Merge branch 'dygraph' of https://github.com/PaddlePaddle/PaddleOCR into dygraph
commit
e627147a47
|
@ -0,0 +1,472 @@
|
|||
# 智能运营:通用中文表格识别
|
||||
|
||||
- [1. 背景介绍](#1-背景介绍)
|
||||
- [2. 中文表格识别](#2-中文表格识别)
|
||||
- [2.1 环境准备](#21-环境准备)
|
||||
- [2.2 准备数据集](#22-准备数据集)
|
||||
- [2.2.1 划分训练测试集](#221-划分训练测试集)
|
||||
- [2.2.2 查看数据集](#222-查看数据集)
|
||||
- [2.3 训练](#23-训练)
|
||||
- [2.4 验证](#24-验证)
|
||||
- [2.5 训练引擎推理](#25-训练引擎推理)
|
||||
- [2.6 模型导出](#26-模型导出)
|
||||
- [2.7 预测引擎推理](#27-预测引擎推理)
|
||||
- [2.8 表格识别](#28-表格识别)
|
||||
- [3. 表格属性识别](#3-表格属性识别)
|
||||
- [3.1 代码、环境、数据准备](#31-代码环境数据准备)
|
||||
- [3.1.1 代码准备](#311-代码准备)
|
||||
- [3.1.2 环境准备](#312-环境准备)
|
||||
- [3.1.3 数据准备](#313-数据准备)
|
||||
- [3.2 表格属性识别训练](#32-表格属性识别训练)
|
||||
- [3.3 表格属性识别推理和部署](#33-表格属性识别推理和部署)
|
||||
- [3.3.1 模型转换](#331-模型转换)
|
||||
- [3.3.2 模型推理](#332-模型推理)
|
||||
|
||||
## 1. 背景介绍
|
||||
|
||||
中文表格识别在金融行业有着广泛的应用,如保险理赔、财报分析和信息录入等领域。当前,金融行业的表格识别主要以手动录入为主,开发一种自动表格识别成为丞待解决的问题。
|
||||
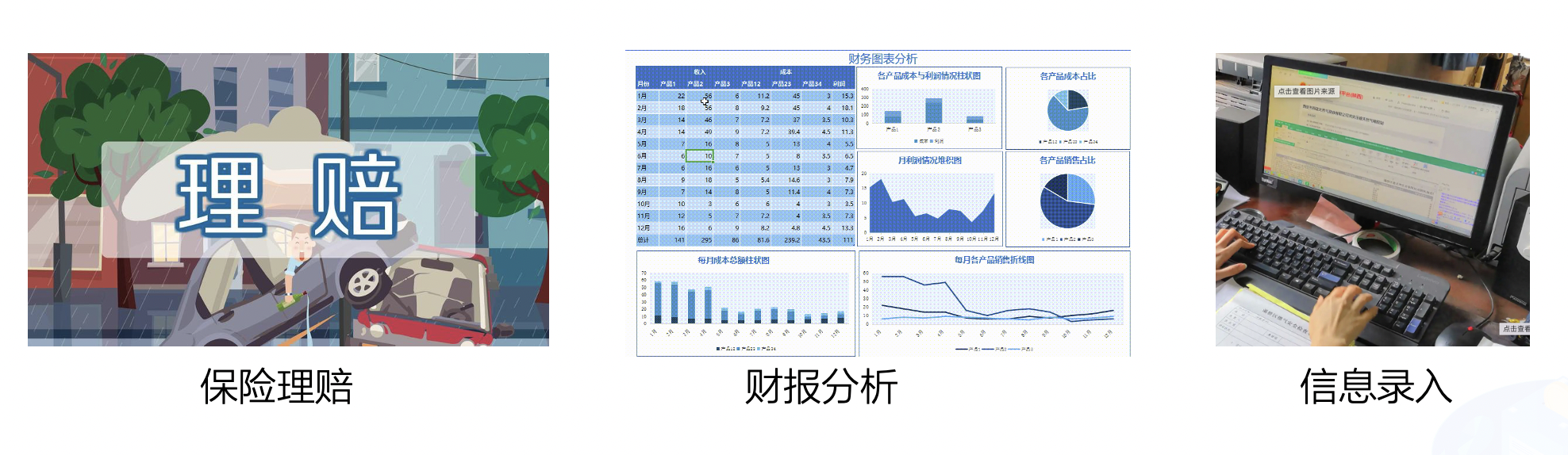
|
||||
|
||||
|
||||
在金融行业中,表格图像主要有清单类的单元格密集型表格,申请表类的大单元格表格,拍照表格和倾斜表格四种主要形式。
|
||||
|
||||
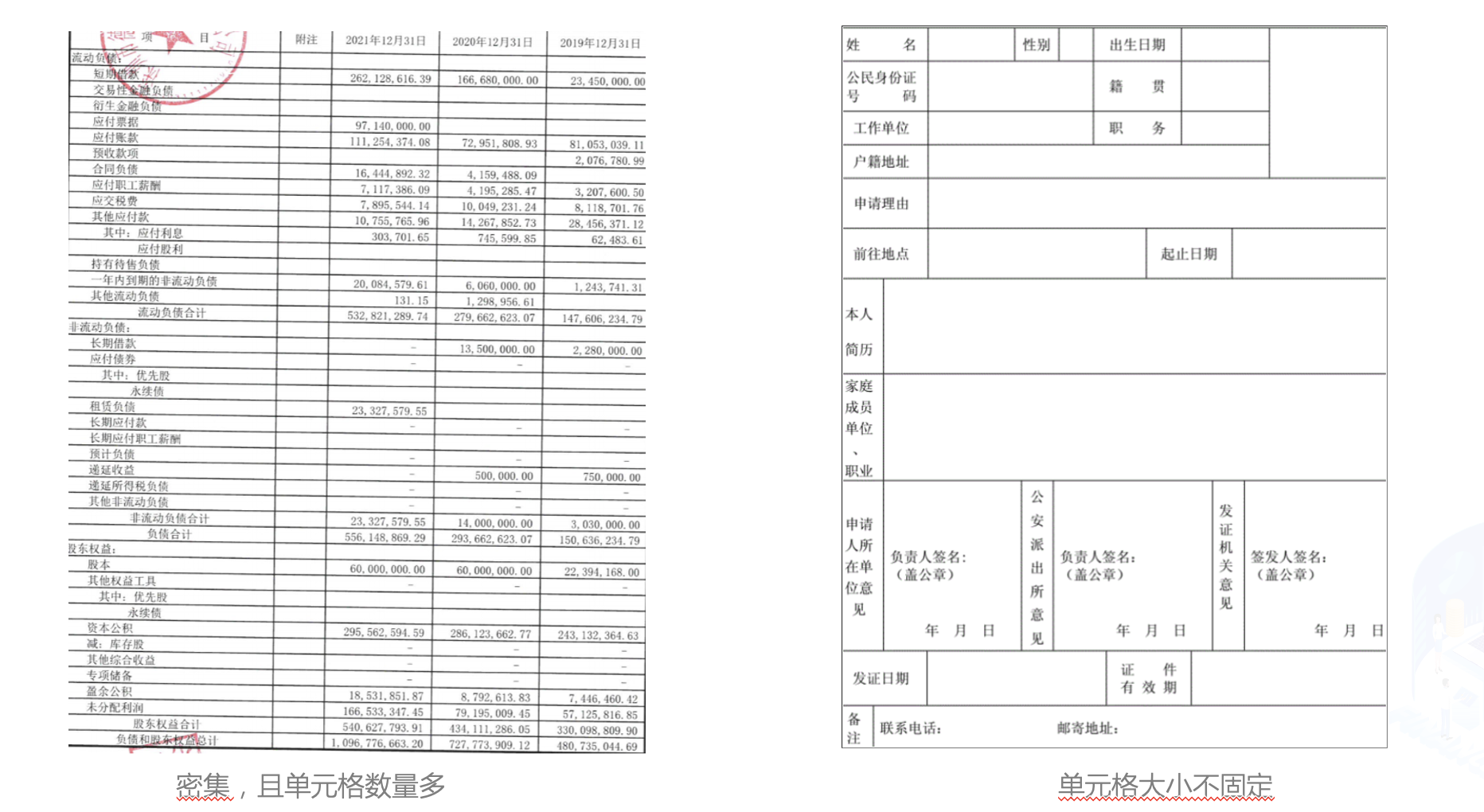
|
||||
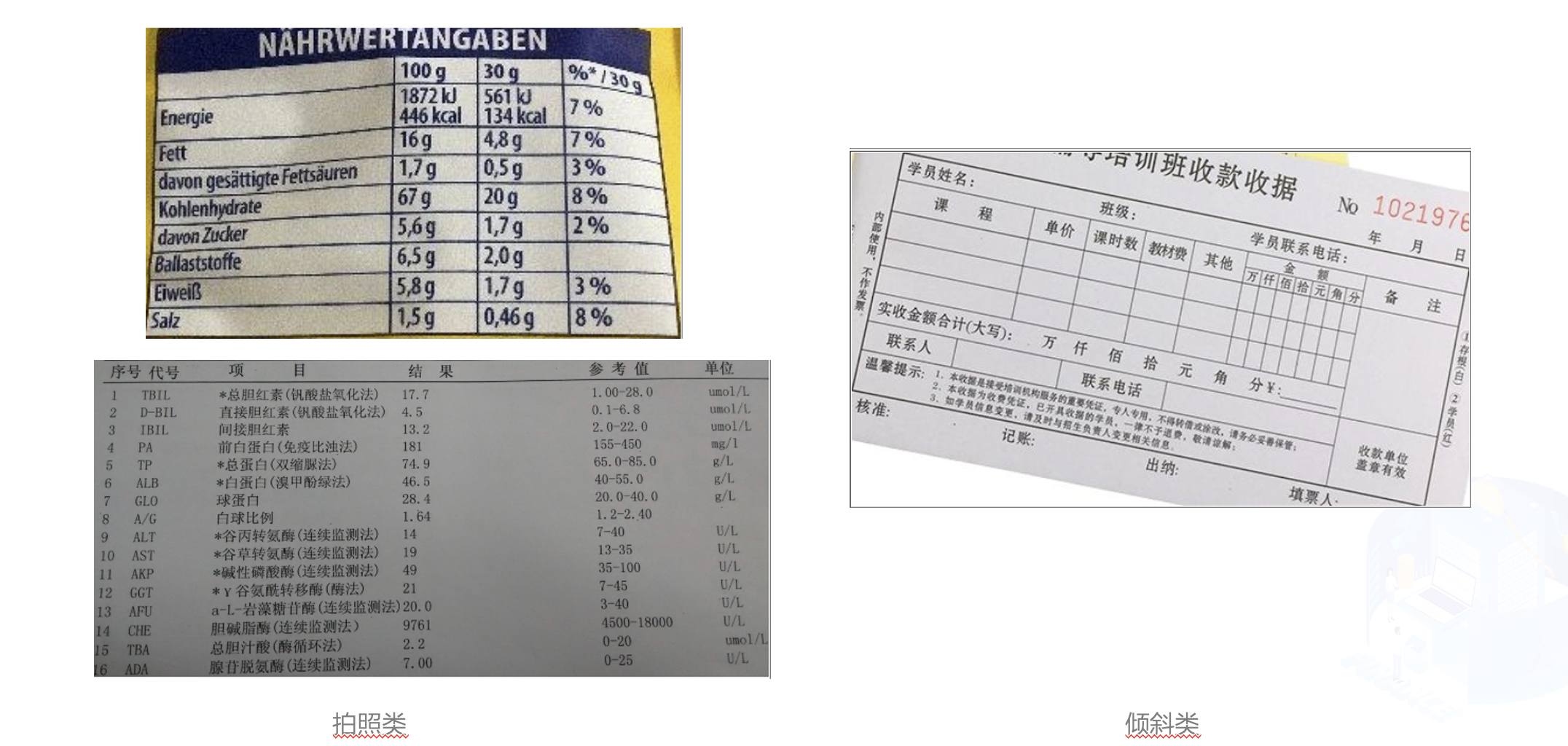
|
||||
|
||||
|
||||
当前的表格识别算法不能很好的处理这些场景下的表格图像。在本例中,我们使用PP-Structurev2最新发布的表格识别模型SLANet来演示如何进行中文表格是识别。同时,为了方便作业流程,我们使用表格属性识别模型对表格图像的属性进行识别,对表格的难易程度进行判断,加快人工进行校对速度。
|
||||
|
||||
本项目AI Studio链接:https://aistudio.baidu.com/aistudio/projectdetail/4588067
|
||||
|
||||
## 2. 中文表格识别
|
||||
### 2.1 环境准备
|
||||
|
||||
|
||||
```python
|
||||
# 下载PaddleOCR代码
|
||||
! git clone -b dygraph https://gitee.com/paddlepaddle/PaddleOCR
|
||||
```
|
||||
|
||||
|
||||
```python
|
||||
# 安装PaddleOCR环境
|
||||
! pip install -r PaddleOCR/requirements.txt --force-reinstall
|
||||
! pip install protobuf==3.19
|
||||
```
|
||||
|
||||
### 2.2 准备数据集
|
||||
|
||||
本例中使用的数据集采用表格[生成工具](https://github.com/WenmuZhou/TableGeneration)制作。
|
||||
|
||||
使用如下命令对数据集进行解压,并查看数据集大小
|
||||
|
||||
|
||||
```python
|
||||
! cd data/data165849 && tar -xf table_gen_dataset.tar && cd -
|
||||
! wc -l data/data165849/table_gen_dataset/gt.txt
|
||||
```
|
||||
|
||||
#### 2.2.1 划分训练测试集
|
||||
|
||||
使用下述命令将数据集划分为训练集和测试集, 这里将90%划分为训练集,10%划分为测试集
|
||||
|
||||
|
||||
```python
|
||||
import random
|
||||
with open('/home/aistudio/data/data165849/table_gen_dataset/gt.txt') as f:
|
||||
lines = f.readlines()
|
||||
random.shuffle(lines)
|
||||
train_len = int(len(lines)*0.9)
|
||||
train_list = lines[:train_len]
|
||||
val_list = lines[train_len:]
|
||||
|
||||
# 保存结果
|
||||
with open('/home/aistudio/train.txt','w',encoding='utf-8') as f:
|
||||
f.writelines(train_list)
|
||||
with open('/home/aistudio/val.txt','w',encoding='utf-8') as f:
|
||||
f.writelines(val_list)
|
||||
```
|
||||
|
||||
划分完成后,数据集信息如下
|
||||
|
||||
|类型|数量|图片地址|标注文件路径|
|
||||
|---|---|---|---|
|
||||
|训练集|18000|/home/aistudio/data/data165849/table_gen_dataset|/home/aistudio/train.txt|
|
||||
|测试集|2000|/home/aistudio/data/data165849/table_gen_dataset|/home/aistudio/val.txt|
|
||||
|
||||
#### 2.2.2 查看数据集
|
||||
|
||||
|
||||
```python
|
||||
import cv2
|
||||
import os, json
|
||||
import numpy as np
|
||||
from matplotlib import pyplot as plt
|
||||
%matplotlib inline
|
||||
|
||||
def parse_line(data_dir, line):
|
||||
data_line = line.strip("\n")
|
||||
info = json.loads(data_line)
|
||||
file_name = info['filename']
|
||||
cells = info['html']['cells'].copy()
|
||||
structure = info['html']['structure']['tokens'].copy()
|
||||
|
||||
img_path = os.path.join(data_dir, file_name)
|
||||
if not os.path.exists(img_path):
|
||||
print(img_path)
|
||||
return None
|
||||
data = {
|
||||
'img_path': img_path,
|
||||
'cells': cells,
|
||||
'structure': structure,
|
||||
'file_name': file_name
|
||||
}
|
||||
return data
|
||||
|
||||
def draw_bbox(img_path, points, color=(255, 0, 0), thickness=2):
|
||||
if isinstance(img_path, str):
|
||||
img_path = cv2.imread(img_path)
|
||||
img_path = img_path.copy()
|
||||
for point in points:
|
||||
cv2.polylines(img_path, [point.astype(int)], True, color, thickness)
|
||||
return img_path
|
||||
|
||||
|
||||
def rebuild_html(data):
|
||||
html_code = data['structure']
|
||||
cells = data['cells']
|
||||
to_insert = [i for i, tag in enumerate(html_code) if tag in ('<td>', '>')]
|
||||
|
||||
for i, cell in zip(to_insert[::-1], cells[::-1]):
|
||||
if cell['tokens']:
|
||||
text = ''.join(cell['tokens'])
|
||||
# skip empty text
|
||||
sp_char_list = ['<b>', '</b>', '\u2028', ' ', '<i>', '</i>']
|
||||
text_remove_style = skip_char(text, sp_char_list)
|
||||
if len(text_remove_style) == 0:
|
||||
continue
|
||||
html_code.insert(i + 1, text)
|
||||
|
||||
html_code = ''.join(html_code)
|
||||
return html_code
|
||||
|
||||
|
||||
def skip_char(text, sp_char_list):
|
||||
"""
|
||||
skip empty cell
|
||||
@param text: text in cell
|
||||
@param sp_char_list: style char and special code
|
||||
@return:
|
||||
"""
|
||||
for sp_char in sp_char_list:
|
||||
text = text.replace(sp_char, '')
|
||||
return text
|
||||
|
||||
save_dir = '/home/aistudio/vis'
|
||||
os.makedirs(save_dir, exist_ok=True)
|
||||
image_dir = '/home/aistudio/data/data165849/'
|
||||
html_str = '<table border="1">'
|
||||
|
||||
# 解析标注信息并还原html表格
|
||||
data = parse_line(image_dir, val_list[0])
