fix conflict
commit
5016894e7b
applications
configs/cls
ch_PP-OCRv3
ppocr
modeling
ppstructure/table
test_tipc
configs
ch_PP-OCRv2
ch_PP-OCRv2_det
ch_PP-OCRv2_rec
ch_PP-OCRv3
ch_PP-OCRv3_det
|
@ -0,0 +1,648 @@
|
|||
# 基于PP-OCRv3的PCB字符识别
|
||||
|
||||
- [1. 项目介绍](#1-项目介绍)
|
||||
- [2. 安装说明](#2-安装说明)
|
||||
- [3. 数据准备](#3-数据准备)
|
||||
- [4. 文本检测](#4-文本检测)
|
||||
- [4.1 预训练模型直接评估](#41-预训练模型直接评估)
|
||||
- [4.2 预训练模型+验证集padding直接评估](#42-预训练模型验证集padding直接评估)
|
||||
- [4.3 预训练模型+fine-tune](#43-预训练模型fine-tune)
|
||||
- [5. 文本识别](#5-文本识别)
|
||||
- [5.1 预训练模型直接评估](#51-预训练模型直接评估)
|
||||
- [5.2 三种fine-tune方案](#52-三种fine-tune方案)
|
||||
- [6. 模型导出](#6-模型导出)
|
||||
- [7. 端对端评测](#7-端对端评测)
|
||||
- [8. Jetson部署](#8-Jetson部署)
|
||||
- [9. 总结](#9-总结)
|
||||
- [更多资源](#更多资源)
|
||||
|
||||
# 1. 项目介绍
|
||||
|
||||
印刷电路板(PCB)是电子产品中的核心器件,对于板件质量的测试与监控是生产中必不可少的环节。在一些场景中,通过PCB中信号灯颜色和文字组合可以定位PCB局部模块质量问题,PCB文字识别中存在如下难点:
|
||||
|
||||
- 裁剪出的PCB图片宽高比例较小
|
||||
- 文字区域整体面积也较小
|
||||
- 包含垂直、水平多种方向文本
|
||||
|
||||
针对本场景,PaddleOCR基于全新的PP-OCRv3通过合成数据、微调以及其他场景适配方法完成小字符文本识别任务,满足企业上线要求。PCB检测、识别效果如 **图1** 所示:
|
||||
|
||||
<div align=center><img src='https://ai-studio-static-online.cdn.bcebos.com/95d8e95bf1ab476987f2519c0f8f0c60a0cdc2c444804ed6ab08f2f7ab054880', width='500'></div>
|
||||
<div align=center>图1 PCB检测识别效果</div>
|
||||
|
||||
注:欢迎在AIStudio领取免费算力体验线上实训,项目链接: [基于PP-OCRv3实现PCB字符识别](https://aistudio.baidu.com/aistudio/projectdetail/4008973)
|
||||
|
||||
# 2. 安装说明
|
||||
|
||||
|
||||
下载PaddleOCR源码,安装依赖环境。
|
||||
|
||||
|
||||
```python
|
||||
# 如仍需安装or安装更新,可以执行以下步骤
|
||||
git clone https://github.com/PaddlePaddle/PaddleOCR.git
|
||||
# git clone https://gitee.com/PaddlePaddle/PaddleOCR
|
||||
```
|
||||
|
||||
|
||||
```python
|
||||
# 安装依赖包
|
||||
pip install -r /home/aistudio/PaddleOCR/requirements.txt
|
||||
```
|
||||
|
||||
# 3. 数据准备
|
||||
|
||||
我们通过图片合成工具生成 **图2** 所示的PCB图片,整图只有高25、宽150左右、文字区域高9、宽45左右,包含垂直和水平2种方向的文本:
|
||||
|
||||
<div align=center><img src="https://ai-studio-static-online.cdn.bcebos.com/bb7a345687814a3d83a29790f2a2b7d081495b3a920b43988c93da6039cad653" width="1000" ></div>
|
||||
<div align=center>图2 数据集示例</div>
|
||||
|
||||
暂时不开源生成的PCB数据集,但是通过更换背景,通过如下代码生成数据即可:
|
||||
|
||||
```
|
||||
cd gen_data
|
||||
python3 gen.py --num_img=10
|
||||
```
|
||||
|
||||
生成图片参数解释:
|
||||
|
||||
```
|
||||
num_img:生成图片数量
|
||||
font_min_size、font_max_size:字体最大、最小尺寸
|
||||
bg_path:文字区域背景存放路径
|
||||
det_bg_path:整图背景存放路径
|
||||
fonts_path:字体路径
|
||||
corpus_path:语料路径
|
||||
output_dir:生成图片存储路径
|
||||
```
|
||||
|
||||
这里生成 **100张** 相同尺寸和文本的图片,如 **图3** 所示,方便大家跑通实验。通过如下代码解压数据集:
|
||||
|
||||
<div align=center><img src="https://ai-studio-static-online.cdn.bcebos.com/3277b750159f4b68b2b58506bfec9005d49aeb5fb1d9411e83f96f9ff7eb66a5" width="1000" ></div>
|
||||
<div align=center>图3 案例提供数据集示例</div>
|
||||
|
||||
|
||||
```python
|
||||
tar xf ./data/data148165/dataset.tar -C ./
|
||||
```
|
||||
|
||||
在生成数据集的时需要生成检测和识别训练需求的格式:
|
||||
|
||||
|
||||
- **文本检测**
|
||||
|
||||
标注文件格式如下,中间用'\t'分隔:
|
||||
|
||||
```
|
||||
" 图像文件名 json.dumps编码的图像标注信息"
|
||||
ch4_test_images/img_61.jpg [{"transcription": "MASA", "points": [[310, 104], [416, 141], [418, 216], [312, 179]]}, {...}]
|
||||
```
|
||||
|
||||
json.dumps编码前的图像标注信息是包含多个字典的list,字典中的 `points` 表示文本框的四个点的坐标(x, y),从左上角的点开始顺时针排列。 `transcription` 表示当前文本框的文字,***当其内容为“###”时,表示该文本框无效,在训练时会跳过。***
|
||||
|
||||
- **文本识别**
|
||||
|
||||
标注文件的格式如下, txt文件中默认请将图片路径和图片标签用'\t'分割,如用其他方式分割将造成训练报错。
|
||||
|
||||
```
|
||||
" 图像文件名 图像标注信息 "
|
||||
|
||||
train_data/rec/train/word_001.jpg 简单可依赖
|
||||
train_data/rec/train/word_002.jpg 用科技让复杂的世界更简单
|
||||
...
|
||||
```
|
||||
|
||||
|
||||
# 4. 文本检测
|
||||
|
||||
选用飞桨OCR开发套件[PaddleOCR](https://github.com/PaddlePaddle/PaddleOCR)中的PP-OCRv3模型进行文本检测和识别。针对检测模型和识别模型,进行了共计9个方面的升级:
|
||||
|
||||
- PP-OCRv3检测模型对PP-OCRv2中的CML协同互学习文本检测蒸馏策略进行了升级,分别针对教师模型和学生模型进行进一步效果优化。其中,在对教师模型优化时,提出了大感受野的PAN结构LK-PAN和引入了DML蒸馏策略;在对学生模型优化时,提出了残差注意力机制的FPN结构RSE-FPN。
|
||||
|
||||
- PP-OCRv3的识别模块是基于文本识别算法SVTR优化。SVTR不再采用RNN结构,通过引入Transformers结构更加有效地挖掘文本行图像的上下文信息,从而提升文本识别能力。PP-OCRv3通过轻量级文本识别网络SVTR_LCNet、Attention损失指导CTC损失训练策略、挖掘文字上下文信息的数据增广策略TextConAug、TextRotNet自监督预训练模型、UDML联合互学习策略、UIM无标注数据挖掘方案,6个方面进行模型加速和效果提升。
|
||||
|
||||
更多细节请参考PP-OCRv3[技术报告](https://github.com/PaddlePaddle/PaddleOCR/blob/release/2.5/doc/doc_ch/PP-OCRv3_introduction.md)。
|
||||
|
||||
|
||||
我们使用 **3种方案** 进行检测模型的训练、评估:
|
||||
- **PP-OCRv3英文超轻量检测预训练模型直接评估**
|
||||
- PP-OCRv3英文超轻量检测预训练模型 + **验证集padding**直接评估
|
||||
- PP-OCRv3英文超轻量检测预训练模型 + **fine-tune**
|
||||
|
||||
## **4.1 预训练模型直接评估**
|
||||
|
||||
我们首先通过PaddleOCR提供的预训练模型在验证集上进行评估,如果评估指标能满足效果,可以直接使用预训练模型,不再需要训练。
|
||||
|
||||
使用预训练模型直接评估步骤如下:
|
||||
|
||||
**1)下载预训练模型**
|
||||
|
||||
|
||||
PaddleOCR已经提供了PP-OCR系列模型,部分模型展示如下表所示:
|
||||
|
||||
| 模型简介 | 模型名称 | 推荐场景 | 检测模型 | 方向分类器 | 识别模型 |
|
||||
| ------------------------------------- | ----------------------- | --------------- | ------------------------------------------------------------ | ------------------------------------------------------------ | ------------------------------------------------------------ |
|
||||
| 中英文超轻量PP-OCRv3模型(16.2M) | ch_PP-OCRv3_xx | 移动端&服务器端 | [推理模型](https://paddleocr.bj.bcebos.com/PP-OCRv3/chinese/ch_PP-OCRv3_det_infer.tar) / [训练模型](https://paddleocr.bj.bcebos.com/PP-OCRv3/chinese/ch_PP-OCRv3_det_distill_train.tar) | [推理模型](https://paddleocr.bj.bcebos.com/dygraph_v2.0/ch/ch_ppocr_mobile_v2.0_cls_infer.tar) / [训练模型](https://paddleocr.bj.bcebos.com/dygraph_v2.0/ch/ch_ppocr_mobile_v2.0_cls_train.tar) | [推理模型](https://paddleocr.bj.bcebos.com/PP-OCRv3/chinese/ch_PP-OCRv3_rec_infer.tar) / [训练模型](https://paddleocr.bj.bcebos.com/PP-OCRv3/chinese/ch_PP-OCRv3_rec_train.tar) |
|
||||
| 英文超轻量PP-OCRv3模型(13.4M) | en_PP-OCRv3_xx | 移动端&服务器端 | [推理模型](https://paddleocr.bj.bcebos.com/PP-OCRv3/english/en_PP-OCRv3_det_infer.tar) / [训练模型](https://paddleocr.bj.bcebos.com/PP-OCRv3/english/en_PP-OCRv3_det_distill_train.tar) | [推理模型](https://paddleocr.bj.bcebos.com/dygraph_v2.0/ch/ch_ppocr_mobile_v2.0_cls_infer.tar) / [训练模型](https://paddleocr.bj.bcebos.com/dygraph_v2.0/ch/ch_ppocr_mobile_v2.0_cls_train.tar) | [推理模型](https://paddleocr.bj.bcebos.com/PP-OCRv3/english/en_PP-OCRv3_rec_infer.tar) / [训练模型](https://paddleocr.bj.bcebos.com/PP-OCRv3/english/en_PP-OCRv3_rec_train.tar) |
|
||||
| 中英文超轻量PP-OCRv2模型(13.0M) | ch_PP-OCRv2_xx | 移动端&服务器端 | [推理模型](https://paddleocr.bj.bcebos.com/PP-OCRv2/chinese/ch_PP-OCRv2_det_infer.tar) / [训练模型](https://paddleocr.bj.bcebos.com/PP-OCRv2/chinese/ch_PP-OCRv2_det_distill_train.tar) | [推理模型](https://paddleocr.bj.bcebos.com/dygraph_v2.0/ch/ch_ppocr_mobile_v2.0_cls_infer.tar) / [预训练模型](https://paddleocr.bj.bcebos.com/dygraph_v2.0/ch/ch_ppocr_mobile_v2.0_cls_train.tar) | [推理模型](https://paddleocr.bj.bcebos.com/PP-OCRv2/chinese/ch_PP-OCRv2_rec_infer.tar) / [训练模型](https://paddleocr.bj.bcebos.com/PP-OCRv2/chinese/ch_PP-OCRv2_rec_train.tar) |
|
||||
| 中英文超轻量PP-OCR mobile模型(9.4M) | ch_ppocr_mobile_v2.0_xx | 移动端&服务器端 | [推理模型](https://paddleocr.bj.bcebos.com/dygraph_v2.0/ch/ch_ppocr_mobile_v2.0_det_infer.tar) / [预训练模型](https://paddleocr.bj.bcebos.com/dygraph_v2.0/ch/ch_ppocr_mobile_v2.0_det_train.tar) | [推理模型](https://paddleocr.bj.bcebos.com/dygraph_v2.0/ch/ch_ppocr_mobile_v2.0_cls_infer.tar) / [预训练模型](https://paddleocr.bj.bcebos.com/dygraph_v2.0/ch/ch_ppocr_mobile_v2.0_cls_train.tar) | [推理模型](https://paddleocr.bj.bcebos.com/dygraph_v2.0/ch/ch_ppocr_mobile_v2.0_rec_infer.tar) / [预训练模型](https://paddleocr.bj.bcebos.com/dygraph_v2.0/ch/ch_ppocr_mobile_v2.0_rec_pre.tar) |
|
||||
| 中英文通用PP-OCR server模型(143.4M) | ch_ppocr_server_v2.0_xx | 服务器端 | [推理模型](https://paddleocr.bj.bcebos.com/dygraph_v2.0/ch/ch_ppocr_server_v2.0_det_infer.tar) / [预训练模型](https://paddleocr.bj.bcebos.com/dygraph_v2.0/ch/ch_ppocr_server_v2.0_det_train.tar) | [推理模型](https://paddleocr.bj.bcebos.com/dygraph_v2.0/ch/ch_ppocr_mobile_v2.0_cls_infer.tar) / [预训练模型](https://paddleocr.bj.bcebos.com/dygraph_v2.0/ch/ch_ppocr_mobile_v2.0_cls_train.tar) | [推理模型](https://paddleocr.bj.bcebos.com/dygraph_v2.0/ch/ch_ppocr_server_v2.0_rec_infer.tar) / [预训练模型](https://paddleocr.bj.bcebos.com/dygraph_v2.0/ch/ch_ppocr_server_v2.0_rec_pre.tar) |
|
||||
|
||||
更多模型下载(包括多语言),可以参[考PP-OCR系列模型下载](https://github.com/PaddlePaddle/PaddleOCR/blob/release/2.5/doc/doc_ch/models_list.md)
|
||||
|
||||
这里我们使用PP-OCRv3英文超轻量检测模型,下载并解压预训练模型:
|
||||
|
||||
|
||||
|
||||
|
||||
```python
|
||||
# 如果更换其他模型,更新下载链接和解压指令就可以
|
||||
cd /home/aistudio/PaddleOCR
|
||||
mkdir pretrain_models
|
||||
cd pretrain_models
|
||||
# 下载英文预训练模型
|
||||
wget https://paddleocr.bj.bcebos.com/PP-OCRv3/english/en_PP-OCRv3_det_distill_train.tar
|
||||
tar xf en_PP-OCRv3_det_distill_train.tar && rm -rf en_PP-OCRv3_det_distill_train.tar
|
||||
%cd ..
|
||||
```
|
||||
|
||||
**模型评估**
|
||||
|
||||
|
||||
首先修改配置文件`configs/det/ch_PP-OCRv3/ch_PP-OCRv3_det_cml.yml`中的以下字段:
|
||||
```
|
||||
Eval.dataset.data_dir:指向验证集图片存放目录,'/home/aistudio/dataset'
|
||||
Eval.dataset.label_file_list:指向验证集标注文件,'/home/aistudio/dataset/det_gt_val.txt'
|
||||
Eval.dataset.transforms.DetResizeForTest: 尺寸
|
||||
limit_side_len: 48
|
||||
limit_type: 'min'
|
||||
```
|
||||
|
||||
然后在验证集上进行评估,具体代码如下:
|
||||
|
||||
|
||||
|
||||
```python
|
||||
cd /home/aistudio/PaddleOCR
|
||||
python tools/eval.py \
|
||||
-c configs/det/ch_PP-OCRv3/ch_PP-OCRv3_det_cml.yml \
|
||||
-o Global.checkpoints="./pretrain_models/en_PP-OCRv3_det_distill_train/best_accuracy"
|
||||
```
|
||||
|
||||
## **4.2 预训练模型+验证集padding直接评估**
|
||||
|
||||
考虑到PCB图片比较小,宽度只有25左右、高度只有140-170左右,我们在原图的基础上进行padding,再进行检测评估,padding前后效果对比如 **图4** 所示:
|
||||
|
||||
<div align=center><img src='https://ai-studio-static-online.cdn.bcebos.com/e61e6ba685534eda992cea30a63a9c461646040ffd0c4d208a5eebb85897dcf7' width='600'></div>
|
||||
<div align=center>图4 padding前后对比图</div>
|
||||
|
||||
将图片都padding到300*300大小,因为坐标信息发生了变化,我们同时要修改标注文件,在`/home/aistudio/dataset`目录里也提供了padding之后的图片,大家也可以尝试训练和评估:
|
||||
|
||||
同上,我们需要修改配置文件`configs/det/ch_PP-OCRv3/ch_PP-OCRv3_det_cml.yml`中的以下字段:
|
||||
```
|
||||
Eval.dataset.data_dir:指向验证集图片存放目录,'/home/aistudio/dataset'
|
||||
Eval.dataset.label_file_list:指向验证集标注文件,/home/aistudio/dataset/det_gt_padding_val.txt
|
||||
Eval.dataset.transforms.DetResizeForTest: 尺寸
|
||||
limit_side_len: 1100
|
||||
limit_type: 'min'
|
||||
```
|
||||
|
||||
然后执行评估代码
|
||||
|
||||
|
||||
```python
|
||||
cd /home/aistudio/PaddleOCR
|
||||
python tools/eval.py \
|
||||
-c configs/det/ch_PP-OCRv3/ch_PP-OCRv3_det_cml.yml \
|
||||
-o Global.checkpoints="./pretrain_models/en_PP-OCRv3_det_distill_train/best_accuracy"
|
||||
```
|
||||
|
||||
## **4.3 预训练模型+fine-tune**
|
||||
|
||||
|
||||
基于预训练模型,在生成的1500图片上进行fine-tune训练和评估,其中train数据1200张,val数据300张,修改配置文件`configs/det/ch_PP-OCRv3/ch_PP-OCRv3_det_student.yml`中的以下字段:
|
||||
```
|
||||
Global.epoch_num: 这里设置为1,方便快速跑通,实际中根据数据量调整该值
|
||||
Global.save_model_dir:模型保存路径
|
||||
Global.pretrained_model:指向预训练模型路径,'./pretrain_models/en_PP-OCRv3_det_distill_train/student.pdparams'
|
||||
Optimizer.lr.learning_rate:调整学习率,本实验设置为0.0005
|
||||
Train.dataset.data_dir:指向训练集图片存放目录,'/home/aistudio/dataset'
|
||||
Train.dataset.label_file_list:指向训练集标注文件,'/home/aistudio/dataset/det_gt_train.txt'
|
||||
Train.dataset.transforms.EastRandomCropData.size:训练尺寸改为[480,64]
|
||||
Eval.dataset.data_dir:指向验证集图片存放目录,'/home/aistudio/dataset/'
|
||||
Eval.dataset.label_file_list:指向验证集标注文件,'/home/aistudio/dataset/det_gt_val.txt'
|
||||
Eval.dataset.transforms.DetResizeForTest:评估尺寸,添加如下参数
|
||||
limit_side_len: 64
|
||||
limit_type:'min'
|
||||
```
|
||||
执行下面命令启动训练:
|
||||
|
||||
|
||||
```python
|
||||
cd /home/aistudio/PaddleOCR/
|
||||
python tools/train.py \
|
||||
-c configs/det/ch_PP-OCRv3/ch_PP-OCRv3_det_student.yml
|
||||
```
|
||||
|
||||
**模型评估**
|
||||
|
||||
|
||||
使用训练好的模型进行评估,更新模型路径`Global.checkpoints`:
|
||||
|
||||
|
||||
```python
|
||||
cd /home/aistudio/PaddleOCR/
|
||||
python3 tools/eval.py \
|
||||
-c configs/det/ch_PP-OCRv3/ch_PP-OCRv3_det_student.yml \
|
||||
-o Global.checkpoints="./output/ch_PP-OCR_V3_det/latest"
|
||||
```
|
||||
|
||||
使用训练好的模型进行评估,指标如下所示:
|
||||
|
||||
|
||||
| 序号 | 方案 | hmean | 效果提升 | 实验分析 |
|
||||
| -------- | -------- | -------- | -------- | -------- |
|
||||
| 1 | PP-OCRv3英文超轻量检测预训练模型 | 64.64% | - | 提供的预训练模型具有泛化能力 |
|
||||
| 2 | PP-OCRv3英文超轻量检测预训练模型 + 验证集padding | 72.13% |+7.5% | padding可以提升尺寸较小图片的检测效果|
|
||||
| 3 | PP-OCRv3英文超轻量检测预训练模型 + fine-tune | 100% | +27.9% | fine-tune会提升垂类场景效果 |
|
||||
|
||||
|
||||
```
|
||||
注:上述实验结果均是在1500张图片(1200张训练集,300张测试集)上训练、评估的得到,AIstudio只提供了100张数据,所以指标有所差异属于正常,只要策略有效、规律相同即可。
|
||||
```
|
||||
|
||||
# 5. 文本识别
|
||||
|
||||
我们分别使用如下4种方案进行训练、评估:
|
||||
|
||||
- **方案1**:**PP-OCRv3中英文超轻量识别预训练模型直接评估**
|
||||
- **方案2**:PP-OCRv3中英文超轻量检测预训练模型 + **fine-tune**
|
||||
- **方案3**:PP-OCRv3中英文超轻量检测预训练模型 + fine-tune + **公开通用识别数据集**
|
||||
- **方案4**:PP-OCRv3中英文超轻量检测预训练模型 + fine-tune + **增加PCB图像数量**
|
||||
|
||||
|
||||
## **5.1 预训练模型直接评估**
|
||||
|
||||
同检测模型,我们首先使用PaddleOCR提供的识别预训练模型在PCB验证集上进行评估。
|
||||
|
||||
使用预训练模型直接评估步骤如下:
|
||||
|
||||
**1)下载预训练模型**
|
||||
|
||||
|
||||
我们使用PP-OCRv3中英文超轻量文本识别模型,下载并解压预训练模型:
|
||||
|
||||
|
||||
```python
|
||||
# 如果更换其他模型,更新下载链接和解压指令就可以
|
||||
cd /home/aistudio/PaddleOCR/pretrain_models/
|
||||
wget https://paddleocr.bj.bcebos.com/PP-OCRv3/chinese/ch_PP-OCRv3_rec_train.tar
|
||||
tar xf ch_PP-OCRv3_rec_train.tar && rm -rf ch_PP-OCRv3_rec_train.tar
|
||||
cd ..
|
||||
```
|
||||
|
||||
**模型评估**
|
||||
|
||||
|
||||
首先修改配置文件`configs/det/ch_PP-OCRv3/ch_PP-OCRv2_rec_distillation.yml`中的以下字段:
|
||||
|
||||
```
|
||||
Metric.ignore_space: True:忽略空格
|
||||
Eval.dataset.data_dir:指向验证集图片存放目录,'/home/aistudio/dataset'
|
||||
Eval.dataset.label_file_list:指向验证集标注文件,'/home/aistudio/dataset/rec_gt_val.txt'
|
||||
```
|
||||
|
||||
我们使用下载的预训练模型进行评估:
|
||||
|
||||
|
||||
```python
|
||||
cd /home/aistudio/PaddleOCR
|
||||
python3 tools/eval.py \
|
||||
-c configs/rec/PP-OCRv3/ch_PP-OCRv3_rec_distillation.yml \
|
||||
-o Global.checkpoints=pretrain_models/ch_PP-OCRv3_rec_train/best_accuracy
|
||||
|
||||
```
|
||||
|
||||
## **5.2 三种fine-tune方案**
|
||||
|
||||
方案2、3、4训练和评估方式是相同的,因此在我们了解每个技术方案之后,再具体看修改哪些参数是相同,哪些是不同的。
|
||||
|
||||
**方案介绍:**
|
||||
|
||||
1) **方案2**:预训练模型 + **fine-tune**
|
||||
|
||||
- 在预训练模型的基础上进行fine-tune,使用1500张PCB进行训练和评估,其中训练集1200张,验证集300张。
|
||||
|
||||
|
||||
2) **方案3**:预训练模型 + fine-tune + **公开通用识别数据集**
|
||||
|
||||
- 当识别数据比较少的情况,可以考虑添加公开通用识别数据集。在方案2的基础上,添加公开通用识别数据集,如lsvt、rctw等。
|
||||
|
||||
3)**方案4**:预训练模型 + fine-tune + **增加PCB图像数量**
|
||||
|
||||
- 如果能够获取足够多真实场景,我们可以通过增加数据量提升模型效果。在方案2的基础上,增加PCB的数量到2W张左右。
|
||||
|
||||
|
||||
**参数修改:**
|
||||
|
||||
接着我们看需要修改的参数,以上方案均需要修改配置文件`configs/rec/PP-OCRv3/ch_PP-OCRv3_rec.yml`的参数,**修改一次即可**:
|
||||
|
||||
```
|
||||
Global.pretrained_model:指向预训练模型路径,'pretrain_models/ch_PP-OCRv3_rec_train/best_accuracy'
|
||||
Optimizer.lr.values:学习率,本实验设置为0.0005
|
||||
Train.loader.batch_size_per_card: batch size,默认128,因为数据量小于128,因此我们设置为8,数据量大可以按默认的训练
|
||||
Eval.loader.batch_size_per_card: batch size,默认128,设置为4
|
||||
Metric.ignore_space: 忽略空格,本实验设置为True
|
||||
```
|
||||
|
||||
**更换不同的方案**每次需要修改的参数:
|
||||
```
|
||||
Global.epoch_num: 这里设置为1,方便快速跑通,实际中根据数据量调整该值
|
||||
Global.save_model_dir:指向模型保存路径
|
||||
Train.dataset.data_dir:指向训练集图片存放目录
|
||||
Train.dataset.label_file_list:指向训练集标注文件
|
||||
Eval.dataset.data_dir:指向验证集图片存放目录
|
||||
Eval.dataset.label_file_list:指向验证集标注文件
|
||||
```
|
||||
|
||||
同时**方案3**修改以下参数
|
||||
```
|
||||
Eval.dataset.label_file_list:添加公开通用识别数据标注文件
|
||||
Eval.dataset.ratio_list:数据和公开通用识别数据每次采样比例,按实际修改即可
|
||||
```
|
||||
如 **图5** 所示:
|
||||
<div align=center><img src='https://ai-studio-static-online.cdn.bcebos.com/0fa18b25819042d9bbf3397c3af0e21433b23d52f7a84b0a8681b8e6a308d433' wdith=''></div>
|
||||
<div align=center>图5 添加公开通用识别数据配置文件示例</div>
|
||||
|
||||
|
||||
我们提取Student模型的参数,在PCB数据集上进行fine-tune,可以参考如下代码:
|
||||
|
||||
|
||||
```python
|
||||
import paddle
|
||||
# 加载预训练模型
|
||||
all_params = paddle.load("./pretrain_models/ch_PP-OCRv3_rec_train/best_accuracy.pdparams")
|
||||
# 查看权重参数的keys
|
||||
print(all_params.keys())
|
||||
# 学生模型的权重提取
|
||||
s_params = {key[len("student_model."):]: all_params[key] for key in all_params if "student_model." in key}
|
||||
# 查看学生模型权重参数的keys
|
||||
print(s_params.keys())
|
||||
# 保存
|
||||
paddle.save(s_params, "./pretrain_models/ch_PP-OCRv3_rec_train/student.pdparams")
|
||||
```
|
||||
|
||||
修改参数后,**每个方案**都执行如下命令启动训练:
|
||||
|
||||
|
||||
|
||||
```python
|
||||
cd /home/aistudio/PaddleOCR/
|
||||
python3 tools/train.py -c configs/rec/PP-OCRv3/ch_PP-OCRv3_rec.yml
|
||||
```
|
||||
|
||||
|
||||
使用训练好的模型进行评估,更新模型路径`Global.checkpoints`:
|
||||
|
||||
|
||||
```python
|
||||
cd /home/aistudio/PaddleOCR/
|
||||
python3 tools/eval.py \
|
||||
-c configs/rec/PP-OCRv3/ch_PP-OCRv3_rec.yml \
|
||||
-o Global.checkpoints=./output/rec_ppocr_v3/latest
|
||||
```
|
||||
|
||||
所有方案评估指标如下:
|
||||
|
||||
| 序号 | 方案 | acc | 效果提升 | 实验分析 |
|
||||
| -------- | -------- | -------- | -------- | -------- |
|
||||
| 1 | PP-OCRv3中英文超轻量识别预训练模型直接评估 | 46.67% | - | 提供的预训练模型具有泛化能力 |
|
||||
| 2 | PP-OCRv3中英文超轻量识别预训练模型 + fine-tune | 42.02% |-4.6% | 在数据量不足的情况,反而比预训练模型效果低(也可以通过调整超参数再试试)|
|
||||
| 3 | PP-OCRv3中英文超轻量识别预训练模型 + fine-tune + 公开通用识别数据集 | 77% | +30% | 在数据量不足的情况下,可以考虑补充公开数据训练 |
|
||||
| 4 | PP-OCRv3中英文超轻量识别预训练模型 + fine-tune + 增加PCB图像数量 | 99.99% | +23% | 如果能获取更多数据量的情况,可以通过增加数据量提升效果 |
|
||||
|
||||
```
|
||||
注:上述实验结果均是在1500张图片(1200张训练集,300张测试集)、2W张图片、添加公开通用识别数据集上训练、评估的得到,AIstudio只提供了100张数据,所以指标有所差异属于正常,只要策略有效、规律相同即可。
|
||||
```
|
||||
|
||||
# 6. 模型导出
|
||||
|
||||
inference 模型(paddle.jit.save保存的模型) 一般是模型训练,把模型结构和模型参数保存在文件中的固化模型,多用于预测部署场景。 训练过程中保存的模型是checkpoints模型,保存的只有模型的参数,多用于恢复训练等。 与checkpoints模型相比,inference 模型会额外保存模型的结构信息,在预测部署、加速推理上性能优越,灵活方便,适合于实际系统集成。
|
||||
|
||||
|
||||
```python
|
||||
# 导出检测模型
|
||||
python3 tools/export_model.py \
|
||||
-c configs/det/ch_PP-OCRv3/ch_PP-OCRv3_det_student.yml \
|
||||
-o Global.pretrained_model="./output/ch_PP-OCR_V3_det/latest" \
|
||||
Global.save_inference_dir="./inference_model/ch_PP-OCR_V3_det/"
|
||||
```
|
||||
|
||||
因为上述模型只训练了1个epoch,因此我们使用训练最优的模型进行预测,存储在`/home/aistudio/best_models/`目录下,解压即可
|
||||
|
||||
|
||||
```python
|
||||
cd /home/aistudio/best_models/
|
||||
wget https://paddleocr.bj.bcebos.com/fanliku/PCB/det_ppocr_v3_en_infer_PCB.tar
|
||||
tar xf /home/aistudio/best_models/det_ppocr_v3_en_infer_PCB.tar -C /home/aistudio/PaddleOCR/pretrain_models/
|
||||
```
|
||||
|
||||
|
||||
```python
|
||||
# 检测模型inference模型预测
|
||||
cd /home/aistudio/PaddleOCR/
|
||||
python3 tools/infer/predict_det.py \
|
||||
--image_dir="/home/aistudio/dataset/imgs/0000.jpg" \
|
||||
--det_algorithm="DB" \
|
||||
--det_model_dir="./pretrain_models/det_ppocr_v3_en_infer_PCB/" \
|
||||
--det_limit_side_len=48 \
|
||||
--det_limit_type='min' \
|
||||
--det_db_unclip_ratio=2.5 \
|
||||
--use_gpu=True
|
||||
```
|
||||
|
||||
结果存储在`inference_results`目录下,检测如下图所示:
|
||||
<div align=center><img src='https://ai-studio-static-online.cdn.bcebos.com/5939ae15a1f0445aaeec15c68107dbd897740a1ddd284bf8b583bb6242099157' width=''></div>
|
||||
<div align=center>图6 检测结果</div>
|
||||
|
||||
|
||||
同理,导出识别模型并进行推理。
|
||||
|
||||
```python
|
||||
# 导出识别模型
|
||||
python3 tools/export_model.py \
|
||||
-c configs/rec/PP-OCRv3/ch_PP-OCRv3_rec.yml \
|
||||
-o Global.pretrained_model="./output/rec_ppocr_v3/latest" \
|
||||
Global.save_inference_dir="./inference_model/rec_ppocr_v3/"
|
||||
|
||||
```
|
||||
|
||||
同检测模型,识别模型也只训练了1个epoch,因此我们使用训练最优的模型进行预测,存储在`/home/aistudio/best_models/`目录下,解压即可
|
||||
|
||||
|
||||
```python
|
||||
cd /home/aistudio/best_models/
|
||||
wget https://paddleocr.bj.bcebos.com/fanliku/PCB/rec_ppocr_v3_ch_infer_PCB.tar
|
||||
tar xf /home/aistudio/best_models/rec_ppocr_v3_ch_infer_PCB.tar -C /home/aistudio/PaddleOCR/pretrain_models/
|
||||
```
|
||||
|
||||
|
||||
```python
|
||||
# 识别模型inference模型预测
|
||||
cd /home/aistudio/PaddleOCR/
|
||||
python3 tools/infer/predict_rec.py \
|
||||
--image_dir="../test_imgs/0000_rec.jpg" \
|
||||
--rec_model_dir="./pretrain_models/rec_ppocr_v3_ch_infer_PCB" \
|
||||
--rec_image_shape="3, 48, 320" \
|
||||
--use_space_char=False \
|
||||
--use_gpu=True
|
||||
```
|
||||
|
||||
```python
|
||||
# 检测+识别模型inference模型预测
|
||||
cd /home/aistudio/PaddleOCR/
|
||||
python3 tools/infer/predict_system.py \
|
||||
--image_dir="../test_imgs/0000.jpg" \
|
||||
--det_model_dir="./pretrain_models/det_ppocr_v3_en_infer_PCB" \
|
||||
--det_limit_side_len=48 \
|
||||
--det_limit_type='min' \
|
||||
--det_db_unclip_ratio=2.5 \
|
||||
--rec_model_dir="./pretrain_models/rec_ppocr_v3_ch_infer_PCB" \
|
||||
--rec_image_shape="3, 48, 320" \
|
||||
--draw_img_save_dir=./det_rec_infer/ \
|
||||
--use_space_char=False \
|
||||
--use_angle_cls=False \
|
||||
--use_gpu=True
|
||||
|
||||
```
|
||||
|
||||
端到端预测结果存储在`det_res_infer`文件夹内,结果如下图所示:
|
||||
<div align=center><img src='https://ai-studio-static-online.cdn.bcebos.com/c570f343c29846c792da56ebaca16c50708477514dd048cea8bef37ffa85d03f'></div>
|
||||
<div align=center>图7 检测+识别结果</div>
|
||||
|
||||
# 7. 端对端评测
|
||||
|
||||
接下来介绍文本检测+文本识别的端对端指标评估方式。主要分为三步:
|
||||
|
||||
1)首先运行`tools/infer/predict_system.py`,将`image_dir`改为需要评估的数据文件家,得到保存的结果:
|
||||
|
||||
|
||||
```python
|
||||
# 检测+识别模型inference模型预测
|
||||
python3 tools/infer/predict_system.py \
|
||||
--image_dir="../dataset/imgs/" \
|
||||
--det_model_dir="./pretrain_models/det_ppocr_v3_en_infer_PCB" \
|
||||
--det_limit_side_len=48 \
|
||||
--det_limit_type='min' \
|
||||
--det_db_unclip_ratio=2.5 \
|
||||
--rec_model_dir="./pretrain_models/rec_ppocr_v3_ch_infer_PCB" \
|
||||
--rec_image_shape="3, 48, 320" \
|
||||
--draw_img_save_dir=./det_rec_infer/ \
|
||||
--use_space_char=False \
|
||||
--use_angle_cls=False \
|
||||
--use_gpu=True
|
||||
```
|
||||
|
||||
得到保存结果,文本检测识别可视化图保存在`det_rec_infer/`目录下,预测结果保存在`det_rec_infer/system_results.txt`中,格式如下:`0018.jpg [{"transcription": "E295", "points": [[88, 33], [137, 33], [137, 40], [88, 40]]}]`
|
||||
|
||||
2)然后将步骤一保存的数据转换为端对端评测需要的数据格式: 修改 `tools/end2end/convert_ppocr_label.py`中的代码,convert_label函数中设置输入标签路径,Mode,保存标签路径等,对预测数据的GTlabel和预测结果的label格式进行转换。
|
||||
```
|
||||
ppocr_label_gt = "/home/aistudio/dataset/det_gt_val.txt"
|
||||
convert_label(ppocr_label_gt, "gt", "./save_gt_label/")
|
||||
|
||||
ppocr_label_gt = "/home/aistudio/PaddleOCR/PCB_result/det_rec_infer/system_results.txt"
|
||||
convert_label(ppocr_label_gt, "pred", "./save_PPOCRV2_infer/")
|
||||
```
|
||||
|
||||
运行`convert_ppocr_label.py`:
|
||||
|
||||
|
||||
```python
|
||||
python3 tools/end2end/convert_ppocr_label.py
|
||||
```
|
||||
|
||||
得到如下结果:
|
||||
```
|
||||
├── ./save_gt_label/
|
||||
├── ./save_PPOCRV2_infer/
|
||||
```
|
||||
|
||||
3) 最后,执行端对端评测,运行`tools/end2end/eval_end2end.py`计算端对端指标,运行方式如下:
|
||||
|
||||
|
||||
```python
|
||||
pip install editdistance
|
||||
python3 tools/end2end/eval_end2end.py ./save_gt_label/ ./save_PPOCRV2_infer/
|
||||
```
|
||||
|
||||
使用`预训练模型+fine-tune'检测模型`、`预训练模型 + 2W张PCB图片funetune`识别模型,在300张PCB图片上评估得到如下结果,fmeasure为主要关注的指标:
|
||||
<div align=center><img src='https://ai-studio-static-online.cdn.bcebos.com/37206ea48a244212ae7a821d50d1fd51faf3d7fe97ac47a29f04dfcbb377b019', width='700'></div>
|
||||
<div align=center>图8 端到端评估指标</div>
|
||||
|
||||
```
|
||||
注: 使用上述命令不能跑出该结果,因为数据集不相同,可以更换为自己训练好的模型,按上述流程运行
|
||||
```
|
||||
|
||||
# 8. Jetson部署
|
||||
|
||||
我们只需要以下步骤就可以完成Jetson nano部署模型,简单易操作:
|
||||
|
||||
**1、在Jetson nano开发版上环境准备:**
|
||||
|
||||
* 安装PaddlePaddle
|
||||
|
||||
* 下载PaddleOCR并安装依赖
|
||||
|
||||
**2、执行预测**
|
||||
|
||||
* 将推理模型下载到jetson
|
||||
|
||||
* 执行检测、识别、串联预测即可
|
||||
|
||||
详细[参考流程](https://github.com/PaddlePaddle/PaddleOCR/blob/release/2.5/deploy/Jetson/readme_ch.md)。
|
||||
|
||||
# 9. 总结
|
||||
|
||||
检测实验分别使用PP-OCRv3预训练模型在PCB数据集上进行了直接评估、验证集padding、 fine-tune 3种方案,识别实验分别使用PP-OCRv3预训练模型在PCB数据集上进行了直接评估、 fine-tune、添加公开通用识别数据集、增加PCB图片数量4种方案,指标对比如下:
|
||||
|
||||
* 检测
|
||||
|
||||
|
||||
| 序号 | 方案 | hmean | 效果提升 | 实验分析 |
|
||||
| ---- | -------------------------------------------------------- | ------ | -------- | ------------------------------------- |
|
||||
| 1 | PP-OCRv3英文超轻量检测预训练模型直接评估 | 64.64% | - | 提供的预训练模型具有泛化能力 |
|
||||
| 2 | PP-OCRv3英文超轻量检测预训练模型 + 验证集padding直接评估 | 72.13% | +7.5% | padding可以提升尺寸较小图片的检测效果 |
|
||||
| 3 | PP-OCRv3英文超轻量检测预训练模型 + fine-tune | 100% | +27.9% | fine-tune会提升垂类场景效果 |
|
||||
|
||||
* 识别
|
||||
|
||||
| 序号 | 方案 | acc | 效果提升 | 实验分析 |
|
||||
| ---- | ------------------------------------------------------------ | ------ | -------- | ------------------------------------------------------------ |
|
||||
| 1 | PP-OCRv3中英文超轻量识别预训练模型直接评估 | 46.67% | - | 提供的预训练模型具有泛化能力 |
|
||||
| 2 | PP-OCRv3中英文超轻量识别预训练模型 + fine-tune | 42.02% | -4.6% | 在数据量不足的情况,反而比预训练模型效果低(也可以通过调整超参数再试试) |
|
||||
| 3 | PP-OCRv3中英文超轻量识别预训练模型 + fine-tune + 公开通用识别数据集 | 77% | +30% | 在数据量不足的情况下,可以考虑补充公开数据训练 |
|
||||
| 4 | PP-OCRv3中英文超轻量识别预训练模型 + fine-tune + 增加PCB图像数量 | 99.99% | +23% | 如果能获取更多数据量的情况,可以通过增加数据量提升效果 |
|
||||
|
||||
* 端到端
|
||||
|
||||
| det | rec | fmeasure |
|
||||
| --------------------------------------------- | ------------------------------------------------------------ | -------- |
|
||||
| PP-OCRv3英文超轻量检测预训练模型 + fine-tune | PP-OCRv3中英文超轻量识别预训练模型 + fine-tune + 增加PCB图像数量 | 93.3% |
|
||||
|
||||
*结论*
|
||||
|
||||
PP-OCRv3的检测模型在未经过fine-tune的情况下,在PCB数据集上也有64.64%的精度,说明具有泛化能力。验证集padding之后,精度提升7.5%,在图片尺寸较小的情况,我们可以通过padding的方式提升检测效果。经过 fine-tune 后能够极大的提升检测效果,精度达到100%。
|
||||
|
||||
PP-OCRv3的识别模型方案1和方案2对比可以发现,当数据量不足的情况,预训练模型精度可能比fine-tune效果还要高,所以我们可以先尝试预训练模型直接评估。如果在数据量不足的情况下想进一步提升模型效果,可以通过添加公开通用识别数据集,识别效果提升30%,非常有效。最后如果我们能够采集足够多的真实场景数据集,可以通过增加数据量提升模型效果,精度达到99.99%。
|
||||
|
||||
# 更多资源
|
||||
|
||||
- 更多深度学习知识、产业案例、面试宝典等,请参考:[awesome-DeepLearning](https://github.com/paddlepaddle/awesome-DeepLearning)
|
||||
|
||||
- 更多PaddleOCR使用教程,请参考:[PaddleOCR](https://github.com/PaddlePaddle/PaddleOCR/tree/dygraph)
|
||||
|
||||
|
||||
- 飞桨框架相关资料,请参考:[飞桨深度学习平台](https://www.paddlepaddle.org.cn/?fr=paddleEdu_aistudio)
|
||||
|
||||
# 参考
|
||||
|
||||
* 数据生成代码库:https://github.com/zcswdt/Color_OCR_image_generator
|
Binary file not shown.
