
||||
contour = contour.copy()
|
||||
contour = np.reshape(contour, (-1, 2))
|
||||
|
||||
xmin = np.clip(np.min(contour[:, 0]), 0, w - 1)
|
||||
xmax = np.clip(np.max(contour[:, 0]), 0, w - 1)
|
||||
ymin = np.clip(np.min(contour[:, 1]), 0, h - 1)
|
||||
ymax = np.clip(np.max(contour[:, 1]), 0, h - 1)
|
||||
|
||||
mask = np.zeros((ymax - ymin + 1, xmax - xmin + 1), dtype=np.uint8)
|
||||
|
||||
contour[:, 0] = contour[:, 0] - xmin
|
||||
contour[:, 1] = contour[:, 1] - ymin
|
||||
|
||||
cv2.fillPoly(mask, contour.reshape(1, -1, 2).astype(np.int32), 1)
|
||||
return cv2.mean(bitmap[ymin:ymax + 1, xmin:xmax + 1], mask)[0]
|
||||
|
||||
def __call__(self, outs_dict, shape_list):
|
||||
pred = outs_dict['maps']
|
||||
if isinstance(pred, torch.Tensor):
|
||||
pred = pred.cpu().detach().numpy()
|
||||
pred = pred[:, 0, :, :]
|
||||
segmentation = pred > self.thresh
|
||||
|
||||
# boxes_batch = []
|
||||
boxes_batch = {'points': []}
|
||||
for batch_index in range(pred.shape[0]):
|
||||
src_h, src_w, c = shape_list[batch_index]
|
||||
if self.dilation_kernel is not None:
|
||||
mask = cv2.dilate(
|
||||
np.array(segmentation[batch_index]).astype(np.uint8),
|
||||
self.dilation_kernel)
|
||||
else:
|
||||
mask = segmentation[batch_index]
|
||||
boxes, scores = self.boxes_from_bitmap(pred[batch_index], mask,
|
||||
src_w, src_h)
|
||||
|
||||
# boxes_batch.append({'points': boxes})
|
||||
boxes_batch['points'].append(boxes)
|
||||
return boxes_batch
|
|
@ -0,0 +1,198 @@
|
|||
# copyright (c) 2020 PaddlePaddle Authors. All Rights Reserve.
|
||||
#
|
||||
# Licensed under the Apache License, Version 2.0 (the "License");
|
||||
# you may not use this file except in compliance with the License.
|
||||
# You may obtain a copy of the License at
|
||||
#
|
||||
# http://www.apache.org/licenses/LICENSE-2.0
|
||||
#
|
||||
# Unless required by applicable law or agreed to in writing, software
|
||||
# distributed under the License is distributed on an "AS IS" BASIS,
|
||||
# WITHOUT WARRANTIES OR CONDITIONS OF ANY KIND, either express or implied.
|
||||
# See the License for the specific language governing permissions and
|
||||
# limitations under the License.
|
||||
# Modified from https://github.com/PaddlePaddle/PaddleOCR/blob/release/2.6/ppocr/postprocess/rec_postprocess.py
|
||||
import os.path as osp
|
||||
import re
|
||||
import string
|
||||
|
||||
import numpy as np
|
||||
import requests
|
||||
import torch
|
||||
|
||||
|
||||
class BaseRecLabelDecode(object):
|
||||
""" Convert between text-label and text-index """
|
||||
|
||||
def __init__(self, character_dict_path=None, use_space_char=False):
|
||||
self.beg_str = 'sos'
|
||||
self.end_str = 'eos'
|
||||
|
||||
self.character_str = []
|
||||
if character_dict_path is None:
|
||||
self.character_str = '0123456789abcdefghijklmnopqrstuvwxyz'
|
||||
dict_character = list(self.character_str)
|
||||
else:
|
||||
if character_dict_path.startswith('http'):
|
||||
r = requests.get(character_dict_path)
|
||||
tpath = character_dict_path.split('/')[-1]
|
||||
while not osp.exists(tpath):
|
||||
try:
|
||||
with open(tpath, 'wb') as code:
|
||||
code.write(r.content)
|
||||
except:
|
||||
pass
|
||||
character_dict_path = tpath
|
||||
with open(character_dict_path, 'rb') as fin:
|
||||
lines = fin.readlines()
|
||||
for line in lines:
|
||||
line = line.decode('utf-8').strip('\n').strip('\r\n')
|
||||
self.character_str.append(line)
|
||||
if use_space_char:
|
||||
self.character_str.append(' ')
|
||||
dict_character = list(self.character_str)
|
||||
|
||||
dict_character = self.add_special_char(dict_character)
|
||||
self.dict = {}
|
||||
for i, char in enumerate(dict_character):
|
||||
self.dict[char] = i
|
||||
self.character = dict_character
|
||||
|
||||
def add_special_char(self, dict_character):
|
||||
return dict_character
|
||||
|
||||
def decode(self, text_index, text_prob=None, is_remove_duplicate=False):
|
||||
""" convert text-index into text-label. """
|
||||
result_list = []
|
||||
ignored_tokens = self.get_ignored_tokens()
|
||||
batch_size = len(text_index)
|
||||
for batch_idx in range(batch_size):
|
||||
selection = np.ones(len(text_index[batch_idx]), dtype=bool)
|
||||
if is_remove_duplicate:
|
||||
selection[1:] = text_index[batch_idx][1:] != text_index[