|
||||
|
||||
img = cv2.imread(data['img_path'])
|
||||
img_name = ''.join(os.path.basename(data['file_name']).split('.')[:-1])
|
||||
img_save_name = os.path.join(save_dir, img_name)
|
||||
boxes = [np.array(x['bbox']) for x in data['cells']]
|
||||
show_img = draw_bbox(data['img_path'], boxes)
|
||||
cv2.imwrite(img_save_name + '_show.jpg', show_img)
|
||||
|
||||
html = rebuild_html(data)
|
||||
html_str += html
|
||||
html_str += '</table>'
|
||||
|
||||
# 显示标注的html字符串
|
||||
from IPython.core.display import display, HTML
|
||||
display(HTML(html_str))
|
||||
# 显示单元格坐标
|
||||
plt.figure(figsize=(15,15))
|
||||
plt.imshow(show_img)
|
||||
plt.show()
|
||||
```
|
||||
|
||||
### 2.3 训练
|
||||
|
||||
这里选用PP-Structurev2中的表格识别模型[SLANet](https://github.com/PaddlePaddle/PaddleOCR/blob/dygraph/configs/table/SLANet.yml)
|
||||
|
||||
SLANet是PP-Structurev2全新推出的表格识别模型,相比PP-Structurev1中TableRec-RARE,在速度不变的情况下精度提升4.7%。TEDS提升2%
|
||||
|
||||
|
||||
|算法|Acc|[TEDS(Tree-Edit-Distance-based Similarity)](https://github.com/ibm-aur-nlp/PubTabNet/tree/master/src)|Speed|
|
||||
| --- | --- | --- | ---|
|
||||
| EDD<sup>[2]</sup> |x| 88.3% |x|
|
||||
| TableRec-RARE(ours) | 71.73%| 93.88% |779ms|
|
||||
| SLANet(ours) | 76.31%| 95.89%|766ms|
|
||||
|
||||
进行训练之前先使用如下命令下载预训练模型
|
||||
|
||||
|
||||
```python
|
||||
# 进入PaddleOCR工作目录
|
||||
os.chdir('/home/aistudio/PaddleOCR')
|
||||
# 下载英文预训练模型
|
||||
! wget -nc -P ./pretrain_models/ https://paddleocr.bj.bcebos.com/ppstructure/models/slanet/en_ppstructure_mobile_v2.0_SLANet_train.tar --no-check-certificate
|
||||
! cd ./pretrain_models/ && tar xf en_ppstructure_mobile_v2.0_SLANet_train.tar && cd ../
|
||||
```
|
||||
|
||||
使用如下命令即可启动训练,需要修改的配置有
|
||||
|
||||
|字段|修改值|含义|
|
||||
|---|---|---|
|
||||
|Global.pretrained_model|./pretrain_models/en_ppstructure_mobile_v2.0_SLANet_train/best_accuracy.pdparams|指向英文表格预训练模型地址|
|
||||
|Global.eval_batch_step|562|模型多少step评估一次,一般设置为一个epoch总的step数|
|
||||
|Optimizer.lr.name|Const|学习率衰减器 |
|
||||
|Optimizer.lr.learning_rate|0.0005|学习率设为之前的0.05倍 |
|
||||
|Train.dataset.data_dir|/home/aistudio/data/data165849|指向训练集图片存放目录 |
|
||||
|Train.dataset.label_file_list|/home/aistudio/data/data165849/table_gen_dataset/train.txt|指向训练集标注文件 |
|
||||
|Train.loader.batch_size_per_card|32|训练时每张卡的batch_size |
|
||||
|Train.loader.num_workers|1|训练集多进程数据读取的进程数,在aistudio中需要设为1 |
|
||||
|Eval.dataset.data_dir|/home/aistudio/data/data165849|指向测试集图片存放目录 |
|
||||
|Eval.dataset.label_file_list|/home/aistudio/data/data165849/table_gen_dataset/val.txt|指向测试集标注文件 |
|
||||
|Eval.loader.batch_size_per_card|32|测试时每张卡的batch_size |
|
||||
|Eval.loader.num_workers|1|测试集多进程数据读取的进程数,在aistudio中需要设为1 |
|
||||
|
||||
|
||||
已经修改好的配置存储在 `/home/aistudio/SLANet_ch.yml`
|
||||
|
||||
|
||||
```python
|
||||
import os
|
||||
os.chdir('/home/aistudio/PaddleOCR')
|
||||
! python3 tools/train.py -c /home/aistudio/SLANet_ch.yml
|
||||
```
|
||||
|
||||
大约在7个epoch后达到最高精度 97.49%
|
||||
|
||||
### 2.4 验证
|
||||
|
||||
训练完成后,可使用如下命令在测试集上评估最优模型的精度
|
||||
|
||||
|
||||
```python
|
||||
! python3 tools/eval.py -c /home/aistudio/SLANet_ch.yml -o Global.checkpoints=/home/aistudio/PaddleOCR/output/SLANet_ch/best_accuracy.pdparams
|
||||
```
|
||||
|
||||
### 2.5 训练引擎推理
|
||||
使用如下命令可使用训练引擎对单张图片进行推理
|
||||
|
||||
|
||||
```python
|
||||
import os;os.chdir('/home/aistudio/PaddleOCR')
|
||||
! python3 tools/infer_table.py -c /home/aistudio/SLANet_ch.yml -o Global.checkpoints=/home/aistudio/PaddleOCR/output/SLANet_ch/best_accuracy.pdparams Global.infer_img=/home/aistudio/data/data165849/table_gen_dataset/img/no_border_18298_G7XZH93DDCMATGJQ8RW2.jpg
|
||||
```
|
||||
|
||||
|
||||
```python
|
||||
import cv2
|
||||
from matplotlib import pyplot as plt
|
||||
%matplotlib inline
|
||||
|
||||
# 显示原图
|
||||
show_img = cv2.imread('/home/aistudio/data/data165849/table_gen_dataset/img/no_border_18298_G7XZH93DDCMATGJQ8RW2.jpg')
|
||||
plt.figure(figsize=(15,15))
|
||||
plt.imshow(show_img)
|
||||
plt.show()
|
||||
|
||||
# 显示预测的单元格
|
||||
show_img = cv2.imread('/home/aistudio/PaddleOCR/output/infer/no_border_18298_G7XZH93DDCMATGJQ8RW2.jpg')
|
||||
plt.figure(figsize=(15,15))
|
||||
plt.imshow(show_img)
|
||||
plt.show()
|
||||
```
|
||||
|
||||
### 2.6 模型导出
|
||||
|
||||
使用如下命令可将模型导出为inference模型
|
||||
|
||||
|
||||
```python
|
||||
! python3 tools/export_model.py -c /home/aistudio/SLANet_ch.yml -o Global.checkpoints=/home/aistudio/PaddleOCR/output/SLANet_ch/best_accuracy.pdparams Global.save_inference_dir=/home/aistudio/SLANet_ch/infer
|
||||
```
|
||||
|
||||
### 2.7 预测引擎推理
|
||||
使用如下命令可使用预测引擎对单张图片进行推理
|
||||
|
||||
|
||||
|
||||
```python
|
||||
os.chdir('/home/aistudio/PaddleOCR/ppstructure')
|
||||
! python3 table/predict_structure.py \
|
||||
--table_model_dir=/home/aistudio/SLANet_ch/infer \
|
||||
--table_char_dict_path=../ppocr/utils/dict/table_structure_dict.txt \
|
||||
--image_dir=/home/aistudio/data/data165849/table_gen_dataset/img/no_border_18298_G7XZH93DDCMATGJQ8RW2.jpg \
|
||||
--output=../output/inference
|
||||
```
|
||||
|
||||
|
||||
```python
|
||||
# 显示原图
|
||||
show_img = cv2.imread('/home/aistudio/data/data165849/table_gen_dataset/img/no_border_18298_G7XZH93DDCMATGJQ8RW2.jpg')
|
||||
plt.figure(figsize=(15,15))
|
||||
plt.imshow(show_img)
|
||||
plt.show()