After Width: | Height: | Size: 2.0 KiB |
|
@ -0,0 +1,30 @@
|
|||
5ZQ
|
||||
I4UL
|
||||
PWL
|
||||
SNOG
|
||||
ZL02
|
||||
1C30
|
||||
O3H
|
||||
YHRS
|
||||
N03S
|
||||
1U5Y
|
||||
JTK
|
||||
EN4F
|
||||
YKJ
|
||||
DWNH
|
||||
R42W
|
||||
X0V
|
||||
4OF5
|
||||
08AM
|
||||
Y93S
|
||||
GWE2
|
||||
0KR
|
||||
9U2A
|
||||
DBQ
|
||||
Y6J
|
||||
ROZ
|
||||
K06
|
||||
KIEY
|
||||
NZQJ
|
||||
UN1B
|
||||
6X4
|
Binary file not shown.
After Width: | Height: | Size: 145 B |
Binary file not shown.
After Width: | Height: | Size: 141 B |
|
@ -0,0 +1,261 @@
|
|||
# copyright (c) 2020 PaddlePaddle Authors. All Rights Reserve.
|
||||
#
|
||||
# Licensed under the Apache License, Version 2.0 (the "License");
|
||||
# you may not use this file except in compliance with the License.
|
||||
# You may obtain a copy of the License at
|
||||
#
|
||||
# http://www.apache.org/licenses/LICENSE-2.0
|
||||
#
|
||||
# Unless required by applicable law or agreed to in writing, software
|
||||
# distributed under the License is distributed on an "AS IS" BASIS,
|
||||
# WITHOUT WARRANTIES OR CONDITIONS OF ANY KIND, either express or implied.
|
||||
# See the License for the specific language governing permissions and
|
||||
# limitations under the License.
|
||||
"""
|
||||
This code is refer from:
|
||||
https://github.com/zcswdt/Color_OCR_image_generator
|
||||
"""
|
||||
import os
|
||||
import random
|
||||
from PIL import Image, ImageDraw, ImageFont
|
||||
import json
|
||||
import argparse
|
||||
|
||||
|
||||
def get_char_lines(txt_root_path):
|
||||
"""
|
||||
desc:get corpus line
|
||||
"""
|
||||
txt_files = os.listdir(txt_root_path)
|
||||
char_lines = []
|
||||
for txt in txt_files:
|
||||
f = open(os.path.join(txt_root_path, txt), mode='r', encoding='utf-8')
|
||||
lines = f.readlines()
|
||||
f.close()
|
||||
for line in lines:
|
||||
char_lines.append(line.strip())
|
||||
return char_lines
|
||||
|
||||
|
||||
def get_horizontal_text_picture(image_file, chars, fonts_list, cf):
|
||||
"""
|
||||
desc:gen horizontal text picture
|
||||
"""
|
||||
img = Image.open(image_file)
|
||||
if img.mode != 'RGB':
|
||||
img = img.convert('RGB')
|
||||
img_w, img_h = img.size
|
||||
|
||||
# random choice font
|
||||
font_path = random.choice(fonts_list)
|
||||
# random choice font size
|
||||
font_size = random.randint(cf.font_min_size, cf.font_max_size)
|
||||
font = ImageFont.truetype(font_path, font_size)
|
||||
|
||||
ch_w = []
|
||||
ch_h = []
|
||||
for ch in chars:
|
||||
wt, ht = font.getsize(ch)
|
||||
ch_w.append(wt)
|
||||
ch_h.append(ht)
|
||||
f_w = sum(ch_w)
|
||||
f_h = max(ch_h)
|
||||
|
||||
# add space
|
||||
char_space_width = max(ch_w)
|
||||
f_w += (char_space_width * (len(chars) - 1))
|
||||
|
||||
x1 = random.randint(0, img_w - f_w)
|
||||
y1 = random.randint(0, img_h - f_h)
|
||||
x2 = x1 + f_w
|
||||
y2 = y1 + f_h
|
||||
|
||||
crop_y1 = y1
|
||||
crop_x1 = x1
|
||||
crop_y2 = y2
|
||||
crop_x2 = x2
|
||||
|
||||
best_color = (0, 0, 0)
|
||||
draw = ImageDraw.Draw(img)
|
||||
for i, ch in enumerate(chars):
|
||||
draw.text((x1, y1), ch, best_color, font=font)
|
||||
x1 += (ch_w[i] + char_space_width)
|
||||
crop_img = img.crop((crop_x1, crop_y1, crop_x2, crop_y2))
|
||||
return crop_img, chars
|
||||
|
||||
|
||||
def get_vertical_text_picture(image_file, chars, fonts_list, cf):
|
||||
"""
|
||||
desc:gen vertical text picture
|
||||
"""
|
||||
img = Image.open(image_file)
|
||||
if img.mode != 'RGB':
|
||||
img = img.convert('RGB')
|
||||
img_w, img_h = img.size
|
||||
# random choice font
|
||||
font_path = random.choice(fonts_list)
|
||||
# random choice font size
|
||||
font_size = random.randint(cf.font_min_size, cf.font_max_size)
|
||||
font = ImageFont.truetype(font_path, font_size)
|
||||
|
||||
ch_w = []
|
||||
ch_h = []
|
||||
for ch in chars:
|
||||
wt, ht = font.getsize(ch)
|
||||
ch_w.append(wt)
|
||||
ch_h.append(ht)
|
||||
f_w = max(ch_w)
|
||||
f_h = sum(ch_h)
|
||||
|
||||
x1 = random.randint(0, img_w - f_w)
|
||||
y1 = random.randint(0, img_h - f_h)
|
||||
x2 = x1 + f_w
|
||||
y2 = y1 + f_h
|
||||
|
||||
crop_y1 = y1
|
||||
crop_x1 = x1
|
||||
crop_y2 = y2
|
||||
crop_x2 = x2
|
||||
|
||||
best_color = (0, 0, 0)
|
||||
draw = ImageDraw.Draw(img)
|
||||
i = 0
|
||||
for ch in chars:
|
||||
draw.text((x1, y1), ch, best_color, font=font)
|
||||
y1 = y1 + ch_h[i]
|
||||
i = i + 1
|
||||
crop_img = img.crop((crop_x1, crop_y1, crop_x2, crop_y2))
|
||||
crop_img = crop_img.transpose(Image.ROTATE_90)
|
||||
return crop_img, chars
|
||||
|
||||
|
||||
def get_fonts(fonts_path):
|
||||
"""
|
||||
desc: get all fonts
|
||||
"""
|
||||
font_files = os.listdir(fonts_path)
|
||||
fonts_list=[]
|
||||
for font_file in font_files:
|
||||
font_path=os.path.join(fonts_path, font_file)
|
||||
fonts_list.append(font_path)
|
||||
return fonts_list
|
||||
|
||||
if __name__ == '__main__':
|
||||
parser = argparse.ArgumentParser()
|
||||
parser.add_argument('--num_img', type=int, default=30, help="Number of images to generate")
|
||||
parser.add_argument('--font_min_size', type=int, default=11)
|
||||
parser.add_argument('--font_max_size', type=int, default=12,
|
||||
help="Help adjust the size of the generated text and the size of the picture")
|
||||
parser.add_argument('--bg_path', type=str, default='./background',
|
||||
help='The generated text pictures will be pasted onto the pictures of this folder')
|
||||
parser.add_argument('--det_bg_path', type=str, default='./det_background',
|
||||
help='The generated text pictures will use the pictures of this folder as the background')
|
||||
parser.add_argument('--fonts_path', type=str, default='../../StyleText/fonts',
|
||||
help='The font used to generate the picture')
|
||||
parser.add_argument('--corpus_path', type=str, default='./corpus',
|
||||
help='The corpus used to generate the text picture')
|
||||
parser.add_argument('--output_dir', type=str, default='./output/', help='Images save dir')
|
||||
|
||||
|
||||
cf = parser.parse_args()
|
||||
# save path
|
||||
if not os.path.exists(cf.output_dir):
|
||||
os.mkdir(cf.output_dir)
|
||||
|
||||
# get corpus
|
||||
txt_root_path = cf.corpus_path
|
||||
char_lines = get_char_lines(txt_root_path=txt_root_path)
|
||||
|
||||
# get all fonts
|
||||
fonts_path = cf.fonts_path
|
||||
fonts_list = get_fonts(fonts_path)
|
||||
|
||||
# rec bg
|
||||
img_root_path = cf.bg_path
|
||||
imnames=os.listdir(img_root_path)
|
||||
|
||||
# det bg
|
||||
det_bg_path = cf.det_bg_path
|
||||
bg_pics = os.listdir(det_bg_path)
|
||||
|
||||
# OCR det files
|
||||
det_val_file = open(cf.output_dir + 'det_gt_val.txt', 'w', encoding='utf-8')
|
||||
det_train_file = open(cf.output_dir + 'det_gt_train.txt', 'w', encoding='utf-8')
|
||||
# det imgs
|
||||
det_save_dir = 'imgs/'
|
||||
if not os.path.exists(cf.output_dir + det_save_dir):
|
||||
os.mkdir(cf.output_dir + det_save_dir)
|
||||
det_val_save_dir = 'imgs_val/'
|
||||
if not os.path.exists(cf.output_dir + det_val_save_dir):
|
||||
os.mkdir(cf.output_dir + det_val_save_dir)
|
||||
|
||||
# OCR rec files
|
||||
rec_val_file = open(cf.output_dir + 'rec_gt_val.txt', 'w', encoding='utf-8')
|
||||
rec_train_file = open(cf.output_dir + 'rec_gt_train.txt', 'w', encoding='utf-8')
|
||||
# rec imgs
|
||||
rec_save_dir = 'rec_imgs/'
|
||||
if not os.path.exists(cf.output_dir + rec_save_dir):
|
||||
os.mkdir(cf.output_dir + rec_save_dir)
|
||||
rec_val_save_dir = 'rec_imgs_val/'
|
||||
if not os.path.exists(cf.output_dir + rec_val_save_dir):
|
||||
os.mkdir(cf.output_dir + rec_val_save_dir)
|
||||
|
||||
|
||||
val_ratio = cf.num_img * 0.2 # val dataset ratio
|
||||
|
||||
print('start generating...')
|
||||
for i in range(0, cf.num_img):
|
||||
imname = random.choice(imnames)
|
||||
img_path = os.path.join(img_root_path, imname)
|
||||
|
||||
rnd = random.random()
|
||||
# gen horizontal text picture
|
||||
if rnd < 0.5:
|
||||
gen_img, chars = get_horizontal_text_picture(img_path, char_lines[i], fonts_list, cf)
|
||||
ori_w, ori_h = gen_img.size
|
||||
gen_img = gen_img.crop((0, 3, ori_w, ori_h))
|
||||
# gen vertical text picture
|
||||
else:
|
||||
gen_img, chars = get_vertical_text_picture(img_path, char_lines[i], fonts_list, cf)
|
||||
ori_w, ori_h = gen_img.size
|
||||
gen_img = gen_img.crop((3, 0, ori_w, ori_h))
|
||||
|
||||
ori_w, ori_h = gen_img.size
|
||||
|
||||
# rec imgs
|
||||
save_img_name = str(i).zfill(4) + '.jpg'
|
||||
if i < val_ratio:
|
||||
save_dir = os.path.join(rec_val_save_dir, save_img_name)
|
||||
line = save_dir + '\t' + char_lines[i] + '\n'
|
||||
rec_val_file.write(line)
|
||||
else:
|
||||
save_dir = os.path.join(rec_save_dir, save_img_name)
|
||||
line = save_dir + '\t' + char_lines[i] + '\n'
|
||||
rec_train_file.write(line)
|
||||
gen_img.save(cf.output_dir + save_dir, quality = 95, subsampling=0)
|
||||
|
||||
# det img
|
||||
# random choice bg
|
||||
bg_pic = random.sample(bg_pics, 1)[0]
|
||||
det_img = Image.open(os.path.join(det_bg_path, bg_pic))
|
||||
# the PCB position is fixed, modify it according to your own scenario
|
||||
if bg_pic == '1.png':
|
||||
x1 = 38
|
||||
y1 = 3
|
||||
else:
|
||||
x1 = 34
|
||||
y1 = 1
|
||||
|
||||
det_img.paste(gen_img, (x1, y1))
|
||||
# text pos
|
||||
chars_pos = [[x1, y1], [x1 + ori_w, y1], [x1 + ori_w, y1 + ori_h], [x1, y1 + ori_h]]
|
||||
label = [{"transcription":char_lines[i], "points":chars_pos}]
|
||||
if i < val_ratio:
|
||||
save_dir = os.path.join(det_val_save_dir, save_img_name)
|
||||
det_val_file.write(save_dir + '\t' + json.dumps(
|
||||
label, ensure_ascii=False) + '\n')
|
||||
else:
|
||||
save_dir = os.path.join(det_save_dir, save_img_name)
|
||||
det_train_file.write(save_dir + '\t' + json.dumps(
|
||||
label, ensure_ascii=False) + '\n')
|
||||
det_img.save(cf.output_dir + save_dir, quality = 95, subsampling=0)
|
|
@ -1,646 +0,0 @@
|
|||
# 一种基于PaddleOCR的轻量级车牌识别模型
|
||||
|
||||
- [1. 项目介绍](#1-项目介绍)
|
||||
- [2. 环境搭建](#2-环境搭建)
|
||||
- [3. 数据集准备](#3-数据集准备)
|
||||
- [3.1 数据集标注规则](#31-数据集标注规则)
|
||||
- [3.2 制作符合PP-OCR训练格式的标注文件](#32-制作符合pp-ocr训练格式的标注文件)
|
||||
- [4. 实验](#4-实验)
|
||||
- [4.1 检测](#41-检测)
|
||||
- [4.1.1 预训练模型直接预测](#411-预训练模型直接预测)
|
||||
- [4.1.2 CCPD车牌数据集fine-tune](#412-ccpd车牌数据集fine-tune)
|
||||
- [4.1.3 CCPD车牌数据集fine-tune+量化训练](#413-ccpd车牌数据集fine-tune量化训练)
|
||||
- [4.1.4 模型导出](#414-模型导出)
|
||||
- [4.2 识别](#42-识别)
|
||||
- [4.2.1 预训练模型直接预测](#421-预训练模型直接预测)
|
||||
- [4.2.2 预训练模型直接预测+改动后处理](#422-预训练模型直接预测改动后处理)
|
||||
- [4.2.3 CCPD车牌数据集fine-tune](#423-ccpd车牌数据集fine-tune)
|
||||
- [4.2.4 CCPD车牌数据集fine-tune+量化训练](#424-ccpd车牌数据集fine-tune量化训练)
|
||||
- [4.2.5 模型导出](#425-模型导出)
|
||||
- [4.3 串联推理](#43-串联推理)
|
||||
- [4.4 实验总结](#44-实验总结)
|
||||
|
||||
## 1. 项目介绍
|
||||
|
||||
车牌识别(Vehicle License Plate Recognition,VLPR) 是计算机视频图像识别技术在车辆牌照识别中的一种应用。车牌识别技术要求能够将运动中的汽车牌照从复杂背景中提取并识别出来,在高速公路车辆管理,停车场管理和中得到广泛应用。
|
||||
|
||||
结合我国国情,目前车牌识别技术的难点有:
|
||||
|
||||
1. 车牌样式多。我国车牌颜色大致有四种:黄底黑字、蓝底白字、白底黑字、黑底白字;车牌格式包括民用车牌、武警车牌、军车车牌、外交车牌、特种车牌、消防车牌等等。
|
||||
2. 车牌位置不固定。由于不同汽车品牌公司出产的汽车型号和外形各有不同,每辆车的车牌悬挂位置也不一样;
|
||||
3. 图像质量差: 运动模糊,由于强光,反射或阴影造成的光照和对比度较差, 车牌(部分)遮挡;
|
||||
4. 在车辆管理等场景场景对于模型速度有着一定限制。
|
||||
|
||||
针对以上问题, 本例选用 [PP-OCRv3](../doc/doc_ch/PP-OCRv3_introduction.md) 这一开源超轻量OCR系统进行车牌识别系统的开发。基于PP-OCRv3模型,在CCPD数据集达到99%的检测和94%的识别精度,模型大小12.8M(2.5M+10.3M)。基于量化对模型体积进行进一步压缩到5.8M(1M+4.8M), 同时推理速度提升x%。
|
||||
|
||||
aistudio项目链接: [基于PaddleOCR的轻量级车牌识别范例](https://aistudio.baidu.com/aistudio/projectdetail/3919091?contributionType=1)
|
||||
|
||||
## 2. 环境搭建
|
||||
|
||||
本任务基于Aistudio完成, 具体环境如下:
|
||||
|
||||
- 操作系统: Linux
|
||||
- PaddlePaddle: 2.3
|
||||
- paddleslim: 2.2.2
|
||||
- PaddleOCR: Release/2.5
|
||||
|
||||
下载 PaddleOCR代码
|
||||
|
||||
```bash
|
||||
git clone -b dygraph https://github.com/PaddlePaddle/PaddleOCR
|
||||
```
|
||||
|
||||
安装依赖库
|
||||
|
||||
```bash
|
||||
pip install -r PaddleOCR/requirements.txt
|
||||
```
|
||||
|
||||
## 3. 数据集准备
|
||||
|
||||
所使用的数据集为 CCPD2020 新能源车牌数据集,该数据集为
|
||||
|
||||
该数据集分布如下:
|
||||
|
||||
|数据集类型|数量|
|
||||
|---|---|
|
||||
|训练集| 5769|
|
||||
|验证集| 1001|
|
||||
|测试集| 5006|
|
||||
|
||||
数据集图片示例如下:
|
||||
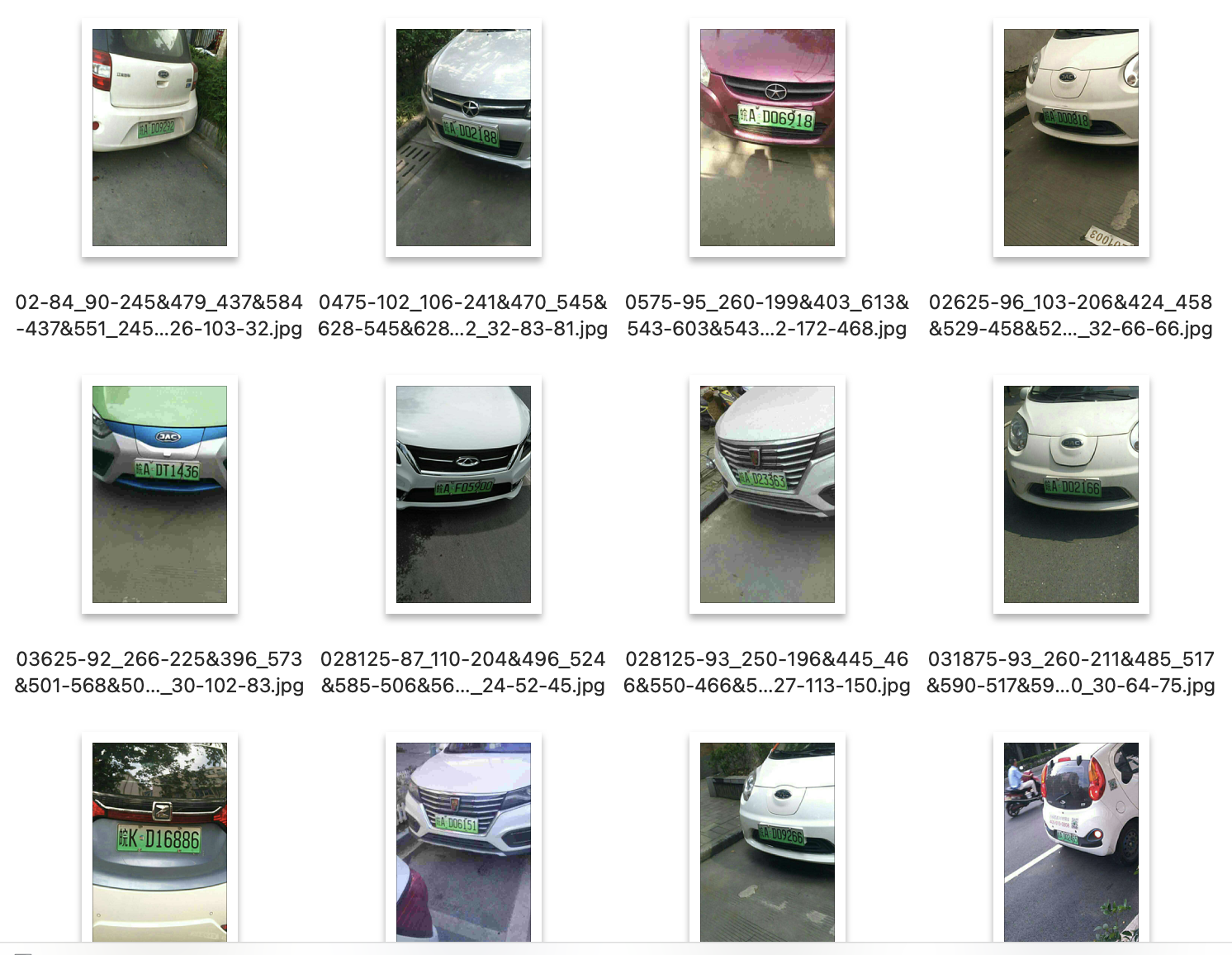
|
||||
|
||||
数据集可以从这里下载 https://aistudio.baidu.com/aistudio/datasetdetail/101595
|
||||
|
||||
下载好数据集后对数据集进行解压
|
||||
|
||||
```bash
|
||||
unzip -d /home/aistudio/data /home/aistudio/data/data101595/CCPD2020.zip
|
||||
```
|
||||
|
||||
### 3.1 数据集标注规则
|
||||
|
||||
CPPD数据集的图片文件名具有特殊规则,详细可查看:https://github.com/detectRecog/CCPD
|
||||
|
||||
具体规则如下:
|
||||
|
||||
例如: 025-95_113-154&383_386&473-386&473_177&454_154&383_363&402-0_0_22_27_27_33_16-37-15.jpg
|
||||
|
||||
由分隔符'-'分为几个部分:
|
||||
|
||||
- 025:车牌区域与整个图片区域的面积比。
|
||||
|
||||
- 95_113: 车牌水平倾斜度和垂直倾斜度, 水平95°, 竖直113°
|
||||
|
||||
- 154&383_386&473: 车牌边界框坐标:左上(154, 383), 右下(386, 473)
|
||||
|
||||
- 386&473_177&454_154&383_363&402: 车牌四个角点坐标, 坐标顺序为[右下,左下,左上,右上]
|
||||
|
||||
- 0_0_22_27_27_33_16: 车牌号码,CCPD中的每个图像只有一个LP。每个LP编号由一个汉字、一个字母和五个字母或数字组成。有效的中国车牌由七个字符组成:省(1个字符)、字母(1个字符)、字母+数字(5个字符)。“0_0_22_27_27_33_16”是每个字符的索引。这三个数组的定义如下。每个数组的最后一个字符是字母O,而不是数字0。我们使用O作为“无字符”的标志,因为中国车牌字符中没有O。
|
||||
|
||||
```python
|
||||
provinces = ["皖", "沪", "津", "渝", "冀", "晋", "蒙", "辽", "吉", "黑", "苏", "浙", "京", "闽", "赣", "鲁", "豫", "鄂", "湘", "粤", "桂", "琼", "川", "贵", "云", "藏", "陕", "甘", "青", "宁", "新", "警", "学", "O"]
|
||||
alphabets = ['A', 'B', 'C', 'D', 'E', 'F', 'G', 'H', 'J', 'K', 'L', 'M', 'N', 'P', 'Q', 'R', 'S', 'T', 'U', 'V', 'W',
|
||||
'X', 'Y', 'Z', 'O']
|
||||
ads = ['A', 'B', 'C', 'D', 'E', 'F', 'G', 'H', 'J', 'K', 'L', 'M', 'N', 'P', 'Q', 'R', 'S', 'T', 'U', 'V', 'W', 'X',
|
||||
'Y', 'Z', '0', '1', '2', '3', '4', '5', '6', '7', '8', '9', 'O']
|
||||
|
||||
```
|
||||
|
||||
### 3.2 制作符合PP-OCR训练格式的标注文件
|
||||
|
||||
在开始训练之前,可使用如下代码制作符合PP-OCR训练格式的标注文件。
|
||||
|
||||
|
||||
```python
|
||||
import cv2
|
||||
import os
|
||||
import json
|
||||
from tqdm import tqdm
|
||||
import numpy as np
|
||||
|
||||
provinces = ["皖", "沪", "津", "渝", "冀", "晋", "蒙", "辽", "吉", "黑", "苏", "浙", "京", "闽", "赣", "鲁", "豫", "鄂", "湘", "粤", "桂", "琼", "川", "贵", "云", "藏", "陕", "甘", "青", "宁", "新", "警", "学", "O"]
|
||||
alphabets = ['A', 'B', 'C', 'D', 'E', 'F', 'G', 'H', 'J', 'K', 'L', 'M', 'N', 'P', 'Q', 'R', 'S', 'T', 'U', 'V', 'W', 'X', 'Y', 'Z', 'O']
|
||||
ads = ['A', 'B', 'C', 'D', 'E', 'F', 'G', 'H', 'J', 'K', 'L', 'M', 'N', 'P', 'Q', 'R', 'S', 'T', 'U', 'V', 'W', 'X', 'Y', 'Z', '0', '1', '2', '3', '4', '5', '6', '7', '8', '9', 'O']
|
||||
|
||||
def make_label(img_dir, save_gt_folder, phase):
|
||||
crop_img_save_dir = os.path.join(save_gt_folder, phase, 'crop_imgs')
|
||||
os.makedirs(crop_img_save_dir, exist_ok=True)
|
||||
|
||||
f_det = open(os.path.join(save_gt_folder, phase, 'det.txt'), 'w', encoding='utf-8')
|
||||
f_rec = open(os.path.join(save_gt_folder, phase, 'rec.txt'), 'w', encoding='utf-8')
|
||||
|
||||
i = 0
|
||||
for filename in tqdm(os.listdir(os.path.join(img_dir, phase))):
|
||||
str_list = filename.split('-')
|
||||
if len(str_list) < 5:
|
||||
continue
|
||||
coord_list = str_list[3].split('_')
|
||||
txt_list = str_list[4].split('_')
|
||||
boxes = []
|
||||
for coord in coord_list:
|
||||
boxes.append([int(x) for x in coord.split("&")])
|
||||
boxes = [boxes[2], boxes[3], boxes[0], boxes[1]]
|
||||
lp_number = provinces[int(txt_list[0])] + alphabets[int(txt_list[1])] + ''.join([ads[int(x)] for x in txt_list[2:]])
|
||||
|
||||
# det
|
||||
det_info = [{'points':boxes, 'transcription':lp_number}]
|
||||
f_det.write('{}\t{}\n'.format(os.path.join(phase, filename), json.dumps(det_info, ensure_ascii=False)))
|
||||
|
||||
# rec
|
||||
boxes = np.float32(boxes)
|
||||
img = cv2.imread(os.path.join(img_dir, phase, filename))
|
||||
# crop_img = img[int(boxes[:,1].min()):int(boxes[:,1].max()),int(boxes[:,0].min()):int(boxes[:,0].max())]
|
||||
crop_img = get_rotate_crop_image(img, boxes)
|
||||
crop_img_save_filename = '{}_{}.jpg'.format(i,'_'.join(txt_list))
|
||||
crop_img_save_path = os.path.join(crop_img_save_dir, crop_img_save_filename)
|
||||
cv2.imwrite(crop_img_save_path, crop_img)
|
||||
f_rec.write('{}/crop_imgs/{}\t{}\n'.format(phase, crop_img_save_filename, lp_number))
|
||||
i+=1
|
||||
f_det.close()
|
||||
f_rec.close()
|
||||
|
||||
def get_rotate_crop_image(img, points):
|
||||
'''
|
||||
img_height, img_width = img.shape[0:2]
|
||||
left = int(np.min(points[:, 0]))
|
||||
right = int(np.max(points[:, 0]))
|
||||
top = int(np.min(points[:, 1]))
|
||||
bottom = int(np.max(points[:, 1]))
|
||||
img_crop = img[top:bottom, left:right, :].copy()
|
||||
points[:, 0] = points[:, 0] - left
|
||||
points[:, 1] = points[:, 1] - top
|
||||
'''
|
||||
assert len(points) == 4, "shape of points must be 4*2"
|
||||
img_crop_width = int(
|
||||
max(
|
||||
np.linalg.norm(points[0] - points[1]),
|
||||
np.linalg.norm(points[2] - points[3])))
|
||||
img_crop_height = int(
|
||||
max(
|
||||
np.linalg.norm(points[0] - points[3]),
|
||||
np.linalg.norm(points[1] - points[2])))
|
||||
pts_std = np.float32([[0, 0], [img_crop_width, 0],
|
||||
[img_crop_width, img_crop_height],
|
||||
[0, img_crop_height]])
|
||||
M = cv2.getPerspectiveTransform(points, pts_std)
|
||||
dst_img = cv2.warpPerspective(
|
||||
img,
|
||||
M, (img_crop_width, img_crop_height),
|
||||
borderMode=cv2.BORDER_REPLICATE,
|
||||
flags=cv2.INTER_CUBIC)
|
||||
dst_img_height, dst_img_width = dst_img.shape[0:2]
|
||||
if dst_img_height * 1.0 / dst_img_width >= 1.5:
|
||||
dst_img = np.rot90(dst_img)
|
||||
return dst_img
|
||||
|
||||
img_dir = '/home/aistudio/data/CCPD2020/ccpd_green'
|
||||
save_gt_folder = '/home/aistudio/data/CCPD2020/PPOCR'
|
||||
# phase = 'train' # change to val and test to make val dataset and test dataset
|
||||
for phase in ['train','val','test']:
|
||||
make_label(img_dir, save_gt_folder, phase)
|
||||
```
|
||||
|
||||
通过上述命令可以完成了`训练集`,`验证集`和`测试集`的制作,制作完成的数据集信息如下:
|
||||
|
||||
| 类型 | 数据集 | 图片地址 | 标签地址 | 图片数量 |
|
||||
| --- | --- | --- | --- | --- |
|
||||
| 检测 | 训练集 | /home/aistudio/data/CCPD2020/ccpd_green/train | /home/aistudio/data/CCPD2020/PPOCR/train/det.txt | 5769 |
|
||||
| 检测 | 验证集 | /home/aistudio/data/CCPD2020/ccpd_green/val | /home/aistudio/data/CCPD2020/PPOCR/val/det.txt | 1001 |
|
||||
| 检测 | 测试集 | /home/aistudio/data/CCPD2020/ccpd_green/test | /home/aistudio/data/CCPD2020/PPOCR/test/det.txt | 5006 |
|
||||
| 识别 | 训练集 | /home/aistudio/data/CCPD2020/PPOCR/train/crop_imgs | /home/aistudio/data/CCPD2020/PPOCR/train/rec.txt | 5769 |
|
||||
| 识别 | 验证集 | /home/aistudio/data/CCPD2020/PPOCR/val/crop_imgs | /home/aistudio/data/CCPD2020/PPOCR/val/rec.txt | 1001 |
|
||||
| 识别 | 测试集 | /home/aistudio/data/CCPD2020/PPOCR/test/crop_imgs | /home/aistudio/data/CCPD2020/PPOCR/test/rec.txt | 5006 |
|
||||
|
||||
在普遍的深度学习流程中,都是在训练集训练,在验证集选择最优模型后在测试集上进行测试。在本例中,我们省略中间步骤,直接在训练集训练,在测试集选择最优模型,因此我们只使用训练集和测试集。
|
||||
|
||||
## 4. 实验
|
||||
|
||||
由于数据集比较少,为了模型更好和更快的收敛,这里选用 PaddleOCR 中的 PP-OCRv3 模型进行文本检测和识别,并且使用 PP-OCRv3 模型参数作为预训练模型。PP-OCRv3在PP-OCRv2的基础上,中文场景端到端Hmean指标相比于PP-OCRv2提升5%, 英文数字模型端到端效果提升11%。详细优化细节请参考[PP-OCRv3](../doc/doc_ch/PP-OCRv3_introduction.md)技术报告。
|
||||
|
||||
由于车牌场景均为端侧设备部署,因此对速度和模型大小有比较高的要求,因此还需要采用量化训练的方式进行模型大小的压缩和模型推理速度的加速。模型量化可以在基本不损失模型的精度的情况下,将FP32精度的模型参数转换为Int8精度,减小模型参数大小并加速计算,使用量化后的模型在移动端等部署时更具备速度优势。