|
||||
batch_idx][:-1]
|
||||
for ignored_token in ignored_tokens:
|
||||
selection &= text_index[batch_idx] != ignored_token
|
||||
char_list = [
|
||||
self.character[text_id]
|
||||
for text_id in text_index[batch_idx][selection]
|
||||
]
|
||||
if text_prob is not None:
|
||||
conf_list = text_prob[batch_idx][selection]
|
||||
else:
|
||||
conf_list = [1] * len(selection)
|
||||
if len(conf_list) == 0:
|
||||
conf_list = [0]
|
||||
|
||||
text = ''.join(char_list)
|
||||
result_list.append((text, np.mean(conf_list).tolist()))
|
||||
return result_list
|
||||
|
||||
def get_ignored_tokens(self):
|
||||
return [0] # for ctc blank
|
||||
|
||||
|
||||
class CTCLabelDecode(BaseRecLabelDecode):
|
||||
""" Convert between text-label and text-index """
|
||||
|
||||
def __init__(self,
|
||||
character_dict_path=None,
|
||||
use_space_char=False,
|
||||
**kwargs):
|
||||
super(CTCLabelDecode, self).__init__(character_dict_path,
|
||||
use_space_char)
|
||||
|
||||
def __call__(self, preds, label=None, *args, **kwargs):
|
||||
if isinstance(preds, tuple) or isinstance(preds, list):
|
||||
preds = preds[-1]
|
||||
if isinstance(preds, torch.Tensor):
|
||||
preds = preds.numpy()
|
||||
preds_idx = preds.argmax(axis=2)
|
||||
preds_prob = preds.max(axis=2)
|
||||
text = self.decode(preds_idx, preds_prob, is_remove_duplicate=True)
|
||||
if label is None:
|
||||
return text
|
||||
label = self.decode(label)
|
||||
return text, label
|
||||
|
||||
def add_special_char(self, dict_character):
|
||||
dict_character = ['blank'] + dict_character
|
||||
return dict_character
|
||||
|
||||
|
||||
class SARLabelDecode(BaseRecLabelDecode):
|
||||
""" Convert between text-label and text-index """
|
||||
|
||||
def __init__(self,
|
||||
character_dict_path=None,
|
||||
use_space_char=False,
|
||||
**kwargs):
|
||||
super(SARLabelDecode, self).__init__(character_dict_path,
|
||||
use_space_char)
|
||||
|
||||
self.rm_symbol = kwargs.get('rm_symbol', False)
|
||||
|
||||
def add_special_char(self, dict_character):
|
||||
beg_end_str = '<BOS/EOS>'
|
||||
unknown_str = '<UKN>'
|
||||
padding_str = '<PAD>'
|
||||
dict_character = dict_character + [unknown_str]
|
||||
self.unknown_idx = len(dict_character) - 1
|
||||
dict_character = dict_character + [beg_end_str]
|
||||
self.start_idx = len(dict_character) - 1
|
||||
self.end_idx = len(dict_character) - 1
|
||||
dict_character = dict_character + [padding_str]
|
||||
self.padding_idx = len(dict_character) - 1
|
||||
return dict_character
|
||||
|
||||
def decode(self, text_index, text_prob=None, is_remove_duplicate=False):
|
||||
""" convert text-index into text-label. """
|
||||
result_list = []
|
||||
ignored_tokens = self.get_ignored_tokens()
|
||||
|
||||
batch_size = len(text_index)
|
||||
for batch_idx in range(batch_size):
|
||||
char_list = []
|
||||
conf_list = []
|
||||
for idx in range(len(text_index[batch_idx])):
|
||||
if text_index[batch_idx][idx] in ignored_tokens:
|
||||
continue
|
||||
if int(text_index[batch_idx][idx]) == int(self.end_idx):
|
||||
if text_prob is None and idx == 0:
|
||||
continue
|
||||
else:
|
||||
break
|
||||
if is_remove_duplicate:
|
||||
# only for predict
|
||||
if idx > 0 and text_index[batch_idx][
|
||||
idx - 1] == text_index[batch_idx][idx]:
|
||||
continue
|
||||
char_list.append(self.character[int(
|
||||
text_index[batch_idx][idx])])
|
||||
if text_prob is not None:
|
||||
conf_list.append(text_prob[batch_idx][idx])
|
||||
else:
|
||||
conf_list.append(1)
|
||||
text = ''.join(char_list)
|
||||
if self.rm_symbol:
|
||||
comp = re.compile('[^A-Z^a-z^0-9^\u4e00-\u9fa5]')
|
||||
text = text.lower()
|
||||
text = comp.sub('', text)
|
||||
result_list.append((text, np.mean(conf_list).tolist()))
|
||||
return result_list
|
||||
|
||||
def __call__(self, preds, label=None, *args, **kwargs):
|
||||
if isinstance(preds, torch.Tensor):
|
||||
preds = preds.cpu().numpy()
|
||||
preds_idx = preds.argmax(axis=2)
|
||||
preds_prob = preds.max(axis=2)
|
||||
|
||||
text = self.decode(preds_idx, preds_prob, is_remove_duplicate=False)
|
||||
|
||||
if label is None:
|
||||
return text
|
||||
label = self.decode(label, is_remove_duplicate=False)
|
||||
return text, label
|
||||
|
||||
def get_ignored_tokens(self):
|
||||
return [self.padding_idx]