|
||||
|
||||
# 显示预测的单元格
|
||||
show_img = cv2.imread('/home/aistudio/PaddleOCR/output/inference/no_border_18298_G7XZH93DDCMATGJQ8RW2.jpg')
|
||||
plt.figure(figsize=(15,15))
|
||||
plt.imshow(show_img)
|
||||
plt.show()
|
||||
```
|
||||
|
||||
### 2.8 表格识别
|
||||
|
||||
在表格结构模型训练完成后,可结合OCR检测识别模型,对表格内容进行识别。
|
||||
|
||||
首先下载PP-OCRv3文字检测识别模型
|
||||
|
||||
|
||||
```python
|
||||
# 下载PP-OCRv3文本检测识别模型并解压
|
||||
! wget -nc -P ./inference/ https://paddleocr.bj.bcebos.com/PP-OCRv3/chinese/ch_PP-OCRv3_det_slim_infer.tar --no-check-certificate
|
||||
! wget -nc -P ./inference/ https://paddleocr.bj.bcebos.com/PP-OCRv3/chinese/ch_PP-OCRv3_rec_slim_infer.tar --no-check-certificate
|
||||
! cd ./inference/ && tar xf ch_PP-OCRv3_det_slim_infer.tar && tar xf ch_PP-OCRv3_rec_slim_infer.tar && cd ../
|
||||
```
|
||||
|
||||
模型下载完成后,使用如下命令进行表格识别
|
||||
|
||||
|
||||
```python
|
||||
import os;os.chdir('/home/aistudio/PaddleOCR/ppstructure')
|
||||
! python3 table/predict_table.py \
|
||||
--det_model_dir=inference/ch_PP-OCRv3_det_slim_infer \
|
||||
--rec_model_dir=inference/ch_PP-OCRv3_rec_slim_infer \
|
||||
--table_model_dir=/home/aistudio/SLANet_ch/infer \
|
||||
--rec_char_dict_path=../ppocr/utils/ppocr_keys_v1.txt \
|
||||
--table_char_dict_path=../ppocr/utils/dict/table_structure_dict.txt \
|
||||
--image_dir=/home/aistudio/data/data165849/table_gen_dataset/img/no_border_18298_G7XZH93DDCMATGJQ8RW2.jpg \
|
||||
--output=../output/table
|
||||
```
|
||||
|
||||
|
||||
```python
|
||||
# 显示原图
|
||||
show_img = cv2.imread('/home/aistudio/data/data165849/table_gen_dataset/img/no_border_18298_G7XZH93DDCMATGJQ8RW2.jpg')
|
||||
plt.figure(figsize=(15,15))
|
||||
plt.imshow(show_img)
|
||||
plt.show()
|
||||
|
||||
# 显示预测结果
|
||||
from IPython.core.display import display, HTML
|
||||
display(HTML('<html><body><table><tr><td colspan="5">alleadersh</td><td rowspan="2">不贰过,推</td><td rowspan="2">从自己参与浙江数</td><td rowspan="2">。另一方</td></tr><tr><td>AnSha</td><td>自己越</td><td>共商共建工作协商</td><td>w.east </td><td>抓好改革试点任务</td></tr><tr><td>Edime</td><td>ImisesElec</td><td>怀天下”。</td><td></td><td>22.26 </td><td>31.61</td><td>4.30 </td><td>794.94</td></tr><tr><td rowspan="2">ip</td><td> Profundi</td><td>:2019年12月1</td><td>Horspro</td><td>444.48</td><td>2.41 </td><td>87</td><td>679.98</td></tr><tr><td> iehaiTrain</td><td>组长蒋蕊</td><td>Toafterdec</td><td>203.43</td><td>23.54 </td><td>4</td><td>4266.62</td></tr><tr><td>Tyint </td><td> roudlyRol</td><td>谢您的好意,我知道</td><td>ErChows</td><td></td><td>48.90</td><td>1031</td><td>6</td></tr><tr><td>NaFlint</td><td></td><td>一辈的</td><td>aterreclam</td><td>7823.86</td><td>9829.23</td><td>7.96 </td><td> 3068</td></tr><tr><td>家上下游企业,5</td><td>Tr</td><td>景象。当地球上的我们</td><td>Urelaw</td><td>799.62</td><td>354.96</td><td>12.98</td><td>33 </td></tr><tr><td>赛事(</td><td> uestCh</td><td>复制的业务模式并</td><td>Listicjust</td><td>9.23</td><td></td><td>92</td><td>53.22</td></tr><tr><td> Ca</td><td> Iskole</td><td>扶贫"之名引导</td><td> Papua </td><td>7191.90</td><td>1.65</td><td>3.62</td><td>48</td></tr><tr><td rowspan="2">避讳</td><td>ir</td><td>但由于</td><td>Fficeof</td><td>0.22</td><td>6.37</td><td>7.17</td><td>3397.75</td></tr><tr><td>ndaTurk</td><td>百处遗址</td><td>gMa</td><td>1288.34</td><td>2053.66</td><td>2.29</td><td>885.45</td></tr></table></body></html>'))
|
||||
```
|
||||
|
||||
## 3. 表格属性识别
|
||||
### 3.1 代码、环境、数据准备
|
||||
#### 3.1.1 代码准备
|
||||
首先,我们需要准备训练表格属性的代码,PaddleClas集成了PULC方案,该方案可以快速获得一个在CPU上用时2ms的属性识别模型。PaddleClas代码可以clone下载得到。获取方式如下:
|
||||
|
||||
|
||||
|
||||
```python
|
||||
! git clone -b develop https://gitee.com/paddlepaddle/PaddleClas
|
||||
```
|
||||
|
||||
#### 3.1.2 环境准备
|
||||
其次,我们需要安装训练PaddleClas相关的依赖包
|
||||
|
||||
|
||||
```python
|
||||
! pip install -r PaddleClas/requirements.txt --force-reinstall
|
||||
! pip install protobuf==3.20.0
|
||||
```
|
||||
|
||||
|
||||
#### 3.1.3 数据准备
|
||||
|
||||
最后,准备训练数据。在这里,我们一共定义了表格的6个属性,分别是表格来源、表格数量、表格颜色、表格清晰度、表格有无干扰、表格角度。其可视化如下:
|
||||
|
||||
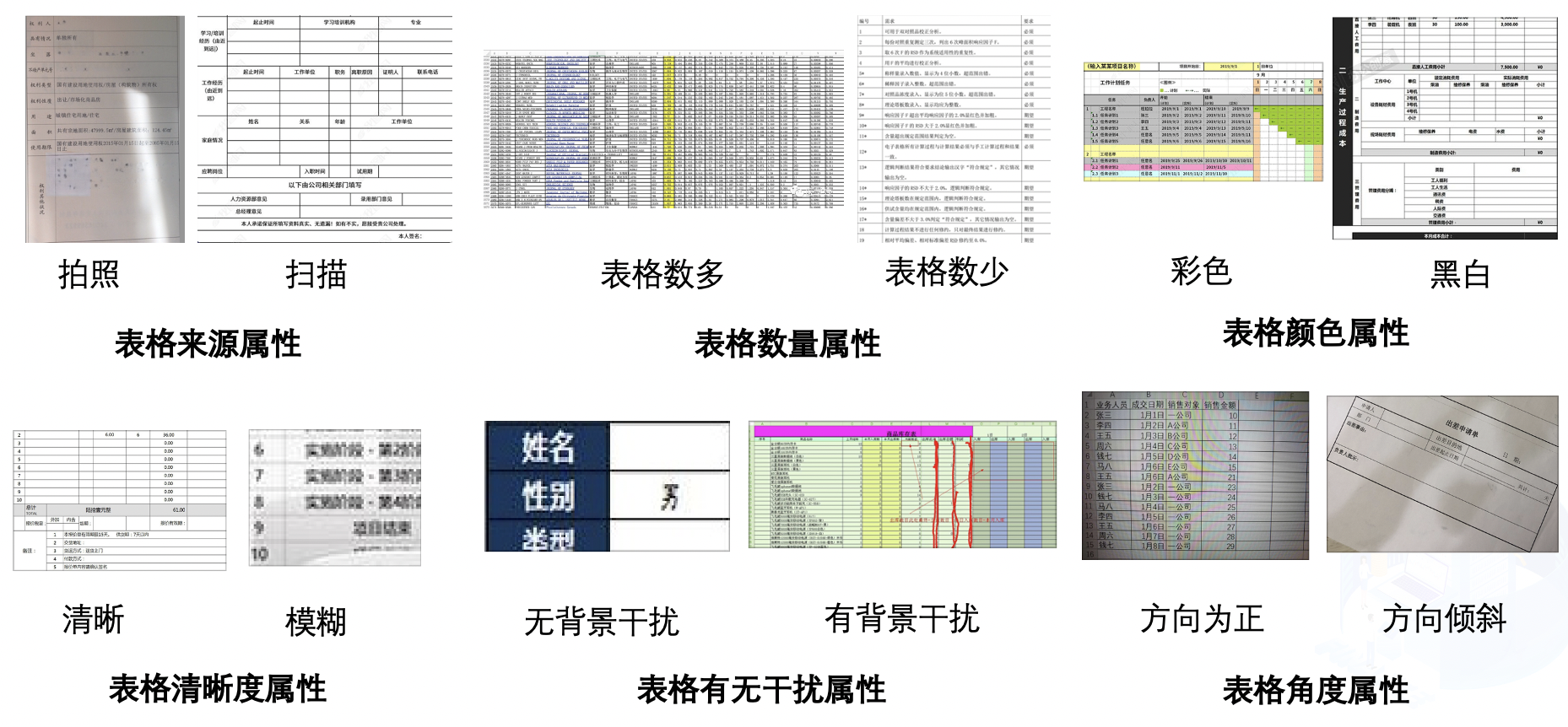
|
||||
|
||||
这里,我们提供了一个表格属性的demo子集,可以快速迭代体验。下载方式如下:
|
||||
|
||||
|
||||
```python
|
||||
%cd PaddleClas/dataset
|
||||
!wget https://paddleclas.bj.bcebos.com/data/PULC/table_attribute.tar
|
||||
!tar -xf table_attribute.tar
|
||||
%cd ../PaddleClas/dataset
|
||||
%cd ../
|
||||
```
|
||||
|
||||
### 3.2 表格属性识别训练
|
||||
表格属性训练整体pipelinie如下:
|
||||
|
||||
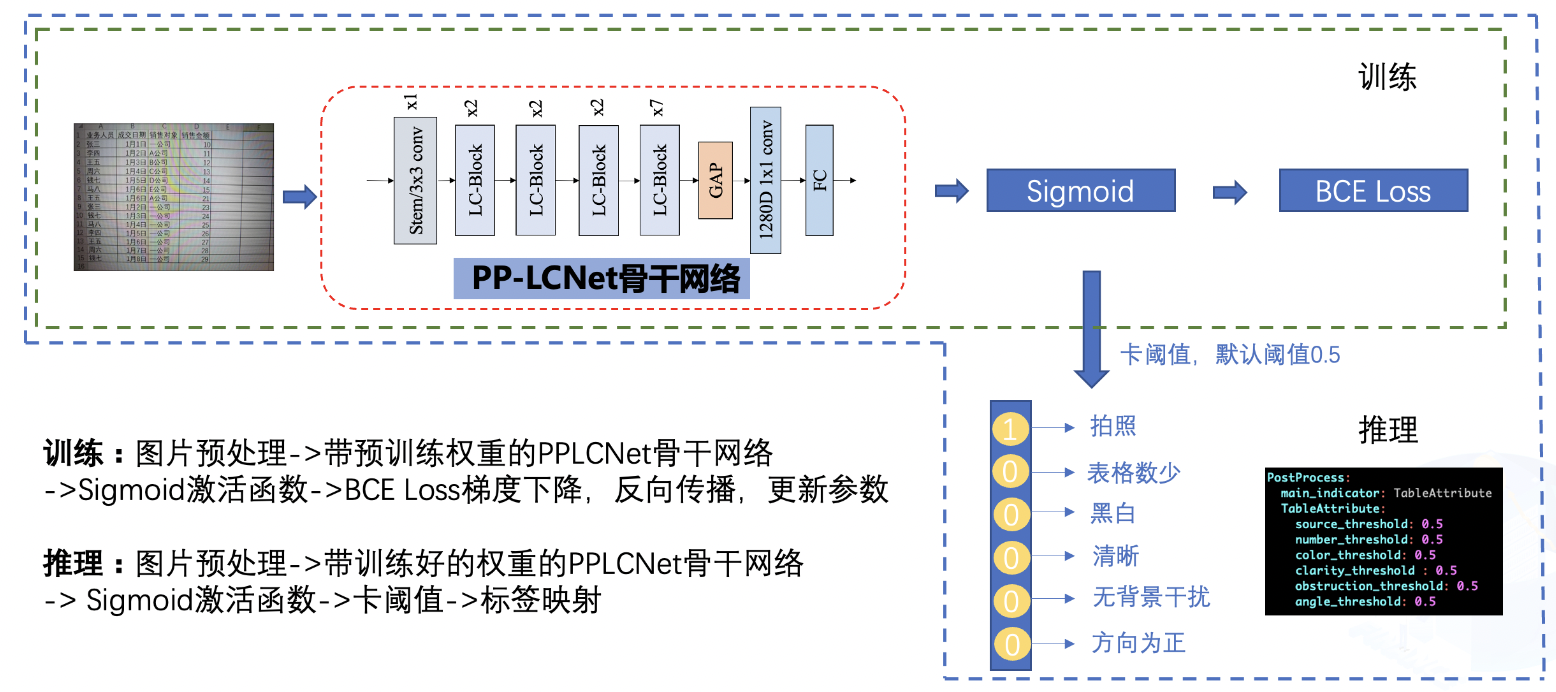
|
||||
|
||||
1.训练过程中,图片经过预处理之后,送入到骨干网络之中,骨干网络将抽取表格图片的特征,最终该特征连接输出的FC层,FC层经过Sigmoid激活函数后和真实标签做交叉熵损失函数,优化器通过对该损失函数做梯度下降来更新骨干网络的参数,经过多轮训练后,骨干网络的参数可以对为止图片做很好的预测;
|
||||
|
||||
2.推理过程中,图片经过预处理之后,送入到骨干网络之中,骨干网络加载学习好的权重后对该表格图片做出预测,预测的结果为一个6维向量,该向量中的每个元素反映了每个属性对应的概率值,通过对该值进一步卡阈值之后,得到最终的输出,最终的输出描述了该表格的6个属性。
|
||||
|
||||
当准备好相关的数据之后,可以一键启动表格属性的训练,训练代码如下:
|
||||
|
||||
|
||||
```python
|
||||
|
||||
!python tools/train.py -c ./ppcls/configs/PULC/table_attribute/PPLCNet_x1_0.yaml -o Global.device=cpu -o Global.epochs=10
|
||||
```
|
||||
|
||||
### 3.3 表格属性识别推理和部署
|
||||
#### 3.3.1 模型转换
|
||||
当训练好模型之后,需要将模型转换为推理模型进行部署。转换脚本如下:
|
||||
|
||||
|
||||
```python
|
||||
!python tools/export_model.py -c ppcls/configs/PULC/table_attribute/PPLCNet_x1_0.yaml -o Global.pretrained_model=output/PPLCNet_x1_0/best_model
|
||||
```
|
||||
|
||||
执行以上命令之后,会在当前目录上生成`inference`文件夹,该文件夹中保存了当前精度最高的推理模型。