|
||||
|
||||
因此,本实验中对于车牌检测和识别有如下3种方案:
|
||||
|
||||
1. PP-OCRv3中英文超轻量预训练模型直接预测
|
||||
2. CCPD车牌数据集在PP-OCRv3模型上fine-tune
|
||||
3. CCPD车牌数据集在PP-OCRv3模型上fine-tune后量化
|
||||
|
||||
### 4.1 检测
|
||||
#### 4.1.1 预训练模型直接预测
|
||||
|
||||
从下表中下载PP-OCRv3文本检测预训练模型
|
||||
|
||||
|模型名称|模型简介|配置文件|推理模型大小|下载地址|
|
||||
| --- | --- | --- | --- | --- |
|
||||
|ch_PP-OCRv3_det| 【最新】原始超轻量模型,支持中英文、多语种文本检测 |[ch_PP-OCRv3_det_cml.yml](https://github.com/PaddlePaddle/PaddleOCR/blob/dygraph/configs/det/ch_PP-OCRv3/ch_PP-OCRv3_det_cml.yml)| 3.8M |[推理模型](https://paddleocr.bj.bcebos.com/PP-OCRv3/chinese/ch_PP-OCRv3_det_infer.tar) / [训练模型](https://paddleocr.bj.bcebos.com/PP-OCRv3/chinese/ch_PP-OCRv3_det_distill_train.tar)|
|
||||
|
||||
使用如下命令下载预训练模型
|
||||
|
||||
```bash
|
||||
mkdir models
|
||||
cd models
|
||||
wget https://paddleocr.bj.bcebos.com/PP-OCRv3/chinese/ch_PP-OCRv3_det_distill_train.tar
|
||||
tar -xf ch_PP-OCRv3_det_distill_train.tar
|
||||
cd /home/aistudio/PaddleOCR
|
||||
```
|
||||
|
||||
预训练模型下载完成后,我们使用[ch_PP-OCRv3_det_student.yml](../configs/chepai/ch_PP-OCRv3_det_student.yml) 配置文件进行后续实验,在开始评估之前需要对配置文件中部分字段进行设置,具体如下:
|
||||
|
||||
1. 模型存储和训练相关:
|
||||
1. Global.pretrained_model: 指向PP-OCRv3文本检测预训练模型地址
|
||||
2. 数据集相关
|
||||
1. Eval.dataset.data_dir:指向测试集图片存放目录
|
||||
2. Eval.dataset.label_file_list:指向测试集标注文件
|
||||
|
||||
上述字段均为必须修改的字段,可以通过修改配置文件的方式改动,也可在不需要修改配置文件的情况下,改变训练的参数。这里使用不改变配置文件的方式 。使用如下命令进行PP-OCRv3文本检测预训练模型的评估
|
||||
|
||||
|
||||
```bash
|
||||
python tools/eval.py -c configs/det/ch_PP-OCRv3/ch_PP-OCRv3_det_student.yml -o \
|
||||
Global.pretrained_model=models/ch_PP-OCRv3_det_distill_train/student.pdparams \
|
||||
Eval.dataset.data_dir=/home/aistudio/data/CCPD2020/ccpd_green \
|
||||
Eval.dataset.label_file_list=[/home/aistudio/data/CCPD2020/PPOCR/test/det.txt]
|
||||
```
|
||||
上述指令中,通过-c 选择训练使用配置文件,通过-o参数在不需要修改配置文件的情况下,改变训练的参数。
|
||||
|
||||
使用预训练模型进行评估,指标如下所示:
|
||||
|
||||
| 方案 |hmeans|
|
||||
|---------------------------|---|
|
||||
| PP-OCRv3中英文超轻量检测预训练模型直接预测 |76.12%|
|
||||
|
||||
#### 4.1.2 CCPD车牌数据集fine-tune
|
||||
|
||||
**训练**
|
||||
|
||||
为了进行fine-tune训练,我们需要在配置文件中设置需要使用的预训练模型地址,学习率和数据集等参数。 具体如下:
|
||||
|
||||
1. 模型存储和训练相关:
|
||||
1. Global.pretrained_model: 指向PP-OCRv3文本检测预训练模型地址
|
||||
2. Global.eval_batch_step: 模型多少step评估一次,这里设为从第0个step开始没隔772个step评估一次,772为一个epoch总的step数。
|
||||
2. 优化器相关:
|
||||
1. Optimizer.lr.name: 学习率衰减器设为常量 Const
|
||||
2. Optimizer.lr.learning_rate: 做finetune实验,学习率需要设置的比较小,此处学习率设为配置文件中的0.05倍
|
||||
3. Optimizer.lr.warmup_epoch: warmup_epoch设为0
|
||||
3. 数据集相关:
|
||||
1. Train.dataset.data_dir:指向训练集图片存放目录
|
||||
2. Train.dataset.label_file_list:指向训练集标注文件
|
||||
3. Eval.dataset.data_dir:指向测试集图片存放目录
|
||||
4. Eval.dataset.label_file_list:指向测试集标注文件
|
||||
|
||||
使用如下代码即可启动在CCPD车牌数据集上的fine-tune。
|
||||
|
||||
```bash
|
||||
python tools/train.py -c configs/det/ch_PP-OCRv3/ch_PP-OCRv3_det_student.yml -o \
|
||||
Global.pretrained_model=models/ch_PP-OCRv3_det_distill_train/student.pdparams \
|
||||
Global.save_model_dir=output/CCPD/det \
|
||||
Global.eval_batch_step="[0, 772]" \
|
||||
Optimizer.lr.name=Const \
|
||||
Optimizer.lr.learning_rate=0.0005 \
|
||||
Optimizer.lr.warmup_epoch=0 \
|
||||
Train.dataset.data_dir=/home/aistudio/data/CCPD2020/ccpd_green \
|
||||
Train.dataset.label_file_list=[/home/aistudio/data/CCPD2020/PPOCR/train/det.txt] \
|
||||
Eval.dataset.data_dir=/home/aistudio/data/CCPD2020/ccpd_green \
|
||||
Eval.dataset.label_file_list=[/home/aistudio/data/CCPD2020/PPOCR/test/det.txt]
|
||||
```
|
||||
|
||||
在上述命令中,通过`-o`的方式修改了配置文件中的参数。
|
||||
|
||||
训练好的模型地址为: [det_ppocr_v3_finetune.tar](https://paddleocr.bj.bcebos.com/fanliku/license_plate_recognition/det_ppocr_v3_finetune.tar)
|
||||
|
||||
**评估**
|
||||
|
||||
训练完成后使用如下命令进行评估
|
||||
|
||||
|
||||
```bash
|
||||
python tools/eval.py -c configs/det/ch_PP-OCRv3/ch_PP-OCRv3_det_student.yml -o \
|
||||
Global.pretrained_model=output/CCPD/det/best_accuracy.pdparams \
|
||||
Eval.dataset.data_dir=/home/aistudio/data/CCPD2020/ccpd_green \
|
||||
Eval.dataset.label_file_list=[/home/aistudio/data/CCPD2020/PPOCR/test/det.txt]
|
||||
```
|
||||
|
||||
使用预训练模型和CCPD车牌数据集fine-tune,指标分别如下:
|
||||
|
||||
|方案|hmeans|
|
||||
|---|---|
|
||||
|PP-OCRv3中英文超轻量检测预训练模型直接预测|76.12%|
|
||||
|PP-OCRv3中英文超轻量检测预训练模型 fine-tune|99%|
|
||||
|
||||
可以看到进行fine-tune能显著提升车牌检测的效果。
|
||||
|
||||
#### 4.1.3 CCPD车牌数据集fine-tune+量化训练
|
||||
|
||||
此处采用 PaddleOCR 中提供好的[量化教程](../deploy/slim/quantization/README.md)对模型进行量化训练。
|
||||
|
||||
量化训练可通过如下命令启动:
|
||||
|
||||
```bash
|
||||
python3.7 deploy/slim/quantization/quant.py -c configs/det/ch_PP-OCRv3/ch_PP-OCRv3_det_student.yml -o \
|
||||
Global.pretrained_model=output/CCPD/det/best_accuracy.pdparams \
|
||||
Global.save_model_dir=output/CCPD/det_quant \
|
||||
Global.eval_batch_step="[0, 772]" \
|
||||
Optimizer.lr.name=Const \
|
||||
Optimizer.lr.learning_rate=0.0005 \
|
||||
Optimizer.lr.warmup_epoch=0 \
|
||||
Train.dataset.data_dir=/home/aistudio/data/CCPD2020/ccpd_green \
|
||||
Train.dataset.label_file_list=[/home/aistudio/data/CCPD2020/PPOCR/train/det.txt] \
|
||||
Eval.dataset.data_dir=/home/aistudio/data/CCPD2020/ccpd_green \
|
||||
Eval.dataset.label_file_list=[/home/aistudio/data/CCPD2020/PPOCR/test/det.txt]
|
||||
```
|
||||
|
||||
训练好的模型地址为: [det_ppocr_v3_quant.tar](https://paddleocr.bj.bcebos.com/fanliku/license_plate_recognition/det_ppocr_v3_quant.tar)
|
||||
|
||||
量化后指标对比如下
|
||||
|
||||
|方案|hmeans| 模型大小 |预测速度(lite)|
|
||||
|---|---|------|---|
|
||||
|PP-OCRv3中英文超轻量检测预训练模型 fine-tune|99%| 2.5M | 223ms/image |
|
||||
|PP-OCRv3中英文超轻量检测预训练模型 fine-tune+量化|98.91%| 1M | 189ms/image |
|
||||
|
||||
可以看到量化后能显著降低模型体积并且精度几乎无损。
|
||||
|
||||
预测速度是在android骁龙855上预测275张图像的平均耗时。模型在移动端的部署步骤参考[文档](../deploy/lite/readme_ch.md)
|
||||
|
||||
#### 4.1.4 模型导出
|
||||
|
||||
使用如下命令可以将训练好的模型进行导出
|
||||
|
||||
* 非量化模型
|
||||
```bash
|
||||
python tools/export_model.py -c configs/det/ch_PP-OCRv3/ch_PP-OCRv3_det_student.yml -o \
|
||||
Global.pretrained_model=output/CCPD/det/best_accuracy.pdparams \
|
||||
Global.save_inference_dir=output/det/infer
|
||||
```
|
||||
* 量化模型
|
||||
```bash
|
||||
python deploy/slim/quantization/export_model.py -c configs/det/ch_PP-OCRv3/ch_PP-OCRv3_det_student.yml -o \
|
||||
Global.pretrained_model=output/CCPD/det_quant/best_accuracy.pdparams \
|
||||
Global.save_inference_dir=output/det/infer
|
||||
```
|
||||
|
||||
### 4.2 识别
|
||||
#### 4.2.1 预训练模型直接预测
|
||||
|
||||
从下表中下载PP-OCRv3文本识别预训练模型
|
||||
|
||||
|模型名称|模型简介|配置文件|推理模型大小|下载地址|
|
||||
| --- | --- | --- | --- | --- |
|
||||
|ch_PP-OCRv3_rec|【最新】原始超轻量模型,支持中英文、数字识别|[ch_PP-OCRv3_rec_distillation.yml](https://github.com/PaddlePaddle/PaddleOCR/blob/dygraph/configs/rec/PP-OCRv3/ch_PP-OCRv3_rec_distillation.yml)| 12.4M |[推理模型](https://paddleocr.bj.bcebos.com/PP-OCRv3/chinese/ch_PP-OCRv3_rec_infer.tar) / [训练模型](https://paddleocr.bj.bcebos.com/PP-OCRv3/chinese/ch_PP-OCRv3_rec_train.tar) |
|
||||
|
||||
使用如下命令下载预训练模型
|
||||
|
||||
```bash
|
||||
mkdir models
|
||||
cd models
|
||||
wget https://paddleocr.bj.bcebos.com/PP-OCRv3/chinese/ch_PP-OCRv3_rec_train.tar
|
||||
tar -xf ch_PP-OCRv3_rec_train.tar
|
||||
cd /home/aistudio/PaddleOCR
|
||||
```
|
||||
|
||||
PaddleOCR提供的PP-OCRv3识别模型采用蒸馏训练策略,因此提供的预训练模型中会包含`Teacher`和`Student`模型的参数,详细信息可参考[knowledge_distillation.md](../doc/doc_ch/knowledge_distillation.md)。 因此,模型下载完成后需要使用如下代码提取`Student`模型的参数:
|
||||
|
||||
```python
|
||||
import paddle
|
||||
# 加载预训练模型
|
||||
all_params = paddle.load("models/ch_PP-OCRv3_rec_train/best_accuracy.pdparams")
|
||||
# 查看权重参数的keys
|
||||
print(all_params.keys())
|
||||
# 学生模型的权重提取
|
||||
s_params = {key[len("Student."):]: all_params[key] for key in all_params if "Student." in key}
|
||||
# 查看学生模型权重参数的keys
|
||||
print(s_params.keys())
|
||||
# 保存
|
||||
paddle.save(s_params, "models/ch_PP-OCRv3_rec_train/student.pdparams")
|
||||
```
|
||||
|
||||
预训练模型下载完成后,我们使用[ch_PP-OCRv3_rec.yml](../configs/rec/PP-OCRv3/ch_PP-OCRv3_rec.yml) 配置文件进行后续实验,在开始评估之前需要对配置文件中部分字段进行设置,具体如下:
|
||||
|
||||
1. 模型存储和训练相关:
|
||||
1. Global.pretrained_model: 指向PP-OCRv3文本识别预训练模型地址
|
||||
2. 数据集相关
|
||||
1. Eval.dataset.data_dir:指向测试集图片存放目录
|
||||
2. Eval.dataset.label_file_list:指向测试集标注文件
|
||||
|
||||
使用如下命令进行PP-OCRv3文本识别预训练模型的评估
|
||||
|
||||
```bash
|
||||
python tools/eval.py -c configs/rec/PP-OCRv3/ch_PP-OCRv3_rec.yml -o \
|
||||
Global.pretrained_model=models/ch_PP-OCRv3_rec_train/student.pdparams \
|
||||
Eval.dataset.data_dir=/home/aistudio/data/CCPD2020/PPOCR \
|
||||
Eval.dataset.label_file_list=[/home/aistudio/data/CCPD2020/PPOCR/test/rec.txt]
|
||||
```
|
||||
|
||||
评估部分日志如下:
|
||||
```bash
|
||||
[2022/05/12 19:52:02] ppocr INFO: load pretrain successful from models/ch_PP-OCRv3_rec_train/best_accuracy
|
||||
eval model:: 100%|██████████████████████████████| 40/40 [00:15<00:00, 2.57it/s]
|
||||
[2022/05/12 19:52:17] ppocr INFO: metric eval ***************
|
||||
[2022/05/12 19:52:17] ppocr INFO: acc:0.0
|
||||
[2022/05/12 19:52:17] ppocr INFO: norm_edit_dis:0.8656084923002452
|
||||
[2022/05/12 19:52:17] ppocr INFO: Teacher_acc:0.000399520574511545
|
||||
[2022/05/12 19:52:17] ppocr INFO: Teacher_norm_edit_dis:0.8657902943394548
|
||||
[2022/05/12 19:52:17] ppocr INFO: fps:1443.1801978719905
|
||||
|
||||
```
|
||||
使用预训练模型进行评估,指标如下所示:
|
||||
|
||||
|方案|acc|
|
||||
|---|---|
|
||||
|PP-OCRv3中英文超轻量识别预训练模型直接预测|0%|
|
||||
|
||||
从评估日志中可以看到,直接使用PP-OCRv3预训练模型进行评估,acc非常低,但是norm_edit_dis很高。因此,我们猜测是模型大部分文字识别是对的,只有少部分文字识别错误。使用如下命令进行infer查看模型的推理结果进行验证:
|
||||
|
||||
|
||||
```bash
|
||||
python tools/infer_rec.py -c configs/rec/PP-OCRv3/ch_PP-OCRv3_rec.yml -o \
|
||||
Global.pretrained_model=models/ch_PP-OCRv3_rec_train/student.pdparams \
|
||||
Global.infer_img=/home/aistudio/data/CCPD2020/PPOCR/test/crop_imgs/0_0_0_3_32_30_31_30_30.jpg
|
||||
```
|
||||
|
||||
输出部分日志如下:
|
||||
```bash
|
||||
[2022/05/01 08:51:57] ppocr INFO: train with paddle 2.2.2 and device CUDAPlace(0)
|
||||
W0501 08:51:57.127391 11326 device_context.cc:447] Please NOTE: device: 0, GPU Compute Capability: 7.0, Driver API Version: 11.0, Runtime API Version: 10.1
|
||||
W0501 08:51:57.132315 11326 device_context.cc:465] device: 0, cuDNN Version: 7.6.
|
||||
[2022/05/01 08:52:00] ppocr INFO: load pretrain successful from models/ch_PP-OCRv3_rec_train/student
|
||||
[2022/05/01 08:52:00] ppocr INFO: infer_img: /home/aistudio/data/CCPD2020/PPOCR/test/crop_imgs/0_0_3_32_30_31_30_30.jpg
|
||||
[2022/05/01 08:52:00] ppocr INFO: result: {"Student": {"label": "皖A·D86766", "score": 0.9552637934684753}, "Teacher": {"label": "皖A·D86766", "score": 0.9917094707489014}}
|
||||
[2022/05/01 08:52:00] ppocr INFO: success!
|
||||
```
|
||||
|
||||
从infer结果可以看到,车牌中的文字大部分都识别正确,只是多识别出了一个`·`。针对这种情况,有如下两种方案:
|
||||
1. 直接通过后处理去掉多识别的`·`。
|
||||
2. 进行finetune。
|
||||
|
||||
#### 4.2.2 预训练模型直接预测+改动后处理
|
||||
|
||||
直接通过后处理去掉多识别的`·`,在后处理的改动比较简单,只需在 [ppocr/postprocess/rec_postprocess.py](../ppocr/postprocess/rec_postprocess.py) 文件的76行添加如下代码:
|
||||
```python
|
||||
text = text.replace('·','')
|
||||
```
|
||||
|
||||
改动前后指标对比:
|
||||
|
||||
|方案|acc|
|
||||
|---|---|
|
||||
|PP-OCRv3中英文超轻量识别预训练模型直接预测|0.2%|
|
||||
|PP-OCRv3中英文超轻量识别预训练模型直接预测+后处理去掉多识别的`·`|90.97%|
|
||||
|
||||
可以看到,去掉多余的`·`能大幅提高精度。
|
||||
|
||||
#### 4.2.3 CCPD车牌数据集fine-tune
|
||||
|
||||
**训练**
|
||||
|
||||
为了进行fine-tune训练,我们需要在配置文件中设置需要使用的预训练模型地址,学习率和数据集等参数。 具体如下:
|
||||
|
||||
1. 模型存储和训练相关:
|
||||
1. Global.pretrained_model: 指向PP-OCRv3文本识别预训练模型地址
|
||||
2. Global.eval_batch_step: 模型多少step评估一次,这里设为从第0个step开始没隔45个step评估一次,45为一个epoch总的step数。
|
||||
2. 优化器相关
|
||||
1. Optimizer.lr.name: 学习率衰减器设为常量 Const
|
||||
2. Optimizer.lr.learning_rate: 做finetune实验,学习率需要设置的比较小,此处学习率设为配置文件中的0.05倍
|
||||
3. Optimizer.lr.warmup_epoch: warmup_epoch设为0
|
||||
3. 数据集相关
|
||||
1. Train.dataset.data_dir:指向训练集图片存放目录
|
||||
2. Train.dataset.label_file_list:指向训练集标注文件
|
||||
3. Eval.dataset.data_dir:指向测试集图片存放目录
|
||||
4. Eval.dataset.label_file_list:指向测试集标注文件
|
||||
|
||||
使用如下命令启动finetune
|
||||
|
||||
```bash
|
||||
python tools/train.py -c configs/rec/PP-OCRv3/ch_PP-OCRv3_rec.yml -o \
|
||||
Global.pretrained_model=models/ch_PP-OCRv3_rec_train/student.pdparams \
|
||||
Global.save_model_dir=output/CCPD/rec/ \
|
||||
Global.eval_batch_step="[0, 90]" \
|
||||
Optimizer.lr.name=Const \
|
||||
Optimizer.lr.learning_rate=0.0005 \
|
||||
Optimizer.lr.warmup_epoch=0 \
|
||||
Train.dataset.data_dir=/home/aistudio/data/CCPD2020/PPOCR \
|
||||
Train.dataset.label_file_list=[/home/aistudio/data/CCPD2020/PPOCR/train/rec.txt] \
|
||||
Eval.dataset.data_dir=/home/aistudio/data/CCPD2020/PPOCR \
|
||||
Eval.dataset.label_file_list=[/home/aistudio/data/CCPD2020/PPOCR/test/rec.txt]
|
||||
```
|
||||
训练好的模型地址为: [rec_ppocr_v3_finetune.tar](https://paddleocr.bj.bcebos.com/fanliku/license_plate_recognition/rec_ppocr_v3_finetune.tar)
|
||||
|
||||
**评估**
|
||||
|
||||
训练完成后使用如下命令进行评估
|
||||
|
||||
```bash
|
||||
python tools/eval.py -c configs/rec/PP-OCRv3/ch_PP-OCRv3_rec.yml -o \
|
||||
Global.pretrained_model=output/CCPD/rec/best_accuracy.pdparams \
|
||||
Eval.dataset.data_dir=/home/aistudio/data/CCPD2020/PPOCR \
|
||||
Eval.dataset.label_file_list=[/home/aistudio/data/CCPD2020/PPOCR/test/rec.txt]
|
||||
```
|
||||
|
||||
使用预训练模型和CCPD车牌数据集fine-tune,指标分别如下:
|
||||
|
||||
|方案| acc |
|
||||
|---|--------|
|
||||
|PP-OCRv3中英文超轻量识别预训练模型直接预测| 0% |
|
||||
|PP-OCRv3中英文超轻量识别预训练模型直接预测+后处理去掉多识别的`·`| 90.97% |
|
||||
|PP-OCRv3中英文超轻量识别预训练模型 fine-tune| 94.54% |
|
||||
|
||||
可以看到进行fine-tune能显著提升车牌识别的效果。
|
||||
|
||||
#### 4.2.4 CCPD车牌数据集fine-tune+量化训练
|
||||
|
||||
此处采用 PaddleOCR 中提供好的[量化教程](../deploy/slim/quantization/README.md)对模型进行量化训练。
|
||||
|
||||
量化训练可通过如下命令启动:
|
||||
|
||||
```bash
|
||||
python3.7 deploy/slim/quantization/quant.py -c configs/rec/PP-OCRv3/ch_PP-OCRv3_rec.yml -o \
|
||||
Global.pretrained_model=output/CCPD/rec/best_accuracy.pdparams \
|
||||
Global.save_model_dir=output/CCPD/rec_quant/ \
|
||||
Global.eval_batch_step="[0, 90]" \
|
||||
Optimizer.lr.name=Const \
|
||||
Optimizer.lr.learning_rate=0.0005 \
|
||||
Optimizer.lr.warmup_epoch=0 \
|
||||
Train.dataset.data_dir=/home/aistudio/data/CCPD2020/PPOCR \
|
||||
Train.dataset.label_file_list=[/home/aistudio/data/CCPD2020/PPOCR/train/rec.txt] \
|
||||
Eval.dataset.data_dir=/home/aistudio/data/CCPD2020/PPOCR \
|
||||
Eval.dataset.label_file_list=[/home/aistudio/data/CCPD2020/PPOCR/test/rec.txt]
|
||||
```
|
||||
训练好的模型地址为: [rec_ppocr_v3_quant.tar](https://paddleocr.bj.bcebos.com/fanliku/license_plate_recognition/rec_ppocr_v3_quant.tar)
|
||||
|
||||
量化后指标对比如下
|
||||
|
||||
|方案| acc | 模型大小 |预测速度(lite)|
|
||||
|---|--------|-------|---|
|
||||
|PP-OCRv3中英文超轻量识别预训练模型 fine-tune| 94.54% | 10.3M | 4.2ms/image |
|
||||
|PP-OCRv3中英文超轻量识别预训练模型 fine-tune + 量化| 93.4% | 4.8M | 1.8ms/image; |
|
||||
|
||||
可以看到量化后能显著降低模型体积,但是由于识别数据过少,量化带来了1%的精度下降。
|
||||
|
||||
预测速度是在android骁龙855上预测5006张识别文字图像的平均耗时。模型在移动端的部署步骤参考[文档](../deploy/lite/readme_ch.md)
|
||||
|
||||
#### 4.2.5 模型导出
|
||||
|
||||
使用如下命令可以将训练好的模型进行导出。
|
||||
|
||||
* 非量化模型
|
||||
```bash
|
||||
python tools/export_model.py -c configs/rec/PP-OCRv3/ch_PP-OCRv3_rec.yml -o \
|
||||
Global.pretrained_model=output/CCPD/rec/best_accuracy.pdparams \
|
||||
Global.save_inference_dir=output/CCPD/rec/infer
|
||||
```
|
||||
* 量化模型
|
||||
```bash
|
||||
python deploy/slim/quantization/export_model.py -c configs/rec/PP-OCRv3/ch_PP-OCRv3_rec.yml -o \
|
||||
Global.pretrained_model=output/CCPD/rec_quant/best_accuracy.pdparams \
|
||||
Global.save_inference_dir=output/CCPD/rec_quant/infer
|
||||
```
|
||||
|
||||
### 4.3 串联推理
|
||||
|
||||
检测模型和识别模型分别fine-tune并导出为inference模型之后,可以使用如下命令进行端到端推理并对结果进行可视化。
|
||||
|
||||
|
||||
```bash
|
||||
python tools/infer/predict_system.py \
|
||||
--det_model_dir=output/CCPD/det/infer/ \
|
||||
--rec_model_dir=output/CCPD/rec/infer/ \
|
||||
--image_dir="/home/aistudio/data/CCPD2020/ccpd_green/test/04131106321839081-92_258-159&509_530&611-527&611_172&599_159&509_530&525-0_0_3_32_30_31_30_30-109-106.jpg" \
|
||||
--rec_image_shape=3,48,320
|
||||
```
|
||||
推理结果如下
|
||||
|
||||
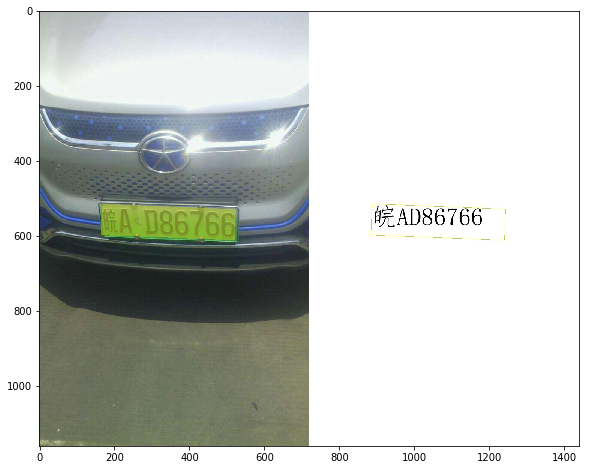
|
||||
|
||||
|
||||
### 4.4 实验总结
|
||||
|
||||
我们分别使用PP-OCRv3中英文超轻量预训练模型在车牌数据集上进行了直接评估和finetune 和finetune+量化3种方案的实验,指标对比如下:
|
||||
|
||||
- 检测
|
||||
|
||||
|方案|hmeans| 模型大小 |预测速度(lite)|
|
||||
|---|---|------|---|
|
||||
|PP-OCRv3中英文超轻量检测预训练模型直接预测|76.12%|2.5M| 223ms/image |
|
||||
|PP-OCRv3中英文超轻量检测预训练模型 fine-tune|99%| 2.5M | 223ms/image |
|
||||
|PP-OCRv3中英文超轻量检测预训练模型 fine-tune+量化|98.91%| 1M | 189ms/image |
|
||||
|
||||
预测速度是在android骁龙855上预测275张图像的平均耗时。
|
||||
|
||||
- 识别
|
||||
|
||||
|方案| acc | 模型大小 |预测速度(lite)|
|
||||
|---|--------|-------|---|
|
||||
|PP-OCRv3中英文超轻量识别预训练模型直接预测| 0% |10.3M| 4.2ms/image |
|
||||
|PP-OCRv3中英文超轻量识别预训练模型直接预测+后处理去掉多识别的`·`| 90.97% |10.3M| 4.2ms/image |
|
||||
|PP-OCRv3中英文超轻量识别预训练模型 fine-tune| 94.54% | 10.3M | 4.2ms/image |
|
||||
|PP-OCRv3中英文超轻量识别预训练模型 fine-tune + 量化| 94.4% | 4.8M | 1.8ms/image |
|
||||
|
||||
预测速度是在android骁龙855上预测5006张识别文字图像的平均耗时。
|
||||
|
||||
- 结论
|
||||
|
||||
PP-OCRv3的检测模型在未经过fine-tune的情况下,在车牌数据集上也有一定的精度,经过finetune后能够极大的提升检测效果,精度达到99%。在使用量化训练后检测模型的精度几乎无损,并且模型大小压缩60%。
|
||||
|
||||
PP-OCRv3的识别模型在未经过fine-tune的情况下,在车牌数据集上精度为0,但是经过分析可以知道,模型大部分字符都预测正确,但是会多预测一个特殊字符,去掉这个特殊字符后,精度达到90%。PP-OCRv3识别模型在经过finetune后识别精度进一步提升,达到94.4%。在使用量化训练后识别模型大小压缩53%,但是由于数据量多少,带来了1%的精度损失。
|
File diff suppressed because one or more lines are too long
|
@ -63,8 +63,7 @@ Train:
|
|||
- DecodeImage:
|
||||
img_mode: BGR
|
||||
channel_first: false
|
||||
- RecAug:
|
||||
use_tia: False
|
||||
- BaseDataAugmentation:
|
||||
- RandAugment:
|
||||
- SSLRotateResize:
|
||||
image_shape: [3, 48, 320]
|
||||
|
|
|
@ -60,8 +60,7 @@ Train:
|
|||
img_mode: BGR
|
||||
channel_first: False
|
||||
- ClsLabelEncode: # Class handling label
|
||||
- RecAug:
|
||||
use_tia: False
|
||||
- BaseDataAugmentation:
|
||||
- RandAugment:
|
||||
- ClsResizeImg:
|
||||
image_shape: [3, 48, 192]
|
||||
|
|
|
@ -92,6 +92,8 @@ include_directories("${PADDLE_LIB}/third_party/install/glog/include")
|
|||
include_directories("${PADDLE_LIB}/third_party/install/gflags/include")
|
||||
include_directories("${PADDLE_LIB}/third_party/install/xxhash/include")
|
||||
include_directories("${PADDLE_LIB}/third_party/install/zlib/include")
|
||||
include_directories("${PADDLE_LIB}/third_party/install/onnxruntime/include")
|
||||
include_directories("${PADDLE_LIB}/third_party/install/paddle2onnx/include")
|
||||
include_directories("${PADDLE_LIB}/third_party/boost")
|
||||
include_directories("${PADDLE_LIB}/third_party/eigen3")
|
||||
|
||||
|
@ -110,6 +112,8 @@ link_directories("${PADDLE_LIB}/third_party/install/protobuf/lib")
|
|||
link_directories("${PADDLE_LIB}/third_party/install/glog/lib")
|
||||
link_directories("${PADDLE_LIB}/third_party/install/gflags/lib")
|
||||
link_directories("${PADDLE_LIB}/third_party/install/xxhash/lib")
|
||||
link_directories("${PADDLE_LIB}/third_party/install/onnxruntime/lib")
|
||||
link_directories("${PADDLE_LIB}/third_party/install/paddle2onnx/lib")
|
||||
link_directories("${PADDLE_LIB}/paddle/lib")
|
||||
|
||||
|
||||
|
|
|
@ -208,7 +208,7 @@ Execute the built executable file:
|
|||
./build/ppocr [--param1] [--param2] [...]