|
|
@ -0,0 +1,2 @@
|
|||
# Copyright (c) Alibaba, Inc. and its affiliates.
|
||||
from .ocr_rec import OCRRecNet
|
|
@ -0,0 +1,115 @@
|
|||
# Copyright (c) Alibaba, Inc. and its affiliates.
|
||||
import numpy as np
|
||||
import torch
|
||||
import torch.nn as nn
|
||||
import torch.nn.functional as F
|
||||
|
||||
from easycv.models import builder
|
||||
from easycv.models.base import BaseModel
|
||||
from easycv.models.builder import MODELS
|
||||
from easycv.models.ocr.postprocess.rec_postprocess import CTCLabelDecode
|
||||
from easycv.utils.checkpoint import load_checkpoint
|
||||
from easycv.utils.logger import get_root_logger
|
||||
|
||||
|
||||
@MODELS.register_module()
|
||||
class OCRRecNet(BaseModel):
|
||||
"""for text recognition
|
||||
"""
|
||||
|
||||
def __init__(
|
||||
self,
|
||||
backbone,
|
||||
head,
|
||||
postprocess,
|
||||
neck=None,
|
||||
loss=None,
|
||||
pretrained=None,
|
||||
**kwargs,
|
||||
):
|
||||
super(OCRRecNet, self).__init__()
|
||||
|
||||
self.pretrained = pretrained
|
||||
|
||||
# self.backbone = eval(backbone.type)(**backbone)
|
||||
self.backbone = builder.build_backbone(backbone)
|
||||
self.neck = builder.build_neck(neck) if neck else None
|
||||
self.head = builder.build_head(head)
|
||||
self.loss = builder.build_loss(loss) if loss else None
|
||||
self.postprocess_op = eval(postprocess.type)(**postprocess)
|
||||
self.init_weights()
|
||||
|
||||
def init_weights(self):
|
||||
logger = get_root_logger()
|
||||
if self.pretrained:
|
||||
load_checkpoint(self, self.pretrained, strict=False, logger=logger)
|
||||
else:
|
||||
# weight initialization
|
||||
for m in self.modules():
|
||||
if isinstance(m, nn.Conv2d) or isinstance(
|
||||
m, nn.ConvTranspose2d):
|
||||
nn.init.kaiming_normal_(m.weight, mode='fan_out')
|
||||
if m.bias is not None:
|
||||
nn.init.zeros_(m.bias)
|
||||
elif isinstance(m, nn.BatchNorm2d):
|
||||
nn.init.ones_(m.weight)
|
||||
if m.bias is not None:
|
||||
nn.init.zeros_(m.bias)
|
||||
elif isinstance(m, nn.Linear):
|
||||
nn.init.normal_(m.weight, 0, 0.01)
|
||||
if m.bias is not None:
|
||||
nn.init.zeros_(m.bias)
|
||||
|
||||
def extract_feat(self, x, label=None, valid_ratios=None):
|
||||
y = dict()
|
||||
x = self.backbone(x)
|
||||
y['backbone_out'] = x
|
||||
if self.neck:
|
||||
x = self.neck(x)
|
||||
y['neck_out'] = x
|
||||
x = self.head(x, label=label, valid_ratios=valid_ratios)
|
||||
# for multi head, save ctc neck out for udml
|
||||
if isinstance(x, dict) and 'ctc_nect' in x.keys():
|
||||
y['neck_out'] = x['ctc_neck']
|
||||
y['head_out'] = x
|
||||
elif isinstance(x, dict):
|
||||
y.update(x)
|
||||
else:
|
||||
y['head_out'] = x
|
||||
return y
|
||||
|
||||
def forward_train(self, img, **kwargs):
|
||||
label_ctc = kwargs.get('label_ctc', None)
|
||||
label_sar = kwargs.get('label_sar', None)
|
||||
length = kwargs.get('length', None)
|
||||
valid_ratio = kwargs.get('valid_ratio', None)
|
||||
predicts = self.extract_feat(
|
||||
img, label=label_sar, valid_ratios=valid_ratio)
|
||||
loss = self.loss(
|
||||
predicts, label_ctc=label_ctc, label_sar=label_sar, length=length)
|
||||
return loss
|
||||
|
||||
def forward_test(self, img, **kwargs):
|
||||
label_ctc = kwargs.get('label_ctc', None)
|
||||
result = {}
|
||||
with torch.no_grad():
|
||||
preds = self.extract_feat(img)
|
||||
if label_ctc == None:
|
||||
preds_text = self.postprocess(preds)
|
||||
else:
|
||||
preds_text, label_text = self.postprocess(preds, label_ctc)
|
||||
result['label_text'] = label_text
|
||||
result['preds_text'] = preds_text
|
||||
return result
|
||||
|
||||
def postprocess(self, preds, label=None):
|
||||
if isinstance(preds, dict):
|
||||
preds = preds['head_out']
|
||||
if isinstance(preds, list):
|
||||
preds = [v.cpu().detach().numpy() for v in preds]
|
||||
else:
|
||||
preds = preds.cpu().detach().numpy()
|
||||
label = label.cpu().detach().numpy() if label != None else label
|
||||
text_out = self.postprocess_op(preds, label)
|
||||
|
||||
return text_out
|
|
@ -259,24 +259,21 @@ class PredictorV2(object):
|
|||
"""Process model batch outputs.
|
||||
"""
|
||||
outputs = []
|
||||
out_i = {}
|
||||
batch_size = 1
|
||||
# get current batch size
|
||||
for k, batch_v in inputs.items():
|
||||
if batch_v is not None:
|
||||
batch_size = len(batch_v)
|
||||
break
|
||||
|
||||
for i in range(batch_size):
|
||||
out_i = {}
|
||||
for k, batch_v in inputs.items():
|
||||
if batch_v is not None:
|
||||
out_i[k] = batch_v[i]
|
||||
else:
|
||||
out_i[k] = None
|
||||
|
||||
out_i = self.postprocess_single(out_i, *args, **kwargs)
|
||||
outputs.append(out_i)
|
||||
|
||||
return outputs
|
||||
|
||||
def postprocess_single(self, inputs, *args, **kwargs):
|
||||
|
@ -328,5 +325,4 @@ class PredictorV2(object):
|
|||
results_list.extend(results)
|
||||
else:
|
||||
results_list.append(results)