|
||||
|
||||
#### 3.3.2 模型推理
|
||||
安装推理需要的paddleclas包, 此时需要通过下载安装paddleclas的develop的whl包
|
||||
|
||||
|
||||
|
||||
```python
|
||||
!pip install https://paddleclas.bj.bcebos.com/whl/paddleclas-0.0.0-py3-none-any.whl
|
||||
```
|
||||
|
||||
进入`deploy`目录下即可对模型进行推理
|
||||
|
||||
|
||||
```python
|
||||
%cd deploy/
|
||||
```
|
||||
|
||||
推理命令如下:
|
||||
|
||||
|
||||
```python
|
||||
!python python/predict_cls.py -c configs/PULC/table_attribute/inference_table_attribute.yaml -o Global.inference_model_dir="../inference" -o Global.infer_imgs="../dataset/table_attribute/Table_val/val_9.jpg"
|
||||
!python python/predict_cls.py -c configs/PULC/table_attribute/inference_table_attribute.yaml -o Global.inference_model_dir="../inference" -o Global.infer_imgs="../dataset/table_attribute/Table_val/val_3253.jpg"
|
||||
```
|
||||
|
||||
推理的表格图片:
|
||||
|
||||
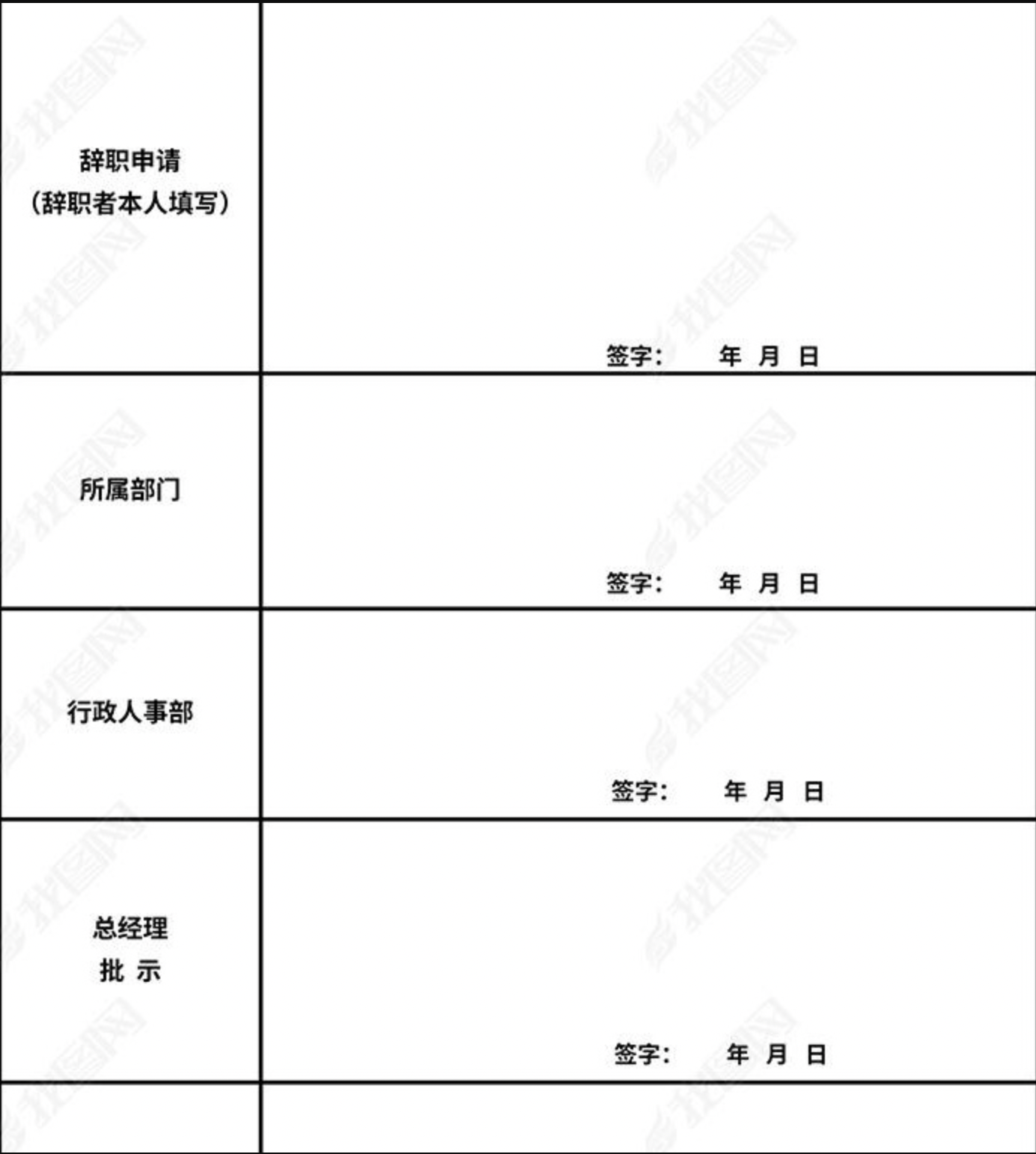
|
||||
|
||||
预测结果如下:
|
||||
```
|
||||
val_9.jpg: {'attributes': ['Scanned', 'Little', 'Black-and-White', 'Clear', 'Without-Obstacles', 'Horizontal'], 'output': [1, 1, 1, 1, 1, 1]}
|
||||
```
|
||||
|
||||
|
||||
推理的表格图片:
|
||||
|
||||
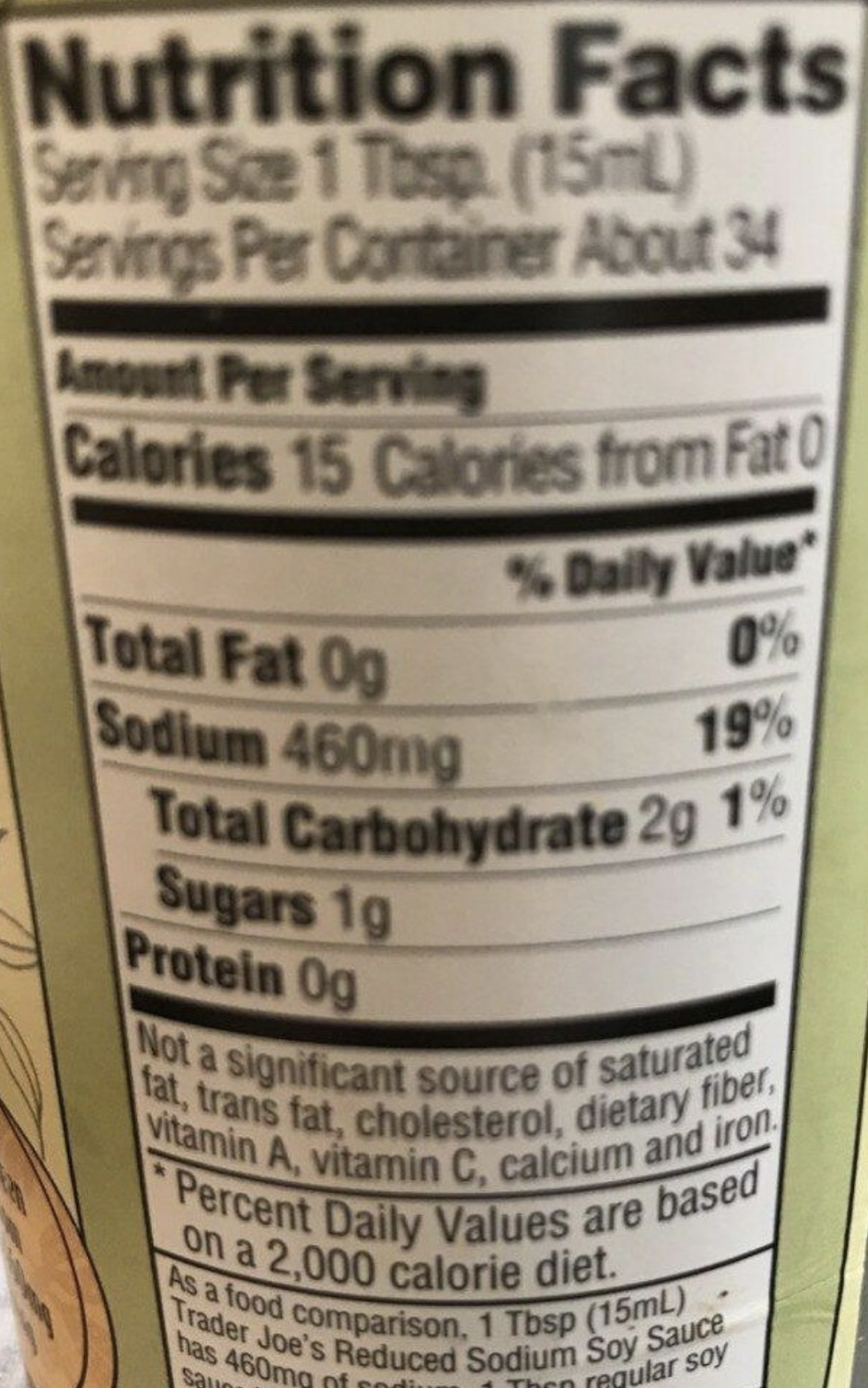
|
||||
|
||||
预测结果如下:
|
||||
```
|
||||
val_3253.jpg: {'attributes': ['Photo', 'Little', 'Black-and-White', 'Blurry', 'Without-Obstacles', 'Tilted'], 'output': [0, 1, 1, 0, 1, 0]}
|
||||
```
|
||||
|
||||
对比两张图片可以发现,第一张图片比较清晰,表格属性的结果也偏向于比较容易识别,我们可以更相信表格识别的结果,第二张图片比较模糊,且存在倾斜现象,表格识别可能存在错误,需要我们人工进一步校验。通过表格的属性识别能力,可以进一步将“人工”和“智能”很好的结合起来,为表格识别能力的落地的精度提供保障。
|
File diff suppressed because it is too large
Load Diff
|
@ -0,0 +1,782 @@
|
|||
# 快速构建卡证类OCR
|
||||
|
||||
|
||||
- [快速构建卡证类OCR](#快速构建卡证类ocr)
|
||||
- [1. 金融行业卡证识别应用](#1-金融行业卡证识别应用)
|
||||
- [1.1 金融行业中的OCR相关技术](#11-金融行业中的ocr相关技术)
|
||||
- [1.2 金融行业中的卡证识别场景介绍](#12-金融行业中的卡证识别场景介绍)
|
||||
- [1.3 OCR落地挑战](#13-ocr落地挑战)
|
||||
- [2. 卡证识别技术解析](#2-卡证识别技术解析)
|
||||
- [2.1 卡证分类模型](#21-卡证分类模型)
|
||||
- [2.2 卡证识别模型](#22-卡证识别模型)
|
||||
- [3. OCR技术拆解](#3-ocr技术拆解)
|
||||
- [3.1技术流程](#31技术流程)
|
||||
- [3.2 OCR技术拆解---卡证分类](#32-ocr技术拆解---卡证分类)
|
||||
- [卡证分类:数据、模型准备](#卡证分类数据模型准备)
|
||||
- [卡证分类---修改配置文件](#卡证分类---修改配置文件)
|
||||
- [卡证分类---训练](#卡证分类---训练)
|
||||
- [3.2 OCR技术拆解---卡证识别](#32-ocr技术拆解---卡证识别)
|
||||
- [身份证识别:检测+分类](#身份证识别检测分类)
|
||||
- [数据标注](#数据标注)
|
||||
- [4 . 项目实践](#4--项目实践)
|
||||
- [4.1 环境准备](#41-环境准备)
|
||||
- [4.2 配置文件修改](#42-配置文件修改)
|
||||
- [4.3 代码修改](#43-代码修改)
|
||||
- [4.3.1 数据读取](#431-数据读取)
|
||||
- [4.3.2 head修改](#432--head修改)
|
||||
- [4.3.3 修改loss](#433-修改loss)
|
||||
- [4.3.4 后处理](#434-后处理)
|
||||
- [4.4. 模型启动](#44-模型启动)
|
||||
- [5 总结](#5-总结)
|
||||
- [References](#references)
|
||||
|
||||
## 1. 金融行业卡证识别应用
|
||||
|
||||
### 1.1 金融行业中的OCR相关技术
|
||||
|
||||
* 《“十四五”数字经济发展规划》指出,2020年我国数字经济核心产业增加值占GDP比重达7.8%,随着数字经济迈向全面扩展,到2025年该比例将提升至10%。
|
||||
|
||||
* 在过去数年的跨越发展与积累沉淀中,数字金融、金融科技已在对金融业的重塑与再造中充分印证了其自身价值。
|
||||
|
||||
* 以智能为目标,提升金融数字化水平,实现业务流程自动化,降低人力成本。
|
||||
|
||||
|
||||
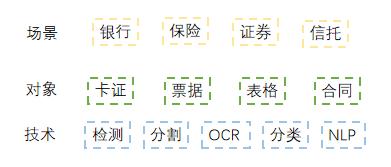
|
||||
|
||||
|
||||
|
||||
### 1.2 金融行业中的卡证识别场景介绍
|
||||
|
||||
应用场景:身份证、银行卡、营业执照、驾驶证等。
|
||||
|
||||
应用难点:由于数据的采集来源多样,以及实际采集数据各种噪声:反光、褶皱、模糊、倾斜等各种问题干扰。
|
||||
|
||||
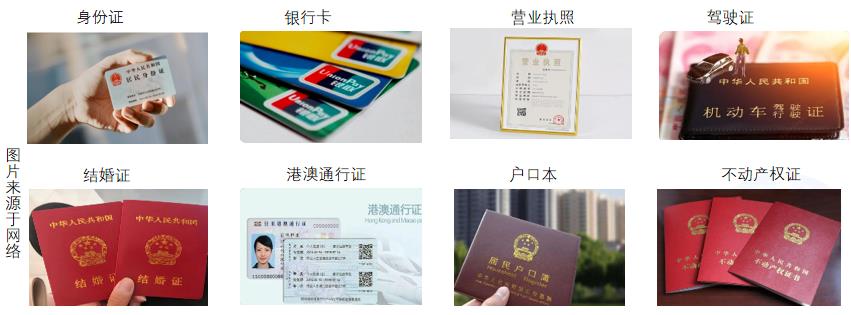
|
||||
|
||||
|
||||
|
||||
### 1.3 OCR落地挑战
|
||||
|
||||
|
||||
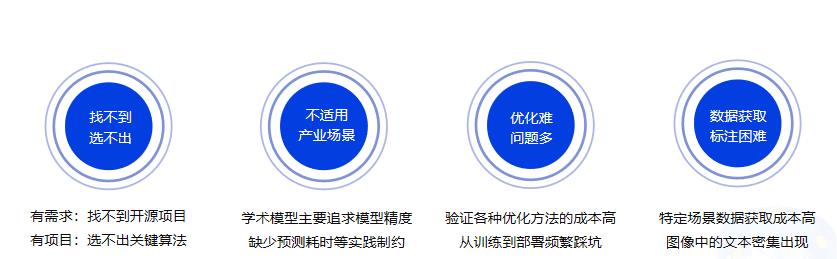
|
||||
|
||||
|
||||
|
||||
|
||||
|
||||
## 2. 卡证识别技术解析
|
||||
|
||||
|
||||
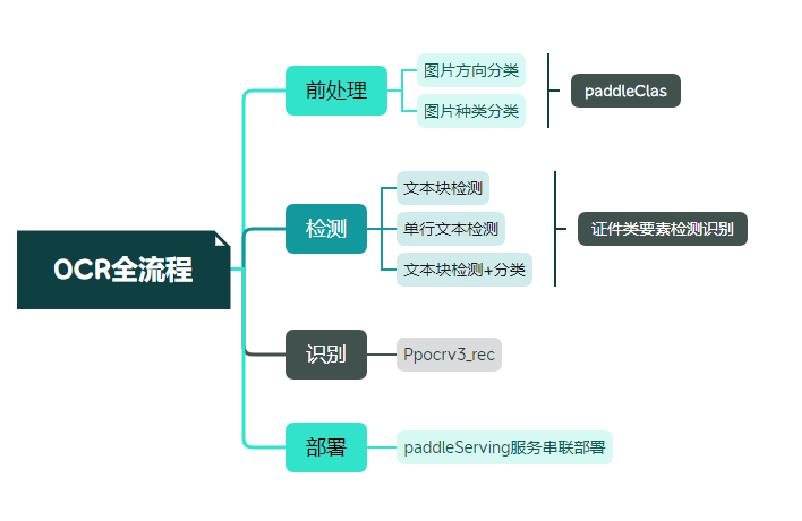
|
||||
|
||||
|
||||
### 2.1 卡证分类模型
|
||||
|
||||
卡证分类:基于PPLCNet
|
||||
|
||||
与其他轻量级模型相比在CPU环境下ImageNet数据集上的表现
|
||||
|
||||
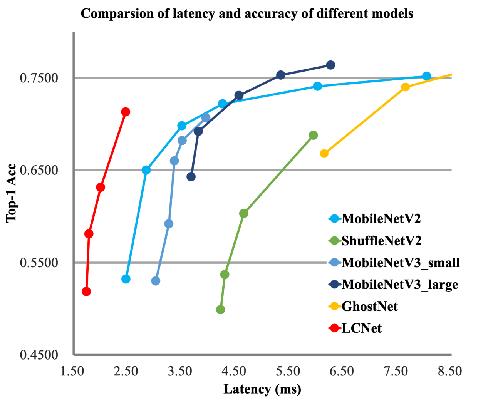
|
||||
|
||||
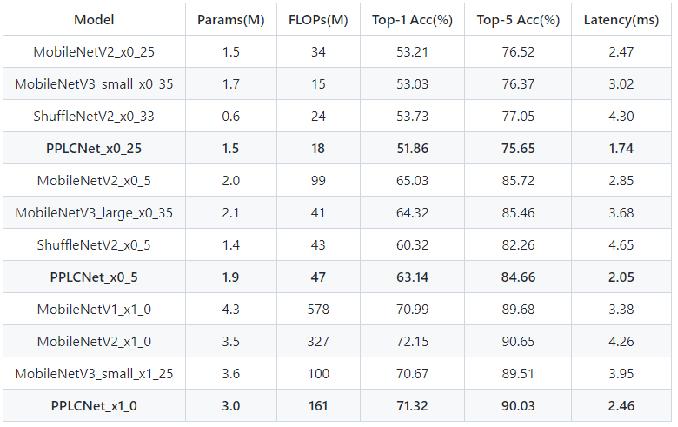
|
||||
|
||||
|
||||
|
||||
* 模型来自模型库PaddleClas,它是一个图像识别和图像分类任务的工具集,助力使用者训练出更好的视觉模型和应用落地。
|
||||
|
||||
|
||||
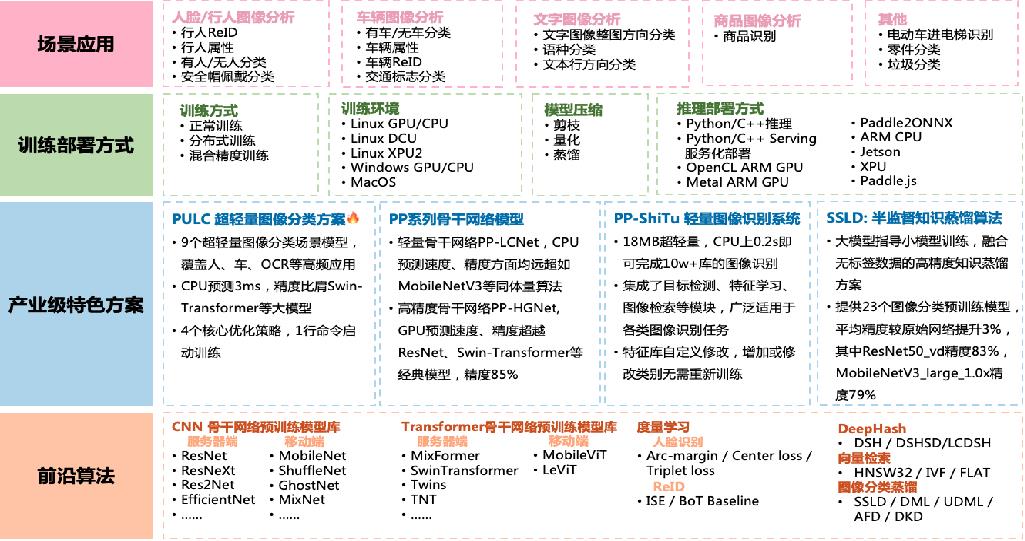
|
||||
|
||||
|
||||
|
||||
### 2.2 卡证识别模型
|
||||
|
||||
* 检测:DBNet 识别:SVRT
|
||||
|
||||

|
||||
|
||||
|
||||
* PPOCRv3在文本检测、识别进行了一系列改进优化,在保证精度的同时提升预测效率
|
||||
|
||||
|
||||
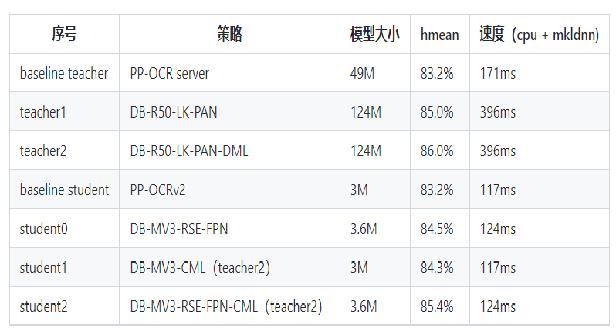
|
||||
|
||||
|
||||
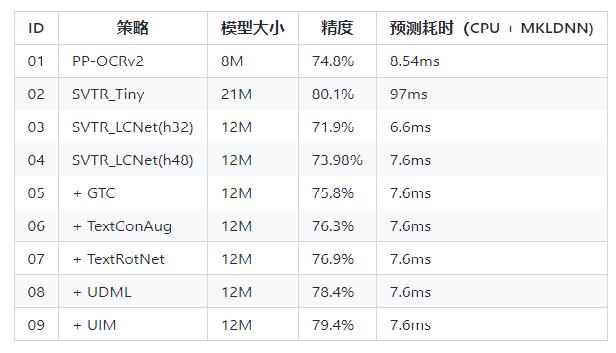
|
||||
|
||||
|
||||
## 3. OCR技术拆解
|
||||
|
||||
### 3.1技术流程
|
||||
|
||||
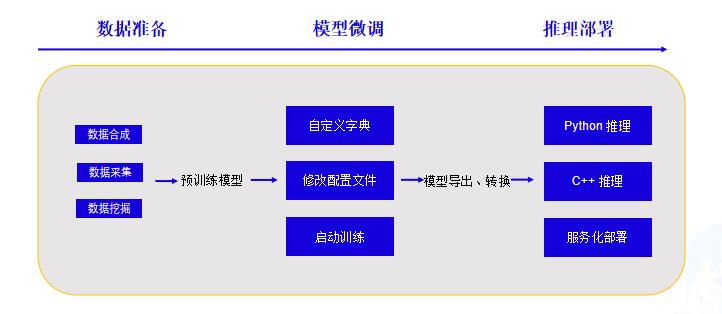
|
||||
|
||||
|
||||
### 3.2 OCR技术拆解---卡证分类
|
||||
|
||||
#### 卡证分类:数据、模型准备
|
||||
|
||||
|
||||
A 使用爬虫获取无标注数据,将相同类别的放在同一文件夹下,文件名从0开始命名。具体格式如下图所示。
|
||||
|
||||
注:卡证类数据,建议每个类别数据量在500张以上
|
||||
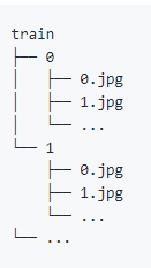
|
||||
|
||||
|
||||
B 一行命令生成标签文件
|
||||
|
||||
```
|
||||
tree -r -i -f | grep -E "jpg|JPG|jpeg|JPEG|png|PNG|webp" | awk -F "/" '{print $0" "$2}' > train_list.txt
|
||||
```
|
||||
|
||||
C [下载预训练模型 ](https://github.com/PaddlePaddle/PaddleClas/blob/release/2.4/docs/zh_CN/models/PP-LCNet.md)
|
||||
|
||||
|
||||
|
||||
#### 卡证分类---修改配置文件
|
||||
|
||||
|
||||
配置文件主要修改三个部分:
|
||||
|
||||
全局参数:预训练模型路径/训练轮次/图像尺寸
|
||||
|
||||
模型结构:分类数
|
||||
|
||||
数据处理:训练/评估数据路径
|
||||
|
||||
|
||||
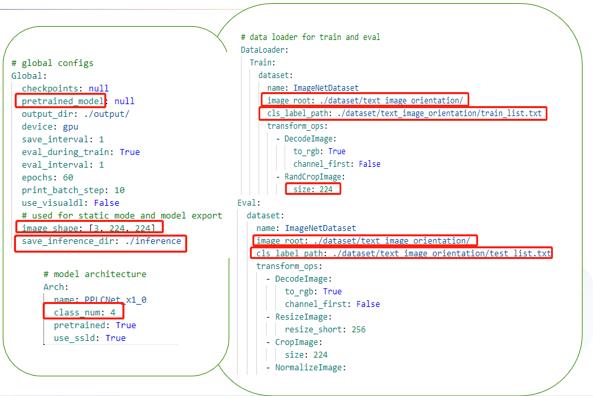
|
||||
|
||||
#### 卡证分类---训练
|
||||
|
||||
|
||||
指定配置文件启动训练:
|
||||
|
||||
```
|
||||
!python /home/aistudio/work/PaddleClas/tools/train.py -c /home/aistudio/work/PaddleClas/ppcls/configs/PULC/text_image_orientation/PPLCNet_x1_0.yaml
|
||||
```
|
||||
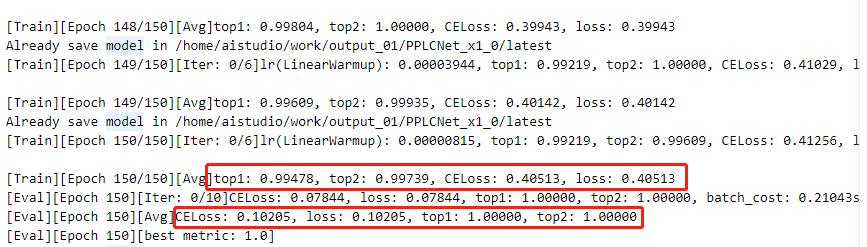
|
||||
|
||||