|
||||
```
|
||||
|
||||
**Note**:ppocr uses the `PP-OCRv3` model by default, and the input shape used by the recognition model is `3, 48, 320`, so if you use the recognition function, you need to add the parameter `--rec_img_h=48`, if you do not use the default `PP-OCRv3` model, you do not need to set this parameter.
|
||||
**Note**:ppocr uses the `PP-OCRv3` model by default, and the input shape used by the recognition model is `3, 48, 320`, if you want to use the old version model, you should add the parameter `--rec_img_h=32`.
|
||||
|
||||
Specifically,
|
||||
|
||||
|
@ -222,7 +222,6 @@ Specifically,
|
|||
--det=true \
|
||||
--rec=true \
|
||||
--cls=true \
|
||||
--rec_img_h=48\
|
||||
```
|
||||
|
||||
##### 2. det+rec:
|
||||
|
@ -234,7 +233,6 @@ Specifically,
|
|||
--det=true \
|
||||
--rec=true \
|
||||
--cls=false \
|
||||
--rec_img_h=48\
|
||||
```
|
||||
|
||||
##### 3. det
|
||||
|
@ -254,7 +252,6 @@ Specifically,
|
|||
--det=false \
|
||||
--rec=true \
|
||||
--cls=true \
|
||||
--rec_img_h=48\
|
||||
```
|
||||
|
||||
##### 5. rec
|
||||
|
@ -265,7 +262,6 @@ Specifically,
|
|||
--det=false \
|
||||
--rec=true \
|
||||
--cls=false \
|
||||
--rec_img_h=48\
|
||||
```
|
||||
|
||||
##### 6. cls
|
||||
|
@ -330,7 +326,7 @@ More parameters are as follows,
|
|||
|rec_model_dir|string|-|Address of recognition inference model|
|
||||
|rec_char_dict_path|string|../../ppocr/utils/ppocr_keys_v1.txt|dictionary file|
|
||||
|rec_batch_num|int|6|batch size of recognition|
|
||||
|rec_img_h|int|32|image height of recognition|
|
||||
|rec_img_h|int|48|image height of recognition|
|
||||
|rec_img_w|int|320|image width of recognition|
|
||||
|
||||
* Multi-language inference is also supported in PaddleOCR, you can refer to [recognition tutorial](../../doc/doc_en/recognition_en.md) for more supported languages and models in PaddleOCR. Specifically, if you want to infer using multi-language models, you just need to modify values of `rec_char_dict_path` and `rec_model_dir`.
|
||||
|
|
|
@ -213,7 +213,7 @@ CUDNN_LIB_DIR=/your_cudnn_lib_dir
|
|||
|
||||
本demo支持系统串联调用,也支持单个功能的调用,如,只使用检测或识别功能。
|
||||
|
||||
**注意** ppocr默认使用`PP-OCRv3`模型,识别模型使用的输入shape为`3,48,320`, 因此如果使用识别功能,需要添加参数`--rec_img_h=48`,如果不使用默认的`PP-OCRv3`模型,则无需设置该参数。
|
||||
**注意** ppocr默认使用`PP-OCRv3`模型,识别模型使用的输入shape为`3,48,320`, 如需使用旧版本的PP-OCR模型,则需要设置参数`--rec_img_h=32`。
|
||||
|
||||
|
||||
运行方式:
|
||||
|
@ -232,7 +232,6 @@ CUDNN_LIB_DIR=/your_cudnn_lib_dir
|
|||
--det=true \
|
||||
--rec=true \
|
||||
--cls=true \
|
||||
--rec_img_h=48\
|
||||
```
|
||||
|
||||
##### 2. 检测+识别:
|
||||
|
@ -244,7 +243,6 @@ CUDNN_LIB_DIR=/your_cudnn_lib_dir
|
|||
--det=true \
|
||||
--rec=true \
|
||||
--cls=false \
|
||||
--rec_img_h=48\
|
||||
```
|
||||
|
||||
##### 3. 检测:
|
||||
|
@ -264,7 +262,6 @@ CUDNN_LIB_DIR=/your_cudnn_lib_dir
|
|||
--det=false \
|
||||
--rec=true \
|
||||
--cls=true \
|
||||
--rec_img_h=48\
|
||||
```
|
||||
|
||||
##### 5. 识别:
|
||||
|
@ -275,7 +272,6 @@ CUDNN_LIB_DIR=/your_cudnn_lib_dir
|
|||
--det=false \
|
||||
--rec=true \
|
||||
--cls=false \
|
||||
--rec_img_h=48\
|
||||
```
|
||||
|
||||
##### 6. 分类:
|
||||
|
@ -339,7 +335,7 @@ CUDNN_LIB_DIR=/your_cudnn_lib_dir
|
|||
|rec_model_dir|string|-|识别模型inference model地址|
|
||||
|rec_char_dict_path|string|../../ppocr/utils/ppocr_keys_v1.txt|字典文件|
|
||||
|rec_batch_num|int|6|识别模型batchsize|
|
||||
|rec_img_h|int|32|识别模型输入图像高度|
|
||||
|rec_img_h|int|48|识别模型输入图像高度|
|
||||
|rec_img_w|int|320|识别模型输入图像宽度|
|
||||
|
||||
|
||||
|
|
|
@ -47,7 +47,7 @@ DEFINE_string(rec_model_dir, "", "Path of rec inference model.");
|
|||
DEFINE_int32(rec_batch_num, 6, "rec_batch_num.");
|
||||
DEFINE_string(rec_char_dict_path, "../../ppocr/utils/ppocr_keys_v1.txt",
|
||||
"Path of dictionary.");
|
||||
DEFINE_int32(rec_img_h, 32, "rec image height");
|
||||
DEFINE_int32(rec_img_h, 48, "rec image height");
|
||||
DEFINE_int32(rec_img_w, 320, "rec image width");
|
||||
|
||||
// ocr forward related
|
||||
|
|
|
@ -83,7 +83,7 @@ void CRNNRecognizer::Run(std::vector<cv::Mat> img_list,
|
|||
int out_num = std::accumulate(predict_shape.begin(), predict_shape.end(), 1,
|
||||
std::multiplies<int>());
|
||||
predict_batch.resize(out_num);
|
||||
|
||||
// predict_batch is the result of Last FC with softmax
|
||||
output_t->CopyToCpu(predict_batch.data());
|
||||
auto inference_end = std::chrono::steady_clock::now();
|
||||
inference_diff += inference_end - inference_start;
|
||||
|
@ -98,9 +98,11 @@ void CRNNRecognizer::Run(std::vector<cv::Mat> img_list,
|
|||
float max_value = 0.0f;
|
||||
|
||||
for (int n = 0; n < predict_shape[1]; n++) {
|
||||
// get idx
|
||||
argmax_idx = int(Utility::argmax(
|
||||
&predict_batch[(m * predict_shape[1] + n) * predict_shape[2]],
|
||||
&predict_batch[(m * predict_shape[1] + n + 1) * predict_shape[2]]));
|
||||
// get score
|
||||
max_value = float(*std::max_element(
|
||||
&predict_batch[(m * predict_shape[1] + n) * predict_shape[2]],
|
||||
&predict_batch[(m * predict_shape[1] + n + 1) * predict_shape[2]]));
|
||||
|
@ -132,7 +134,9 @@ void CRNNRecognizer::LoadModel(const std::string &model_dir) {
|
|||
paddle_infer::Config config;
|
||||
config.SetModel(model_dir + "/inference.pdmodel",
|
||||
model_dir + "/inference.pdiparams");
|
||||
|
||||
std::cout << "In PP-OCRv3, default rec_img_h is 48,"
|
||||
<< "if you use other model, you should set the param rec_img_h=32"
|
||||
<< std::endl;
|
||||
if (this->use_gpu_) {
|
||||
config.EnableUseGpu(this->gpu_mem_, this->gpu_id_);
|
||||
if (this->use_tensorrt_) {
|
||||
|
|
|
@ -136,7 +136,7 @@ The recognition model is the same.
|
|||
2. Run the following command to start the service.
|
||||
```
|
||||
# Start the service and save the running log in log.txt
|
||||
python3 web_service.py &>log.txt &
|
||||
python3 web_service.py --config=config.yml &>log.txt &
|
||||
```
|
||||
After the service is successfully started, a log similar to the following will be printed in log.txt
|
||||

|
||||
|
@ -217,7 +217,7 @@ The C++ service deployment is the same as python in the environment setup and da
|
|||
2. Run the following command to start the service.
|
||||
```
|
||||
# Start the service and save the running log in log.txt
|
||||
python3 -m paddle_serving_server.serve --model ppocr_det_v3_serving ppocr_rec_v3_serving --op GeneralDetectionOp GeneralInferOp --port 9293 &>log.txt &
|
||||
python3 -m paddle_serving_server.serve --model ppocr_det_v3_serving ppocr_rec_v3_serving --op GeneralDetectionOp GeneralInferOp --port 8181 &>log.txt &
|
||||
```
|
||||
After the service is successfully started, a log similar to the following will be printed in log.txt
|
||||

|
||||
|
|
|
@ -135,7 +135,7 @@ python3 -m paddle_serving_client.convert --dirname ./ch_PP-OCRv3_rec_infer/ \
|
|||
2. 启动服务可运行如下命令:
|
||||
```
|
||||
# 启动服务,运行日志保存在log.txt
|
||||
python3 web_service.py &>log.txt &
|
||||
python3 web_service.py --config=config.yml &>log.txt &
|
||||
```
|
||||
成功启动服务后,log.txt中会打印类似如下日志
|
||||

|
||||
|
@ -230,7 +230,7 @@ cp -rf general_detection_op.cpp Serving/core/general-server/op
|
|||
|
||||
```
|
||||
# 启动服务,运行日志保存在log.txt
|
||||
python3 -m paddle_serving_server.serve --model ppocr_det_v3_serving ppocr_rec_v3_serving --op GeneralDetectionOp GeneralInferOp --port 9293 &>log.txt &
|
||||
python3 -m paddle_serving_server.serve --model ppocr_det_v3_serving ppocr_rec_v3_serving --op GeneralDetectionOp GeneralInferOp --port 8181 &>log.txt &
|
||||
```
|
||||
成功启动服务后,log.txt中会打印类似如下日志
|
||||

|
||||
|
|
|
@ -22,15 +22,16 @@ import cv2
|
|||
from paddle_serving_app.reader import Sequential, URL2Image, ResizeByFactor
|
||||
from paddle_serving_app.reader import Div, Normalize, Transpose
|
||||
from ocr_reader import OCRReader
|
||||
import codecs
|
||||
|
||||
client = Client()
|
||||
# TODO:load_client need to load more than one client model.
|
||||
# this need to figure out some details.
|
||||
client.load_client_config(sys.argv[1:])
|
||||
client.connect(["127.0.0.1:9293"])
|
||||
client.connect(["127.0.0.1:8181"])
|
||||
|
||||
import paddle
|
||||
test_img_dir = "../../doc/imgs/"
|
||||
test_img_dir = "../../doc/imgs/1.jpg"
|
||||
|
||||
ocr_reader = OCRReader(char_dict_path="../../ppocr/utils/ppocr_keys_v1.txt")
|
||||
|
||||
|
@ -40,14 +41,43 @@ def cv2_to_base64(image):
|
|||
'utf8') #data.tostring()).decode('utf8')
|
||||
|
||||
|
||||
for img_file in os.listdir(test_img_dir):
|
||||
with open(os.path.join(test_img_dir, img_file), 'rb') as file:
|
||||
def _check_image_file(path):
|
||||
img_end = {'jpg', 'bmp', 'png', 'jpeg', 'rgb', 'tif', 'tiff', 'gif'}
|
||||
return any([path.lower().endswith(e) for e in img_end])
|
||||
|
||||
|
||||
test_img_list = []
|
||||
if os.path.isfile(test_img_dir) and _check_image_file(test_img_dir):
|
||||
test_img_list.append(test_img_dir)
|
||||
elif os.path.isdir(test_img_dir):
|
||||
for single_file in os.listdir(test_img_dir):
|
||||
file_path = os.path.join(test_img_dir, single_file)
|
||||
if os.path.isfile(file_path) and _check_image_file(file_path):
|
||||
test_img_list.append(file_path)
|
||||
if len(test_img_list) == 0:
|
||||
raise Exception("not found any img file in {}".format(test_img_dir))
|
||||
|
||||
for img_file in test_img_list:
|
||||
with open(img_file, 'rb') as file:
|
||||
image_data = file.read()
|
||||
image = cv2_to_base64(image_data)
|
||||
res_list = []
|
||||
fetch_map = client.predict(feed={"x": image}, fetch=[], batch=True)
|
||||
one_batch_res = ocr_reader.postprocess(fetch_map, with_score=True)
|
||||
for res in one_batch_res:
|
||||
res_list.append(res[0])
|
||||
res = {"res": str(res_list)}
|
||||
print(res)
|
||||
if fetch_map is None:
|
||||
print('no results')
|
||||
else:
|
||||
if "text" in fetch_map:
|
||||
for x in fetch_map["text"]:
|
||||
x = codecs.encode(x)
|
||||
words = base64.b64decode(x).decode('utf-8')
|
||||
res_list.append(words)
|
||||
else:
|
||||
try:
|
||||
one_batch_res = ocr_reader.postprocess(
|
||||
fetch_map, with_score=True)
|
||||
for res in one_batch_res:
|
||||
res_list.append(res[0])
|
||||
except:
|
||||
print('no results')
|
||||
res = {"res": str(res_list)}
|
||||
print(res)
|
||||
|
|
|
@ -339,7 +339,7 @@ class CharacterOps(object):
|
|||
class OCRReader(object):
|
||||
def __init__(self,
|
||||
algorithm="CRNN",
|
||||
image_shape=[3, 32, 320],
|
||||
image_shape=[3, 48, 320],
|
||||
char_type="ch",
|
||||
batch_num=1,
|
||||
char_dict_path="./ppocr_keys_v1.txt"):
|
||||
|
@ -356,7 +356,7 @@ class OCRReader(object):
|
|||
def resize_norm_img(self, img, max_wh_ratio):
|
||||
imgC, imgH, imgW = self.rec_image_shape
|
||||
if self.character_type == "ch":
|
||||
imgW = int(32 * max_wh_ratio)
|
||||
imgW = int(imgH * max_wh_ratio)
|
||||
h = img.shape[0]
|
||||
w = img.shape[1]
|
||||
ratio = w / float(h)
|
||||
|
@ -377,7 +377,7 @@ class OCRReader(object):
|
|||
def preprocess(self, img_list):
|
||||
img_num = len(img_list)
|
||||
norm_img_batch = []
|
||||
max_wh_ratio = 0
|
||||
max_wh_ratio = 320/48.
|
||||
for ino in range(img_num):
|
||||
h, w = img_list[ino].shape[0:2]
|
||||
wh_ratio = w * 1.0 / h
|
||||
|
|
|
@ -36,11 +36,27 @@ def cv2_to_base64(image):
|
|||
return base64.b64encode(image).decode('utf8')
|
||||
|
||||
|
||||
def _check_image_file(path):
|
||||
img_end = {'jpg', 'bmp', 'png', 'jpeg', 'rgb', 'tif', 'tiff', 'gif'}
|
||||
return any([path.lower().endswith(e) for e in img_end])
|
||||
|
||||
|
||||
url = "http://127.0.0.1:9998/ocr/prediction"
|
||||
test_img_dir = args.image_dir
|
||||
|
||||
for idx, img_file in enumerate(os.listdir(test_img_dir)):
|
||||
with open(os.path.join(test_img_dir, img_file), 'rb') as file:
|
||||
test_img_list = []
|
||||
if os.path.isfile(test_img_dir) and _check_image_file(test_img_dir):
|
||||
test_img_list.append(test_img_dir)
|
||||
elif os.path.isdir(test_img_dir):
|
||||
for single_file in os.listdir(test_img_dir):
|
||||
file_path = os.path.join(test_img_dir, single_file)
|
||||
if os.path.isfile(file_path) and _check_image_file(file_path):
|
||||
test_img_list.append(file_path)
|
||||
if len(test_img_list) == 0:
|
||||
raise Exception("not found any img file in {}".format(test_img_dir))
|
||||
|
||||
for idx, img_file in enumerate(test_img_list):
|
||||
with open(img_file, 'rb') as file:
|
||||
image_data1 = file.read()
|
||||
# print file name
|
||||
print('{}{}{}'.format('*' * 10, img_file, '*' * 10))
|
||||
|
@ -70,4 +86,4 @@ for idx, img_file in enumerate(os.listdir(test_img_dir)):
|
|||
print(
|
||||
"For details about error message, see PipelineServingLogs/pipeline.log"
|
||||
)
|
||||
print("==> total number of test imgs: ", len(os.listdir(test_img_dir)))
|
||||
print("==> total number of test imgs: ", len(test_img_list))
|
||||
|
|
|
@ -0,0 +1,16 @@
|
|||
feed_var {
|
||||
name: "x"
|
||||
alias_name: "x"
|
||||
is_lod_tensor: false
|
||||
feed_type: 20
|
||||
shape: 1
|
||||
}
|
||||
fetch_var {
|
||||
name: "save_infer_model/scale_0.tmp_1"
|
||||
alias_name: "save_infer_model/scale_0.tmp_1"
|
||||
is_lod_tensor: false
|
||||
fetch_type: 1
|
||||
shape: 1
|
||||
shape: 640
|
||||
shape: 640
|
||||
}
|
|
@ -19,7 +19,7 @@ import copy
|
|||
import cv2
|
||||
import base64
|
||||
# from paddle_serving_app.reader import OCRReader
|
||||
from ocr_reader import OCRReader, DetResizeForTest
|
||||
from ocr_reader import OCRReader, DetResizeForTest, ArgsParser
|
||||
from paddle_serving_app.reader import Sequential, ResizeByFactor
|
||||
from paddle_serving_app.reader import Div, Normalize, Transpose
|
||||
from paddle_serving_app.reader import DBPostProcess, FilterBoxes, GetRotateCropImage, SortedBoxes
|
||||
|
@ -63,7 +63,6 @@ class DetOp(Op):
|
|||
dt_boxes_list = self.post_func(det_out, [ratio_list])
|
||||
dt_boxes = self.filter_func(dt_boxes_list[0], [self.ori_h, self.ori_w])
|
||||
out_dict = {"dt_boxes": dt_boxes, "image": self.raw_im}
|
||||
|
||||
return out_dict, None, ""
|
||||
|
||||
|
||||
|
@ -86,7 +85,7 @@ class RecOp(Op):
|
|||
dt_boxes = copy.deepcopy(self.dt_list)
|
||||
feed_list = []
|
||||
img_list = []
|
||||
max_wh_ratio = 0
|
||||
max_wh_ratio = 320 / 48.
|
||||
## Many mini-batchs, the type of feed_data is list.
|
||||
max_batch_size = 6 # len(dt_boxes)
|
||||
|
||||
|
@ -150,7 +149,8 @@ class RecOp(Op):
|
|||
for i in range(dt_num):
|
||||
text = rec_list[i]
|
||||
dt_box = self.dt_list[i]
|
||||
result_list.append([text, dt_box.tolist()])
|
||||
if text[1] >= 0.5:
|
||||
result_list.append([text, dt_box.tolist()])
|
||||
res = {"result": str(result_list)}
|
||||
return res, None, ""
|
||||
|
||||
|
@ -163,5 +163,6 @@ class OcrService(WebService):
|
|||
|
||||
|
||||
uci_service = OcrService(name="ocr")
|
||||
uci_service.prepare_pipeline_config("config.yml")
|
||||
FLAGS = ArgsParser().parse_args()
|
||||
uci_service.prepare_pipeline_config(yml_dict=FLAGS.conf_dict)
|
||||
uci_service.run_service()
|
||||
|
|
|
@ -682,7 +682,7 @@ lr:
|
|||
|
||||
#### Q: 关于dygraph分支中,文本识别模型训练,要使用数据增强应该如何设置?
|
||||
|
||||
**A**:可以参考[配置文件](../../configs/rec/ch_ppocr_v2.0/rec_chinese_lite_train_v2.0.yml)在Train['dataset']['transforms']添加RecAug字段,使数据增强生效。可以通过添加对aug_prob设置,表示每种数据增强采用的概率。aug_prob默认是0.4.由于tia数据增强特殊性,默认不采用,可以通过添加use_tia设置,使tia数据增强生效。详细设置可以参考[ISSUE 1744](https://github.com/PaddlePaddle/PaddleOCR/issues/1744)。
|
||||
**A**:可以参考[配置文件](../../configs/rec/ch_ppocr_v2.0/rec_chinese_lite_train_v2.0.yml)在Train['dataset']['transforms']添加RecAug字段,使数据增强生效。可以通过添加对aug_prob设置,表示每种数据增强采用的概率。aug_prob默认是0.4。详细设置可以参考[ISSUE 1744](https://github.com/PaddlePaddle/PaddleOCR/issues/1744)。