|
||||
|
||||
return results_list
|
||||
|
|
|
@ -0,0 +1,297 @@
|
|||
# Copyright (c) Alibaba, Inc. and its affiliates.
|
||||
import copy
|
||||
import math
|
||||
import os
|
||||
|
||||
import cv2
|
||||
import numpy as np
|
||||
import torch
|
||||
from PIL import Image, ImageDraw, ImageFont
|
||||
from torchvision.transforms import Compose
|
||||
|
||||
from easycv.datasets.registry import PIPELINES
|
||||
from easycv.file import io
|
||||
from easycv.models import build_model
|
||||
from easycv.predictors.builder import PREDICTORS
|
||||
from easycv.predictors.interface import PredictorInterface
|
||||
from easycv.utils.checkpoint import load_checkpoint
|
||||
from easycv.utils.registry import build_from_cfg
|
||||
from .base import PredictorV2
|
||||
|
||||
|
||||
@PREDICTORS.register_module()
|
||||
class OCRDetPredictor(PredictorV2):
|
||||
|
||||
def __init__(self,
|
||||
model_path,
|
||||
config_file=None,
|
||||
batch_size=1,
|
||||
device=None,
|
||||
save_results=False,
|
||||
save_path=None,
|
||||
pipelines=None,
|
||||
*args,
|
||||
**kwargs):
|
||||
|
||||
super(OCRDetPredictor, self).__init__(
|
||||
model_path,
|
||||
config_file,
|
||||
batch_size=batch_size,
|
||||
device=device,
|
||||
save_results=save_results,
|
||||
save_path=save_path,
|
||||
pipelines=pipelines,
|
||||
*args,
|
||||
**kwargs)
|
||||
|
||||
def show_result(self, dt_boxes, img):
|
||||
img = img.astype(np.uint8)
|
||||
for box in dt_boxes:
|
||||
box = np.array(box).astype(np.int32).reshape(-1, 2)
|
||||
cv2.polylines(img, [box], True, color=(255, 255, 0), thickness=2)
|
||||
return img
|
||||
|
||||
|
||||
@PREDICTORS.register_module()
|
||||
class OCRRecPredictor(PredictorV2):
|
||||
|
||||
def __init__(self,
|
||||
model_path,
|
||||
config_file=None,
|
||||
batch_size=1,
|
||||
device=None,
|
||||
save_results=False,
|
||||
save_path=None,
|
||||
pipelines=None,
|
||||
*args,
|
||||
**kwargs):
|
||||
|
||||
super(OCRRecPredictor, self).__init__(
|
||||
model_path,
|
||||
config_file,
|
||||
batch_size=batch_size,
|
||||
device=device,
|
||||
save_results=save_results,
|
||||
save_path=save_path,
|
||||
pipelines=pipelines,
|
||||
*args,
|
||||
**kwargs)
|
||||
|
||||
|
||||
@PREDICTORS.register_module()
|
||||
class OCRClsPredictor(PredictorV2):
|
||||
|
||||
def __init__(self,
|
||||
model_path,
|
||||
config_file=None,
|
||||
batch_size=1,
|
||||
device=None,
|
||||
save_results=False,
|
||||
save_path=None,
|
||||
pipelines=None,
|
||||
*args,
|
||||
**kwargs):
|
||||
|
||||
super(OCRClsPredictor, self).__init__(
|
||||
model_path,
|
||||
config_file,
|
||||
batch_size=batch_size,
|
||||
device=device,
|
||||
save_results=save_results,
|
||||
save_path=save_path,
|
||||
pipelines=pipelines,
|
||||
*args,
|
||||
**kwargs)
|
||||
|
||||
|
||||
@PREDICTORS.register_module()
|
||||
class OCRPredictor(object):
|
||||
|
||||
def __init__(self,
|
||||
det_model_path,
|
||||
rec_model_path,
|
||||
cls_model_path=None,
|
||||
det_batch_size=1,
|
||||
rec_batch_size=64,
|
||||
cls_batch_size=64,
|
||||
drop_score=0.5,
|
||||
use_angle_cls=False):
|
||||
|
||||
self.use_angle_cls = use_angle_cls
|
||||
if use_angle_cls:
|
||||
self.cls_predictor = OCRClsPredictor(
|
||||
cls_model_path, batch_size=cls_batch_size)
|
||||
self.det_predictor = OCRDetPredictor(
|
||||
det_model_path, batch_size=det_batch_size)
|
||||
self.rec_predictor = OCRRecPredictor(
|
||||
rec_model_path, batch_size=rec_batch_size)
|
||||
self.drop_score = drop_score
|
||||
|
||||
def sorted_boxes(self, dt_boxes):
|
||||
"""
|
||||
Sort text boxes in order from top to bottom, left to right
|
||||
args:
|
||||
dt_boxes(array):detected text boxes with shape [4, 2]
|
||||
return:
|
||||
sorted boxes(array) with shape [4, 2]
|
||||
"""
|
||||
|
||||
num_boxes = dt_boxes.shape[0]
|
||||
sorted_boxes = sorted(dt_boxes, key=lambda x: (x[0][1], x[0][0]))
|
||||
_boxes = list(sorted_boxes)
|
||||
|
||||
for i in range(num_boxes - 1):
|
||||
if abs(_boxes[i + 1][0][1] - _boxes[i][0][1]) < 10 and \
|
||||
(_boxes[i + 1][0][0] < _boxes[i][0][0]):
|
||||
tmp = _boxes[i]
|
||||
_boxes[i] = _boxes[i + 1]
|
||||
_boxes[i + 1] = tmp
|
||||
return _boxes
|
||||
|
||||
def get_rotate_crop_image(self, img, points):
|
||||
'''
|
||||
img_height, img_width = img.shape[0:2]
|
||||
left = int(np.min(points[:, 0]))
|
||||
right = int(np.max(points[:, 0]))
|
||||
top = int(np.min(points[:, 1]))
|
||||
bottom = int(np.max(points[:, 1]))
|
||||
img_crop = img[top:bottom, left:right, :].copy()
|
||||
points[:, 0] = points[:, 0] - left
|
||||
points[:, 1] = points[:, 1] - top
|
||||
'''
|
||||
img_crop_width = int(
|
||||
max(
|
||||
np.linalg.norm(points[0] - points[1]),
|
||||
np.linalg.norm(points[2] - points[3])))
|
||||
img_crop_height = int(
|
||||
max(
|
||||
np.linalg.norm(points[0] - points[3]),
|
||||