注:日志中显示了训练结果和评估结果(训练时可以设置固定轮数评估一次)
|
||||
|
||||
|
||||
### 3.2 OCR技术拆解---卡证识别
|
||||
|
||||
卡证识别(以身份证检测为例)
|
||||
存在的困难及问题:
|
||||
|
||||
* 在自然场景下,由于各种拍摄设备以及光线、角度不同等影响导致实际得到的证件影像千差万别。
|
||||
|
||||
* 如何快速提取需要的关键信息
|
||||
|
||||
* 多行的文本信息,检测结果如何正确拼接
|
||||
|
||||
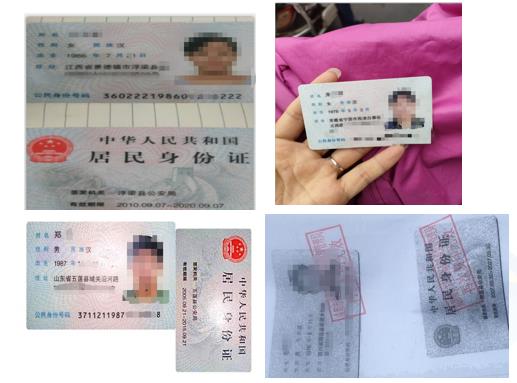
|
||||
|
||||
|
||||
|
||||
* OCR技术拆解---OCR工具库
|
||||
|
||||
PaddleOCR是一个丰富、领先且实用的OCR工具库,助力开发者训练出更好的模型并应用落地
|
||||
|
||||
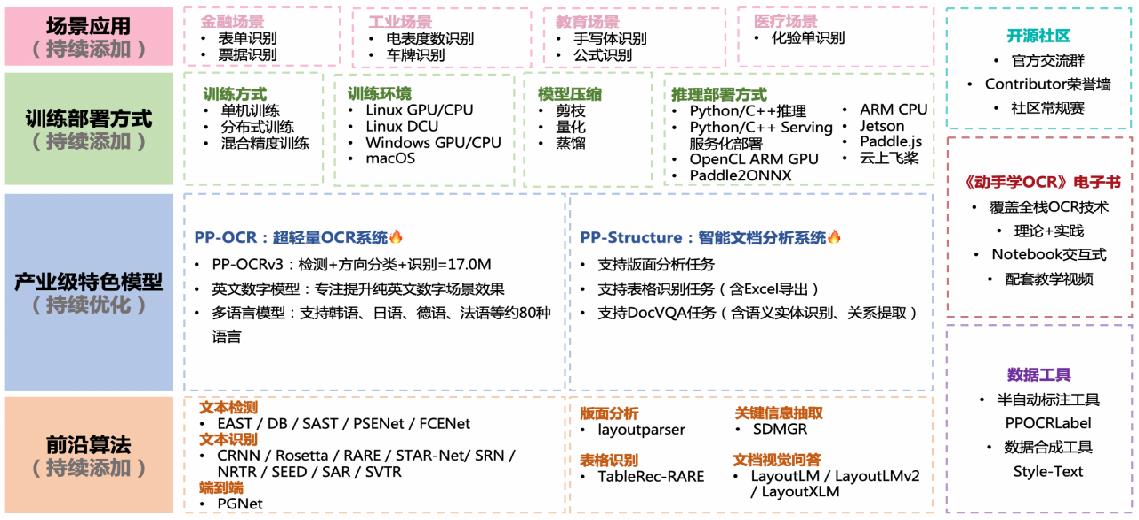
|
||||
|
||||
|
||||
身份证识别:用现有的方法识别
|
||||
|
||||
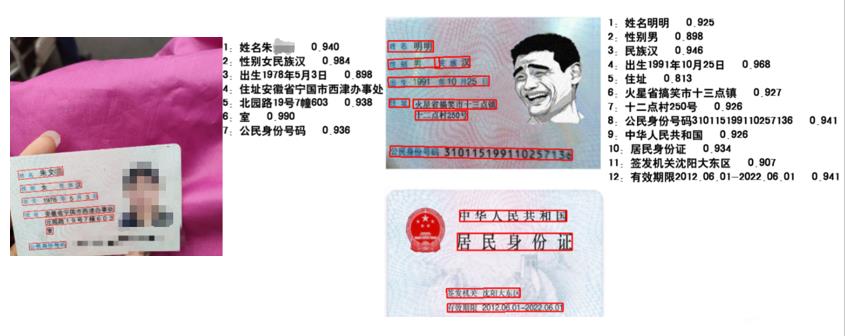
|
||||
|
||||
|
||||
|
||||
|
||||
#### 身份证识别:检测+分类
|
||||
|
||||
> 方法:基于现有的dbnet检测模型,加入分类方法。检测同时进行分类,从一定程度上优化识别流程
|
||||
|
||||
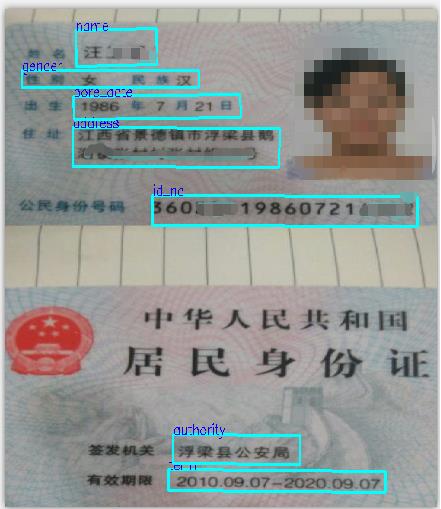
|
||||
|
||||
|
||||
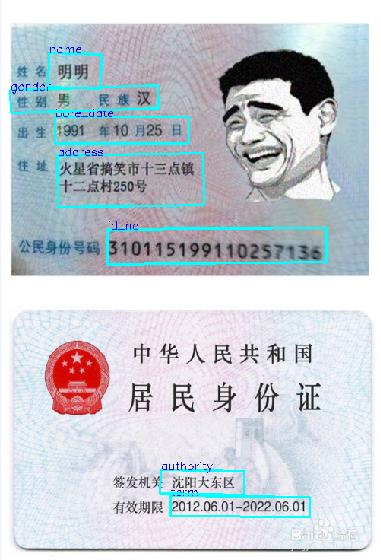
|
||||
|
||||
#### 数据标注
|
||||
|
||||
使用PaddleOCRLable进行快速标注
|
||||
|
||||
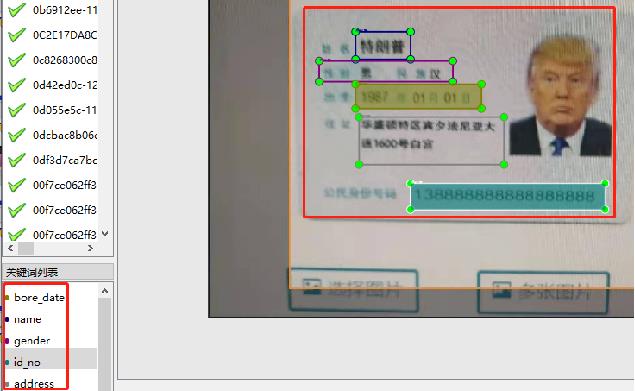
|
||||
|
||||
|
||||
* 修改PPOCRLabel.py,将下图中的kie参数设置为True
|
||||
|
||||
|
||||

|
||||
|
||||
|
||||
* 数据标注踩坑分享
|
||||
|
||||
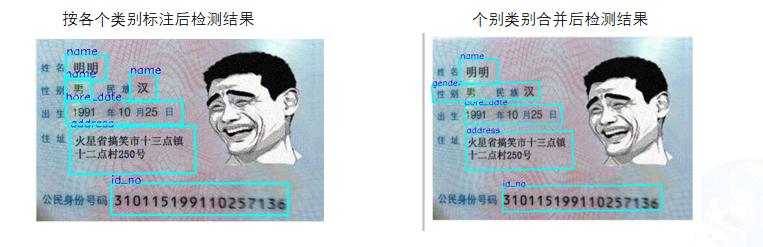
|
||||
|
||||
注:两者只有标注有差别,训练参数数据集都相同
|
||||
|
||||
## 4 . 项目实践
|
||||
|
||||
AIStudio项目链接:[快速构建卡证类OCR](https://aistudio.baidu.com/aistudio/projectdetail/4459116)
|
||||
|
||||
### 4.1 环境准备
|
||||
|
||||
1)拉取[paddleocr](https://github.com/PaddlePaddle/PaddleOCR)项目,如果从github上拉取速度慢可以选择从gitee上获取。
|
||||
```
|
||||
!git clone https://github.com/PaddlePaddle/PaddleOCR.git -b release/2.6 /home/aistudio/work/
|
||||
```
|
||||
|
||||
2)获取并解压预训练模型,如果要使用其他模型可以从模型库里自主选择合适模型。
|
||||
```
|
||||
!wget -P work/pre_trained/ https://paddleocr.bj.bcebos.com/PP-OCRv3/chinese/ch_PP-OCRv3_det_distill_train.tar
|
||||
!tar -vxf /home/aistudio/work/pre_trained/ch_PP-OCRv3_det_distill_train.tar -C /home/aistudio/work/pre_trained
|
||||
```
|
||||
3) 安装必要依赖
|
||||
```
|
||||
!pip install -r /home/aistudio/work/requirements.txt
|
||||
```
|
||||
|
||||
### 4.2 配置文件修改
|
||||
|
||||
修改配置文件 *work/configs/det/detmv3db.yml*
|
||||
|
||||
具体修改说明如下:
|
||||
|
||||
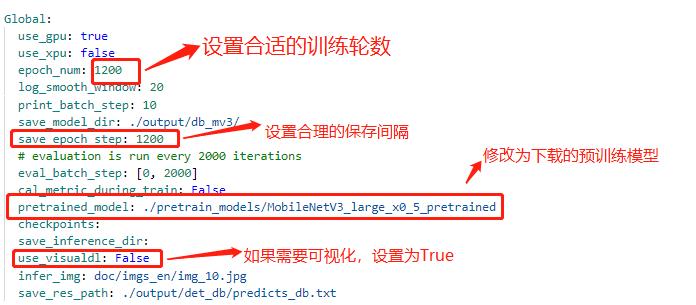
|
||||
|
||||
注:在上述的配置文件的Global变量中需要添加以下两个参数:
|
||||
|
||||
label_list 为标签表
|
||||
num_classes 为分类数
|
||||
上述两个参数根据实际的情况配置即可
|
||||
|
||||
|
||||

|
||||
|
||||
其中lable_list内容如下例所示,***建议第一个参数设置为 background,不要设置为实际要提取的关键信息种类***:
|
||||
|
||||
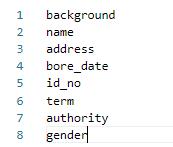
|
||||
|
||||
配置文件中的其他设置说明
|
||||
|
||||
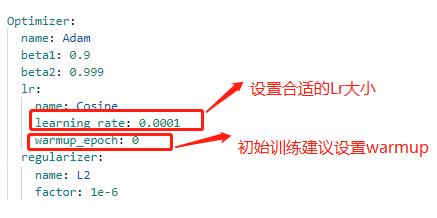
|
||||
|
||||
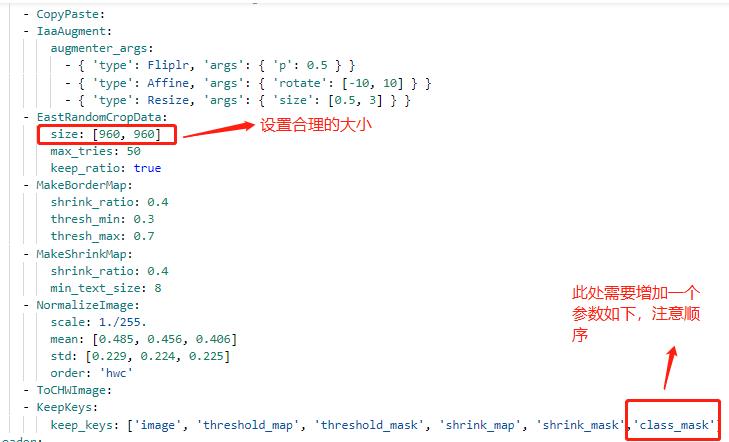
|
||||
|
||||
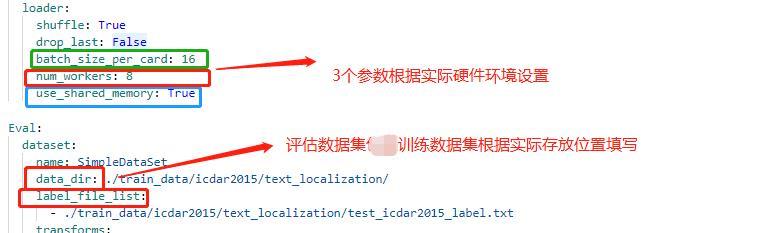
|
||||
|
||||
|
||||
|
||||
|
||||
### 4.3 代码修改
|
||||
|
||||
|
||||
#### 4.3.1 数据读取
|
||||
|
||||
|
||||
|
||||
* 修改 PaddleOCR/ppocr/data/imaug/label_ops.py中的DetLabelEncode
|
||||
|
||||
|
||||
```python
|
||||
class DetLabelEncode(object):
|
||||
|
||||
# 修改检测标签的编码处,新增了参数分类数:num_classes,重写初始化方法,以及分类标签的读取
|
||||
|
||||
def __init__(self, label_list, num_classes=8, **kwargs):
|
||||
self.num_classes = num_classes
|
||||
self.label_list = []
|
||||
if label_list:
|
||||
if isinstance(label_list, str):
|
||||
with open(label_list, 'r+', encoding='utf-8') as f:
|
||||
for line in f.readlines():
|
||||
self.label_list.append(line.replace("\n", ""))
|
||||
else:
|
||||
self.label_list = label_list
|
||||
else:
|
||||
assert ' please check label_list whether it is none or config is right'
|
||||
|
||||
if num_classes != len(self.label_list): # 校验分类数和标签的一致性
|
||||
assert 'label_list length is not equal to the num_classes'
|
||||
|
||||
def __call__(self, data):
|
||||
label = data['label']
|
||||
label = json.loads(label)
|
||||
nBox = len(label)
|
||||
boxes, txts, txt_tags, classes = [], [], [], []
|
||||
for bno in range(0, nBox):
|
||||
box = label[bno]['points']
|
||||
txt = label[bno]['key_cls'] # 此处将kie中的参数作为分类读取
|
||||
boxes.append(box)
|
||||
txts.append(txt)
|
||||
|
||||
if txt in ['*', '###']:
|
||||
txt_tags.append(True)
|
||||
if self.num_classes > 1:
|
||||
classes.append(-2)
|
||||
else:
|
||||
txt_tags.append(False)
|
||||
if self.num_classes > 1: # 将KIE内容的key标签作为分类标签使用
|
||||
classes.append(int(self.label_list.index(txt)))
|
||||
|
||||
if len(boxes) == 0:
|
||||
|
||||
return None
|
||||
boxes = self.expand_points_num(boxes)
|
||||
boxes = np.array(boxes, dtype=np.float32)
|
||||
txt_tags = np.array(txt_tags, dtype=np.bool)
|
||||
classes = classes
|
||||
data['polys'] = boxes
|
||||
data['texts'] = txts
|
||||
data['ignore_tags'] = txt_tags
|
||||
if self.num_classes > 1:
|
||||
data['classes'] = classes
|
||||
return data
|
||||
```
|
||||
|
||||
* 修改 PaddleOCR/ppocr/data/imaug/make_shrink_map.py中的MakeShrinkMap类。这里需要注意的是,如果我们设置的label_list中的第一个参数为要检测的信息那么会得到如下的mask,
|
||||
|
||||
举例说明:
|
||||
这是检测的mask图,图中有四个mask那么实际对应的分类应该是4类
|
||||
|
||||
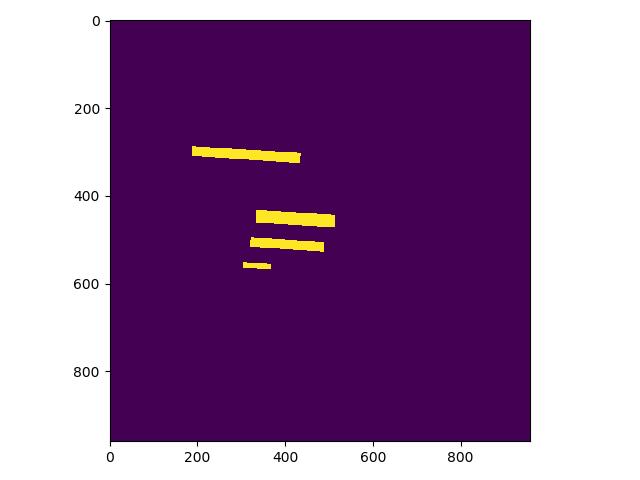
|
||||
|
||||
|
||||
|
||||
label_list中第一个为关键分类,则得到的分类Mask实际如下,与上图相比,少了一个box:
|
||||
|
||||
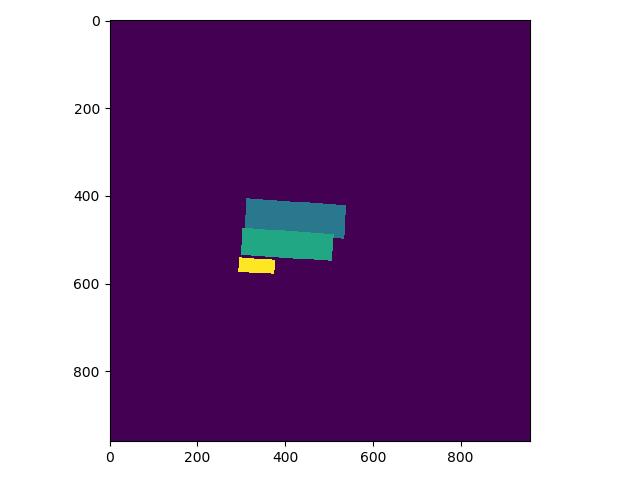
|
||||
|
||||
|
||||
|
||||
```python
|
||||
class MakeShrinkMap(object):
|
||||
r'''
|
||||
Making binary mask from detection data with ICDAR format.
|
||||
Typically following the process of class `MakeICDARData`.
|
||||
'''
|
||||
|
||||
def __init__(self, min_text_size=8, shrink_ratio=0.4, num_classes=8, **kwargs):
|
||||
self.min_text_size = min_text_size
|
||||
self.shrink_ratio = shrink_ratio