|
||||
|
||||
#### Q: 训练过程中,训练程序意外退出/挂起,应该如何解决?
|
||||
|
||||
|
@ -720,6 +720,13 @@ C++TensorRT预测需要使用支持TRT的预测库并在编译时打开[-DWITH_T
|
|||
|
||||
注:建议使用TensorRT大于等于6.1.0.5以上的版本。
|
||||
|
||||
#### Q: 为什么识别模型做预测的时候,预测图片的数量数量还会影响预测的精度
|
||||
**A**: 推理时识别模型默认的batch_size=6, 如预测图片长度变化大,可能影响预测效果。如果出现上述问题可在推理的时候设置识别bs=1,命令如下:
|
||||
|
||||
```
|
||||
python3 tools/infer/predict_rec.py --image_dir="./doc/imgs_words/ch/word_4.jpg" --rec_model_dir="./ch_PP-OCRv3_rec_infer/" --rec_batch_num=1
|
||||
```
|
||||
|
||||
<a name="213"></a>
|
||||
|
||||
### 2.13 推理部署
|
||||
|
|
|
@ -15,8 +15,8 @@
|
|||
- **数据简介**:publaynet数据集的训练集合中包含35万张图像,验证集合中包含1.1万张图像。总共包含5个类别,分别是: `text, title, list, table, figure`。部分图像以及标注框可视化如下所示。
|
||||
|
||||
<div align="center">
|
||||
<img src="../datasets/publaynet_demo/gt_PMC3724501_00006.jpg" width="500">
|
||||
<img src="../datasets/publaynet_demo/gt_PMC5086060_00002.jpg" width="500">
|
||||
<img src="../../datasets/publaynet_demo/gt_PMC3724501_00006.jpg" width="500">
|
||||
<img src="../../datasets/publaynet_demo/gt_PMC5086060_00002.jpg" width="500">
|
||||
</div>
|
||||
|
||||
- **下载地址**:https://developer.ibm.com/exchanges/data/all/publaynet/
|
||||
|
@ -30,8 +30,8 @@
|
|||
- **数据简介**:CDLA据集的训练集合中包含5000张图像,验证集合中包含1000张图像。总共包含10个类别,分别是: `Text, Title, Figure, Figure caption, Table, Table caption, Header, Footer, Reference, Equation`。部分图像以及标注框可视化如下所示。
|
||||
|
||||
<div align="center">
|
||||
<img src="../datasets/CDLA_demo/val_0633.jpg" width="500">
|
||||
<img src="../datasets/CDLA_demo/val_0941.jpg" width="500">
|
||||
<img src="../../datasets/CDLA_demo/val_0633.jpg" width="500">
|
||||
<img src="../../datasets/CDLA_demo/val_0941.jpg" width="500">
|
||||
</div>
|
||||
|
||||
- **下载地址**:https://github.com/buptlihang/CDLA
|
||||
|
@ -45,8 +45,8 @@
|
|||
- **数据简介**:TableBank数据集包含Latex(训练集187199张,验证集7265张,测试集5719张)与Word(训练集73383张,验证集2735张,测试集2281张)两种类别的文档。仅包含`Table` 1个类别。部分图像以及标注框可视化如下所示。
|
||||
|
||||
<div align="center">
|
||||
<img src="../datasets/tablebank_demo/004.png" height="700">
|
||||
<img src="../datasets/tablebank_demo/005.png" height="700">
|
||||
<img src="../../datasets/tablebank_demo/004.png" height="700">
|
||||
<img src="../../datasets/tablebank_demo/005.png" height="700">
|
||||
</div>
|
||||
|
||||
- **下载地址**:https://doc-analysis.github.io/tablebank-page/index.html
|
||||
|
|
|
@ -13,6 +13,7 @@
|
|||
- [2.5 分布式训练](#25-分布式训练)
|
||||
- [2.6 知识蒸馏训练](#26-知识蒸馏训练)
|
||||
- [2.7 其他训练环境](#27-其他训练环境)
|
||||
- [2.8 模型微调](#28-模型微调)
|
||||
- [3. 模型评估与预测](#3-模型评估与预测)
|
||||
- [3.1 指标评估](#31-指标评估)
|
||||
- [3.2 测试检测效果](#32-测试检测效果)
|
||||
|
@ -141,7 +142,8 @@ python3 tools/train.py -c configs/det/det_mv3_db.yml \
|
|||
Global.use_amp=True Global.scale_loss=1024.0 Global.use_dynamic_loss_scaling=True
|
||||
```
|
||||
|
||||
<a name="26---fleet---"></a>
|
||||
<a name="25---fleet---"></a>
|
||||
|
||||
## 2.5 分布式训练
|
||||
|
||||
多机多卡训练时,通过 `--ips` 参数设置使用的机器IP地址,通过 `--gpus` 参数设置使用的GPU ID:
|
||||
|
@ -151,7 +153,7 @@ python3 -m paddle.distributed.launch --ips="xx.xx.xx.xx,xx.xx.xx.xx" --gpus '0,1
|
|||
-o Global.pretrained_model=./pretrain_models/MobileNetV3_large_x0_5_pretrained
|
||||
```
|
||||
|
||||
**注意:** 采用多机多卡训练时,需要替换上面命令中的ips值为您机器的地址,机器之间需要能够相互ping通。另外,训练时需要在多个机器上分别启动命令。查看机器ip地址的命令为`ifconfig`。
|
||||
**注意:** (1)采用多机多卡训练时,需要替换上面命令中的ips值为您机器的地址,机器之间需要能够相互ping通;(2)训练时需要在多个机器上分别启动命令。查看机器ip地址的命令为`ifconfig`;(3)更多关于分布式训练的性能优势等信息,请参考:[分布式训练教程](./distributed_training.md)。
|
||||
|
||||
|
||||
<a name="26---distill---"></a>
|
||||
|
@ -177,6 +179,13 @@ Windows平台只支持`单卡`的训练与预测,指定GPU进行训练`set CUD
|
|||
- Linux DCU
|
||||
DCU设备上运行需要设置环境变量 `export HIP_VISIBLE_DEVICES=0,1,2,3`,其余训练评估预测命令与Linux GPU完全相同。
|
||||
|
||||
<a name="28-模型微调"></a>
|
||||
|
||||
## 2.8 模型微调
|
||||
|
||||
实际使用过程中,建议加载官方提供的预训练模型,在自己的数据集中进行微调,关于检测模型的微调方法,请参考:[模型微调教程](./finetune.md)。
|
||||
|
||||
|
||||
<a name="3--------"></a>
|
||||
# 3. 模型评估与预测
|
||||
|
||||
|
@ -196,6 +205,7 @@ python3 tools/eval.py -c configs/det/det_mv3_db.yml -o Global.checkpoints="{pat
|
|||
## 3.2 测试检测效果
|
||||
|
||||
测试单张图像的检测效果:
|
||||
|
||||
```shell
|
||||
python3 tools/infer_det.py -c configs/det/det_mv3_db.yml -o Global.infer_img="./doc/imgs_en/img_10.jpg" Global.pretrained_model="./output/det_db/best_accuracy"
|
||||
```
|
||||
|
@ -226,14 +236,19 @@ python3 tools/export_model.py -c configs/det/det_mv3_db.yml -o Global.pretrained
|
|||
```
|
||||
|
||||
DB检测模型inference 模型预测:
|
||||
|
||||
```shell
|
||||
python3 tools/infer/predict_det.py --det_algorithm="DB" --det_model_dir="./output/det_db_inference/" --image_dir="./doc/imgs/" --use_gpu=True
|
||||
```
|
||||
如果是其他检测,比如EAST模型,det_algorithm参数需要修改为EAST,默认为DB算法:
|
||||
|
||||
```shell
|
||||
python3 tools/infer/predict_det.py --det_algorithm="EAST" --det_model_dir="./output/det_db_inference/" --image_dir="./doc/imgs/" --use_gpu=True
|
||||
```
|
||||
|
||||
更多关于推理超参数的配置与解释,请参考:[模型推理超参数解释教程](./inference_args.md)。
|
||||
|
||||
|
||||
<a name="5-faq"></a>
|
||||
# 5. FAQ
|
||||
|
||||
|
|
|
@ -41,11 +41,16 @@ python3 -m paddle.distributed.launch \
|
|||
|
||||
## 性能效果测试
|
||||
|
||||
* 基于单机8卡P40,和2机8卡P40,在26W公开识别数据集(LSVT, RCTW, MTWI)上进行训练,最终耗时如下。
|
||||
* 在2机8卡P40的机器上,基于26W公开识别数据集(LSVT, RCTW, MTWI)上进行训练,最终耗时如下。
|
||||
|
||||
| 模型 | 配置文件 | 机器数量 | 每台机器的GPU数量 | 训练时间 | 识别Acc | 加速比 |
|
||||
| :----------------------: | :------------: | :------------: | :---------------: | :----------: | :-----------: | :-----------: |
|
||||
| CRNN | configs/rec/ch_ppocr_v2.0/rec_chinese_lite_train_v2.0.yml | 1 | 8 | 60h | 66.7% | - |
|
||||
| CRNN | configs/rec/ch_ppocr_v2.0/rec_chinese_lite_train_v2.0.yml | 2 | 8 | 40h | 67.0% | 150% |
|
||||
| 模型 | 配置 | 精度 | 单机8卡耗时 | 2机8卡耗时 | 加速比 |
|
||||
|------|-----|--------|--------|--------|-----|
|
||||
| CRNN | [rec_chinese_lite_train_v2.0.yml](../../configs/rec/ch_ppocr_v2.0/rec_chinese_lite_train_v2.0.yml) | 67.0% | 2.50d | 1.67d | **1.5** |
|
||||
|
||||
可以看出,精度没有下降的情况下,训练时间由60h缩短为了40h,加速比可以达到60h/40h=150%,效率为60h/(40h*2)=75%。
|
||||
|
||||
* 在4机8卡V100的机器上,基于全量数据训练,最终耗时如下
|
||||
|
||||
|
||||
| 模型 | 配置 | 精度 | 单机8卡耗时 | 4机8卡耗时 | 加速比 |
|
||||
|------|-----|--------|--------|--------|-----|
|
||||
| SVTR | [ch_PP-OCRv3_rec_distillation.yml](../../configs/rec/PP-OCRv3/ch_PP-OCRv3_rec_distillation.yml) | 74.0% | 10d | 2.84d | **3.5** |
|
||||
|
|
|
@ -0,0 +1,120 @@
|
|||
# PaddleOCR模型推理参数解释
|
||||
|
||||
在使用PaddleOCR进行模型推理时,可以自定义修改参数,来修改模型、数据、预处理、后处理等内容(参数文件:[utility.py](../../tools/infer/utility.py)),详细的参数解释如下所示。
|
||||
|
||||
* 全局信息
|
||||
|
||||
| 参数名称 | 类型 | 默认值 | 含义 |
|
||||
| :--: | :--: | :--: | :--: |
|
||||
| image_dir | str | 无,必须显式指定 | 图像或者文件夹路径 |
|
||||
| vis_font_path | str | "./doc/fonts/simfang.ttf" | 用于可视化的字体路径 |
|
||||
| drop_score | float | 0.5 | 识别得分小于该值的结果会被丢弃,不会作为返回结果 |
|
||||
| use_pdserving | bool | False | 是否使用Paddle Serving进行预测 |
|
||||
| warmup | bool | False | 是否开启warmup,在统计预测耗时的时候,可以使用这种方法 |
|
||||
| draw_img_save_dir | str | "./inference_results" | 系统串联预测OCR结果的保存文件夹 |
|
||||
| save_crop_res | bool | False | 是否保存OCR的识别文本图像 |
|
||||
| crop_res_save_dir | str | "./output" | 保存OCR识别出来的文本图像路径 |
|
||||
| use_mp | bool | False | 是否开启多进程预测 |
|
||||
| total_process_num | int | 6 | 开启的进城数,`use_mp`为`True`时生效 |
|
||||
| process_id | int | 0 | 当前进程的id号,无需自己修改 |
|
||||
| benchmark | bool | False | 是否开启benchmark,对预测速度、显存占用等进行统计 |
|
||||
| save_log_path | str | "./log_output/" | 开启`benchmark`时,日志结果的保存文件夹 |
|
||||
| show_log | bool | True | 是否显示预测中的日志信息 |
|
||||
| use_onnx | bool | False | 是否开启onnx预测 |
|
||||
|
||||
|
||||
* 预测引擎相关
|
||||
|
||||
| 参数名称 | 类型 | 默认值 | 含义 |
|
||||
| :--: | :--: | :--: | :--: |
|
||||
| use_gpu | bool | True | 是否使用GPU进行预测 |
|
||||
| ir_optim | bool | True | 是否对计算图进行分析与优化,开启后可以加速预测过程 |
|
||||
| use_tensorrt | bool | False | 是否开启tensorrt |
|
||||
| min_subgraph_size | int | 15 | tensorrt中最小子图size,当子图的size大于该值时,才会尝试对该子图使用trt engine计算 |
|
||||
| precision | str | fp32 | 预测的精度,支持`fp32`, `fp16`, `int8` 3种输入 |
|
||||
| enable_mkldnn | bool | True | 是否开启mkldnn |
|
||||
| cpu_threads | int | 10 | 开启mkldnn时,cpu预测的线程数 |
|
||||
|
||||
* 文本检测模型相关
|
||||
|
||||
| 参数名称 | 类型 | 默认值 | 含义 |
|
||||
| :--: | :--: | :--: | :--: |
|
||||
| det_algorithm | str | "DB" | 文本检测算法名称,目前支持`DB`, `EAST`, `SAST`, `PSE` |
|
||||
| det_model_dir | str | xx | 检测inference模型路径 |
|
||||
| det_limit_side_len | int | 960 | 检测的图像边长限制 |
|
||||
| det_limit_type | str | "max" | 检测的变成限制类型,目前支持`min`, `max`,`min`表示保证图像最短边不小于`det_limit_side_len`,`max`表示保证图像最长边不大于`det_limit_side_len` |
|
||||
|
||||
其中,DB算法相关参数如下
|
||||
|
||||
| 参数名称 | 类型 | 默认值 | 含义 |
|
||||
| :--: | :--: | :--: | :--: |
|
||||
| det_db_thresh | float | 0.3 | DB输出的概率图中,得分大于该阈值的像素点才会被认为是文字像素点 |
|
||||
| det_db_box_thresh | float | 0.6 | 检测结果边框内,所有像素点的平均得分大于该阈值时,该结果会被认为是文字区域 |
|
||||
| det_db_unclip_ratio | float | 1.5 | `Vatti clipping`算法的扩张系数,使用该方法对文字区域进行扩张 |
|
||||
| max_batch_size | int | 10 | 预测的batch size |
|
||||
| use_dilation | bool | False | 是否对分割结果进行膨胀以获取更优检测效果 |
|
||||
| det_db_score_mode | str | "fast" | DB的检测结果得分计算方法,支持`fast`和`slow`,`fast`是根据polygon的外接矩形边框内的所有像素计算平均得分,`slow`是根据原始polygon内的所有像素计算平均得分,计算速度相对较慢一些,但是更加准确一些。 |
|
||||
|
||||
EAST算法相关参数如下
|
||||
|
||||
| 参数名称 | 类型 | 默认值 | 含义 |
|
||||
| :--: | :--: | :--: | :--: |
|
||||
| det_east_score_thresh | float | 0.8 | EAST后处理中score map的阈值 |
|
||||
| det_east_cover_thresh | float | 0.1 | EAST后处理中文本框的平均得分阈值 |
|
||||
| det_east_nms_thresh | float | 0.2 | EAST后处理中nms的阈值 |
|
||||
|
||||
SAST算法相关参数如下
|
||||
|
||||
| 参数名称 | 类型 | 默认值 | 含义 |
|
||||
| :--: | :--: | :--: | :--: |
|
||||
| det_sast_score_thresh | float | 0.5 | SAST后处理中的得分阈值 |
|
||||
| det_sast_nms_thresh | float | 0.5 | SAST后处理中nms的阈值 |
|
||||
| det_sast_polygon | bool | False | 是否多边形检测,弯曲文本场景(如Total-Text)设置为True |
|
||||
|
||||
PSE算法相关参数如下
|
||||
|
||||
| 参数名称 | 类型 | 默认值 | 含义 |
|
||||
| :--: | :--: | :--: | :--: |
|
||||
| det_pse_thresh | float | 0.0 | 对输出图做二值化的阈值 |
|
||||
| det_pse_box_thresh | float | 0.85 | 对box进行过滤的阈值,低于此阈值的丢弃 |
|
||||
| det_pse_min_area | float | 16 | box的最小面积,低于此阈值的丢弃 |
|
||||
| det_pse_box_type | str | "box" | 返回框的类型,box:四点坐标,poly: 弯曲文本的所有点坐标 |
|
||||
| det_pse_scale | int | 1 | 输入图像相对于进后处理的图的比例,如`640*640`的图像,网络输出为`160*160`,scale为2的情况下,进后处理的图片shape为`320*320`。这个值调大可以加快后处理速度,但是会带来精度的下降 |
|
||||
|
||||
* 文本识别模型相关
|
||||
|
||||
| 参数名称 | 类型 | 默认值 | 含义 |
|
||||
| :--: | :--: | :--: | :--: |
|
||||
| rec_algorithm | str | "CRNN" | 文本识别算法名称,目前支持`CRNN`, `SRN`, `RARE`, `NETR`, `SAR` |
|
||||
| rec_model_dir | str | 无,如果使用识别模型,该项是必填项 | 识别inference模型路径 |
|
||||
| rec_image_shape | list | [3, 32, 320] | 识别时的图像尺寸, |
|
||||
| rec_batch_num | int | 6 | 识别的batch size |
|
||||
| max_text_length | int | 25 | 识别结果最大长度,在`SRN`中有效 |
|
||||
| rec_char_dict_path | str | "./ppocr/utils/ppocr_keys_v1.txt" | 识别的字符字典文件 |
|
||||
| use_space_char | bool | True | 是否包含空格,如果为`True`,则会在最后字符字典中补充`空格`字符 |
|
||||
|
||||
|
||||
* 端到端文本检测与识别模型相关
|
||||
|
||||
| 参数名称 | 类型 | 默认值 | 含义 |
|
||||
| :--: | :--: | :--: | :--: |
|
||||
| e2e_algorithm | str | "PGNet" | 端到端算法名称,目前支持`PGNet` |
|
||||
| e2e_model_dir | str | 无,如果使用端到端模型,该项是必填项 | 端到端模型inference模型路径 |
|
||||
| e2e_limit_side_len | int | 768 | 端到端的输入图像边长限制 |
|
||||
| e2e_limit_type | str | "max" | 端到端的边长限制类型,目前支持`min`, `max`,`min`表示保证图像最短边不小于`e2e_limit_side_len`,`max`表示保证图像最长边不大于`e2e_limit_side_len` |
|
||||
| e2e_pgnet_score_thresh | float | 0.5 | 端到端得分阈值,小于该阈值的结果会被丢弃 |
|
||||
| e2e_char_dict_path | str | "./ppocr/utils/ic15_dict.txt" | 识别的字典文件路径 |
|
||||
| e2e_pgnet_valid_set | str | "totaltext" | 验证集名称,目前支持`totaltext`, `partvgg`,不同数据集对应的后处理方式不同,与训练过程保持一致即可 |
|
||||
| e2e_pgnet_mode | str | "fast" | PGNet的检测结果得分计算方法,支持`fast`和`slow`,`fast`是根据polygon的外接矩形边框内的所有像素计算平均得分,`slow`是根据原始polygon内的所有像素计算平均得分,计算速度相对较慢一些,但是更加准确一些。 |
|
||||
|
||||
|
||||
* 方向分类器模型相关
|
||||
|
||||
| 参数名称 | 类型 | 默认值 | 含义 |
|
||||
| :--: | :--: | :--: | :--: |
|
||||
| use_angle_cls | bool | False | 是否使用方向分类器 |
|
||||
| cls_model_dir | str | 无,如果需要使用,则必须显式指定路径 | 方向分类器inference模型路径 |
|
||||
| cls_image_shape | list | [3, 48, 192] | 预测尺度 |
|
||||
| label_list | list | ['0', '180'] | class id对应的角度值 |
|
||||
| cls_batch_num | int | 6 | 方向分类器预测的batch size |
|
||||
| cls_thresh | float | 0.9 | 预测阈值,模型预测结果为180度,且得分大于该阈值时,认为最终预测结果为180度,需要翻转 |
|
|
@ -30,11 +30,11 @@ PP-OCR系统pipeline如下:
|
|||
|
||||
PP-OCR系统在持续迭代优化,目前已发布PP-OCR和PP-OCRv2两个版本:
|
||||
|
||||
PP-OCR从骨干网络选择和调整、预测头部的设计、数据增强、学习率变换策略、正则化参数选择、预训练模型使用以及模型自动裁剪量化8个方面,采用19个有效策略,对各个模块的模型进行效果调优和瘦身(如绿框所示),最终得到整体大小为3.5M的超轻量中英文OCR和2.8M的英文数字OCR。更多细节请参考PP-OCR技术方案 https://arxiv.org/abs/2009.09941
|
||||
PP-OCR从骨干网络选择和调整、预测头部的设计、数据增强、学习率变换策略、正则化参数选择、预训练模型使用以及模型自动裁剪量化8个方面,采用19个有效策略,对各个模块的模型进行效果调优和瘦身(如绿框所示),最终得到整体大小为3.5M的超轻量中英文OCR和2.8M的英文数字OCR。更多细节请参考[PP-OCR技术报告](https://arxiv.org/abs/2009.09941)。
|
||||
|
||||
#### PP-OCRv2
|
||||
|
||||
PP-OCRv2在PP-OCR的基础上,进一步在5个方面重点优化,检测模型采用CML协同互学习知识蒸馏策略和CopyPaste数据增广策略;识别模型采用LCNet轻量级骨干网络、UDML 改进知识蒸馏策略和[Enhanced CTC loss](./enhanced_ctc_loss.md)损失函数改进(如上图红框所示),进一步在推理速度和预测效果上取得明显提升。更多细节请参考PP-OCRv2[技术报告](https://arxiv.org/abs/2109.03144)。
|
||||
PP-OCRv2在PP-OCR的基础上,进一步在5个方面重点优化,检测模型采用CML协同互学习知识蒸馏策略和CopyPaste数据增广策略;识别模型采用LCNet轻量级骨干网络、UDML 改进知识蒸馏策略和[Enhanced CTC loss](./enhanced_ctc_loss.md)损失函数改进(如上图红框所示),进一步在推理速度和预测效果上取得明显提升。更多细节请参考[PP-OCRv2技术报告](https://arxiv.org/abs/2109.03144)。
|
||||
|
||||
#### PP-OCRv3
|
||||
|
||||
|
@ -48,7 +48,7 @@ PP-OCRv3系统pipeline如下:
|
|||
<img src="../ppocrv3_framework.png" width="800">
|
||||
</div>
|
||||
|
||||
更多细节请参考PP-OCRv3[技术报告](./PP-OCRv3_introduction.md)。
|
||||
更多细节请参考[PP-OCRv3技术报告](https://arxiv.org/abs/2206.03001v2) 👉[中文简洁版](./PP-OCRv3_introduction.md)
|
||||
|
||||
|
||||
<a name="2"></a>
|
||||
|
|
|
@ -101,8 +101,17 @@ cd /path/to/ppocr_img
|
|||
['韩国小馆', 0.994467]
|
||||
```
|
||||
|
||||
**版本说明**
|
||||
paddleocr默认使用PP-OCRv3模型(`--ocr_version PP-OCRv3`),如需使用其他版本可通过设置参数`--ocr_version`,具体版本说明如下:
|
||||
| 版本名称 | 版本说明 |
|
||||
| --- | --- |
|
||||
| PP-OCRv3 | 支持中、英文检测和识别,方向分类器,支持多语种识别 |
|
||||
| PP-OCRv2 | 支持中英文的检测和识别,方向分类器,多语言暂未更新 |
|
||||
| PP-OCR | 支持中、英文检测和识别,方向分类器,支持多语种识别 |
|
||||
|
||||
如需使用2.0模型,请指定参数`--ocr_version PP-OCR`,paddleocr默认使用PP-OCRv3模型(`--ocr_version PP-OCRv3`)。更多whl包使用可参考[whl包文档](./whl.md)
|
||||
如需新增自己训练的模型,可以在[paddleocr](../../paddleocr.py)中增加模型链接和字段,重新编译即可。
|
||||
|
||||
更多whl包使用可参考[whl包文档](./whl.md)
|
||||
|
||||
<a name="212"></a>
|
||||
|
||||
|
|
|
@ -18,6 +18,7 @@
|
|||
- [2.6. 知识蒸馏训练](#26-知识蒸馏训练)
|
||||
- [2.7. 多语言模型训练](#27-多语言模型训练)
|
||||
- [2.8. 其他训练环境](#28-其他训练环境)
|
||||
- [2.9. 模型微调](#29-模型微调)
|
||||
- [3. 模型评估与预测](#3-模型评估与预测)
|
||||
- [3.1. 指标评估](#31-指标评估)
|
||||
- [3.2. 测试识别效果](#32-测试识别效果)
|
||||
|
@ -217,6 +218,30 @@ python3 tools/train.py -c configs/rec/PP-OCRv3/en_PP-OCRv3_rec.yml -o Global.pre
|
|||
python3 -m paddle.distributed.launch --gpus '0,1,2,3' tools/train.py -c configs/rec/PP-OCRv3/en_PP-OCRv3_rec.yml -o Global.pretrained_model=./pretrain_models/en_PP-OCRv3_rec_train/best_accuracy
|
||||
```
|
||||
|
||||
正常启动训练后,会看到以下log输出:
|
||||
|
||||
```
|
||||
[2022/02/22 07:58:05] root INFO: epoch: [1/800], iter: 10, lr: 0.000000, loss: 0.754281, acc: 0.000000, norm_edit_dis: 0.000008, reader_cost: 0.55541 s, batch_cost: 0.91654 s, samples: 1408, ips: 153.62133
|
||||
[2022/02/22 07:58:13] root INFO: epoch: [1/800], iter: 20, lr: 0.000001, loss: 0.924677, acc: 0.000000, norm_edit_dis: 0.000008, reader_cost: 0.00236 s, batch_cost: 0.28528 s, samples: 1280, ips: 448.68599
|
||||
[2022/02/22 07:58:23] root INFO: epoch: [1/800], iter: 30, lr: 0.000002, loss: 0.967231, acc: 0.000000, norm_edit_dis: 0.000008, reader_cost: 0.14527 s, batch_cost: 0.42714 s, samples: 1280, ips: 299.66507
|
||||
[2022/02/22 07:58:31] root INFO: epoch: [1/800], iter: 40, lr: 0.000003, loss: 0.895318, acc: 0.000000, norm_edit_dis: 0.000008, reader_cost: 0.00173 s, batch_cost: 0.27719 s, samples: 1280, ips: 461.77252
|
||||
```
|
||||
|
||||
log 中自动打印如下信息:
|
||||
|
||||
| 字段 | 含义 |
|
||||
| :----: | :------: |
|
||||
| epoch | 当前迭代轮次 |
|
||||
| iter | 当前迭代次数 |
|
||||
| lr | 当前学习率 |
|
||||
| loss | 当前损失函数 |
|
||||
| acc | 当前batch的准确率 |
|
||||
| norm_edit_dis | 当前 batch 的编辑距离 |
|
||||
| reader_cost | 当前 batch 数据处理耗时 |
|
||||
| batch_cost | 当前 batch 总耗时 |
|
||||
| samples | 当前 batch 内的样本数 |
|
||||
| ips | 每秒处理图片的数量 |
|
||||
|
||||
|
||||
PaddleOCR支持训练和评估交替进行, 可以在 `configs/rec/PP-OCRv3/en_PP-OCRv3_rec.yml` 中修改 `eval_batch_step` 设置评估频率,默认每500个iter评估一次。评估过程中默认将最佳acc模型,保存为 `output/en_PP-OCRv3_rec/best_accuracy` 。
|
||||
|
||||
|
@ -363,7 +388,7 @@ python3 -m paddle.distributed.launch --ips="xx.xx.xx.xx,xx.xx.xx.xx" --gpus '0,1
|
|||
-o Global.pretrained_model=./pretrain_models/en_PP-OCRv3_rec_train/best_accuracy
|
||||
```
|
||||
|
||||
**注意:** 采用多机多卡训练时,需要替换上面命令中的ips值为您机器的地址,机器之间需要能够相互ping通。另外,训练时需要在多个机器上分别启动命令。查看机器ip地址的命令为`ifconfig`。
|
||||
**注意:** (1)采用多机多卡训练时,需要替换上面命令中的ips值为您机器的地址,机器之间需要能够相互ping通;(2)训练时需要在多个机器上分别启动命令。查看机器ip地址的命令为`ifconfig`;(3)更多关于分布式训练的性能优势等信息,请参考:[分布式训练教程](./distributed_training.md)。
|
||||
|
||||
## 2.6. 知识蒸馏训练
|
||||
|
||||
|
@ -438,6 +463,11 @@ Windows平台只支持`单卡`的训练与预测,指定GPU进行训练`set CUD
|
|||
- Linux DCU
|
||||
DCU设备上运行需要设置环境变量 `export HIP_VISIBLE_DEVICES=0,1,2,3`,其余训练评估预测命令与Linux GPU完全相同。
|
||||
|
||||
## 2.9 模型微调
|
||||
|
||||
实际使用过程中,建议加载官方提供的预训练模型,在自己的数据集中进行微调,关于识别模型的微调方法,请参考:[模型微调教程](./finetune.md)。
|
||||
|
||||
|
||||
# 3. 模型评估与预测
|
||||
|
||||
## 3.1. 指标评估
|
||||
|
@ -540,12 +570,13 @@ inference/en_PP-OCRv3_rec/
|
|||
|
||||
- 自定义模型推理
|
||||
|
||||
如果训练时修改了文本的字典,在使用inference模型预测时,需要通过`--rec_char_dict_path`指定使用的字典路径
|
||||
如果训练时修改了文本的字典,在使用inference模型预测时,需要通过`--rec_char_dict_path`指定使用的字典路径,更多关于推理超参数的配置与解释,请参考:[模型推理超参数解释教程](./inference_args.md)。
|
||||
|
||||
```
|
||||
python3 tools/infer/predict_rec.py --image_dir="./doc/imgs_words_en/word_336.png" --rec_model_dir="./your inference model" --rec_image_shape="3, 48, 320" --rec_char_dict_path="your text dict path"
|
||||
```
|
||||
|
||||
|
||||
# 5. FAQ
|
||||
|
||||
Q1: 训练模型转inference 模型之后预测效果不一致?
|
||||
|
|
|
@ -76,7 +76,7 @@ LK-PAN (Large Kernel PAN) is a lightweight [PAN](https://arxiv.org/pdf/1803.0153
|
|||
|
||||
**(2) DML: Deep Mutual Learning Strategy for Teacher Model**
|
||||
|
||||
[DML](https://arxiv.org/abs/1706.00384)(Collaborative Mutual Learning), as shown in the figure below, can effectively improve the accuracy of the text detection model by learning from each other with two models with the same structure. The DML strategy is adopted in the teacher model training, and the hmean is increased from 85% to 86%. By updating the teacher model of CML in PP-OCRv2 to the above-mentioned higher-precision one, the hmean of the student model can be further improved from 83.2% to 84.3%.
|
||||
[DML](https://arxiv.org/abs/1706.00384)(Deep Mutual Learning), as shown in the figure below, can effectively improve the accuracy of the text detection model by learning from each other with two models with the same structure. The DML strategy is adopted in the teacher model training, and the hmean is increased from 85% to 86%. By updating the teacher model of CML in PP-OCRv2 to the above-mentioned higher-precision one, the hmean of the student model can be further improved from 83.2% to 84.3%.
|
||||
|
||||
|
||||
<div align="center">
|
||||
|
@ -100,7 +100,7 @@ Considering that the features of some channels will be suppressed if the convolu
|
|||
|
||||
The recognition module of PP-OCRv3 is optimized based on the text recognition algorithm [SVTR](https://arxiv.org/abs/2205.00159). RNN is abandoned in SVTR, and the context information of the text line image is more effectively mined by introducing the Transformers structure, thereby improving the text recognition ability.
|
||||
|
||||
The recognition accuracy of SVTR_inty outperforms PP-OCRv2 recognition model by 5.3%, while the prediction speed nearly 11 times slower. It takes nearly 100ms to predict a text line on CPU. Therefore, as shown in the figure below, PP-OCRv3 adopts the following six optimization strategies to accelerate the recognition model.
|
||||
The recognition accuracy of SVTR_tiny outperforms PP-OCRv2 recognition model by 5.3%, while the prediction speed nearly 11 times slower. It takes nearly 100ms to predict a text line on CPU. Therefore, as shown in the figure below, PP-OCRv3 adopts the following six optimization strategies to accelerate the recognition model.
|
||||
|
||||
<div align="center">
|
||||
<img src="../ppocr_v3/v3_rec_pipeline.png" width=800>
|
||||
|
|
|
@ -0,0 +1,139 @@
|
|||
# STAR-Net
|
||||
|
||||
- [1. Introduction](#1)
|
||||
- [2. Environment](#2)
|
||||
- [3. Model Training / Evaluation / Prediction](#3)
|
||||
- [3.1 Training](#3-1)
|
||||
- [3.2 Evaluation](#3-2)
|
||||
- [3.3 Prediction](#3-3)
|
||||
- [4. Inference and Deployment](#4)
|
||||
- [4.1 Python Inference](#4-1)
|
||||
- [4.2 C++ Inference](#4-2)
|
||||
- [4.3 Serving](#4-3)
|
||||
- [4.4 More](#4-4)
|
||||
- [5. FAQ](#5)
|
||||
|
||||
<a name="1"></a>
|
||||
## 1. Introduction
|
||||
|
||||
Paper information:
|
||||
> [STAR-Net: a spatial attention residue network for scene text recognition.](http://www.bmva.org/bmvc/2016/papers/paper043/paper043.pdf)
|
||||
> Wei Liu, Chaofeng Chen, Kwan-Yee K. Wong, Zhizhong Su and Junyu Han.
|
||||
> BMVC, pages 43.1-43.13, 2016
|
||||
|
||||
Refer to [DTRB](https://arxiv.org/abs/1904.01906) text Recognition Training and Evaluation Process . Using MJSynth and SynthText two text recognition datasets for training, and evaluating on IIIT, SVT, IC03, IC13, IC15, SVTP, CUTE datasets, the algorithm reproduction effect is as follows:
|
||||
|
||||
|Models|Backbone Networks|Avg Accuracy|Configuration Files|Download Links|
|
||||
| --- | --- | --- | --- | --- |
|
||||
|StarNet|Resnet34_vd|84.44%|[configs/rec/rec_r34_vd_tps_bilstm_ctc.yml](../../configs/rec/rec_r34_vd_tps_bilstm_ctc.yml)|[trained model](https://paddleocr.bj.bcebos.com/dygraph_v2.0/en/rec_r34_vd_tps_bilstm_ctc_v2.0_train.tar)|
|
||||
|StarNet|MobileNetV3|81.42%|[configs/rec/rec_mv3_tps_bilstm_ctc.yml](../../configs/rec/rec_mv3_tps_bilstm_ctc.yml)|[ trained model](https://paddleocr.bj.bcebos.com/dygraph_v2.0/en/rec_mv3_tps_bilstm_ctc_v2.0_train.tar)|
|
||||
|
||||
|
||||
<a name="2"></a>
|
||||
## 2. Environment
|
||||
Please refer to [Operating Environment Preparation](./environment_en.md) to configure the PaddleOCR operating environment, and refer to [Project Clone](./clone_en.md) to clone the project code.
|
||||
|
||||
<a name="3"></a>
|
||||
## 3. Model Training / Evaluation / Prediction
|
||||
|
||||
Please refer to [Text Recognition Training Tutorial](./recognition_en.md). PaddleOCR modularizes the code, and training different recognition models only requires **changing the configuration file**. Take the backbone network based on Resnet34_vd as an example:
|
||||
|
||||
<a name="3-1"></a>
|
||||
### 3.1 Training
|
||||
After the data preparation is complete, the training can be started. The training command is as follows:
|
||||
|
||||
````
|
||||
#Single card training (long training period, not recommended)
|
||||
python3 tools/train.py -c configs/rec/rec_r34_vd_tps_bilstm_ctc.yml #Multi-card training, specify the card number through the --gpus parameter
|
||||
python3 -m paddle.distributed.launch --gpus '0,1,2,3' tools/train.py -c rec_r34_vd_tps_bilstm_ctc.yml
|
||||
````
|
||||
|
||||
<a name="3-2"></a>
|
||||
### 3.2 Evaluation
|
||||
|
||||
````
|
||||
# GPU evaluation, Global.pretrained_model is the model to be evaluated
|
||||
python3 -m paddle.distributed.launch --gpus '0' tools/eval.py -c configs/rec/rec_r34_vd_tps_bilstm_ctc.yml -o Global.pretrained_model={path/to/weights}/best_accuracy
|
||||
````
|
||||
|
||||
<a name="3-3"></a>
|
||||
### 3.3 Prediction
|
||||
|
||||
````
|
||||
# The configuration file used for prediction must match the training
|
||||
python3 tools/infer_rec.py -c configs/rec/rec_r34_vd_tps_bilstm_ctc.yml -o Global.pretrained_model={path/to/weights}/best_accuracy Global.infer_img=doc/imgs_words/en/word_1.png
|
||||
````
|
||||
|
||||
<a name="4"></a>
|
||||
## 4. Inference
|
||||
|
||||
<a name="4-1"></a>
|
||||
### 4.1 Python Inference
|
||||
First, convert the model saved during the STAR-Net text recognition training process into an inference model. Take the model trained on the MJSynth and SynthText text recognition datasets based on the Resnet34_vd backbone network as an example [Model download address]( https://paddleocr.bj.bcebos.com/dygraph_v2.0/en/rec_r34_vd_none_bilstm_ctc_v2.0_train.tar) , which can be converted using the following command:
|
||||
|
||||
```shell
|
||||
python3 tools/export_model.py -c configs/rec/rec_r34_vd_tps_bilstm_ctc.yml -o Global.pretrained_model=./rec_r34_vd_tps_bilstm_ctc_v2.0_train/best_accuracy Global.save_inference_dir=./inference/rec_starnet
|
||||
````
|
||||
|
||||
STAR-Net text recognition model inference, you can execute the following commands:
|
||||
|
||||
```shell
|
||||
python3 tools/infer/predict_rec.py --image_dir="./doc/imgs_words_en/word_336.png" --rec_model_dir="./inference/rec_starnet/" --rec_image_shape="3, 32, 100" --rec_char_dict_path="./ppocr/utils/ic15_dict.txt"
|
||||
````
|
||||
|
||||

|
||||
|
||||
The inference results are as follows:
|
||||
|
||||
|
||||
```bash
|
||||
Predicts of ./doc/imgs_words_en/word_336.png:('super', 0.9999073)
|
||||
```
|
||||
|
||||
**Attention** Since the above model refers to the [DTRB](https://arxiv.org/abs/1904.01906) text recognition training and evaluation process, it is different from the ultra-lightweight Chinese recognition model training in two aspects:
|
||||
|
||||
- The image resolutions used during training are different. The image resolutions used for training the above models are [3, 32, 100], while for Chinese model training, in order to ensure the recognition effect of long texts, the image resolutions used during training are [ 3, 32, 320]. The default shape parameter of the predictive inference program is the image resolution used for training Chinese, i.e. [3, 32, 320]. Therefore, when inferring the above English model here, it is necessary to set the shape of the recognized image through the parameter rec_image_shape.