np.linalg.norm(points[1] - points[2])))
|
||||
pts_std = np.float32([[0, 0], [img_crop_width, 0],
|
||||
[img_crop_width, img_crop_height],
|
||||
[0, img_crop_height]])
|
||||
points = np.float32(points)
|
||||
M = cv2.getPerspectiveTransform(points, pts_std)
|
||||
dst_img = cv2.warpPerspective(
|
||||
img,
|
||||
M, (img_crop_width, img_crop_height),
|
||||
borderMode=cv2.BORDER_REPLICATE,
|
||||
flags=cv2.INTER_CUBIC)
|
||||
dst_img_height, dst_img_width = dst_img.shape[0:2]
|
||||
if dst_img_height * 1.0 / dst_img_width >= 1.5:
|
||||
dst_img = np.rot90(dst_img)
|
||||
return dst_img
|
||||
|
||||
def __call__(self, inputs):
|
||||
# support srt list(str) list(np.array) as input
|
||||
if isinstance(inputs, str):
|
||||
inputs = [inputs]
|
||||
if isinstance(inputs[0], str):
|
||||
inputs = [cv2.imread(path) for path in inputs]
|
||||
|
||||
dt_boxes_batch = self.det_predictor(inputs)
|
||||
boxes_res = []
|
||||
text_res = []
|
||||
for img, dt_boxes in zip(inputs, dt_boxes_batch):
|
||||
dt_boxes = dt_boxes['points']
|
||||
dt_boxes = self.sorted_boxes(dt_boxes)
|
||||
img_crop_list = []
|
||||
for bno in range(len(dt_boxes)):
|
||||
tmp_box = copy.deepcopy(dt_boxes[bno])
|
||||
img_crop = self.get_rotate_crop_image(img, tmp_box)
|
||||
img_crop_list.append(img_crop)
|
||||
if self.use_angle_cls:
|
||||
cls_res = self.cls_predictor(img_crop_list)
|
||||
img_crop_list, cls_res = self.flip_img(cls_res, img_crop_list)
|
||||
|
||||
rec_res = self.rec_predictor(img_crop_list)
|
||||
filter_boxes, filter_rec_res = [], []
|
||||
for box, rec_reuslt in zip(dt_boxes, rec_res):
|
||||
score = rec_reuslt['preds_text'][1]
|
||||
if score >= self.drop_score:
|
||||
filter_boxes.append(np.float32(box))
|
||||
filter_rec_res.append(rec_reuslt['preds_text'])
|
||||
boxes_res.append(filter_boxes)
|
||||
text_res.append(filter_rec_res)
|
||||
return boxes_res, text_res
|
||||
|
||||
def flip_img(self, result, img_list, threshold=0.9):
|
||||
output = {'labels': [], 'logits': []}
|
||||
img_list_out = []
|
||||
for img, res in zip(img_list, result):
|
||||
label, logit = res['class'], res['neck']
|
||||
output['labels'].append(label)
|
||||
output['logits'].append(logit[label])
|
||||
if label == 1 and logit[label] > threshold:
|
||||
img = cv2.flip(img, -1)
|
||||
img_list_out.append(img)
|
||||
return img_list_out, output
|
||||
|
||||
def show(self, boxes, rec_res, img, drop_score=0.5, font_path=None):
|
||||
if font_path == None:
|
||||
dir_path, _ = os.path.split(os.path.realpath(__file__))
|
||||
font_path = os.path.join(dir_path, '../resource/simhei.ttf')
|
||||
|
||||
img = Image.fromarray(cv2.cvtColor(img, cv2.COLOR_BGR2RGB))
|
||||
txts = [rec_res[i][0] for i in range(len(rec_res))]
|
||||
scores = [rec_res[i][1] for i in range(len(rec_res))]
|
||||
|
||||
draw_img = draw_ocr_box_txt(
|
||||
img, boxes, txts, font_path, scores=scores, drop_score=drop_score)
|
||||
draw_img = draw_img[..., ::-1]
|
||||
return draw_img
|
||||
|
||||
|
||||
def draw_ocr_box_txt(image,
|
||||
boxes,
|
||||
txts,
|
||||
font_path,
|
||||
scores=None,
|
||||
drop_score=0.5):
|
||||
h, w = image.height, image.width
|
||||
img_left = image.copy()
|
||||
img_right = Image.new('RGB', (w, h), (255, 255, 255))
|
||||
|
||||
import random
|
||||
|
||||
random.seed(0)
|
||||
draw_left = ImageDraw.Draw(img_left)
|
||||
draw_right = ImageDraw.Draw(img_right)
|
||||
for idx, (box, txt) in enumerate(zip(boxes, txts)):
|
||||
if scores is not None and scores[idx] < drop_score:
|
||||
continue
|
||||
color = (random.randint(0, 255), random.randint(0, 255),
|
||||
random.randint(0, 255))
|
||||
draw_left.polygon(box, fill=color)
|
||||
draw_right.polygon([
|
||||
box[0][0], box[0][1], box[1][0], box[1][1], box[2][0], box[2][1],
|
||||
box[3][0], box[3][1]
|
||||
],
|
||||
outline=color)
|
||||
box_height = math.sqrt((box[0][0] - box[3][0])**2 +
|
||||
(box[0][1] - box[3][1])**2)
|
||||
box_width = math.sqrt((box[0][0] - box[1][0])**2 +
|
||||
(box[0][1] - box[1][1])**2)
|
||||
if box_height > 2 * box_width:
|
||||
font_size = max(int(box_width * 0.9), 10)
|
||||
font = ImageFont.truetype(font_path, font_size, encoding='utf-8')
|
||||
cur_y = box[0][1]
|
||||
for c in txt:
|
||||
char_size = font.getsize(c)
|
||||
draw_right.text((box[0][0] + 3, cur_y),
|
||||
c,
|
||||
fill=(0, 0, 0),
|
||||
font=font)
|
||||
cur_y += char_size[1]
|
||||
else:
|
||||
font_size = max(int(box_height * 0.8), 10)
|
||||
font = ImageFont.truetype(font_path, font_size, encoding='utf-8')
|
||||
draw_right.text([box[0][0], box[0][1]],
|
||||
txt,
|
||||
fill=(0, 0, 0),
|
||||
font=font)
|
||||
img_left = Image.blend(image, img_left, 0.5)
|
||||
img_show = Image.new('RGB', (w * 2, h), (255, 255, 255))
|
||||
img_show.paste(img_left, (0, 0, w, h))
|
||||
img_show.paste(img_right, (w, 0, w * 2, h))
|
||||
return np.array(img_show)