|
||||
self.num_classes = num_classes # 添加了分类
|
||||
|
||||
def __call__(self, data):
|
||||
image = data['image']
|
||||
text_polys = data['polys']
|
||||
ignore_tags = data['ignore_tags']
|
||||
if self.num_classes > 1:
|
||||
classes = data['classes']
|
||||
|
||||
h, w = image.shape[:2]
|
||||
text_polys, ignore_tags = self.validate_polygons(text_polys,
|
||||
ignore_tags, h, w)
|
||||
gt = np.zeros((h, w), dtype=np.float32)
|
||||
mask = np.ones((h, w), dtype=np.float32)
|
||||
gt_class = np.zeros((h, w), dtype=np.float32) # 新增分类
|
||||
for i in range(len(text_polys)):
|
||||
polygon = text_polys[i]
|
||||
height = max(polygon[:, 1]) - min(polygon[:, 1])
|
||||
width = max(polygon[:, 0]) - min(polygon[:, 0])
|
||||
if ignore_tags[i] or min(height, width) < self.min_text_size:
|
||||
cv2.fillPoly(mask,
|
||||
polygon.astype(np.int32)[np.newaxis, :, :], 0)
|
||||
ignore_tags[i] = True
|
||||
else:
|
||||
polygon_shape = Polygon(polygon)
|
||||
subject = [tuple(l) for l in polygon]
|
||||
padding = pyclipper.PyclipperOffset()
|
||||
padding.AddPath(subject, pyclipper.JT_ROUND,
|
||||
pyclipper.ET_CLOSEDPOLYGON)
|
||||
shrinked = []
|
||||
|
||||
# Increase the shrink ratio every time we get multiple polygon returned back
|
||||
possible_ratios = np.arange(self.shrink_ratio, 1,
|
||||
self.shrink_ratio)
|
||||
np.append(possible_ratios, 1)
|
||||
for ratio in possible_ratios:
|
||||
distance = polygon_shape.area * (
|
||||
1 - np.power(ratio, 2)) / polygon_shape.length
|
||||
shrinked = padding.Execute(-distance)
|
||||
if len(shrinked) == 1:
|
||||
break
|
||||
|
||||
if shrinked == []:
|
||||
cv2.fillPoly(mask,
|
||||
polygon.astype(np.int32)[np.newaxis, :, :], 0)
|
||||
ignore_tags[i] = True
|
||||
continue
|
||||
|
||||
for each_shirnk in shrinked:
|
||||
shirnk = np.array(each_shirnk).reshape(-1, 2)
|
||||
cv2.fillPoly(gt, [shirnk.astype(np.int32)], 1)
|
||||
if self.num_classes > 1: # 绘制分类的mask
|
||||
cv2.fillPoly(gt_class, polygon.astype(np.int32)[np.newaxis, :, :], classes[i])
|
||||
|
||||
|
||||
data['shrink_map'] = gt
|
||||
|
||||
if self.num_classes > 1:
|
||||
data['class_mask'] = gt_class
|
||||
|
||||
data['shrink_mask'] = mask
|
||||
return data
|
||||
```
|
||||
|
||||
由于在训练数据中会对数据进行resize设置,yml中的操作为:EastRandomCropData,所以需要修改PaddleOCR/ppocr/data/imaug/random_crop_data.py中的EastRandomCropData
|
||||
|
||||
|
||||
```python
|
||||
class EastRandomCropData(object):
|
||||
def __init__(self,
|
||||
size=(640, 640),
|
||||
max_tries=10,
|
||||
min_crop_side_ratio=0.1,
|
||||
keep_ratio=True,
|
||||
num_classes=8,
|
||||
**kwargs):
|
||||
self.size = size
|
||||
self.max_tries = max_tries
|
||||
self.min_crop_side_ratio = min_crop_side_ratio
|
||||
self.keep_ratio = keep_ratio
|
||||
self.num_classes = num_classes
|
||||
|
||||
def __call__(self, data):
|
||||
img = data['image']
|
||||
text_polys = data['polys']
|
||||
ignore_tags = data['ignore_tags']
|
||||
texts = data['texts']
|
||||
if self.num_classes > 1:
|
||||
classes = data['classes']
|
||||
all_care_polys = [
|
||||
text_polys[i] for i, tag in enumerate(ignore_tags) if not tag
|
||||
]
|
||||
# 计算crop区域
|
||||
crop_x, crop_y, crop_w, crop_h = crop_area(
|
||||
img, all_care_polys, self.min_crop_side_ratio, self.max_tries)
|
||||
# crop 图片 保持比例填充
|
||||
scale_w = self.size[0] / crop_w
|
||||
scale_h = self.size[1] / crop_h
|
||||
scale = min(scale_w, scale_h)
|
||||
h = int(crop_h * scale)
|
||||
w = int(crop_w * scale)
|
||||
if self.keep_ratio:
|
||||
padimg = np.zeros((self.size[1], self.size[0], img.shape[2]),
|
||||
img.dtype)
|
||||
padimg[:h, :w] = cv2.resize(
|
||||
img[crop_y:crop_y + crop_h, crop_x:crop_x + crop_w], (w, h))
|
||||
img = padimg
|
||||
else:
|
||||
img = cv2.resize(
|
||||
img[crop_y:crop_y + crop_h, crop_x:crop_x + crop_w],
|
||||
tuple(self.size))
|
||||
# crop 文本框
|
||||
text_polys_crop = []
|
||||
ignore_tags_crop = []
|
||||
texts_crop = []
|
||||
classes_crop = []
|
||||
for poly, text, tag,class_index in zip(text_polys, texts, ignore_tags,classes):
|
||||
poly = ((poly - (crop_x, crop_y)) * scale).tolist()
|
||||
if not is_poly_outside_rect(poly, 0, 0, w, h):
|
||||
text_polys_crop.append(poly)
|
||||
ignore_tags_crop.append(tag)
|
||||
texts_crop.append(text)
|
||||
if self.num_classes > 1:
|
||||
classes_crop.append(class_index)
|
||||
data['image'] = img
|
||||
data['polys'] = np.array(text_polys_crop)
|
||||
data['ignore_tags'] = ignore_tags_crop
|
||||
data['texts'] = texts_crop
|
||||
if self.num_classes > 1:
|
||||
data['classes'] = classes_crop
|
||||
return data
|
||||
```
|
||||
|
||||
#### 4.3.2 head修改
|
||||
|
||||
|
||||
|
||||
主要修改 ppocr/modeling/heads/det_db_head.py,将Head类中的最后一层的输出修改为实际的分类数,同时在DBHead中新增分类的head。
|
||||
|
||||
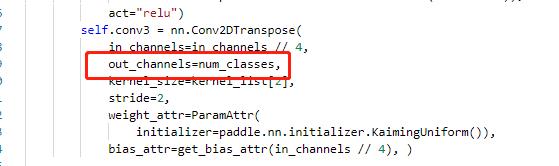
|
||||
|
||||
|
||||
|
||||
#### 4.3.3 修改loss
|
||||
|
||||
|
||||
修改PaddleOCR/ppocr/losses/det_db_loss.py中的DBLoss类,分类采用交叉熵损失函数进行计算。
|
||||
|
||||
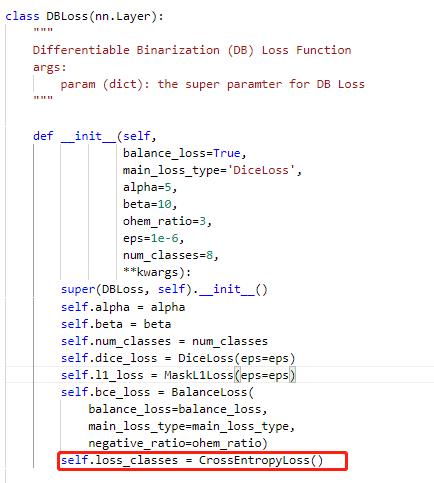
|
||||
|
||||
|
||||
#### 4.3.4 后处理
|
||||
|
||||
|
||||
|
||||
由于涉及到eval以及后续推理能否正常使用,我们需要修改后处理的相关代码,修改位置 PaddleOCR/ppocr/postprocess/db_postprocess.py中的DBPostProcess类
|
||||
|
||||
|
||||
```python
|
||||
class DBPostProcess(object):
|
||||
"""
|
||||
The post process for Differentiable Binarization (DB).