|
||||
|
||||
- Character list, the experiment in the DTRB paper is only for 26 lowercase English letters and 10 numbers, a total of 36 characters. All uppercase and lowercase characters are converted to lowercase characters, and characters not listed above are ignored and considered spaces. Therefore, there is no input character dictionary here, but a dictionary is generated by the following command. Therefore, the parameter rec_char_dict_path needs to be set during inference, which is specified as an English dictionary "./ppocr/utils/ic15_dict.txt".
|
||||
|
||||
```
|
||||
self.character_str = "0123456789abcdefghijklmnopqrstuvwxyz"
|
||||
dict_character = list(self.character_str)
|
||||
|
||||
|
||||
```
|
||||
|
||||
<a name="4-2"></a>
|
||||
### 4.2 C++ Inference
|
||||
|
||||
After preparing the inference model, refer to the [cpp infer](../../deploy/cpp_infer/) tutorial to operate.
|
||||
|
||||
<a name="4-3"></a>
|
||||
### 4.3 Serving
|
||||
|
||||
After preparing the inference model, refer to the [pdserving](../../deploy/pdserving/) tutorial for Serving deployment, including two modes: Python Serving and C++ Serving.
|
||||
|
||||
<a name="4-4"></a>
|
||||
### 4.4 More
|
||||
|
||||
The STAR-Net model also supports the following inference deployment methods:
|
||||
|
||||
- Paddle2ONNX Inference: After preparing the inference model, refer to the [paddle2onnx](../../deploy/paddle2onnx/) tutorial.
|
||||
|
||||
<a name="5"></a>
|
||||
## 5. FAQ
|
||||
|
||||
## Quote
|
||||
|
||||
```bibtex
|
||||
@inproceedings{liu2016star,
|
||||
title={STAR-Net: a spatial attention residue network for scene text recognition.},
|
||||
author={Liu, Wei and Chen, Chaofeng and Wong, Kwan-Yee K and Su, Zhizhong and Han, Junyu},
|
||||
booktitle={BMVC},
|
||||
volume={2},
|
||||
pages={7},
|
||||
year={2016}
|
||||
}
|
||||
```
|
||||
|
||||
|
|
@ -159,7 +159,7 @@ python3 -m paddle.distributed.launch --ips="xx.xx.xx.xx,xx.xx.xx.xx" --gpus '0,1
|
|||
-o Global.pretrained_model=./pretrain_models/MobileNetV3_large_x0_5_pretrained
|
||||
```
|
||||
|
||||
**Note:** When using multi-machine and multi-gpu training, you need to replace the ips value in the above command with the address of your machine, and the machines need to be able to ping each other. In addition, training needs to be launched separately on multiple machines. The command to view the ip address of the machine is `ifconfig`.
|
||||
**Note:** (1) When using multi-machine and multi-gpu training, you need to replace the ips value in the above command with the address of your machine, and the machines need to be able to ping each other. (2) Training needs to be launched separately on multiple machines. The command to view the ip address of the machine is `ifconfig`. (3) For more details about the distributed training speedup ratio, please refer to [Distributed Training Tutorial](./distributed_training_en.md).
|
||||
|
||||
### 2.6 Training with knowledge distillation
|
||||
|
||||
|
|
|
@ -40,11 +40,17 @@ python3 -m paddle.distributed.launch \
|
|||
|
||||
## Performance comparison
|
||||
|
||||
* Based on 26W public recognition dataset (LSVT, rctw, mtwi), training on single 8-card P40 and dual 8-card P40, the final time consumption is as follows.
|
||||
* On two 8-card P40 graphics cards, the final time consumption and speedup ratio for public recognition dataset (LSVT, RCTW, MTWI) containing 260k images are as follows.
|
||||
|
||||
| Model | Config file | Number of machines | Number of GPUs per machine | Training time | Recognition acc | Speedup ratio |
|
||||
| :-------: | :------------: | :----------------: | :----------------------------: | :------------------: | :--------------: | :-----------: |
|
||||
| CRNN | configs/rec/ch_ppocr_v2.0/rec_chinese_lite_train_v2.0.yml | 1 | 8 | 60h | 66.7% | - |
|
||||
| CRNN | configs/rec/ch_ppocr_v2.0/rec_chinese_lite_train_v2.0.yml | 2 | 8 | 40h | 67.0% | 150% |
|
||||
|
||||
It can be seen that the training time is shortened from 60h to 40h, the speedup ratio can reach 150% (60h / 40h), and the efficiency is 75% (60h / (40h * 2)).
|
||||
| Model | Config file | Recognition acc | single 8-card training time | two 8-card training time | Speedup ratio |
|
||||
|------|-----|--------|--------|--------|-----|
|
||||
| CRNN | [rec_chinese_lite_train_v2.0.yml](../../configs/rec/ch_ppocr_v2.0/rec_chinese_lite_train_v2.0.yml) | 67.0% | 2.50d | 1.67d | **1.5** |
|
||||
|
||||
|
||||
* On four 8-card V100 graphics cards, the final time consumption and speedup ratio for full data are as follows.
|
||||
|
||||
|
||||
| Model | Config file | Recognition acc | single 8-card training time | four 8-card training time | Speedup ratio |
|
||||
|------|-----|--------|--------|--------|-----|
|
||||
| SVTR | [ch_PP-OCRv3_rec_distillation.yml](../../configs/rec/PP-OCRv3/ch_PP-OCRv3_rec_distillation.yml) | 74.0% | 10d | 2.84d | **3.5** |
|
|
@ -29,10 +29,10 @@ PP-OCR pipeline is as follows:
|
|||
|
||||
PP-OCR system is in continuous optimization. At present, PP-OCR and PP-OCRv2 have been released:
|
||||
|
||||
PP-OCR adopts 19 effective strategies from 8 aspects including backbone network selection and adjustment, prediction head design, data augmentation, learning rate transformation strategy, regularization parameter selection, pre-training model use, and automatic model tailoring and quantization to optimize and slim down the models of each module (as shown in the green box above). The final results are an ultra-lightweight Chinese and English OCR model with an overall size of 3.5M and a 2.8M English digital OCR model. For more details, please refer to the PP-OCR technical article (https://arxiv.org/abs/2009.09941).
|
||||
PP-OCR adopts 19 effective strategies from 8 aspects including backbone network selection and adjustment, prediction head design, data augmentation, learning rate transformation strategy, regularization parameter selection, pre-training model use, and automatic model tailoring and quantization to optimize and slim down the models of each module (as shown in the green box above). The final results are an ultra-lightweight Chinese and English OCR model with an overall size of 3.5M and a 2.8M English digital OCR model. For more details, please refer to [PP-OCR technical report](https://arxiv.org/abs/2009.09941).
|
||||
|
||||
#### PP-OCRv2
|
||||
On the basis of PP-OCR, PP-OCRv2 is further optimized in five aspects. The detection model adopts CML(Collaborative Mutual Learning) knowledge distillation strategy and CopyPaste data expansion strategy. The recognition model adopts LCNet lightweight backbone network, U-DML knowledge distillation strategy and enhanced CTC loss function improvement (as shown in the red box above), which further improves the inference speed and prediction effect. For more details, please refer to the technical report of PP-OCRv2 (https://arxiv.org/abs/2109.03144).
|
||||
On the basis of PP-OCR, PP-OCRv2 is further optimized in five aspects. The detection model adopts CML(Collaborative Mutual Learning) knowledge distillation strategy and CopyPaste data expansion strategy. The recognition model adopts LCNet lightweight backbone network, U-DML knowledge distillation strategy and enhanced CTC loss function improvement (as shown in the red box above), which further improves the inference speed and prediction effect. For more details, please refer to [PP-OCRv2 technical report](https://arxiv.org/abs/2109.03144).
|
||||
|
||||
#### PP-OCRv3
|
||||
|
||||
|
@ -46,7 +46,7 @@ PP-OCRv3 pipeline is as follows:
|
|||
<img src="../ppocrv3_framework.png" width="800">
|
||||
</div>
|
||||
|
||||
For more details, please refer to [PP-OCRv3 technical report](./PP-OCRv3_introduction_en.md).
|
||||
For more details, please refer to [PP-OCRv3 technical report](https://arxiv.org/abs/2206.03001v2).
|
||||
|
||||
<a name="2"></a>
|
||||
## 2. Features
|
||||
|
|
|
@ -119,7 +119,18 @@ If you do not use the provided test image, you can replace the following `--imag
|
|||
['PAIN', 0.9934559464454651]
|
||||
```
|
||||
|
||||
If you need to use the 2.0 model, please specify the parameter `--ocr_version PP-OCR`, paddleocr uses the PP-OCRv3 model by default(`--ocr_version PP-OCRv3`). More whl package usage can be found in [whl package](./whl_en.md)
|
||||
**Version**
|
||||
paddleocr uses the PP-OCRv3 model by default(`--ocr_version PP-OCRv3`). If you want to use other versions, you can set the parameter `--ocr_version`, the specific version description is as follows:
|
||||
| version name | description |
|
||||
| --- | --- |
|
||||
| PP-OCRv3 | support Chinese and English detection and recognition, direction classifier, support multilingual recognition |
|
||||
| PP-OCRv2 | only supports Chinese and English detection and recognition, direction classifier, multilingual model is not updated |
|
||||
| PP-OCR | support Chinese and English detection and recognition, direction classifier, support multilingual recognition |
|
||||
|
||||
If you want to add your own trained model, you can add model links and keys in [paddleocr](../../paddleocr.py) and recompile.
|
||||
|
||||
More whl package usage can be found in [whl package](./whl_en.md)
|
||||
|
||||
<a name="212-multi-language-model"></a>
|
||||
|
||||
#### 2.1.2 Multi-language Model
|
||||
|
|
|
@ -306,7 +306,7 @@ python3 -m paddle.distributed.launch --ips="xx.xx.xx.xx,xx.xx.xx.xx" --gpus '0,1
|
|||
-o Global.pretrained_model=./pretrain_models/rec_mv3_none_bilstm_ctc_v2.0_train
|
||||
```
|
||||
|
||||
**Note:** When using multi-machine and multi-gpu training, you need to replace the ips value in the above command with the address of your machine, and the machines need to be able to ping each other. In addition, training needs to be launched separately on multiple machines. The command to view the ip address of the machine is `ifconfig`.
|
||||
**Note:** (1) When using multi-machine and multi-gpu training, you need to replace the ips value in the above command with the address of your machine, and the machines need to be able to ping each other. (2) Training needs to be launched separately on multiple machines. The command to view the ip address of the machine is `ifconfig`. (3) For more details about the distributed training speedup ratio, please refer to [Distributed Training Tutorial](./distributed_training_en.md).
|
||||
|
||||
<a name="kd"></a>
|
||||
### 2.6 Training with Knowledge Distillation
|
||||
|
|
|
@ -154,7 +154,13 @@ MODEL_URLS = {
|
|||
'https://paddleocr.bj.bcebos.com/PP-OCRv2/chinese/ch_PP-OCRv2_rec_infer.tar',
|
||||
'dict_path': './ppocr/utils/ppocr_keys_v1.txt'
|
||||
}
|
||||
}
|
||||
},
|
||||
'cls': {
|
||||
'ch': {
|
||||
'url':
|
||||
'https://paddleocr.bj.bcebos.com/dygraph_v2.0/ch/ch_ppocr_mobile_v2.0_cls_infer.tar',
|
||||
}
|
||||
},
|
||||
},
|
||||
'PP-OCR': {
|
||||
'det': {
|
||||
|
|
|
@ -22,7 +22,7 @@ from .make_shrink_map import MakeShrinkMap
|
|||
from .random_crop_data import EastRandomCropData, RandomCropImgMask
|
||||
from .make_pse_gt import MakePseGt
|
||||
|
||||
from .rec_img_aug import RecAug, RecConAug, RecResizeImg, ClsResizeImg, \
|
||||
from .rec_img_aug import BaseDataAugmentation, RecAug, RecConAug, RecResizeImg, ClsResizeImg, \
|
||||
SRNRecResizeImg, NRTRRecResizeImg, SARRecResizeImg, PRENResizeImg
|
||||
from .ssl_img_aug import SSLRotateResize
|
||||
from .randaugment import RandAugment
|
||||
|
|
|
@ -22,13 +22,74 @@ from .text_image_aug import tia_perspective, tia_stretch, tia_distort
|
|||
|
||||
|
||||
class RecAug(object):
|
||||
def __init__(self, use_tia=True, aug_prob=0.4, **kwargs):
|
||||
self.use_tia = use_tia
|
||||
self.aug_prob = aug_prob
|
||||
def __init__(self,
|
||||
tia_prob=0.4,
|
||||
crop_prob=0.4,
|
||||
reverse_prob=0.4,
|
||||
noise_prob=0.4,
|
||||
jitter_prob=0.4,
|
||||
blur_prob=0.4,
|
||||
hsv_aug_prob=0.4,
|
||||
**kwargs):
|
||||
self.tia_prob = tia_prob
|
||||
self.bda = BaseDataAugmentation(crop_prob, reverse_prob, noise_prob,
|
||||
jitter_prob, blur_prob, hsv_aug_prob)
|
||||
|
||||
def __call__(self, data):
|
||||
img = data['image']
|
||||
img = warp(img, 10, self.use_tia, self.aug_prob)
|
||||
h, w, _ = img.shape
|
||||
|
||||
# tia
|
||||
if random.random() <= self.tia_prob:
|
||||
if h >= 20 and w >= 20:
|
||||
img = tia_distort(img, random.randint(3, 6))
|
||||
img = tia_stretch(img, random.randint(3, 6))
|
||||
img = tia_perspective(img)
|
||||
|
||||
# bda
|
||||
data['image'] = img
|
||||
data = self.bda(data)
|
||||
return data
|
||||
|
||||
|
||||
class BaseDataAugmentation(object):
|
||||
def __init__(self,
|
||||
crop_prob=0.4,
|
||||
reverse_prob=0.4,
|
||||
noise_prob=0.4,
|
||||
jitter_prob=0.4,
|
||||
blur_prob=0.4,
|
||||
hsv_aug_prob=0.4,
|
||||
**kwargs):
|
||||
self.crop_prob = crop_prob
|
||||
self.reverse_prob = reverse_prob
|
||||
self.noise_prob = noise_prob
|
||||
self.jitter_prob = jitter_prob
|
||||
self.blur_prob = blur_prob
|
||||
self.hsv_aug_prob = hsv_aug_prob
|
||||
|
||||
def __call__(self, data):
|
||||
img = data['image']
|
||||
h, w, _ = img.shape
|
||||
|
||||
if random.random() <= self.crop_prob and h >= 20 and w >= 20:
|
||||
img = get_crop(img)
|
||||
|
||||
if random.random() <= self.blur_prob:
|
||||
img = blur(img)
|
||||
|
||||
if random.random() <= self.hsv_aug_prob:
|
||||
img = hsv_aug(img)
|
||||
|
||||
if random.random() <= self.jitter_prob:
|
||||
img = jitter(img)
|
||||
|
||||
if random.random() <= self.noise_prob:
|
||||
img = add_gasuss_noise(img)
|
||||
|
||||
if random.random() <= self.reverse_prob:
|
||||
img = 255 - img
|
||||
|
||||
data['image'] = img
|
||||
return data
|
||||
|
||||
|
@ -359,7 +420,7 @@ def flag():
|
|||
return 1 if random.random() > 0.5000001 else -1
|
||||
|
||||
|
||||
def cvtColor(img):
|
||||
def hsv_aug(img):
|
||||
"""
|
||||
cvtColor
|
||||
"""
|
||||
|
@ -427,50 +488,6 @@ def get_crop(image):
|
|||
return crop_img
|
||||
|
||||
|
||||
class Config:
|
||||
"""
|
||||
Config
|
||||
"""
|
||||
|
||||
def __init__(self, use_tia):
|
||||
self.anglex = random.random() * 30
|
||||
self.angley = random.random() * 15
|
||||
self.anglez = random.random() * 10
|
||||
self.fov = 42
|
||||
self.r = 0
|
||||
self.shearx = random.random() * 0.3
|
||||
self.sheary = random.random() * 0.05
|
||||
self.borderMode = cv2.BORDER_REPLICATE
|
||||
self.use_tia = use_tia
|
||||
|
||||
def make(self, w, h, ang):
|
||||
"""
|
||||
make
|
||||
"""
|
||||
self.anglex = random.random() * 5 * flag()
|
||||
self.angley = random.random() * 5 * flag()
|
||||
self.anglez = -1 * random.random() * int(ang) * flag()
|
||||
self.fov = 42
|
||||
self.r = 0
|
||||
self.shearx = 0
|
||||
self.sheary = 0
|
||||
self.borderMode = cv2.BORDER_REPLICATE
|
||||
self.w = w
|
||||
self.h = h
|
||||
|
||||
self.perspective = self.use_tia
|
||||
self.stretch = self.use_tia
|
||||
self.distort = self.use_tia
|
||||
|
||||
self.crop = True
|
||||
self.affine = False
|
||||
self.reverse = True
|
||||
self.noise = True
|
||||
self.jitter = True
|
||||
self.blur = True
|
||||
self.color = True
|
||||
|
||||
|
||||
def rad(x):
|
||||
"""
|
||||
rad
|
||||
|
@ -554,48 +571,3 @@ def get_warpAffine(config):
|
|||
rz = np.array([[np.cos(rad(anglez)), np.sin(rad(anglez)), 0],
|
||||
[-np.sin(rad(anglez)), np.cos(rad(anglez)), 0]], np.float32)
|
||||
return rz
|
||||
|
||||
|
||||
def warp(img, ang, use_tia=True, prob=0.4):
|
||||
"""
|
||||
warp
|
||||
"""
|
||||
h, w, _ = img.shape
|
||||
config = Config(use_tia=use_tia)
|
||||
config.make(w, h, ang)
|
||||
new_img = img
|
||||
|
||||
if config.distort:
|
||||
img_height, img_width = img.shape[0:2]
|
||||
if random.random() <= prob and img_height >= 20 and img_width >= 20:
|
||||
new_img = tia_distort(new_img, random.randint(3, 6))
|
||||
|
||||
if config.stretch:
|
||||
img_height, img_width = img.shape[0:2]
|
||||
if random.random() <= prob and img_height >= 20 and img_width >= 20:
|
||||
new_img = tia_stretch(new_img, random.randint(3, 6))
|
||||
|
||||
if config.perspective:
|
||||
if random.random() <= prob:
|
||||
new_img = tia_perspective(new_img)
|
||||
|
||||
if config.crop:
|
||||
img_height, img_width = img.shape[0:2]
|
||||
if random.random() <= prob and img_height >= 20 and img_width >= 20:
|
||||
new_img = get_crop(new_img)
|
||||
|
||||
if config.blur:
|
||||
if random.random() <= prob:
|
||||
new_img = blur(new_img)
|
||||
if config.color:
|
||||
if random.random() <= prob:
|
||||
new_img = cvtColor(new_img)
|
||||
if config.jitter:
|
||||
new_img = jitter(new_img)
|
||||
if config.noise:
|
||||
if random.random() <= prob:
|
||||
new_img = add_gasuss_noise(new_img)
|
||||
if config.reverse:
|
||||
if random.random() <= prob:
|
||||
new_img = 255 - new_img
|
||||
return new_img
|
||||
|
|
|
@ -33,7 +33,7 @@ class SimpleDataSet(Dataset):
|
|||
self.delimiter = dataset_config.get('delimiter', '\t')
|
||||
label_file_list = dataset_config.pop('label_file_list')
|
||||
data_source_num = len(label_file_list)
|
||||
ratio_list = dataset_config.get("ratio_list", [1.0])
|
||||
ratio_list = dataset_config.get("ratio_list", 1.0)
|
||||
if isinstance(ratio_list, (float, int)):
|
||||
ratio_list = [float(ratio_list)] * int(data_source_num)
|
||||
|
||||
|
|
|
@ -27,12 +27,12 @@ class CosineEmbeddingLoss(nn.Layer):
|
|||
self.epsilon = 1e-12
|
||||
|
||||
def forward(self, x1, x2, target):
|
||||
similarity = paddle.fluid.layers.reduce_sum(
|
||||
similarity = paddle.sum(
|
||||
x1 * x2, dim=-1) / (paddle.norm(
|
||||
x1, axis=-1) * paddle.norm(
|
||||
x2, axis=-1) + self.epsilon)
|
||||
one_list = paddle.full_like(target, fill_value=1)
|
||||
out = paddle.fluid.layers.reduce_mean(
|
||||
out = paddle.mean(
|
||||
paddle.where(
|
||||
paddle.equal(target, one_list), 1. - similarity,
|
||||
paddle.maximum(
|
||||
|
|
|
@ -19,7 +19,6 @@ from __future__ import print_function
|
|||
import paddle
|
||||
from paddle import nn
|
||||
from paddle.nn import functional as F
|
||||
from paddle import fluid
|
||||
|
||||
class TableAttentionLoss(nn.Layer):
|
||||
def __init__(self, structure_weight, loc_weight, use_giou=False, giou_weight=1.0, **kwargs):
|
||||
|
@ -36,13 +35,13 @@ class TableAttentionLoss(nn.Layer):
|
|||
:param bbox:[[x1,y1,x2,y2], [x1,y1,x2,y2],,,]
|
||||
:return: loss
|
||||
'''
|
||||
ix1 = fluid.layers.elementwise_max(preds[:, 0], bbox[:, 0])
|
||||
iy1 = fluid.layers.elementwise_max(preds[:, 1], bbox[:, 1])
|
||||
ix2 = fluid.layers.elementwise_min(preds[:, 2], bbox[:, 2])
|
||||
iy2 = fluid.layers.elementwise_min(preds[:, 3], bbox[:, 3])
|
||||
ix1 = paddle.maximum(preds[:, 0], bbox[:, 0])
|
||||
iy1 = paddle.maximum(preds[:, 1], bbox[:, 1])
|
||||
ix2 = paddle.minimum(preds[:, 2], bbox[:, 2])
|
||||
iy2 = paddle.minimum(preds[:, 3], bbox[:, 3])
|
||||
|
||||
iw = fluid.layers.clip(ix2 - ix1 + 1e-3, 0., 1e10)
|
||||
ih = fluid.layers.clip(iy2 - iy1 + 1e-3, 0., 1e10)
|
||||
iw = paddle.clip(ix2 - ix1 + 1e-3, 0., 1e10)
|
||||
ih = paddle.clip(iy2 - iy1 + 1e-3, 0., 1e10)
|
||||
|
||||
# overlap
|
||||
inters = iw * ih
|
||||
|
@ -55,12 +54,12 @@ class TableAttentionLoss(nn.Layer):
|
|||
# ious
|
||||
ious = inters / uni
|
||||
|
||||
ex1 = fluid.layers.elementwise_min(preds[:, 0], bbox[:, 0])
|
||||
ey1 = fluid.layers.elementwise_min(preds[:, 1], bbox[:, 1])
|
||||
ex2 = fluid.layers.elementwise_max(preds[:, 2], bbox[:, 2])
|
||||
ey2 = fluid.layers.elementwise_max(preds[:, 3], bbox[:, 3])
|
||||
ew = fluid.layers.clip(ex2 - ex1 + 1e-3, 0., 1e10)
|
||||
eh = fluid.layers.clip(ey2 - ey1 + 1e-3, 0., 1e10)
|
||||
ex1 = paddle.minimum(preds[:, 0], bbox[:, 0])
|
||||
ey1 = paddle.minimum(preds[:, 1], bbox[:, 1])
|
||||
ex2 = paddle.maximum(preds[:, 2], bbox[:, 2])
|
||||
ey2 = paddle.maximum(preds[:, 3], bbox[:, 3])
|
||||
ew = paddle.clip(ex2 - ex1 + 1e-3, 0., 1e10)
|
||||
eh = paddle.clip(ey2 - ey1 + 1e-3, 0., 1e10)
|
||||
|
||||
# enclose erea
|
||||
enclose = ew * eh + eps
|
||||
|
|
|
@ -175,12 +175,7 @@ class Kie_backbone(nn.Layer):
|
|||
img, relations, texts, gt_bboxes, tag, img_size)
|
||||
x = self.img_feat(img)
|
||||
boxes, rois_num = self.bbox2roi(gt_bboxes)
|
||||
feats = paddle.fluid.layers.roi_align(
|
||||
x,
|
||||
boxes,
|
||||
spatial_scale=1.0,
|
||||
pooled_height=7,
|
||||
pooled_width=7,
|
||||
rois_num=rois_num)
|
||||
feats = paddle.vision.ops.roi_align(
|
||||
x, boxes, spatial_scale=1.0, output_size=7, boxes_num=rois_num)
|
||||
feats = self.maxpool(feats).squeeze(-1).squeeze(-1)
|
||||
return [relations, texts, feats]
|
||||
|
|
|
@ -18,7 +18,6 @@ from __future__ import print_function
|
|||
|
||||
from paddle import nn, ParamAttr
|
||||
from paddle.nn import functional as F
|
||||
import paddle.fluid as fluid
|
||||
import paddle
|
||||
import numpy as np
|
||||
|
||||
|
|
|
@ -20,13 +20,11 @@ import math
|
|||
import paddle
|
||||
from paddle import nn, ParamAttr
|
||||
from paddle.nn import functional as F
|
||||
import paddle.fluid as fluid
|
||||
import numpy as np
|
||||
from .self_attention import WrapEncoderForFeature
|
||||
from .self_attention import WrapEncoder
|
||||
from paddle.static import Program
|
||||
from ppocr.modeling.backbones.rec_resnet_fpn import ResNetFPN
|
||||
import paddle.fluid.framework as framework
|
||||
|
||||
from collections import OrderedDict
|
||||
gradient_clip = 10
|
||||
|
|
|
@ -22,7 +22,6 @@ import paddle
|
|||
from paddle import ParamAttr, nn
|
||||
from paddle import nn, ParamAttr
|
||||
from paddle.nn import functional as F
|
||||
import paddle.fluid as fluid
|
||||
import numpy as np
|
||||
gradient_clip = 10
|
||||
|
||||
|
@ -288,10 +287,10 @@ class PrePostProcessLayer(nn.Layer):
|
|||
"layer_norm_%d" % len(self.sublayers()),
|
||||
paddle.nn.LayerNorm(
|
||||
normalized_shape=d_model,
|
||||
weight_attr=fluid.ParamAttr(
|
||||
initializer=fluid.initializer.Constant(1.)),
|
||||
bias_attr=fluid.ParamAttr(
|
||||
initializer=fluid.initializer.Constant(0.)))))
|
||||
weight_attr=paddle.ParamAttr(
|
||||
initializer=paddle.nn.initializer.Constant(1.)),
|
||||
bias_attr=paddle.ParamAttr(
|
||||
initializer=paddle.nn.initializer.Constant(0.)))))
|
||||
elif cmd == "d": # add dropout
|
||||
self.functors.append(lambda x: F.dropout(
|
||||
x, p=dropout_rate, mode="downscale_in_infer")
|
||||
|
@ -324,7 +323,7 @@ class PrepareEncoder(nn.Layer):
|
|||
|
||||
def forward(self, src_word, src_pos):
|
||||
src_word_emb = src_word
|
||||
src_word_emb = fluid.layers.cast(src_word_emb, 'float32')
|
||||
src_word_emb = paddle.cast(src_word_emb, 'float32')
|
||||
src_word_emb = paddle.scale(x=src_word_emb, scale=self.src_emb_dim**0.5)
|
||||
src_pos = paddle.squeeze(src_pos, axis=-1)
|
||||
src_pos_enc = self.emb(src_pos)
|
||||
|
@ -367,7 +366,7 @@ class PrepareDecoder(nn.Layer):
|
|||
self.dropout_rate = dropout_rate
|
||||
|
||||
def forward(self, src_word, src_pos):
|
||||
src_word = fluid.layers.cast(src_word, 'int64')
|
||||
src_word = paddle.cast(src_word, 'int64')
|
||||
src_word = paddle.squeeze(src_word, axis=-1)
|
||||
src_word_emb = self.emb0(src_word)
|
||||
src_word_emb = paddle.scale(x=src_word_emb, scale=self.src_emb_dim**0.5)
|
||||
|
|
|
@ -18,7 +18,7 @@ The table recognition mainly contains three models
|
|||
|
||||
The table recognition flow chart is as follows
|
||||
|
||||

|
||||

|
||||
|
||||
1. The coordinates of single-line text is detected by DB model, and then sends it to the recognition model to get the recognition result.
|
||||
2. The table structure and cell coordinates is predicted by RARE model.
|
||||
|
|
|
@ -28,6 +28,7 @@ import numpy as np
|
|||
import time
|
||||
import tools.infer.predict_rec as predict_rec
|
||||
import tools.infer.predict_det as predict_det
|
||||
import tools.infer.utility as utility
|
||||
from ppocr.utils.utility import get_image_file_list, check_and_read_gif
|
||||
from ppocr.utils.logging import get_logger
|
||||
from ppstructure.table.matcher import distance, compute_iou
|
||||
|
@ -59,11 +60,37 @@ class TableSystem(object):
|
|||
self.text_recognizer = predict_rec.TextRecognizer(
|
||||
args) if text_recognizer is None else text_recognizer
|
||||
self.table_structurer = predict_strture.TableStructurer(args)
|
||||
self.benchmark = args.benchmark
|
||||
self.predictor, self.input_tensor, self.output_tensors, self.config = utility.create_predictor(
|
||||
args, 'table', logger)
|
||||
if args.benchmark:
|
||||
import auto_log
|
||||
pid = os.getpid()
|
||||
gpu_id = utility.get_infer_gpuid()
|
||||
self.autolog = auto_log.AutoLogger(
|
||||
model_name="table",
|
||||
model_precision=args.precision,
|
||||
batch_size=1,
|
||||
data_shape="dynamic",
|
||||
save_path=None, #args.save_log_path,
|
||||
inference_config=self.config,
|
||||
pids=pid,
|
||||
process_name=None,
|
||||
gpu_ids=gpu_id if args.use_gpu else None,
|
||||
time_keys=[
|
||||
'preprocess_time', 'inference_time', 'postprocess_time'
|
||||
],
|
||||
warmup=0,
|
||||
logger=logger)
|
||||
|
||||
def __call__(self, img, return_ocr_result_in_table=False):
|
||||
result = dict()
|
||||
ori_im = img.copy()
|
||||
if self.benchmark:
|
||||
self.autolog.times.start()
|
||||
structure_res, elapse = self.table_structurer(copy.deepcopy(img))
|
||||
if self.benchmark:
|
||||
self.autolog.times.stamp()
|
||||
dt_boxes, elapse = self.text_detector(copy.deepcopy(img))
|
||||
dt_boxes = sorted_boxes(dt_boxes)
|
||||
if return_ocr_result_in_table:
|
||||
|
@ -77,13 +104,11 @@ class TableSystem(object):
|
|||
box = [x_min, y_min, x_max, y_max]
|
||||
r_boxes.append(box)
|
||||
dt_boxes = np.array(r_boxes)
|
||||
|
||||
logger.debug("dt_boxes num : {}, elapse : {}".format(
|
||||
len(dt_boxes), elapse))
|
||||
if dt_boxes is None:
|
||||
return None, None
|
||||
img_crop_list = []
|
||||
|
||||
for i in range(len(dt_boxes)):
|
||||
det_box = dt_boxes[i]
|
||||
x0, y0, x1, y1 = expand(2, det_box, ori_im.shape)
|
||||
|
@ -92,10 +117,14 @@ class TableSystem(object):
|
|||
rec_res, elapse = self.text_recognizer(img_crop_list)
|
||||
logger.debug("rec_res num : {}, elapse : {}".format(
|
||||
len(rec_res), elapse))
|
||||
if self.benchmark:
|
||||
self.autolog.times.stamp()
|
||||
if return_ocr_result_in_table:
|
||||
result['rec_res'] = rec_res
|
||||
pred_html, pred = self.rebuild_table(structure_res, dt_boxes, rec_res)
|
||||
result['html'] = pred_html
|
||||
if self.benchmark:
|
||||
self.autolog.times.end(stamp=True)
|
||||
return result
|
||||
|
||||
def rebuild_table(self, structure_res, dt_boxes, rec_res):
|
||||
|
@ -213,6 +242,8 @@ def main(args):
|
|||
logger.info('excel saved to {}'.format(excel_path))
|
||||
elapse = time.time() - starttime
|
||||
logger.info("Predict time : {:.3f}s".format(elapse))
|
||||
if args.benchmark:
|
||||
text_sys.autolog.report()
|
||||
|
||||
|
||||
if __name__ == "__main__":
|
||||
|
|
|
@ -0,0 +1,69 @@
|
|||
#使用镜像:
|
||||
#registry.baidubce.com/paddlepaddle/paddle:latest-dev-cuda10.1-cudnn7-gcc82
|
||||
|
||||
#编译Serving Server:
|
||||
|
||||
#client和app可以直接使用release版本
|
||||
|
||||
#server因为加入了自定义OP,需要重新编译
|
||||
|
||||
apt-get update
|
||||
apt install -y libcurl4-openssl-dev libbz2-dev
|
||||
wget https://paddle-serving.bj.bcebos.com/others/centos_ssl.tar && tar xf centos_ssl.tar && rm -rf centos_ssl.tar && mv libcrypto.so.1.0.2k /usr/lib/libcrypto.so.1.0.2k && mv libssl.so.1.0.2k /usr/lib/libssl.so.1.0.2k && ln -sf /usr/lib/libcrypto.so.1.0.2k /usr/lib/libcrypto.so.10 && ln -sf /usr/lib/libssl.so.1.0.2k /usr/lib/libssl.so.10 && ln -sf /usr/lib/libcrypto.so.10 /usr/lib/libcrypto.so && ln -sf /usr/lib/libssl.so.10 /usr/lib/libssl.so
|
||||
|
||||
# 安装go依赖
|
||||
rm -rf /usr/local/go
|
||||
wget -qO- https://paddle-ci.cdn.bcebos.com/go1.17.2.linux-amd64.tar.gz | tar -xz -C /usr/local
|
||||
export GOROOT=/usr/local/go
|
||||
export GOPATH=/root/gopath
|
||||
export PATH=$PATH:$GOPATH/bin:$GOROOT/bin
|
||||
go env -w GO111MODULE=on
|
||||
go env -w GOPROXY=https://goproxy.cn,direct
|
||||
go install github.com/grpc-ecosystem/grpc-gateway/protoc-gen-grpc-gateway@v1.15.2
|
||||
go install github.com/grpc-ecosystem/grpc-gateway/protoc-gen-swagger@v1.15.2
|
||||
go install github.com/golang/protobuf/protoc-gen-go@v1.4.3
|
||||
go install google.golang.org/grpc@v1.33.0
|
||||
go env -w GO111MODULE=auto
|
||||
|
||||
# 下载opencv库
|
||||
wget https://paddle-qa.bj.bcebos.com/PaddleServing/opencv3.tar.gz && tar -xvf opencv3.tar.gz && rm -rf opencv3.tar.gz
|
||||
export OPENCV_DIR=$PWD/opencv3
|
||||
|
||||
# clone Serving
|
||||
git clone https://github.com/PaddlePaddle/Serving.git -b develop --depth=1
|
||||
cd Serving
|
||||
export Serving_repo_path=$PWD
|
||||
git submodule update --init --recursive
|
||||
python -m pip install -r python/requirements.txt
|
||||
|
||||
|
||||
export PYTHON_INCLUDE_DIR=$(python -c "from distutils.sysconfig import get_python_inc; print(get_python_inc())")
|
||||
export PYTHON_LIBRARIES=$(python -c "import distutils.sysconfig as sysconfig; print(sysconfig.get_config_var('LIBDIR'))")
|
||||
export PYTHON_EXECUTABLE=`which python`
|
||||
|
||||
export CUDA_PATH='/usr/local/cuda'
|
||||
export CUDNN_LIBRARY='/usr/local/cuda/lib64/'
|
||||
export CUDA_CUDART_LIBRARY='/usr/local/cuda/lib64/'
|
||||
export TENSORRT_LIBRARY_PATH='/usr/local/TensorRT6-cuda10.1-cudnn7/targets/x86_64-linux-gnu/'
|
||||
|
||||
# cp 自定义OP代码
|
||||
cp -rf ../deploy/pdserving/general_detection_op.cpp ${Serving_repo_path}/core/general-server/op
|
||||
|
||||
# 编译Server, export SERVING_BIN
|
||||
mkdir server-build-gpu-opencv && cd server-build-gpu-opencv
|
||||
cmake -DPYTHON_INCLUDE_DIR=$PYTHON_INCLUDE_DIR \
|
||||
-DPYTHON_LIBRARIES=$PYTHON_LIBRARIES \
|
||||
-DPYTHON_EXECUTABLE=$PYTHON_EXECUTABLE \
|
||||
-DCUDA_TOOLKIT_ROOT_DIR=${CUDA_PATH} \
|
||||
-DCUDNN_LIBRARY=${CUDNN_LIBRARY} \
|
||||
-DCUDA_CUDART_LIBRARY=${CUDA_CUDART_LIBRARY} \
|
||||
-DTENSORRT_ROOT=${TENSORRT_LIBRARY_PATH} \
|
||||
-DOPENCV_DIR=${OPENCV_DIR} \
|
||||
-DWITH_OPENCV=ON \
|
||||
-DSERVER=ON \
|
||||
-DWITH_GPU=ON ..