|
|
@ -5,14 +5,17 @@ future
|
|||
h5py
|
||||
imgaug
|
||||
json_tricks
|
||||
lmdb
|
||||
numpy
|
||||
opencv-python
|
||||
oss2
|
||||
packaging
|
||||
Pillow
|
||||
prettytable
|
||||
pyclipper
|
||||
pycocotools
|
||||
pytorch_metric_learning>=0.9.89
|
||||
rapidfuzz
|
||||
scikit-image
|
||||
sklearn
|
||||
tensorboard
|
||||
|
|
|
@ -0,0 +1,50 @@
|
|||
# Copyright (c) Alibaba, Inc. and its affiliates.
|
||||
import os
|
||||
import unittest
|
||||
|
||||
import torch
|
||||
from tests.ut_config import SMALL_OCR_CLS_DATA
|
||||
|
||||
from easycv.datasets.builder import build_dataset
|
||||
|
||||
|
||||
class OCRClsDatasetTest(unittest.TestCase):
|
||||
|
||||
def setUp(self):
|
||||
print(('Testing %s.%s' % (type(self).__name__, self._testMethodName)))
|
||||
|
||||
def _get_dataset(self):
|
||||
data_root = SMALL_OCR_CLS_DATA
|
||||
data_train_list = os.path.join(data_root, 'label.txt')
|
||||
pipeline = [
|
||||
dict(type='RecAug', use_tia=False),
|
||||
dict(type='ClsResizeImg', img_shape=(3, 48, 192)),
|
||||
dict(type='MMToTensor'),
|
||||
dict(
|
||||
type='Collect', keys=['img', 'label'], meta_keys=['img_path'])
|
||||
]
|
||||
data = dict(
|
||||
train=dict(
|
||||
type='OCRClsDataset',
|
||||
data_source=dict(
|
||||
type='OCRClsSource',
|
||||
label_file=data_train_list,
|
||||
data_dir=SMALL_OCR_CLS_DATA + '/img',
|
||||
label_list=['0', '180'],
|
||||
),
|
||||
pipeline=pipeline))
|
||||
dataset = build_dataset(data['train'])
|
||||
|
||||
return dataset
|
||||
|
||||
def test_default(self):
|
||||
dataset = self._get_dataset()
|
||||
for _, batch in enumerate(dataset):
|
||||
img, target = batch['img'], batch['label']
|
||||
self.assertEqual(img.shape, torch.Size([3, 48, 192]))
|
||||
self.assertIn(target, list(range(2)))
|
||||
break
|
||||
|
||||
|
||||
if __name__ == '__main__':
|
||||
unittest.main()
|
|
@ -0,0 +1,159 @@
|
|||
# Copyright (c) Alibaba, Inc. and its affiliates.
|
||||
import os
|
||||
import unittest
|
||||
|
||||
import torch
|
||||
from tests.ut_config import (IMG_NORM_CFG, SMALL_OCR_DET_DATA,
|
||||
SMALL_OCR_DET_PAI_DATA)
|
||||
|
||||
from easycv.datasets.builder import build_dataset
|
||||
|
||||
|
||||
class OCRDetDatasetTest(unittest.TestCase):
|
||||
|
||||
def setUp(self):
|
||||
print(('Testing %s.%s' % (type(self).__name__, self._testMethodName)))
|
||||
|
||||
def _get_dataset(self):
|
||||
data_root = SMALL_OCR_DET_DATA
|
||||
data_train_list = os.path.join(data_root, 'label.txt')
|
||||
pipeline = [
|
||||
dict(
|
||||
type='IaaAugment',
|
||||
augmenter_args=[{
|
||||
'type': 'Fliplr',
|
||||
'args': {
|
||||
'p': 0.5
|
||||
}
|
||||
}, {
|
||||
'type': 'Affine',
|
||||
'args': {
|
||||
'rotate': [-10, 10]
|
||||
}
|
||||
}, {
|
||||
'type': 'Resize',
|
||||
'args': {
|
||||
'size': [0.5, 3]
|
||||
}
|
||||
}]),
|
||||
dict(
|
||||
type='EastRandomCropData',
|
||||
size=[640, 640],
|
||||
max_tries=50,
|
||||
keep_ratio=True),
|
||||
dict(
|
||||
type='MakeBorderMap',
|
||||
shrink_ratio=0.4,
|
||||
thresh_min=0.3,
|
||||
thresh_max=0.7),
|
||||
dict(type='MakeShrinkMap', shrink_ratio=0.4, min_text_size=8),
|
||||
dict(type='MMNormalize', **IMG_NORM_CFG),
|
||||
dict(
|
||||
type='ImageToTensor',
|
||||
keys=[
|
||||
'img', 'threshold_map', 'threshold_mask', 'shrink_map',
|
||||
'shrink_mask'
|
||||
]),
|
||||
dict(
|
||||
type='Collect',
|
||||
keys=[
|
||||
'img', 'threshold_map', 'threshold_mask', 'shrink_map',
|
||||
'shrink_mask'
|
||||
]),
|
||||
]
|
||||
data = dict(
|
||||
train=dict(
|
||||
type='OCRDetDataset',
|
||||
data_source=dict(
|
||||
type='OCRDetSource',
|
||||
label_file=data_train_list,
|
||||
data_dir=SMALL_OCR_DET_DATA + '/img',
|
||||
),
|
||||
pipeline=pipeline))
|
||||
dataset = build_dataset(data['train'])
|
||||
|
||||
return dataset
|
||||
|
||||
def _get_dataset_pai(self):
|
||||
data_root = SMALL_OCR_DET_PAI_DATA
|
||||
data_train_list = os.path.join(data_root, 'label.csv')
|
||||
pipeline = [
|
||||
dict(
|
||||
type='IaaAugment',
|
||||
augmenter_args=[{
|
||||
'type': 'Fliplr',
|
||||
'args': {
|
||||
'p': 0.5
|
||||
}
|
||||
}, {
|
||||
'type': 'Affine',