|
||||
"""
|
||||
|
||||
def __init__(self,
|
||||
thresh=0.3,
|
||||
box_thresh=0.7,
|
||||
max_candidates=1000,
|
||||
unclip_ratio=2.0,
|
||||
use_dilation=False,
|
||||
score_mode="fast",
|
||||
**kwargs):
|
||||
self.thresh = thresh
|
||||
self.box_thresh = box_thresh
|
||||
self.max_candidates = max_candidates
|
||||
self.unclip_ratio = unclip_ratio
|
||||
self.min_size = 3
|
||||
self.score_mode = score_mode
|
||||
assert score_mode in [
|
||||
"slow", "fast"
|
||||
], "Score mode must be in [slow, fast] but got: {}".format(score_mode)
|
||||
|
||||
self.dilation_kernel = None if not use_dilation else np.array(
|
||||
[[1, 1], [1, 1]])
|
||||
|
||||
def boxes_from_bitmap(self, pred, _bitmap, classes, dest_width, dest_height):
|
||||
"""
|
||||
_bitmap: single map with shape (1, H, W),
|
||||
whose values are binarized as {0, 1}
|
||||
"""
|
||||
|
||||
bitmap = _bitmap
|
||||
height, width = bitmap.shape
|
||||
|
||||
outs = cv2.findContours((bitmap * 255).astype(np.uint8), cv2.RETR_LIST,
|
||||
cv2.CHAIN_APPROX_SIMPLE)
|
||||
if len(outs) == 3:
|
||||
img, contours, _ = outs[0], outs[1], outs[2]
|
||||
elif len(outs) == 2:
|
||||
contours, _ = outs[0], outs[1]
|
||||
|
||||
num_contours = min(len(contours), self.max_candidates)
|
||||
|
||||
boxes = []
|
||||
scores = []
|
||||
class_indexes = []
|
||||
class_scores = []
|
||||
for index in range(num_contours):
|
||||
contour = contours[index]
|
||||
points, sside = self.get_mini_boxes(contour)
|
||||
if sside < self.min_size:
|
||||
continue
|
||||
points = np.array(points)
|
||||
if self.score_mode == "fast":
|
||||
score, class_index, class_score = self.box_score_fast(pred, points.reshape(-1, 2), classes)
|
||||
else:
|
||||
score, class_index, class_score = self.box_score_slow(pred, contour, classes)
|
||||
if self.box_thresh > score:
|
||||
continue
|
||||
|
||||
box = self.unclip(points).reshape(-1, 1, 2)
|
||||
box, sside = self.get_mini_boxes(box)
|
||||
if sside < self.min_size + 2:
|
||||
continue
|
||||
box = np.array(box)
|
||||
|
||||
box[:, 0] = np.clip(
|
||||
np.round(box[:, 0] / width * dest_width), 0, dest_width)
|
||||
box[:, 1] = np.clip(
|
||||
np.round(box[:, 1] / height * dest_height), 0, dest_height)
|
||||
|
||||
boxes.append(box.astype(np.int16))
|
||||
scores.append(score)
|
||||
|
||||
class_indexes.append(class_index)
|
||||
class_scores.append(class_score)
|
||||
|
||||
if classes is None:
|
||||
return np.array(boxes, dtype=np.int16), scores
|
||||
else:
|
||||
return np.array(boxes, dtype=np.int16), scores, class_indexes, class_scores
|
||||
|
||||
def unclip(self, box):
|
||||
unclip_ratio = self.unclip_ratio
|
||||
poly = Polygon(box)
|
||||
distance = poly.area * unclip_ratio / poly.length
|
||||
offset = pyclipper.PyclipperOffset()
|
||||
offset.AddPath(box, pyclipper.JT_ROUND, pyclipper.ET_CLOSEDPOLYGON)
|
||||
expanded = np.array(offset.Execute(distance))
|
||||
return expanded
|
||||
|
||||
def get_mini_boxes(self, contour):
|
||||
bounding_box = cv2.minAreaRect(contour)
|
||||
points = sorted(list(cv2.boxPoints(bounding_box)), key=lambda x: x[0])
|
||||
|
||||
index_1, index_2, index_3, index_4 = 0, 1, 2, 3
|
||||
if points[1][1] > points[0][1]:
|
||||
index_1 = 0
|
||||
index_4 = 1
|
||||
else:
|
||||
index_1 = 1
|
||||
index_4 = 0
|
||||
if points[3][1] > points[2][1]:
|
||||
index_2 = 2
|
||||
index_3 = 3
|
||||
else:
|
||||
index_2 = 3
|
||||
index_3 = 2
|
||||
|
||||
box = [
|
||||
points[index_1], points[index_2], points[index_3], points[index_4]
|
||||
]
|
||||
return box, min(bounding_box[1])
|
||||
|
||||
def box_score_fast(self, bitmap, _box, classes):
|
||||
'''
|
||||
box_score_fast: use bbox mean score as the mean score
|
||||
'''
|
||||
h, w = bitmap.shape[:2]
|
||||
box = _box.copy()
|
||||
xmin = np.clip(np.floor(box[:, 0].min()).astype(np.int), 0, w - 1)
|
||||
xmax = np.clip(np.ceil(box[:, 0].max()).astype(np.int), 0, w - 1)
|
||||
ymin = np.clip(np.floor(box[:, 1].min()).astype(np.int), 0, h - 1)
|
||||
ymax = np.clip(np.ceil(box[:, 1].max()).astype(np.int), 0, h - 1)
|
||||
|
||||
mask = np.zeros((ymax - ymin + 1, xmax - xmin + 1), dtype=np.uint8)
|
||||
box[:, 0] = box[:, 0] - xmin
|
||||
box[:, 1] = box[:, 1] - ymin
|
||||
cv2.fillPoly(mask, box.reshape(1, -1, 2).astype(np.int32), 1)
|
||||
|
||||
if classes is None:
|
||||
return cv2.mean(bitmap[ymin:ymax + 1, xmin:xmax + 1], mask)[0], None, None
|
||||
else:
|
||||
k = 999
|
||||
class_mask = np.full((ymax - ymin + 1, xmax - xmin + 1), k, dtype=np.int32)
|
||||
|
||||
cv2.fillPoly(class_mask, box.reshape(1, -1, 2).astype(np.int32), 0)
|
||||
classes = classes[ymin:ymax + 1, xmin:xmax + 1]
|
||||
|
||||
new_classes = classes + class_mask
|
||||
a = new_classes.reshape(-1)
|
||||
b = np.where(a >= k)
|
||||
classes = np.delete(a, b[0].tolist())
|
||||
|
||||
class_index = np.argmax(np.bincount(classes))
|
||||
class_score = np.sum(classes == class_index) / len(classes)
|
||||
|
||||
return cv2.mean(bitmap[ymin:ymax + 1, xmin:xmax + 1], mask)[0], class_index, class_score
|
||||
|
||||
def box_score_slow(self, bitmap, contour, classes):
|
||||
"""
|
||||
box_score_slow: use polyon mean score as the mean score
|
||||
"""
|
||||
h, w = bitmap.shape[:2]
|
||||
contour = contour.copy()
|
||||
contour = np.reshape(contour, (-1, 2))
|
||||
|
||||
xmin = np.clip(np.min(contour[:, 0]), 0, w - 1)
|
||||
xmax = np.clip(np.max(contour[:, 0]), 0, w - 1)
|
||||
ymin = np.clip(np.min(contour[:, 1]), 0, h - 1)
|
||||
ymax = np.clip(np.max(contour[:, 1]), 0, h - 1)
|
||||
|
||||
mask = np.zeros((ymax - ymin + 1, xmax - xmin + 1), dtype=np.uint8)
|
||||
|
||||
contour[:, 0] = contour[:, 0] - xmin
|
||||
contour[:, 1] = contour[:, 1] - ymin
|
||||
|
||||
cv2.fillPoly(mask, contour.reshape(1, -1, 2).astype(np.int32), 1)
|
||||
|
||||
if classes is None:
|
||||
return cv2.mean(bitmap[ymin:ymax + 1, xmin:xmax + 1], mask)[0], None, None
|
||||
else:
|
||||
k = 999
|
||||
class_mask = np.full((ymax - ymin + 1, xmax - xmin + 1), k, dtype=np.int32)
|
||||
|
||||
cv2.fillPoly(class_mask, contour.reshape(1, -1, 2).astype(np.int32), 0)
|
||||
classes = classes[ymin:ymax + 1, xmin:xmax + 1]
|
||||
|
||||
new_classes = classes + class_mask
|
||||
a = new_classes.reshape(-1)
|
||||
b = np.where(a >= k)
|
||||
classes = np.delete(a, b[0].tolist())
|
||||
|
||||
class_index = np.argmax(np.bincount(classes))
|
||||
class_score = np.sum(classes == class_index) / len(classes)
|
||||
|
||||
return cv2.mean(bitmap[ymin:ymax + 1, xmin:xmax + 1], mask)[0], class_index, class_score
|
||||
|
||||
def __call__(self, outs_dict, shape_list):
|
||||
pred = outs_dict['maps']
|
||||
if isinstance(pred, paddle.Tensor):
|
||||
pred = pred.numpy()
|
||||
pred = pred[:, 0, :, :]
|
||||
segmentation = pred > self.thresh
|
||||
|
||||
if "classes" in outs_dict:
|
||||
classes = outs_dict['classes']
|
||||
if isinstance(classes, paddle.Tensor):
|
||||
classes = classes.numpy()
|
||||
classes = classes[:, 0, :, :]
|
||||
|
||||
else:
|
||||
classes = None
|
||||
|
||||
boxes_batch = []
|
||||
for batch_index in range(pred.shape[0]):
|
||||
src_h, src_w, ratio_h, ratio_w = shape_list[batch_index]
|
||||
if self.dilation_kernel is not None:
|
||||
mask = cv2.dilate(
|
||||
np.array(segmentation[batch_index]).astype(np.uint8),
|
||||
self.dilation_kernel)
|
||||
else:
|
||||
mask = segmentation[batch_index]
|
||||
|
||||
if classes is None:
|
||||
boxes, scores = self.boxes_from_bitmap(pred[batch_index], mask, None,
|
||||
src_w, src_h)
|
||||
boxes_batch.append({'points': boxes})
|
||||
else:
|
||||
boxes, scores, class_indexes, class_scores = self.boxes_from_bitmap(pred[batch_index], mask,
|
||||
classes[batch_index],
|
||||
src_w, src_h)
|
||||