|
||||
make -j32
|
||||
|
||||
python -m pip install python/dist/paddle*
|
||||
export SERVING_BIN=$PWD/core/general-server/serving
|
||||
cd ../../
|
|
@ -57,10 +57,11 @@ function status_check(){
|
|||
last_status=$1 # the exit code
|
||||
run_command=$2
|
||||
run_log=$3
|
||||
model_name=$4
|
||||
if [ $last_status -eq 0 ]; then
|
||||
echo -e "\033[33m Run successfully with command - ${run_command}! \033[0m" | tee -a ${run_log}
|
||||
echo -e "\033[33m Run successfully with command - ${model_name} - ${run_command}! \033[0m" | tee -a ${run_log}
|
||||
else
|
||||
echo -e "\033[33m Run failed with command - ${run_command}! \033[0m" | tee -a ${run_log}
|
||||
echo -e "\033[33m Run failed with command - ${model_name} - ${run_command}! \033[0m" | tee -a ${run_log}
|
||||
fi
|
||||
}
|
||||
|
||||
|
|
|
@ -0,0 +1,20 @@
|
|||
===========================cpp_infer_params===========================
|
||||
model_name:ch_PP-OCRv2
|
||||
use_opencv:True
|
||||
infer_model:./inference/ch_PP-OCRv2_det_infer/
|
||||
infer_quant:False
|
||||
inference:./deploy/cpp_infer/build/ppocr --rec_char_dict_path=./ppocr/utils/ppocr_keys_v1.txt --rec_img_h=32
|
||||
--use_gpu:True|False
|
||||
--enable_mkldnn:False
|
||||
--cpu_threads:6
|
||||
--rec_batch_num:1
|
||||
--use_tensorrt:False
|
||||
--precision:fp32
|
||||
--det_model_dir:
|
||||
--image_dir:./inference/ch_det_data_50/all-sum-510/
|
||||
--rec_model_dir:./inference/ch_PP-OCRv2_rec_infer/
|
||||
--benchmark:True
|
||||
--det:True
|
||||
--rec:True
|
||||
--cls:False
|
||||
--use_angle_cls:False
|
|
@ -6,10 +6,10 @@ infer_export:null
|
|||
infer_quant:False
|
||||
inference:tools/infer/predict_system.py
|
||||
--use_gpu:False|True
|
||||
--enable_mkldnn:False|True
|
||||
--cpu_threads:1|6
|
||||
--enable_mkldnn:False
|
||||
--cpu_threads:6
|
||||
--rec_batch_num:1
|
||||
--use_tensorrt:False|True
|
||||
--use_tensorrt:False
|
||||
--precision:fp32
|
||||
--det_model_dir:
|
||||
--image_dir:./inference/ch_det_data_50/all-sum-510/
|
||||
|
|
|
@ -0,0 +1,17 @@
|
|||
===========================paddle2onnx_params===========================
|
||||
model_name:ch_PP-OCRv2
|
||||
python:python3.7
|
||||
2onnx: paddle2onnx
|
||||
--det_model_dir:./inference/ch_PP-OCRv2_det_infer/
|
||||
--model_filename:inference.pdmodel
|
||||
--params_filename:inference.pdiparams
|
||||
--det_save_file:./inference/det_v2_onnx/model.onnx
|
||||
--rec_model_dir:./inference/ch_PP-OCRv2_rec_infer/
|
||||
--rec_save_file:./inference/rec_v2_onnx/model.onnx
|
||||
--opset_version:10
|
||||
--enable_onnx_checker:True
|
||||
inference:tools/infer/predict_system.py --rec_image_shape="3,32,320"
|
||||
--use_gpu:True|False
|
||||
--det_model_dir:
|
||||
--rec_model_dir:
|
||||
--image_dir:./inference/ch_det_data_50/all-sum-510/00008790.jpg
|
|
@ -0,0 +1,19 @@
|
|||
===========================serving_params===========================
|
||||
model_name:ch_PP-OCRv2
|
||||
python:python3.7
|
||||
trans_model:-m paddle_serving_client.convert
|
||||
--det_dirname:./inference/ch_PP-OCRv2_det_infer/
|
||||
--model_filename:inference.pdmodel
|
||||
--params_filename:inference.pdiparams
|
||||
--det_serving_server:./deploy/pdserving/ppocr_det_v2_serving/
|
||||
--det_serving_client:./deploy/pdserving/ppocr_det_v2_client/
|
||||
--rec_dirname:./inference/ch_PP-OCRv2_rec_infer/
|
||||
--rec_serving_server:./deploy/pdserving/ppocr_rec_v2_serving/
|
||||
--rec_serving_client:./deploy/pdserving/ppocr_rec_v2_client/
|
||||
serving_dir:./deploy/pdserving
|
||||
web_service:-m paddle_serving_server.serve
|
||||
--op:GeneralDetectionOp GeneralInferOp
|
||||
--port:8181
|
||||
--gpu_id:"0"|null
|
||||
cpp_client:ocr_cpp_client.py
|
||||
--image_dir:../../doc/imgs/1.jpg
|
|
@ -0,0 +1,23 @@
|
|||
===========================serving_params===========================
|
||||
model_name:ch_PP-OCRv2
|
||||
python:python3.7
|
||||
trans_model:-m paddle_serving_client.convert
|
||||
--det_dirname:./inference/ch_PP-OCRv2_det_infer/
|
||||
--model_filename:inference.pdmodel
|
||||
--params_filename:inference.pdiparams
|
||||
--det_serving_server:./deploy/pdserving/ppocr_det_v2_serving/
|
||||
--det_serving_client:./deploy/pdserving/ppocr_det_v2_client/
|
||||
--rec_dirname:./inference/ch_PP-OCRv2_rec_infer/
|
||||
--rec_serving_server:./deploy/pdserving/ppocr_rec_v2_serving/
|
||||
--rec_serving_client:./deploy/pdserving/ppocr_rec_v2_client/
|
||||
serving_dir:./deploy/pdserving
|
||||
web_service:web_service.py --config=config.yml --opt op.det.concurrency="1" op.rec.concurrency="1"
|
||||
op.det.local_service_conf.devices:gpu|null
|
||||
op.det.local_service_conf.use_mkldnn:False
|
||||
op.det.local_service_conf.thread_num:6
|
||||
op.det.local_service_conf.use_trt:False
|
||||
op.det.local_service_conf.precision:fp32
|
||||
op.det.local_service_conf.model_config:
|
||||
op.rec.local_service_conf.model_config:
|
||||
pipline:pipeline_http_client.py
|
||||
--image_dir:../../doc/imgs/1.jpg
|
|
@ -0,0 +1,20 @@
|
|||
===========================cpp_infer_params===========================
|
||||
model_name:ch_PP-OCRv2_det
|
||||
use_opencv:True
|
||||
infer_model:./inference/ch_PP-OCRv2_det_infer/
|
||||
infer_quant:False
|
||||
inference:./deploy/cpp_infer/build/ppocr
|
||||
--use_gpu:True|False
|
||||
--enable_mkldnn:False
|
||||
--cpu_threads:6
|
||||
--rec_batch_num:1
|
||||
--use_tensorrt:False
|
||||
--precision:fp32
|
||||
--det_model_dir:
|
||||
--image_dir:./inference/ch_det_data_50/all-sum-510/
|
||||
null:null
|
||||
--benchmark:True
|
||||
--det:True
|
||||
--rec:False
|
||||
--cls:False
|
||||
--use_angle_cls:False
|
|
@ -0,0 +1,17 @@
|
|||
===========================paddle2onnx_params===========================
|
||||
model_name:ch_PP-OCRv2_det
|
||||
python:python3.7
|
||||
2onnx: paddle2onnx
|
||||
--det_model_dir:./inference/ch_PP-OCRv2_det_infer/
|
||||
--model_filename:inference.pdmodel
|
||||
--params_filename:inference.pdiparams
|
||||
--det_save_file:./inference/det_v2_onnx/model.onnx
|
||||
--rec_model_dir:
|
||||
--rec_save_file:
|
||||
--opset_version:10
|
||||
--enable_onnx_checker:True
|
||||
inference:tools/infer/predict_det.py
|
||||
--use_gpu:True|False
|
||||
--det_model_dir:
|
||||
--rec_model_dir:
|
||||
--image_dir:./inference/ch_det_data_50/all-sum-510/
|
|
@ -0,0 +1,23 @@
|
|||
===========================serving_params===========================
|
||||
model_name:ch_PP-OCRv2_det
|
||||
python:python3.7
|
||||
trans_model:-m paddle_serving_client.convert
|
||||
--det_dirname:./inference/ch_PP-OCRv2_det_infer/
|
||||
--model_filename:inference.pdmodel
|
||||
--params_filename:inference.pdiparams
|
||||
--det_serving_server:./deploy/pdserving/ppocr_det_v2_serving/
|
||||
--det_serving_client:./deploy/pdserving/ppocr_det_v2_client/
|
||||
--rec_dirname:null
|
||||
--rec_serving_server:null
|
||||
--rec_serving_client:null
|
||||
serving_dir:./deploy/pdserving
|
||||
web_service:web_service_det.py --config=config.yml --opt op.det.concurrency="1"
|
||||
op.det.local_service_conf.devices:gpu|null
|
||||
op.det.local_service_conf.use_mkldnn:False
|
||||
op.det.local_service_conf.thread_num:6
|
||||
op.det.local_service_conf.use_trt:False
|
||||
op.det.local_service_conf.precision:fp32
|
||||
op.det.local_service_conf.model_config:
|
||||
op.rec.local_service_conf.model_config:
|
||||
pipline:pipeline_http_client.py
|
||||
--image_dir:../../doc/imgs/1.jpg
|
|
@ -1,10 +1,10 @@
|
|||
===========================train_params===========================
|
||||
model_name:ch_PPOCRv2_det
|
||||
model_name:ch_PP-OCRv2_det
|
||||
python:python3.7
|
||||
gpu_list:0|0,1
|
||||
Global.use_gpu:True|True
|
||||
Global.auto_cast:fp32
|
||||
Global.epoch_num:lite_train_lite_infer=1|whole_train_whole_infer=500
|
||||
Global.epoch_num:lite_train_lite_infer=1|whole_train_whole_infer=50
|
||||
Global.save_model_dir:./output/
|
||||
Train.loader.batch_size_per_card:lite_train_lite_infer=2|whole_train_whole_infer=4
|
||||
Global.pretrained_model:null
|
||||
|
@ -39,11 +39,11 @@ infer_export:null
|
|||
infer_quant:False
|
||||
inference:tools/infer/predict_det.py
|
||||
--use_gpu:True|False
|
||||
--enable_mkldnn:True|False
|
||||
--cpu_threads:1|6
|
||||
--enable_mkldnn:False
|
||||
--cpu_threads:6
|
||||
--rec_batch_num:1
|
||||
--use_tensorrt:False|True
|
||||
--precision:fp32|fp16|int8
|
||||
--use_tensorrt:False
|
||||
--precision:fp32
|
||||
--det_model_dir:
|
||||
--image_dir:./inference/ch_det_data_50/all-sum-510/
|
||||
null:null
|
||||
|
|
|
@ -0,0 +1,53 @@
|
|||
===========================train_params===========================
|
||||
model_name:ch_PP-OCRv2_det
|
||||
python:python3.7
|
||||
gpu_list:192.168.0.1,192.168.0.2;0,1
|
||||
Global.use_gpu:True
|
||||
Global.auto_cast:fp32
|
||||
Global.epoch_num:lite_train_lite_infer=1|whole_train_whole_infer=50
|
||||
Global.save_model_dir:./output/
|
||||
Train.loader.batch_size_per_card:lite_train_lite_infer=2|whole_train_whole_infer=4
|
||||
Global.pretrained_model:null
|
||||
train_model_name:latest
|
||||
train_infer_img_dir:./train_data/icdar2015/text_localization/ch4_test_images/
|
||||
null:null
|
||||
##
|
||||
trainer:norm_train
|
||||
norm_train:tools/train.py -c configs/det/ch_PP-OCRv2/ch_PP-OCRv2_det_cml.yml -o
|
||||
pact_train:null
|
||||
fpgm_train:null
|
||||
distill_train:null
|
||||
null:null
|
||||
null:null
|
||||
##
|
||||
===========================eval_params===========================
|
||||
eval:null
|
||||
null:null
|
||||
##
|
||||
===========================infer_params===========================
|
||||
Global.save_inference_dir:./output/
|
||||
Global.checkpoints:
|
||||
norm_export:tools/export_model.py -c configs/det/ch_PP-OCRv2/ch_PP-OCRv2_det_cml.yml -o
|
||||
quant_export:null
|
||||
fpgm_export:
|
||||
distill_export:null
|
||||
export1:null
|
||||
export2:null
|
||||
inference_dir:Student
|
||||
infer_model:./inference/ch_PP-OCRv2_det_infer/
|
||||
infer_export:null
|
||||
infer_quant:False
|
||||
inference:tools/infer/predict_det.py
|
||||
--use_gpu:False
|
||||
--enable_mkldnn:False
|
||||
--cpu_threads:6
|
||||
--rec_batch_num:1
|
||||
--use_tensorrt:False
|
||||
--precision:fp32
|
||||
--det_model_dir:
|
||||
--image_dir:./inference/ch_det_data_50/all-sum-510/
|
||||
null:null
|
||||
--benchmark:True
|
||||
null:null
|
||||
===========================infer_benchmark_params==========================
|
||||
random_infer_input:[{float32,[3,640,640]}];[{float32,[3,960,960]}]
|
|
@ -1,10 +1,10 @@
|
|||
===========================train_params===========================
|
||||
model_name:ch_PPOCRv2_det
|
||||
model_name:ch_PP-OCRv2_det
|
||||
python:python3.7
|
||||
gpu_list:0|0,1
|
||||
Global.use_gpu:True|True
|
||||
Global.auto_cast:amp
|
||||
Global.epoch_num:lite_train_lite_infer=1|whole_train_whole_infer=500
|
||||
Global.epoch_num:lite_train_lite_infer=1|whole_train_whole_infer=50
|
||||
Global.save_model_dir:./output/
|
||||
Train.loader.batch_size_per_card:lite_train_lite_infer=2|whole_train_whole_infer=4
|
||||
Global.pretrained_model:null
|
||||
|
@ -39,11 +39,11 @@ infer_export:null
|
|||
infer_quant:False
|
||||
inference:tools/infer/predict_det.py
|
||||
--use_gpu:True|False
|
||||
--enable_mkldnn:True|False
|
||||
--cpu_threads:1|6
|
||||
--enable_mkldnn:False
|
||||
--cpu_threads:6
|
||||
--rec_batch_num:1
|
||||
--use_tensorrt:False|True
|
||||
--precision:fp32|fp16|int8
|
||||
--use_tensorrt:False
|
||||
--precision:fp32
|
||||
--det_model_dir:
|
||||
--image_dir:./inference/ch_det_data_50/all-sum-510/
|
||||
null:null
|
||||
|
|
|
@ -0,0 +1,20 @@
|
|||
===========================cpp_infer_params===========================
|
||||
model_name:ch_PP-OCRv2_det_KL
|
||||
use_opencv:True
|
||||
infer_model:./inference/ch_PP-OCRv2_det_klquant_infer
|
||||
infer_quant:False
|
||||
inference:./deploy/cpp_infer/build/ppocr
|
||||
--use_gpu:True|False
|
||||
--enable_mkldnn:False
|
||||
--cpu_threads:6
|
||||
--rec_batch_num:1
|
||||
--use_tensorrt:False
|
||||
--precision:fp32
|
||||
--det_model_dir:
|
||||
--image_dir:./inference/ch_det_data_50/all-sum-510/
|
||||
null:null
|
||||
--benchmark:True
|
||||
--det:True
|
||||
--rec:False
|
||||
--cls:False
|
||||
--use_angle_cls:False
|
|
@ -1,5 +1,5 @@
|
|||
===========================kl_quant_params===========================
|
||||
model_name:PPOCRv2_ocr_det_kl
|
||||
model_name:ch_PP-OCRv2_det_KL
|
||||
python:python3.7
|
||||
Global.pretrained_model:null
|
||||
Global.save_inference_dir:null
|
||||
|
@ -8,10 +8,10 @@ infer_export:deploy/slim/quantization/quant_kl.py -c configs/det/ch_PP-OCRv2/ch_
|
|||
infer_quant:True
|
||||
inference:tools/infer/predict_det.py
|
||||
--use_gpu:False|True
|
||||
--enable_mkldnn:True
|
||||
--cpu_threads:1|6
|
||||
--enable_mkldnn:False
|
||||
--cpu_threads:6
|
||||
--rec_batch_num:1
|
||||
--use_tensorrt:False|True
|
||||
--use_tensorrt:False
|
||||
--precision:int8
|
||||
--det_model_dir:
|
||||
--image_dir:./inference/ch_det_data_50/all-sum-510/
|
||||
|
|
|
@ -0,0 +1,19 @@
|
|||
===========================serving_params===========================
|
||||
model_name:ch_PP-OCRv2_det_KL
|
||||
python:python3.7
|
||||
trans_model:-m paddle_serving_client.convert
|
||||
--det_dirname:./inference/ch_PP-OCRv2_det_klquant_infer/
|
||||
--model_filename:inference.pdmodel
|
||||
--params_filename:inference.pdiparams
|
||||
--det_serving_server:./deploy/pdserving/ppocr_det_v2_kl_serving/
|
||||
--det_serving_client:./deploy/pdserving/ppocr_det_v2_kl_client/
|
||||
--rec_dirname:./inference/ch_PP-OCRv2_rec_klquant_infer/
|
||||
--rec_serving_server:./deploy/pdserving/ppocr_rec_v2_kl_serving/
|
||||
--rec_serving_client:./deploy/pdserving/ppocr_rec_v2_kl_client/
|
||||
serving_dir:./deploy/pdserving
|
||||
web_service:-m paddle_serving_server.serve
|
||||
--op:GeneralDetectionOp GeneralInferOp
|
||||
--port:8181
|
||||
--gpu_id:"0"|null
|
||||
cpp_client:ocr_cpp_client.py
|
||||
--image_dir:../../doc/imgs/1.jpg
|
|
@ -0,0 +1,23 @@
|
|||
===========================serving_params===========================
|
||||
model_name:ch_PP-OCRv2_det_KL
|
||||
python:python3.7
|
||||
trans_model:-m paddle_serving_client.convert
|
||||
--det_dirname:./inference/ch_PP-OCRv2_det_klquant_infer/
|
||||
--model_filename:inference.pdmodel
|
||||
--params_filename:inference.pdiparams
|
||||
--det_serving_server:./deploy/pdserving/ppocr_det_v2_kl_serving/
|
||||
--det_serving_client:./deploy/pdserving/ppocr_det_v2_kl_client/
|
||||
--rec_dirname:null
|
||||
--rec_serving_server:null
|
||||
--rec_serving_client:null
|
||||
serving_dir:./deploy/pdserving
|
||||
web_service:web_service_det.py --config=config.yml --opt op.det.concurrency="1"
|
||||
op.det.local_service_conf.devices:gpu|null
|
||||
op.det.local_service_conf.use_mkldnn:False
|
||||
op.det.local_service_conf.thread_num:6
|
||||
op.det.local_service_conf.use_trt:False
|
||||
op.det.local_service_conf.precision:fp32
|
||||
op.det.local_service_conf.model_config:
|
||||
op.rec.local_service_conf.model_config:
|
||||
pipline:pipeline_http_client.py
|
||||
--image_dir:../../doc/imgs/1.jpg
|
|
@ -0,0 +1,20 @@
|
|||
===========================cpp_infer_params===========================
|
||||
model_name:ch_PP-OCRv2_det_PACT
|
||||
use_opencv:True
|
||||
infer_model:./inference/ch_PP-OCRv2_det_pact_infer
|
||||
infer_quant:False
|
||||
inference:./deploy/cpp_infer/build/ppocr
|
||||
--use_gpu:True|False
|
||||
--enable_mkldnn:False
|
||||
--cpu_threads:6
|
||||
--rec_batch_num:1
|
||||
--use_tensorrt:False
|
||||
--precision:fp32
|
||||
--det_model_dir:
|
||||
--image_dir:./inference/ch_det_data_50/all-sum-510/
|
||||
null:null
|
||||
--benchmark:True
|
||||
--det:True
|
||||
--rec:False
|
||||
--cls:False
|
||||
--use_angle_cls:False
|
|
@ -0,0 +1,19 @@
|
|||
===========================serving_params===========================
|
||||
model_name:ch_PP-OCRv2_det_PACT
|
||||
python:python3.7
|
||||
trans_model:-m paddle_serving_client.convert
|
||||
--det_dirname:./inference/ch_PP-OCRv2_det_pact_infer/
|
||||
--model_filename:inference.pdmodel
|
||||
--params_filename:inference.pdiparams
|
||||
--det_serving_server:./deploy/pdserving/ppocr_det_v2_pact_serving/
|
||||
--det_serving_client:./deploy/pdserving/ppocr_det_v2_pact_client/
|
||||
--rec_dirname:./inference/ch_PP-OCRv2_rec_pact_infer/
|
||||
--rec_serving_server:./deploy/pdserving/ppocr_rec_v2_pact_serving/
|
||||
--rec_serving_client:./deploy/pdserving/ppocr_rec_v2_pact_client/
|
||||
serving_dir:./deploy/pdserving
|
||||
web_service:-m paddle_serving_server.serve
|
||||
--op:GeneralDetectionOp GeneralInferOp
|
||||
--port:8181
|
||||
--gpu_id:"0"|null
|
||||
cpp_client:ocr_cpp_client.py
|
||||
--image_dir:../../doc/imgs/1.jpg
|
|
@ -0,0 +1,23 @@
|
|||
===========================serving_params===========================
|
||||
model_name:ch_PP-OCRv2_det_PACT
|
||||
python:python3.7
|
||||
trans_model:-m paddle_serving_client.convert
|
||||
--det_dirname:./inference/ch_PP-OCRv2_det_pact_infer/
|
||||
--model_filename:inference.pdmodel
|
||||
--params_filename:inference.pdiparams
|
||||
--det_serving_server:./deploy/pdserving/ppocr_det_v2_pact_serving/
|
||||
--det_serving_client:./deploy/pdserving/ppocr_det_v2_pact_client/
|
||||
--rec_dirname:null
|
||||
--rec_serving_server:null
|
||||
--rec_serving_client:null
|
||||
serving_dir:./deploy/pdserving
|
||||
web_service:web_service_det.py --config=config.yml --opt op.det.concurrency="1"
|
||||
op.det.local_service_conf.devices:gpu|null
|
||||
op.det.local_service_conf.use_mkldnn:False
|
||||
op.det.local_service_conf.thread_num:6
|
||||
op.det.local_service_conf.use_trt:False
|
||||
op.det.local_service_conf.precision:fp32
|
||||
op.det.local_service_conf.model_config:
|
||||
op.rec.local_service_conf.model_config:
|
||||
pipline:pipeline_http_client.py
|
||||
--image_dir:../../doc/imgs/1.jpg
|
|
@ -1,10 +1,10 @@
|
|||
===========================train_params===========================
|
||||
model_name:ch_PPOCRv2_det_PACT
|
||||
model_name:ch_PP-OCRv2_det_PACT
|
||||
python:python3.7
|
||||
gpu_list:0|0,1
|
||||
Global.use_gpu:True|True
|
||||
Global.auto_cast:fp32
|
||||
Global.epoch_num:lite_train_lite_infer=1|whole_train_whole_infer=500
|
||||
Global.epoch_num:lite_train_lite_infer=1|whole_train_whole_infer=50
|
||||
Global.save_model_dir:./output/
|
||||
Train.loader.batch_size_per_card:lite_train_lite_infer=1|whole_train_whole_infer=4
|
||||
Global.pretrained_model:null
|
||||
|
@ -39,11 +39,11 @@ infer_export:null
|
|||
infer_quant:False
|
||||
inference:tools/infer/predict_det.py
|
||||
--use_gpu:True|False
|
||||
--enable_mkldnn:True|False
|
||||
--cpu_threads:1|6
|
||||
--enable_mkldnn:False
|
||||
--cpu_threads:6
|
||||
--rec_batch_num:1
|
||||
--use_tensorrt:False|True
|
||||
--precision:fp32|fp16|int8
|
||||
--use_tensorrt:False
|
||||
--precision:fp32
|
||||
--det_model_dir:
|
||||
--image_dir:./inference/ch_det_data_50/all-sum-510/
|
||||
null:null
|
||||
|
|
|
@ -1,10 +1,10 @@
|
|||
===========================train_params===========================
|
||||
model_name:ch_PPOCRv2_det_PACT
|
||||
model_name:ch_PP-OCRv2_det_PACT
|
||||
python:python3.7
|
||||
gpu_list:0|0,1
|
||||
Global.use_gpu:True|True
|
||||
Global.auto_cast:amp
|
||||
Global.epoch_num:lite_train_lite_infer=1|whole_train_whole_infer=500
|
||||
Global.epoch_num:lite_train_lite_infer=1|whole_train_whole_infer=50
|
||||
Global.save_model_dir:./output/
|
||||
Train.loader.batch_size_per_card:lite_train_lite_infer=2|whole_train_whole_infer=4
|
||||
Global.pretrained_model:null
|
||||
|
@ -39,11 +39,11 @@ infer_export:null
|
|||
infer_quant:False
|
||||
inference:tools/infer/predict_det.py
|
||||
--use_gpu:True|False
|
||||
--enable_mkldnn:True|False
|
||||
--cpu_threads:1|6
|
||||
--enable_mkldnn:False
|
||||
--cpu_threads:6
|
||||
--rec_batch_num:1
|
||||
--use_tensorrt:False|True
|
||||
--precision:fp32|fp16|int8
|
||||
--use_tensorrt:False
|
||||
--precision:fp32
|
||||
--det_model_dir:
|
||||
--image_dir:./inference/ch_det_data_50/all-sum-510/
|
||||
null:null
|
||||
|
|
|
@ -0,0 +1,20 @@
|
|||
===========================cpp_infer_params===========================
|
||||
model_name:ch_PP-OCRv2_rec
|
||||
use_opencv:True
|
||||
infer_model:./inference/ch_PP-OCRv2_rec_infer/
|
||||
infer_quant:False
|
||||
inference:./deploy/cpp_infer/build/ppocr --rec_char_dict_path=./ppocr/utils/ppocr_keys_v1.txt --rec_img_h=32
|
||||
--use_gpu:True|False
|
||||
--enable_mkldnn:False
|
||||
--cpu_threads:6
|
||||
--rec_batch_num:6
|
||||
--use_tensorrt:False
|
||||
--precision:fp32
|
||||
--rec_model_dir:
|
||||
--image_dir:./inference/rec_inference/
|
||||
null:null
|
||||
--benchmark:True
|
||||
--det:False
|
||||
--rec:True
|
||||
--cls:False
|
||||
--use_angle_cls:False
|
|
@ -0,0 +1,17 @@
|
|||
===========================paddle2onnx_params===========================
|
||||
model_name:ch_PP-OCRv2_rec
|
||||
python:python3.7
|
||||
2onnx: paddle2onnx
|
||||
--det_model_dir:
|
||||
--model_filename:inference.pdmodel
|
||||
--params_filename:inference.pdiparams
|
||||
--det_save_file:
|
||||
--rec_model_dir:./inference/ch_PP-OCRv2_rec_infer/
|
||||
--rec_save_file:./inference/rec_v2_onnx/model.onnx
|
||||
--opset_version:10
|
||||
--enable_onnx_checker:True
|
||||
inference:tools/infer/predict_rec.py --rec_image_shape="3,32,320"
|
||||
--use_gpu:True|False
|
||||
--det_model_dir:
|
||||
--rec_model_dir:
|
||||
--image_dir:./inference/rec_inference/
|
|
@ -0,0 +1,23 @@
|
|||
===========================serving_params===========================
|
||||
model_name:ch_PP-OCRv2_rec
|
||||
python:python3.7
|
||||
trans_model:-m paddle_serving_client.convert
|
||||
--det_dirname:null
|
||||
--model_filename:inference.pdmodel
|
||||
--params_filename:inference.pdiparams
|
||||
--det_serving_server:null
|
||||
--det_serving_client:null
|
||||
--rec_dirname:./inference/ch_PP-OCRv2_rec_infer/
|
||||
--rec_serving_server:./deploy/pdserving/ppocr_rec_v2_serving/
|
||||
--rec_serving_client:./deploy/pdserving/ppocr_rec_v2_client/
|
||||
serving_dir:./deploy/pdserving
|
||||
web_service:web_service_rec.py --config=config.yml --opt op.rec.concurrency="1"
|
||||
op.det.local_service_conf.devices:gpu|null
|
||||
op.det.local_service_conf.use_mkldnn:False
|
||||
op.det.local_service_conf.thread_num:6
|
||||
op.det.local_service_conf.use_trt:False
|
||||
op.det.local_service_conf.precision:fp32
|
||||
op.det.local_service_conf.model_config:
|
||||
op.rec.local_service_conf.model_config:
|
||||
pipline:pipeline_http_client.py --det=False
|
||||
--image_dir:../../inference/rec_inference
|
|
@ -1,10 +1,10 @@
|
|||
===========================train_params===========================
|
||||
model_name:PPOCRv2_ocr_rec
|
||||
model_name:ch_PP-OCRv2_rec
|
||||
python:python3.7
|
||||
gpu_list:0|0,1
|
||||
Global.use_gpu:True|True
|
||||
Global.auto_cast:fp32
|
||||
Global.epoch_num:lite_train_lite_infer=3|whole_train_whole_infer=300
|
||||
Global.epoch_num:lite_train_lite_infer=3|whole_train_whole_infer=50
|
||||
Global.save_model_dir:./output/
|
||||
Train.loader.batch_size_per_card:lite_train_lite_infer=16|whole_train_whole_infer=128
|
||||
Global.pretrained_model:null
|
||||
|
@ -39,11 +39,11 @@ infer_export:null
|
|||
infer_quant:False
|
||||
inference:tools/infer/predict_rec.py
|
||||
--use_gpu:True|False
|
||||
--enable_mkldnn:True|False
|
||||
--cpu_threads:1|6
|
||||
--enable_mkldnn:False
|
||||
--cpu_threads:6
|
||||
--rec_batch_num:1|6
|
||||
--use_tensorrt:False|True
|
||||
--precision:fp32|int8
|
||||
--use_tensorrt:False
|
||||
--precision:fp32
|
||||
--rec_model_dir:
|
||||
--image_dir:./inference/rec_inference
|
||||
null:null
|
||||
|
|
|
@ -0,0 +1,53 @@
|
|||
===========================train_params===========================
|
||||
model_name:ch_PP-OCRv2_rec
|
||||
python:python3.7
|
||||
gpu_list:192.168.0.1,192.168.0.2;0,1
|
||||
Global.use_gpu:True
|
||||
Global.auto_cast:fp32
|
||||
Global.epoch_num:lite_train_lite_infer=3|whole_train_whole_infer=50
|
||||
Global.save_model_dir:./output/
|
||||
Train.loader.batch_size_per_card:lite_train_lite_infer=16|whole_train_whole_infer=128
|
||||
Global.pretrained_model:null
|
||||
train_model_name:latest
|
||||
train_infer_img_dir:./inference/rec_inference
|
||||
null:null
|
||||
##
|
||||
trainer:norm_train
|
||||
norm_train:tools/train.py -c test_tipc/configs/ch_PP-OCRv2_rec/ch_PP-OCRv2_rec_distillation.yml -o
|
||||
pact_train:null
|
||||
fpgm_train:null
|
||||
distill_train:null
|
||||
null:null
|
||||
null:null
|
||||
##
|
||||
===========================eval_params===========================
|
||||
eval:null
|
||||
null:null
|
||||
##
|
||||
===========================infer_params===========================
|
||||
Global.save_inference_dir:./output/
|
||||
Global.checkpoints:
|
||||
norm_export:tools/export_model.py -c test_tipc/configs/ch_PP-OCRv2_rec/ch_PP-OCRv2_rec_distillation.yml -o