|
||||
'args': {
|
||||
'rotate': [-10, 10]
|
||||
}
|
||||
}, {
|
||||
'type': 'Resize',
|
||||
'args': {
|
||||
'size': [0.5, 3]
|
||||
}
|
||||
}]),
|
||||
dict(
|
||||
type='EastRandomCropData',
|
||||
size=[640, 640],
|
||||
max_tries=50,
|
||||
keep_ratio=True),
|
||||
dict(
|
||||
type='MakeBorderMap',
|
||||
shrink_ratio=0.4,
|
||||
thresh_min=0.3,
|
||||
thresh_max=0.7),
|
||||
dict(type='MakeShrinkMap', shrink_ratio=0.4, min_text_size=8),
|
||||
dict(type='MMNormalize', **IMG_NORM_CFG),
|
||||
dict(
|
||||
type='ImageToTensor',
|
||||
keys=[
|
||||
'img', 'threshold_map', 'threshold_mask', 'shrink_map',
|
||||
'shrink_mask'
|
||||
]),
|
||||
dict(
|
||||
type='Collect',
|
||||
keys=[
|
||||
'img', 'threshold_map', 'threshold_mask', 'shrink_map',
|
||||
'shrink_mask'
|
||||
]),
|
||||
]
|
||||
data = dict(
|
||||
train=dict(
|
||||
type='OCRDetDataset',
|
||||
data_source=dict(
|
||||
type='OCRPaiDetSource',
|
||||
label_file=[data_train_list],
|
||||
data_dir=SMALL_OCR_DET_PAI_DATA + '/img',
|
||||
),
|
||||
pipeline=pipeline))
|
||||
dataset = build_dataset(data['train'])
|
||||
|
||||
return dataset
|
||||
|
||||
def test_default(self):
|
||||
dataset = self._get_dataset()
|
||||
for _, batch in enumerate(dataset):
|
||||
img, threshold_mask, shrink_mask = batch['img'], batch[
|
||||
'threshold_mask'], batch['shrink_mask']
|
||||
self.assertEqual(img.shape, torch.Size([3, 640, 640]))
|
||||
self.assertEqual(threshold_mask.shape, torch.Size([1, 640, 640]))
|
||||
self.assertEqual(shrink_mask.shape, torch.Size([1, 640, 640]))
|
||||
break
|
||||
|
||||
def test_pai(self):
|
||||
dataset = self._get_dataset_pai()
|
||||
for _, batch in enumerate(dataset):
|
||||
img, threshold_mask, shrink_mask = batch['img'], batch[
|
||||
'threshold_mask'], batch['shrink_mask']
|
||||
self.assertEqual(img.shape, torch.Size([3, 640, 640]))
|
||||
self.assertEqual(threshold_mask.shape, torch.Size([1, 640, 640]))
|
||||
self.assertEqual(shrink_mask.shape, torch.Size([1, 640, 640]))
|
||||
break
|
||||
|
||||
|
||||
if __name__ == '__main__':
|
||||
unittest.main()
|
|
@ -0,0 +1,66 @@
|
|||
# Copyright (c) Alibaba, Inc. and its affiliates.
|
||||
import os
|
||||
import unittest
|
||||
|
||||
import torch
|
||||
from tests.ut_config import SMALL_OCR_REC_DATA
|
||||
|
||||
from easycv.datasets.builder import build_dataset
|
||||
|
||||
|
||||
class OCRRecsDatasetTest(unittest.TestCase):
|
||||
|
||||
def setUp(self):
|
||||
print(('Testing %s.%s' % (type(self).__name__, self._testMethodName)))
|
||||
|
||||
def _get_dataset(self):
|
||||
data_root = SMALL_OCR_REC_DATA
|
||||
data_train_list = os.path.join(data_root, 'label.txt')
|
||||
pipeline = [
|
||||
dict(type='RecConAug', prob=0.5, image_shape=(48, 320, 3)),
|
||||
dict(type='RecAug'),
|
||||
dict(
|
||||
type='MultiLabelEncode',
|
||||
max_text_length=25,
|
||||
use_space_char=True,
|
||||
character_dict_path=
|
||||
'http://pai-vision-data-hz.oss-cn-zhangjiakou.aliyuncs.com/EasyCV/modelzoo/ocr/dict/ppocr_keys_v1.txt',
|
||||
),
|
||||
dict(type='RecResizeImg', image_shape=(3, 48, 320)),
|
||||
dict(type='MMToTensor'),
|
||||
dict(
|
||||
type='Collect',
|
||||
keys=[
|
||||
'img', 'label_ctc', 'label_sar', 'length', 'valid_ratio'
|
||||
],
|
||||
meta_keys=['img_path'])
|
||||
]
|
||||
data = dict(
|
||||
train=dict(
|
||||
type='OCRRecDataset',
|
||||
data_source=dict(
|
||||
type='OCRRecSource',
|
||||
label_file=data_train_list,
|
||||
data_dir=SMALL_OCR_REC_DATA + '/img',
|
||||
ext_data_num=0,
|
||||
test_mode=True,
|
||||
),
|
||||
pipeline=pipeline))
|
||||
dataset = build_dataset(data['train'])
|
||||
|
||||
return dataset
|
||||
|
||||
def test_default(self):
|
||||
dataset = self._get_dataset()
|
||||
for _, batch in enumerate(dataset):
|
||||
|
||||
img, label_ctc, label_sar = batch['img'], batch[
|
||||
'label_ctc'], batch['label_sar']
|
||||
self.assertEqual(img.shape, torch.Size([3, 48, 320]))
|
||||
self.assertEqual(label_ctc.shape, (25, ))
|
||||
self.assertEqual(label_sar.shape, (25, ))
|
||||
break
|
||||
|
||||
|
||||
if __name__ == '__main__':
|
||||
unittest.main()