|
||||
quant_export:
|
||||
fpgm_export:
|
||||
distill_export:null
|
||||
export1:null
|
||||
export2:null
|
||||
inference_dir:Student
|
||||
infer_model:./inference/ch_PP-OCRv2_rec_infer
|
||||
infer_export:null
|
||||
infer_quant:False
|
||||
inference:tools/infer/predict_rec.py
|
||||
--use_gpu:False
|
||||
--enable_mkldnn:False
|
||||
--cpu_threads:6
|
||||
--rec_batch_num:1|6
|
||||
--use_tensorrt:False
|
||||
--precision:fp32
|
||||
--rec_model_dir:
|
||||
--image_dir:./inference/rec_inference
|
||||
null:null
|
||||
--benchmark:True
|
||||
null:null
|
||||
===========================infer_benchmark_params==========================
|
||||
random_infer_input:[{float32,[3,32,320]}]
|
|
@ -1,10 +1,10 @@
|
|||
===========================train_params===========================
|
||||
model_name:PPOCRv2_ocr_rec
|
||||
model_name:ch_PP-OCRv2_rec
|
||||
python:python3.7
|
||||
gpu_list:0|0,1
|
||||
Global.use_gpu:True|True
|
||||
Global.auto_cast:amp
|
||||
Global.epoch_num:lite_train_lite_infer=3|whole_train_whole_infer=300
|
||||
Global.epoch_num:lite_train_lite_infer=3|whole_train_whole_infer=50
|
||||
Global.save_model_dir:./output/
|
||||
Train.loader.batch_size_per_card:lite_train_lite_infer=16|whole_train_whole_infer=128
|
||||
Global.pretrained_model:null
|
||||
|
@ -39,11 +39,11 @@ infer_export:null
|
|||
infer_quant:False
|
||||
inference:tools/infer/predict_rec.py
|
||||
--use_gpu:True|False
|
||||
--enable_mkldnn:True|False
|
||||
--cpu_threads:1|6
|
||||
--enable_mkldnn:False
|
||||
--cpu_threads:6
|
||||
--rec_batch_num:1|6
|
||||
--use_tensorrt:False|True
|
||||
--precision:fp32|int8
|
||||
--use_tensorrt:False
|
||||
--precision:fp32
|
||||
--rec_model_dir:
|
||||
--image_dir:./inference/rec_inference
|
||||
null:null
|
||||
|
|
|
@ -0,0 +1,20 @@
|
|||
===========================cpp_infer_params===========================
|
||||
model_name:ch_PP-OCRv2_rec_KL
|
||||
use_opencv:True
|
||||
infer_model:./inference/ch_PP-OCRv2_rec_klquant_infer
|
||||
infer_quant:False
|
||||
inference:./deploy/cpp_infer/build/ppocr --rec_char_dict_path=./ppocr/utils/ppocr_keys_v1.txt --rec_img_h=32
|
||||
--use_gpu:True|False
|
||||
--enable_mkldnn:False
|
||||
--cpu_threads:6
|
||||
--rec_batch_num:6
|
||||
--use_tensorrt:False
|
||||
--precision:fp32
|
||||
--rec_model_dir:
|
||||
--image_dir:./inference/rec_inference/
|
||||
null:null
|
||||
--benchmark:True
|
||||
--det:False
|
||||
--rec:True
|
||||
--cls:False
|
||||
--use_angle_cls:False
|
|
@ -1,17 +1,17 @@
|
|||
===========================kl_quant_params===========================
|
||||
model_name:PPOCRv2_ocr_rec_kl
|
||||
model_name:ch_PP-OCRv2_rec_KL
|
||||
python:python3.7
|
||||
Global.pretrained_model:null
|
||||
Global.save_inference_dir:null
|
||||
infer_model:./inference/ch_PP-OCRv2_rec_infer/
|
||||
infer_export:deploy/slim/quantization/quant_kl.py -c test_tipc/configs/ch_PP-OCRv2_rec/ch_PP-OCRv2_rec_distillation.yml -o
|
||||
infer_quant:True
|
||||
inference:tools/infer/predict_rec.py
|
||||
inference:tools/infer/predict_rec.py --rec_image_shape="3,32,320"
|
||||
--use_gpu:False|True
|
||||
--enable_mkldnn:False|True
|
||||
--cpu_threads:1|6
|
||||
--enable_mkldnn:False
|
||||
--cpu_threads:6
|
||||
--rec_batch_num:1|6
|
||||
--use_tensorrt:True
|
||||
--use_tensorrt:False
|
||||
--precision:int8
|
||||
--rec_model_dir:
|
||||
--image_dir:./inference/rec_inference
|
||||
|
|
|
@ -0,0 +1,19 @@
|
|||
===========================serving_params===========================
|
||||
model_name:ch_PP-OCRv2_rec_KL
|
||||
python:python3.7
|
||||
trans_model:-m paddle_serving_client.convert
|
||||
--det_dirname:./inference/ch_PP-OCRv2_det_klquant_infer/
|
||||
--model_filename:inference.pdmodel
|
||||
--params_filename:inference.pdiparams
|
||||
--det_serving_server:./deploy/pdserving/ppocr_det_v2_kl_serving/
|
||||
--det_serving_client:./deploy/pdserving/ppocr_det_v2_kl_client/
|
||||
--rec_dirname:./inference/ch_PP-OCRv2_rec_klquant_infer/
|
||||
--rec_serving_server:./deploy/pdserving/ppocr_rec_v2_kl_serving/
|
||||
--rec_serving_client:./deploy/pdserving/ppocr_rec_v2_kl_client/
|
||||
serving_dir:./deploy/pdserving
|
||||
web_service:-m paddle_serving_server.serve
|
||||
--op:GeneralDetectionOp GeneralInferOp
|
||||
--port:8181
|
||||
--gpu_id:"0"|null
|
||||
cpp_client:ocr_cpp_client.py
|
||||
--image_dir:../../doc/imgs/1.jpg
|
|
@ -0,0 +1,23 @@
|
|||
===========================serving_params===========================
|
||||
model_name:ch_PP-OCRv2_rec_KL
|
||||
python:python3.7
|
||||
trans_model:-m paddle_serving_client.convert
|
||||
--det_dirname:null
|
||||
--model_filename:inference.pdmodel
|
||||
--params_filename:inference.pdiparams
|
||||
--det_serving_server:null
|
||||
--det_serving_client:null
|
||||
--rec_dirname:./inference/ch_PP-OCRv2_rec_klquant_infer/
|
||||
--rec_serving_server:./deploy/pdserving/ppocr_rec_v2_kl_serving/
|
||||
--rec_serving_client:./deploy/pdserving/ppocr_rec_v2_kl_client/
|
||||
serving_dir:./deploy/pdserving
|
||||
web_service:web_service_rec.py --config=config.yml --opt op.rec.concurrency="1"
|
||||
op.det.local_service_conf.devices:gpu|null
|
||||
op.det.local_service_conf.use_mkldnn:False
|
||||
op.det.local_service_conf.thread_num:6
|
||||
op.det.local_service_conf.use_trt:False
|
||||
op.det.local_service_conf.precision:fp32
|
||||
op.det.local_service_conf.model_config:
|
||||
op.rec.local_service_conf.model_config:
|
||||
pipline:pipeline_http_client.py --det=False
|
||||
--image_dir:../../inference/rec_inference
|
|
@ -0,0 +1,20 @@
|
|||
===========================cpp_infer_params===========================
|
||||
model_name:ch_PP-OCRv2_rec_PACT
|
||||
use_opencv:True
|
||||
infer_model:./inference/ch_PP-OCRv2_rec_pact_infer
|
||||
infer_quant:False
|
||||
inference:./deploy/cpp_infer/build/ppocr --rec_char_dict_path=./ppocr/utils/ppocr_keys_v1.txt --rec_img_h=32
|
||||
--use_gpu:True|False
|
||||
--enable_mkldnn:False
|
||||
--cpu_threads:6
|
||||
--rec_batch_num:6
|
||||
--use_tensorrt:False
|
||||
--precision:fp32
|
||||
--rec_model_dir:
|
||||
--image_dir:./inference/rec_inference/
|
||||
null:null
|
||||
--benchmark:True
|
||||
--det:False
|
||||
--rec:True
|
||||
--cls:False
|
||||
--use_angle_cls:False
|
|
@ -0,0 +1,19 @@
|
|||
===========================serving_params===========================
|
||||
model_name:ch_PP-OCRv2_rec_PACT
|
||||
python:python3.7
|
||||
trans_model:-m paddle_serving_client.convert
|
||||
--det_dirname:./inference/ch_PP-OCRv2_det_pact_infer/
|
||||
--model_filename:inference.pdmodel
|
||||
--params_filename:inference.pdiparams
|
||||
--det_serving_server:./deploy/pdserving/ppocr_det_v2_pact_serving/
|
||||
--det_serving_client:./deploy/pdserving/ppocr_det_v2_pact_client/
|
||||
--rec_dirname:./inference/ch_PP-OCRv2_rec_pact_infer/
|
||||
--rec_serving_server:./deploy/pdserving/ppocr_rec_v2_pact_serving/
|
||||
--rec_serving_client:./deploy/pdserving/ppocr_rec_v2_pact_client/
|
||||
serving_dir:./deploy/pdserving
|
||||
web_service:-m paddle_serving_server.serve
|
||||
--op:GeneralDetectionOp GeneralInferOp
|
||||
--port:8181
|
||||
--gpu_id:"0"|null
|
||||
cpp_client:ocr_cpp_client.py
|
||||
--image_dir:../../doc/imgs/1.jpg
|
|
@ -0,0 +1,23 @@
|
|||
===========================serving_params===========================
|
||||
model_name:ch_PP-OCRv2_rec_PACT
|
||||
python:python3.7
|
||||
trans_model:-m paddle_serving_client.convert
|
||||
--det_dirname:null
|
||||
--model_filename:inference.pdmodel
|
||||
--params_filename:inference.pdiparams
|
||||
--det_serving_server:null
|
||||
--det_serving_client:null
|
||||
--rec_dirname:./inference/ch_PP-OCRv2_rec_pact_infer/
|
||||
--rec_serving_server:./deploy/pdserving/ppocr_rec_v2_pact_serving/
|
||||
--rec_serving_client:./deploy/pdserving/ppocr_rec_v2_pact_client/
|
||||
serving_dir:./deploy/pdserving
|
||||
web_service:web_service_rec.py --config=config.yml --opt op.rec.concurrency="1"
|
||||
op.det.local_service_conf.devices:gpu|null
|
||||
op.det.local_service_conf.use_mkldnn:False
|
||||
op.det.local_service_conf.thread_num:6
|
||||
op.det.local_service_conf.use_trt:False
|
||||
op.det.local_service_conf.precision:fp32
|
||||
op.det.local_service_conf.model_config:
|
||||
op.rec.local_service_conf.model_config:
|
||||
pipline:pipeline_http_client.py --det=False
|
||||
--image_dir:../../inference/rec_inference
|
|
@ -1,10 +1,10 @@
|
|||
===========================train_params===========================
|
||||
model_name:ch_PPOCRv2_rec_PACT
|
||||
model_name:ch_PP-OCRv2_rec_PACT
|
||||
python:python3.7
|
||||
gpu_list:0|0,1
|
||||
Global.use_gpu:True|True
|
||||
Global.auto_cast:fp32
|
||||
Global.epoch_num:lite_train_lite_infer=6|whole_train_whole_infer=300
|
||||
Global.epoch_num:lite_train_lite_infer=1|whole_train_whole_infer=50
|
||||
Global.save_model_dir:./output/
|
||||
Train.loader.batch_size_per_card:lite_train_lite_infer=16|whole_train_whole_infer=128
|
||||
Global.pretrained_model:pretrain_models/ch_PP-OCRv2_rec_train/best_accuracy
|
||||
|
@ -39,11 +39,11 @@ infer_export:null
|
|||
infer_quant:True
|
||||
inference:tools/infer/predict_rec.py
|
||||
--use_gpu:True|False
|
||||
--enable_mkldnn:True|False
|
||||
--cpu_threads:1|6
|
||||
--enable_mkldnn:False
|
||||
--cpu_threads:6
|
||||
--rec_batch_num:1|6
|
||||
--use_tensorrt:False|True
|
||||
--precision:fp32|int8
|
||||
--use_tensorrt:False
|
||||
--precision:fp32
|
||||
--rec_model_dir:
|
||||
--image_dir:./inference/rec_inference
|
||||
null:null
|
||||
|
|
|
@ -1,13 +1,13 @@
|
|||
===========================train_params===========================
|
||||
model_name:ch_PPOCRv2_rec_PACT
|
||||
model_name:ch_PP-OCRv2_rec_PACT
|
||||
python:python3.7
|
||||
gpu_list:0|0,1
|
||||
Global.use_gpu:True|True
|
||||
Global.auto_cast:amp
|
||||
Global.epoch_num:lite_train_lite_infer=3|whole_train_whole_infer=300
|
||||
Global.epoch_num:lite_train_lite_infer=1|whole_train_whole_infer=50
|
||||
Global.save_model_dir:./output/
|
||||
Train.loader.batch_size_per_card:lite_train_lite_infer=16|whole_train_whole_infer=128
|
||||
Global.pretrained_model:null
|
||||
Global.pretrained_model:pretrain_models/ch_PP-OCRv2_rec_train/best_accuracy
|
||||
train_model_name:latest
|
||||
train_infer_img_dir:./inference/rec_inference
|
||||
null:null
|
||||
|
@ -39,11 +39,11 @@ infer_export:null
|
|||
infer_quant:True
|
||||
inference:tools/infer/predict_rec.py
|
||||
--use_gpu:True|False
|
||||
--enable_mkldnn:True|False
|
||||
--cpu_threads:1|6
|
||||
--enable_mkldnn:False
|
||||
--cpu_threads:6
|
||||
--rec_batch_num:1|6
|
||||
--use_tensorrt:False|True
|
||||
--precision:fp32|int8
|
||||
--use_tensorrt:False
|
||||
--precision:fp32
|
||||
--rec_model_dir:
|
||||
--image_dir:./inference/rec_inference
|
||||
null:null
|
||||
|
|
|
@ -1,15 +1,15 @@
|
|||
===========================cpp_infer_params===========================
|
||||
model_name:ocr_system_v3
|
||||
model_name:ch_PP-OCRv3
|
||||
use_opencv:True
|
||||
infer_model:./inference/ch_PP-OCRv3_det_infer/
|
||||
infer_quant:False
|
||||
inference:./deploy/cpp_infer/build/ppocr --rec_img_h=48 --rec_char_dict_path=./ppocr/utils/ppocr_keys_v1.txt
|
||||
--use_gpu:True|False
|
||||
--enable_mkldnn:True|False
|
||||
--cpu_threads:1|6
|
||||
--enable_mkldnn:False
|
||||
--cpu_threads:6
|
||||
--rec_batch_num:1
|
||||
--use_tensorrt:False|True
|
||||
--precision:fp32|fp16
|
||||
--use_tensorrt:False
|
||||
--precision:fp32
|
||||
--det_model_dir:
|
||||
--image_dir:./inference/ch_det_data_50/all-sum-510/
|
||||
--rec_model_dir:./inference/ch_PP-OCRv3_rec_infer/
|
||||
|
|
|
@ -6,10 +6,10 @@ infer_export:null
|
|||
infer_quant:False
|
||||
inference:tools/infer/predict_system.py --rec_image_shape="3,48,320"
|
||||
--use_gpu:False|True
|
||||
--enable_mkldnn:False|True
|
||||
--cpu_threads:1|6
|
||||
--enable_mkldnn:False
|
||||
--cpu_threads:6
|
||||
--rec_batch_num:1
|
||||
--use_tensorrt:False|True
|
||||
--use_tensorrt:False
|
||||
--precision:fp32
|
||||
--det_model_dir:
|
||||
--image_dir:./inference/ch_det_data_50/all-sum-510/
|
||||
|
|
|
@ -0,0 +1,17 @@
|
|||
===========================paddle2onnx_params===========================
|
||||
model_name:ch_PP-OCRv3
|
||||
python:python3.7
|
||||
2onnx: paddle2onnx
|
||||
--det_model_dir:./inference/ch_PP-OCRv3_det_infer/
|
||||
--model_filename:inference.pdmodel
|
||||
--params_filename:inference.pdiparams
|
||||
--det_save_file:./inference/det_v3_onnx/model.onnx
|
||||
--rec_model_dir:./inference/ch_PP-OCRv3_rec_infer/
|
||||
--rec_save_file:./inference/rec_v3_onnx/model.onnx
|
||||
--opset_version:10
|
||||
--enable_onnx_checker:True
|
||||
inference:tools/infer/predict_system.py --rec_image_shape="3,48,320"
|
||||
--use_gpu:True|False
|
||||
--det_model_dir:
|
||||
--rec_model_dir:
|
||||
--image_dir:./inference/ch_det_data_50/all-sum-510/00008790.jpg
|
|
@ -0,0 +1,19 @@
|
|||
===========================serving_params===========================
|
||||
model_name:ch_PP-OCRv3
|
||||
python:python3.7
|
||||
trans_model:-m paddle_serving_client.convert
|
||||
--det_dirname:./inference/ch_PP-OCRv3_det_infer/
|
||||
--model_filename:inference.pdmodel
|
||||
--params_filename:inference.pdiparams
|
||||
--det_serving_server:./deploy/pdserving/ppocr_det_v3_serving/
|
||||
--det_serving_client:./deploy/pdserving/ppocr_det_v3_client/
|
||||
--rec_dirname:./inference/ch_PP-OCRv3_rec_infer/
|
||||
--rec_serving_server:./deploy/pdserving/ppocr_rec_v3_serving/
|
||||
--rec_serving_client:./deploy/pdserving/ppocr_rec_v3_client/
|
||||
serving_dir:./deploy/pdserving
|
||||
web_service:-m paddle_serving_server.serve
|
||||
--op:GeneralDetectionOp GeneralInferOp
|
||||
--port:8181
|
||||
--gpu_id:"0"|null
|
||||
cpp_client:ocr_cpp_client.py
|
||||
--image_dir:../../doc/imgs/1.jpg
|
|
@ -0,0 +1,23 @@
|
|||
===========================serving_params===========================
|
||||
model_name:ch_PP-OCRv3
|
||||
python:python3.7
|
||||
trans_model:-m paddle_serving_client.convert
|
||||
--det_dirname:./inference/ch_PP-OCRv3_det_infer/
|
||||
--model_filename:inference.pdmodel
|
||||
--params_filename:inference.pdiparams
|
||||
--det_serving_server:./deploy/pdserving/ppocr_det_v3_serving/
|
||||
--det_serving_client:./deploy/pdserving/ppocr_det_v3_client/
|
||||
--rec_dirname:./inference/ch_PP-OCRv3_rec_infer/
|
||||
--rec_serving_server:./deploy/pdserving/ppocr_rec_v3_serving/
|
||||
--rec_serving_client:./deploy/pdserving/ppocr_rec_v3_client/
|
||||
serving_dir:./deploy/pdserving
|
||||
web_service:web_service.py --config=config.yml --opt op.det.concurrency="1" op.rec.concurrency="1"
|
||||
op.det.local_service_conf.devices:gpu|null
|
||||
op.det.local_service_conf.use_mkldnn:False
|
||||
op.det.local_service_conf.thread_num:6
|
||||
op.det.local_service_conf.use_trt:False
|
||||
op.det.local_service_conf.precision:fp32
|
||||
op.det.local_service_conf.model_config:
|
||||
op.rec.local_service_conf.model_config:
|
||||
pipline:pipeline_http_client.py
|
||||
--image_dir:../../doc/imgs/1.jpg
|
|
@ -1,15 +1,15 @@
|
|||
===========================cpp_infer_params===========================
|
||||
model_name:ocr_det_v3
|
||||
model_name:ch_PP-OCRv3_det
|
||||
use_opencv:True
|
||||
infer_model:./inference/ch_PP-OCRv3_det_infer/
|
||||
infer_quant:False
|
||||
inference:./deploy/cpp_infer/build/ppocr
|
||||
--use_gpu:True|False
|
||||
--enable_mkldnn:True|False
|
||||
--cpu_threads:1|6
|
||||
--enable_mkldnn:False
|
||||
--cpu_threads:6
|
||||
--rec_batch_num:1
|
||||
--use_tensorrt:False|True
|
||||
--precision:fp32|fp16
|
||||
--use_tensorrt:False
|
||||
--precision:fp32
|
||||
--det_model_dir:
|
||||
--image_dir:./inference/ch_det_data_50/all-sum-510/
|
||||
null:null
|
||||
|
|
|
@ -1,14 +1,17 @@
|
|||
===========================paddle2onnx_params===========================
|
||||
model_name:ocr_det_v3
|
||||
model_name:ch_PP-OCRv3_det
|
||||
python:python3.7
|
||||
2onnx: paddle2onnx
|
||||
--model_dir:./inference/ch_PP-OCRv3_det_infer/
|
||||
--det_model_dir:./inference/ch_PP-OCRv3_det_infer/
|
||||
--model_filename:inference.pdmodel
|
||||
--params_filename:inference.pdiparams
|
||||
--save_file:./inference/det_v3_onnx/model.onnx
|
||||
--det_save_file:./inference/det_v3_onnx/model.onnx
|
||||
--rec_model_dir:
|
||||
--rec_save_file:
|
||||
--opset_version:10
|
||||
--enable_onnx_checker:True
|
||||
inference:tools/infer/predict_det.py
|
||||
--use_gpu:True|False
|
||||
--det_model_dir:
|
||||
--rec_model_dir:
|
||||
--image_dir:./inference/ch_det_data_50/all-sum-510/
|
|
@ -1,18 +1,23 @@
|
|||
===========================serving_params===========================
|
||||
model_name:ocr_det_v3
|
||||
model_name:ch_PP-OCRv3_det
|
||||
python:python3.7
|
||||
trans_model:-m paddle_serving_client.convert
|
||||
--dirname:./inference/ch_PP-OCRv3_det_infer/
|
||||
--det_dirname:./inference/ch_PP-OCRv3_det_infer/
|
||||
--model_filename:inference.pdmodel
|
||||
--params_filename:inference.pdiparams
|
||||
--serving_server:./deploy/pdserving/ppocr_det_v3_serving/
|
||||
--serving_client:./deploy/pdserving/ppocr_det_v3_client/
|
||||
--det_serving_server:./deploy/pdserving/ppocr_det_v3_serving/
|
||||
--det_serving_client:./deploy/pdserving/ppocr_det_v3_client/
|
||||
--rec_dirname:null
|
||||
--rec_serving_server:null
|
||||
--rec_serving_client:null
|
||||
serving_dir:./deploy/pdserving
|
||||
web_service:web_service_det.py --config=config.yml --opt op.det.concurrency="1"
|
||||
op.det.local_service_conf.devices:gpu|null
|
||||
op.det.local_service_conf.use_mkldnn:True|False
|
||||
op.det.local_service_conf.thread_num:1|6
|
||||
op.det.local_service_conf.use_trt:False|True
|
||||
op.det.local_service_conf.precision:fp32|fp16|int8
|
||||
pipline:pipeline_rpc_client.py|pipeline_http_client.py
|
||||
--image_dir:../../doc/imgs
|
||||
op.det.local_service_conf.use_mkldnn:False
|
||||
op.det.local_service_conf.thread_num:6
|
||||
op.det.local_service_conf.use_trt:False
|
||||
op.det.local_service_conf.precision:fp32
|
||||
op.det.local_service_conf.model_config:
|
||||
op.rec.local_service_conf.model_config:
|
||||
pipline:pipeline_http_client.py
|
||||
--image_dir:../../doc/imgs/1.jpg
|
||||
|
|
|
@ -1,10 +1,10 @@
|
|||
===========================train_params===========================
|
||||
model_name:ch_PPOCRv3_det
|
||||
model_name:ch_PP-OCRv3_det
|
||||
python:python3.7
|
||||
gpu_list:0|0,1
|
||||
Global.use_gpu:True|True
|
||||
Global.auto_cast:fp32
|
||||
Global.epoch_num:lite_train_lite_infer=1|whole_train_whole_infer=500
|
||||
Global.epoch_num:lite_train_lite_infer=1|whole_train_whole_infer=50
|
||||
Global.save_model_dir:./output/
|
||||
Train.loader.batch_size_per_card:lite_train_lite_infer=2|whole_train_whole_infer=4
|
||||
Global.pretrained_model:null
|
||||
|
@ -39,11 +39,11 @@ infer_export:null
|
|||
infer_quant:False
|
||||
inference:tools/infer/predict_det.py
|
||||
--use_gpu:True|False
|
||||
--enable_mkldnn:True|False
|
||||
--cpu_threads:1|6
|
||||
--enable_mkldnn:False
|
||||
--cpu_threads:6
|
||||
--rec_batch_num:1
|
||||
--use_tensorrt:False|True
|
||||
--precision:fp32|fp16|int8
|
||||
--use_tensorrt:False
|
||||
--precision:fp32
|
||||
--det_model_dir:
|
||||
--image_dir:./inference/ch_det_data_50/all-sum-510/
|
||||
null:null
|
||||
|
|
|
@ -0,0 +1,53 @@
|
|||
===========================train_params===========================
|
||||
model_name:ch_PP-OCRv3_det
|
||||
python:python3.7
|
||||
gpu_list:192.168.0.1,192.168.0.2;0,1
|
||||
Global.use_gpu:True
|
||||
Global.auto_cast:fp32
|
||||
Global.epoch_num:lite_train_lite_infer=1|whole_train_whole_infer=50
|
||||
Global.save_model_dir:./output/
|
||||
Train.loader.batch_size_per_card:lite_train_lite_infer=2|whole_train_whole_infer=4
|
||||
Global.pretrained_model:null
|
||||
train_model_name:latest
|
||||
train_infer_img_dir:./train_data/icdar2015/text_localization/ch4_test_images/
|
||||
null:null
|
||||
##
|
||||
trainer:norm_train
|
||||
norm_train:tools/train.py -c configs/det/ch_PP-OCRv3/ch_PP-OCRv3_det_cml.yml -o
|
||||
pact_train:null
|
||||
fpgm_train:null
|
||||
distill_train:null
|
||||
null:null
|
||||
null:null
|
||||
##
|
||||
===========================eval_params===========================
|
||||
eval:null
|
||||
null:null
|
||||
##
|
||||
===========================infer_params===========================
|
||||
Global.save_inference_dir:./output/
|
||||
Global.checkpoints:
|
||||
norm_export:tools/export_model.py -c configs/det/ch_PP-OCRv3/ch_PP-OCRv3_det_cml.yml -o
|
||||
quant_export:null
|
||||
fpgm_export:
|
||||
distill_export:null
|
||||
export1:null
|
||||
export2:null
|
||||
inference_dir:Student
|
||||
infer_model:./inference/ch_PP-OCRv3_det_infer/
|
||||
infer_export:null
|
||||
infer_quant:False
|
||||
inference:tools/infer/predict_det.py
|
||||
--use_gpu:False
|
||||
--enable_mkldnn:False
|
||||
--cpu_threads:6
|
||||
--rec_batch_num:1
|
||||
--use_tensorrt:False
|
||||
--precision:fp32
|
||||
--det_model_dir:
|
||||
--image_dir:./inference/ch_det_data_50/all-sum-510/
|
||||
null:null
|
||||
--benchmark:True
|
||||
null:null
|
||||
===========================infer_benchmark_params==========================
|
||||
random_infer_input:[{float32,[3,640,640]}];[{float32,[3,960,960]}]
|
|
@ -1,10 +1,10 @@
|
|||
===========================train_params===========================
|
||||
model_name:ch_PPOCRv3_det
|
||||
model_name:ch_PP-OCRv3_det
|
||||
python:python3.7
|
||||
gpu_list:0|0,1
|
||||
Global.use_gpu:True|True
|
||||
Global.auto_cast:amp
|
||||
Global.epoch_num:lite_train_lite_infer=1|whole_train_whole_infer=500
|
||||
Global.epoch_num:lite_train_lite_infer=1|whole_train_whole_infer=50
|
||||
Global.save_model_dir:./output/
|
||||
Train.loader.batch_size_per_card:lite_train_lite_infer=2|whole_train_whole_infer=4
|
||||
Global.pretrained_model:null
|
||||
|
@ -39,11 +39,11 @@ infer_export:null
|
|||
infer_quant:False
|
||||
inference:tools/infer/predict_det.py
|
||||
--use_gpu:True|False
|
||||
--enable_mkldnn:True|False
|
||||
--cpu_threads:1|6
|
||||
--enable_mkldnn:False
|
||||
--cpu_threads:6
|
||||
--rec_batch_num:1
|
||||
--use_tensorrt:False|True
|
||||
--precision:fp32|fp16|int8
|
||||
--use_tensorrt:False
|
||||
--precision:fp32
|
||||
--det_model_dir:
|
||||
--image_dir:./inference/ch_det_data_50/all-sum-510/
|
||||
null:null
|
||||
|
|
|
@ -0,0 +1,20 @@
|
|||
===========================cpp_infer_params===========================
|
||||
model_name:ch_PP-OCRv3_det_KL
|
||||
use_opencv:True
|
||||
infer_model:./inference/ch_PP-OCRv3_det_klquant_infer
|
||||
infer_quant:False
|
||||
inference:./deploy/cpp_infer/build/ppocr
|
||||
--use_gpu:True|False
|
||||
--enable_mkldnn:False
|
||||
--cpu_threads:6
|
||||
--rec_batch_num:1
|
||||
--use_tensorrt:False
|
||||
--precision:fp32
|
||||
--det_model_dir:
|
||||
--image_dir:./inference/ch_det_data_50/all-sum-510/
|
||||
null:null
|
||||
--benchmark:True
|
||||
--det:True
|
||||
--rec:False
|
||||
--cls:False
|
||||
--use_angle_cls:False
|
|
@ -1,5 +1,5 @@
|
|||
===========================kl_quant_params===========================
|
||||
model_name:PPOCRv3_ocr_det_kl
|
||||
model_name:ch_PP-OCRv3_det_KL
|
||||
python:python3.7
|
||||
Global.pretrained_model:null
|
||||
Global.save_inference_dir:null
|
||||
|
@ -8,10 +8,10 @@ infer_export:deploy/slim/quantization/quant_kl.py -c configs/det/ch_PP-OCRv3/ch_
|
|||
infer_quant:True
|
||||
inference:tools/infer/predict_det.py
|
||||
--use_gpu:False|True
|
||||
--enable_mkldnn:True
|
||||
--cpu_threads:1|6
|
||||
--enable_mkldnn:False
|
||||
--cpu_threads:6
|
||||
--rec_batch_num:1
|
||||
--use_tensorrt:False|True
|
||||
--use_tensorrt:False
|
||||
--precision:int8
|
||||
--det_model_dir:
|
||||
--image_dir:./inference/ch_det_data_50/all-sum-510/
|
||||
|
|
|
@ -0,0 +1,19 @@
|
|||
===========================serving_params===========================
|
||||
model_name:ch_PP-OCRv3_det_KL
|
||||
python:python3.7
|
||||
trans_model:-m paddle_serving_client.convert
|
||||
--det_dirname:./inference/ch_PP-OCRv3_det_klquant_infer/
|
||||
--model_filename:inference.pdmodel
|
||||
--params_filename:inference.pdiparams
|
||||
--det_serving_server:./deploy/pdserving/ppocr_det_v3_kl_serving/
|
||||
--det_serving_client:./deploy/pdserving/ppocr_det_v3_kl_client/
|
||||
--rec_dirname:./inference/ch_PP-OCRv3_rec_klquant_infer/
|
||||
--rec_serving_server:./deploy/pdserving/ppocr_rec_v3_kl_serving/
|
||||
--rec_serving_client:./deploy/pdserving/ppocr_rec_v3_kl_client/
|
||||
serving_dir:./deploy/pdserving
|
||||
web_service:-m paddle_serving_server.serve
|
||||
--op:GeneralDetectionOp GeneralInferOp
|
||||
--port:8181
|
||||
--gpu_id:"0"|null
|
||||
cpp_client:ocr_cpp_client.py
|
||||
--image_dir:../../doc/imgs/1.jpg
|
Some files were not shown because too many files have changed in this diff Show More
Loading…
Reference in New Issue