|
|
@ -0,0 +1,55 @@
|
|||
# Copyright (c) Alibaba, Inc. and its affiliates.
|
||||
"""
|
||||
isort:skip_file
|
||||
"""
|
||||
import json
|
||||
import os
|
||||
import unittest
|
||||
|
||||
import cv2
|
||||
import torch
|
||||
|
||||
from easycv.predictors.ocr import OCRDetPredictor, OCRRecPredictor, OCRClsPredictor, OCRPredictor
|
||||
|
||||
from easycv.utils.test_util import get_tmp_dir
|
||||
from tests.ut_config import (PRETRAINED_MODEL_OCRDET, PRETRAINED_MODEL_OCRREC,
|
||||
PRETRAINED_MODEL_OCRCLS, TEST_IMAGES_DIR)
|
||||
|
||||
|
||||
class TorchOCRTest(unittest.TestCase):
|
||||
|
||||
def test_ocr_det(self):
|
||||
predictor = OCRDetPredictor(PRETRAINED_MODEL_OCRDET)
|
||||
img = cv2.imread(os.path.join(TEST_IMAGES_DIR, 'ocr_det.jpg'))
|
||||
dt_boxes = predictor([img])[0]
|
||||
self.assertEqual(dt_boxes['points'].shape[0], 16) # 16 boxes
|
||||
|
||||
def test_ocr_rec(self):
|
||||
predictor = OCRRecPredictor(PRETRAINED_MODEL_OCRREC)
|
||||
img = cv2.imread(os.path.join(TEST_IMAGES_DIR, 'ocr_rec.jpg'))
|
||||
rec_out = predictor([img])[0]
|
||||
self.assertEqual(rec_out['preds_text'][0], '韩国小馆') # 韩国小馆
|
||||
self.assertGreater(rec_out['preds_text'][1],
|
||||
0.9944) # 0.9944670796394348
|
||||
|
||||
def test_ocr_direction(self):
|
||||
predictor = OCRClsPredictor(PRETRAINED_MODEL_OCRCLS)
|
||||
img = cv2.imread(os.path.join(TEST_IMAGES_DIR, 'ocr_rec.jpg'))
|
||||
cls_out = predictor([img])[0]
|
||||
self.assertEqual(int(cls_out['class']), 0)
|
||||
self.assertGreater(float(cls_out['neck'][0]), 0.9998) # 0.99987
|
||||
|
||||
def test_ocr_end2end(self):
|
||||
predictor = OCRPredictor(
|
||||
det_model_path=PRETRAINED_MODEL_OCRDET,
|
||||
rec_model_path=PRETRAINED_MODEL_OCRREC,
|
||||
cls_model_path=PRETRAINED_MODEL_OCRCLS,
|
||||
use_angle_cls=True)
|
||||
img = cv2.imread(os.path.join(TEST_IMAGES_DIR, 'ocr_det.jpg'))
|
||||
filter_boxes, filter_rec_res = predictor([img])
|
||||
self.assertEqual(filter_rec_res[0][0][0], '纯臻营养护发素')
|
||||
self.assertGreater(filter_rec_res[0][0][1], 0.91)
|
||||
|
||||
|
||||
if __name__ == '__main__':
|
||||
unittest.main()
|
|
@ -73,6 +73,13 @@ COMPRESSION_TEST_DATA = os.path.join(BASE_LOCAL_PATH,
|
|||
SEG_DATA_SMALL_RAW_LOCAL = os.path.join(BASE_LOCAL_PATH,
|
||||
'data/segmentation/small_voc_200')
|
||||
|
||||
# OCR data
|
||||
SMALL_OCR_CLS_DATA = os.path.join(BASE_LOCAL_PATH, 'data/ocr/small_ocr_cls')
|
||||
SMALL_OCR_DET_DATA = os.path.join(BASE_LOCAL_PATH, 'data/ocr/small_ocr_det')
|
||||
SMALL_OCR_DET_PAI_DATA = os.path.join(BASE_LOCAL_PATH,
|
||||
'data/ocr/small_ocr_det_pai')
|
||||
SMALL_OCR_REC_DATA = os.path.join(BASE_LOCAL_PATH, 'data/ocr/small_ocr_rec')
|
||||
|
||||
PRETRAINED_MODEL_MOCO = os.path.join(
|
||||
BASE_LOCAL_PATH, 'pretrained_models/selfsup/moco/moco_epoch_200.pth')
|
||||
PRETRAINED_MODEL_RESNET50 = os.path.join(
|
||||
|
@ -124,6 +131,13 @@ PRETRAINED_MODEL_MASK2FORMER_DIR = os.path.join(
|
|||
BASE_LOCAL_PATH, 'pretrained_models/segmentation/mask2former/')
|
||||
PRETRAINED_MODEL_MASK2FORMER = os.path.join(PRETRAINED_MODEL_MASK2FORMER_DIR,
|
||||
'mask2former_r50_instance.pth')
|
||||
PRETRAINED_MODEL_OCRDET = os.path.join(
|
||||
BASE_LOCAL_PATH, 'pretrained_models/ocr/det/student_export.pth')
|
||||
PRETRAINED_MODEL_OCRREC = os.path.join(
|
||||
BASE_LOCAL_PATH,
|
||||
'pretrained_models/ocr/rec/best_accuracy_student_export.pth')
|
||||
PRETRAINED_MODEL_OCRCLS = os.path.join(
|
||||
BASE_LOCAL_PATH, 'pretrained_models/ocr/cls/best_accuracy_export.pth')
|
||||
PRETRAINED_MODEL_SEGFORMER = os.path.join(
|
||||
BASE_LOCAL_PATH,
|
||||
'pretrained_models/segmentation/segformer/segformer_b0/SegmentationEvaluator_mIoU_best.pth'
|
||||
|
|
Loading…
Reference in New Issue