boxes_batch.append({'points': boxes, "classes": class_indexes, "class_scores": class_scores})
|
||||
|
||||
return boxes_batch
|
||||
```
|
||||
|
||||
### 4.4. 模型启动
|
||||
|
||||
在完成上述步骤后我们就可以正常启动训练
|
||||
|
||||
```
|
||||
!python /home/aistudio/work/PaddleOCR/tools/train.py -c /home/aistudio/work/PaddleOCR/configs/det/det_mv3_db.yml
|
||||
```
|
||||
|
||||
其他命令:
|
||||
```
|
||||
!python /home/aistudio/work/PaddleOCR/tools/eval.py -c /home/aistudio/work/PaddleOCR/configs/det/det_mv3_db.yml
|
||||
!python /home/aistudio/work/PaddleOCR/tools/infer_det.py -c /home/aistudio/work/PaddleOCR/configs/det/det_mv3_db.yml
|
||||
```
|
||||
模型推理
|
||||
```
|
||||
!python /home/aistudio/work/PaddleOCR/tools/infer/predict_det.py --image_dir="/home/aistudio/work/test_img/" --det_model_dir="/home/aistudio/work/PaddleOCR/output/infer"
|
||||
```
|
||||
|
||||
## 5 总结
|
||||
|
||||
1. 分类+检测在一定程度上能够缩短用时,具体的模型选取要根据业务场景恰当选择。
|
||||
2. 数据标注需要多次进行测试调整标注方法,一般进行检测模型微调,需要标注至少上百张。
|
||||
3. 设置合理的batch_size以及resize大小,同时注意lr设置。
|
||||
|
||||
|
||||
## References
|
||||
|
||||
1 https://github.com/PaddlePaddle/PaddleOCR
|
||||
|
||||
2 https://github.com/PaddlePaddle/PaddleClas
|
||||
|
||||
3 https://blog.csdn.net/YY007H/article/details/124491217
|
|
@ -0,0 +1,284 @@
|
|||
# 金融智能核验:扫描合同关键信息抽取
|
||||
|
||||
本案例将使用OCR技术和通用信息抽取技术,实现合同关键信息审核和比对。通过本章的学习,你可以快速掌握:
|
||||
|
||||
1. 使用PaddleOCR提取扫描文本内容
|
||||
2. 使用PaddleNLP抽取自定义信息
|
||||
|
||||
点击进入 [AI Studio 项目](https://aistudio.baidu.com/aistudio/projectdetail/4545772)
|
||||
|
||||
## 1. 项目背景
|
||||
合同审核广泛应用于大中型企业、上市公司、证券、基金公司中,是规避风险的重要任务。
|
||||
- 合同内容对比:合同审核场景中,快速找出不同版本合同修改区域、版本差异;如合同盖章归档场景中有效识别实际签署的纸质合同、电子版合同差异。
|
||||
|
||||
- 合规性检查:法务人员进行合同审核,如合同完备性检查、大小写金额检查、签约主体一致性检查、双方权利和义务对等性分析等。
|
||||
|
||||
- 风险点识别:通过合同审核可识别事实倾向型风险点和数值计算型风险点等,例如交付地点约定不明、合同总价款不一致、重要条款缺失等风险点。
|
||||
|
||||
|
||||
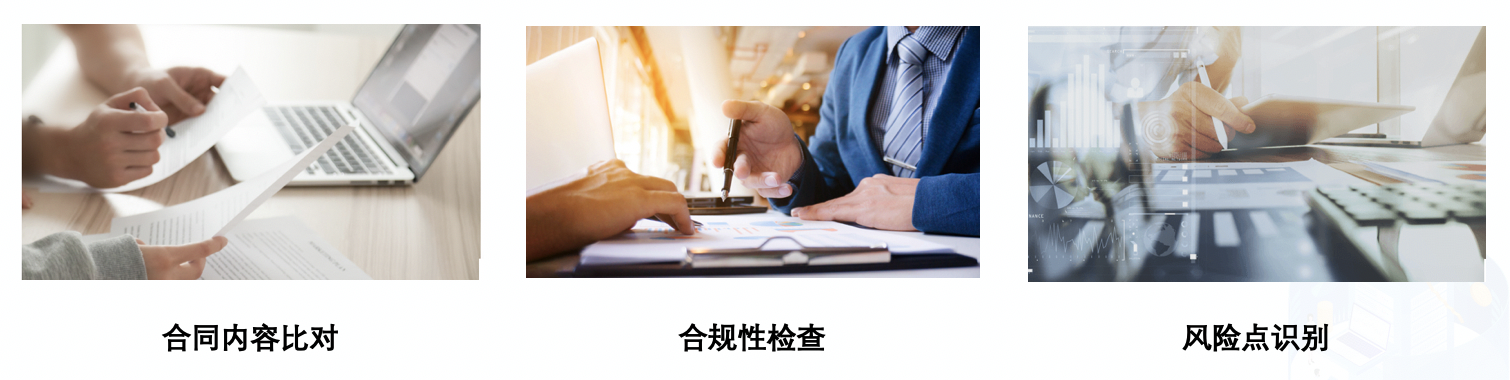
|
||||
|
||||
传统业务中大多使用人工进行纸质版合同审核,存在成本高,工作量大,效率低的问题,且一旦出错将造成巨额损失。
|
||||
|
||||
|
||||
本项目针对以上场景,使用PaddleOCR+PaddleNLP快速提取文本内容,经过少量数据微调即可准确抽取关键信息,**高效完成合同内容对比、合规性检查、风险点识别等任务,提高效率,降低风险**。
|
||||
|
||||
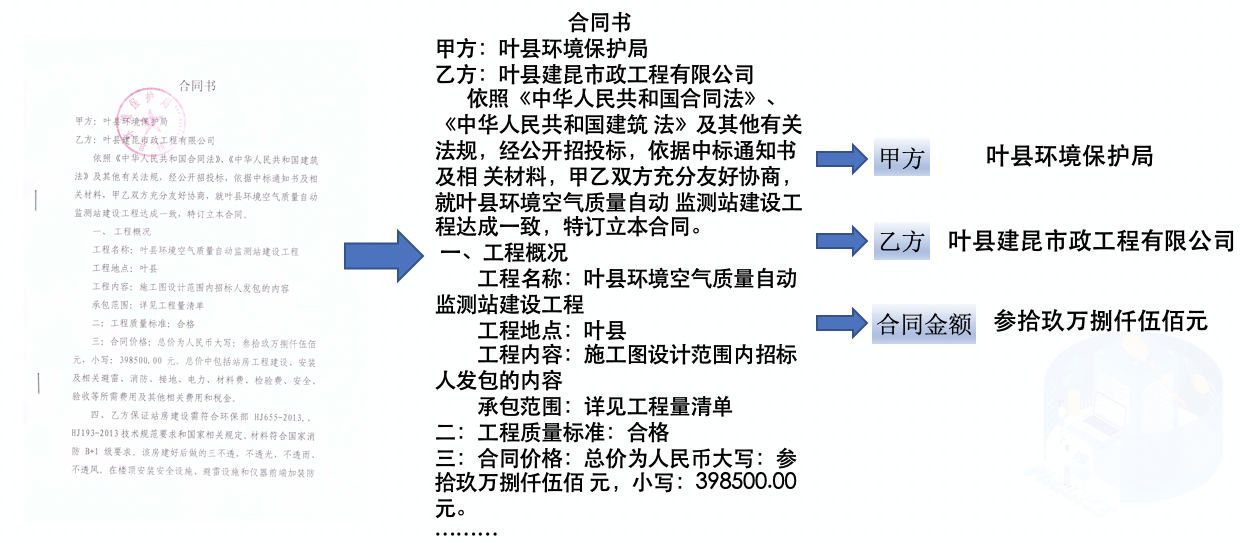
|
||||
|
||||
|
||||
## 2. 解决方案
|
||||
|
||||
### 2.1 扫描合同文本内容提取
|
||||
|
||||
使用PaddleOCR开源的模型可以快速完成扫描文档的文本内容提取,在清晰文档上识别准确率可达到95%+。下面来快速体验一下:
|
||||
|
||||
#### 2.1.1 环境准备
|
||||
|
||||
[PaddleOCR](https://github.com/PaddlePaddle/PaddleOCR)提供了适用于通用场景的高精轻量模型,提供数据预处理-模型推理-后处理全流程,支持pip安装:
|
||||
|
||||
```
|
||||
python -m pip install paddleocr
|
||||
```
|
||||
|
||||
#### 2.1.2 效果测试
|
||||
|
||||
使用一张合同图片作为测试样本,感受ppocrv3模型效果:
|
||||
|
||||
<img src=https://ai-studio-static-online.cdn.bcebos.com/46258d0dc9dc40bab3ea0e70434e4a905646df8a647f4c49921e217de5142def width=300>
|
||||
|
||||
使用中文检测+识别模型提取文本,实例化PaddleOCR类:
|
||||
|
||||
```
|
||||
from paddleocr import PaddleOCR, draw_ocr
|
||||
|
||||
# paddleocr目前支持中英文、英文、法语、德语、韩语、日语等80个语种,可以通过修改lang参数进行切换
|
||||
ocr = PaddleOCR(use_angle_cls=False, lang="ch") # need to run only once to download and load model into memory
|
||||
```
|
||||
|
||||
一行命令启动预测,预测结果包括`检测框`和`文本识别内容`:
|
||||
|
||||
```
|
||||
img_path = "./test_img/hetong2.jpg"
|
||||
result = ocr.ocr(img_path, cls=False)
|
||||
for line in result:
|
||||
print(line)
|
||||
|
||||
# 可视化结果
|
||||
from PIL import Image
|
||||
|
||||
image = Image.open(img_path).convert('RGB')
|
||||
boxes = [line[0] for line in result]
|
||||
txts = [line[1][0] for line in result]
|
||||
scores = [line[1][1] for line in result]
|
||||
im_show = draw_ocr(image, boxes, txts, scores, font_path='./simfang.ttf')
|
||||
im_show = Image.fromarray(im_show)
|
||||
im_show.show()
|
||||
```
|
||||
|
||||
#### 2.1.3 图片预处理
|
||||
|
||||
通过上图可视化结果可以看到,印章部分造成的文本遮盖,影响了文本识别结果,因此可以考虑通道提取,去除图片中的红色印章:
|
||||
|
||||
```
|
||||
import cv2
|
||||
import numpy as np
|
||||
import matplotlib.pyplot as plt
|
||||
|
||||
#读入图像,三通道
|
||||
image=cv2.imread("./test_img/hetong2.jpg",cv2.IMREAD_COLOR) #timg.jpeg
|
||||
|
||||
#获得三个通道
|
||||
Bch,Gch,Rch=cv2.split(image)
|
||||
|
||||
#保存三通道图片
|
||||
cv2.imwrite('blue_channel.jpg',Bch)
|
||||
cv2.imwrite('green_channel.jpg',Gch)
|
||||
cv2.imwrite('red_channel.jpg',Rch)
|
||||
```
|
||||
#### 2.1.4 合同文本信息提取
|
||||
|
||||
经过2.1.3的预处理后,合同照片的红色通道被分离,获得了一张相对更干净的图片,此时可以再次使用ppocr模型提取文本内容:
|
||||
|
||||
```
|
||||
import numpy as np
|
||||
import cv2
|
||||
|
||||
|
||||
img_path = './red_channel.jpg'
|
||||
result = ocr.ocr(img_path, cls=False)
|
||||
|
||||
# 可视化结果
|
||||
from PIL import Image
|
||||
|
||||
image = Image.open(img_path).convert('RGB')
|
||||
boxes = [line[0] for line in result]
|
||||
txts = [line[1][0] for line in result]
|
||||
scores = [line[1][1] for line in result]
|
||||
im_show = draw_ocr(image, boxes, txts, scores, font_path='./simfang.ttf')
|
||||
im_show = Image.fromarray(im_show)
|
||||
vis = np.array(im_show)
|
||||
im_show.show()
|
||||
```
|
||||
|
||||
忽略检测框内容,提取完整的合同文本:
|
||||
|
||||
```
|
||||
txts = [line[1][0] for line in result]
|
||||
all_context = "\n".join(txts)
|
||||
print(all_context)
|
||||
```
|
||||
|
||||
通过以上环节就完成了扫描合同关键信息抽取的第一步:文本内容提取,接下来可以基于识别出的文本内容抽取关键信息
|
||||
|
||||
### 2.2 合同关键信息抽取
|
||||
|
||||
#### 2.2.1 环境准备
|
||||
|
||||
安装PaddleNLP
|
||||
|
||||
|
||||
```
|
||||
pip install --upgrade pip
|
||||
pip install --upgrade paddlenlp
|
||||
```
|
||||
|
||||
#### 2.2.2 合同关键信息抽取
|
||||
|
||||
PaddleNLP 使用 Taskflow 统一管理多场景任务的预测功能,其中`information_extraction` 通过大量的有标签样本进行训练,在通用的场景中一般可以直接使用,只需更换关键字即可。例如在合同信息抽取中,我们重新定义抽取关键字:
|
||||
|
||||
甲方、乙方、币种、金额、付款方式
|
||||
|
||||
|
||||
将使用OCR提取好的文本作为输入,使用三行命令可以对上文中提取到的合同文本进行关键信息抽取:
|
||||
|
||||
```
|
||||
from paddlenlp import Taskflow
|
||||
schema = ["甲方","乙方","总价"]
|
||||
ie = Taskflow('information_extraction', schema=schema)
|
||||
ie.set_schema(schema)
|
||||
ie(all_context)
|
||||
```
|
||||
|
||||
可以看到UIE模型可以准确的提取出关键信息,用于后续的信息比对或审核。
|
||||
|
||||
## 3.效果优化
|
||||
|
||||
### 3.1 文本识别后处理调优
|
||||
|
||||
实际图片采集过程中,可能出现部分图片弯曲等问题,导致使用默认参数识别文本时存在漏检,影响关键信息获取。
|
||||
|
||||
例如下图:
|
||||
|
||||
<img src="https://ai-studio-static-online.cdn.bcebos.com/fe350481be0241c58736d487d1bf06c2e65911bf01254a79944be629c4c10091" height="300" width="300">
|
||||
|
||||
|
||||
直接进行预测:
|
||||
|
||||
```
|
||||
img_path = "./test_img/hetong3.jpg"
|
||||
# 预测结果
|
||||
result = ocr.ocr(img_path, cls=False)
|
||||
# 可视化结果
|
||||
from PIL import Image
|
||||
|
||||
image = Image.open(img_path).convert('RGB')
|
||||
boxes = [line[0] for line in result]
|
||||
txts = [line[1][0] for line in result]
|
||||
scores = [line[1][1] for line in result]
|
||||
im_show = draw_ocr(image, boxes, txts, scores, font_path='./simfang.ttf')
|
||||
im_show = Image.fromarray(im_show)
|
||||
im_show.show()
|
||||
```
|
||||
|
||||
可视化结果可以看到,弯曲图片存在漏检,一般来说可以通过调整后处理参数解决,无需重新训练模型。漏检问题往往是因为检测模型获得的分割图太小,生成框的得分过低被过滤掉了,通常有两种方式调整参数:
|
||||
- 开启`use_dilatiion=True` 膨胀分割区域
|
||||
- 调小`det_db_box_thresh`阈值
|
||||
|
||||
```
|
||||
# 重新实例化 PaddleOCR
|
||||
ocr = PaddleOCR(use_angle_cls=False, lang="ch", det_db_box_thresh=0.3, use_dilation=True)
|
||||
|
||||
# 预测并可视化
|
||||
img_path = "./test_img/hetong3.jpg"
|
||||
# 预测结果
|
||||
result = ocr.ocr(img_path, cls=False)
|
||||
# 可视化结果
|
||||
image = Image.open(img_path).convert('RGB')
|
||||
boxes = [line[0] for line in result]
|
||||
txts = [line[1][0] for line in result]
|
||||
scores = [line[1][1] for line in result]
|
||||
im_show = draw_ocr(image, boxes, txts, scores, font_path='./simfang.ttf')
|
||||
im_show = Image.fromarray(im_show)
|
||||
im_show.show()
|
||||
```
|
||||
|
||||
可以看到漏检问题被很好的解决,提取完整的文本内容:
|
||||
|
||||
```
|
||||
txts = [line[1][0] for line in result]
|
||||
context = "\n".join(txts)
|
||||
print(context)
|
||||
```
|
||||
|
||||
### 3.2 关键信息提取调优
|
||||
|
||||
UIE通过大量有标签样本进行训练,得到了一个开箱即用的高精模型。 然而针对不同场景,可能会出现部分实体无法被抽取的情况。通常来说有以下几个方法进行效果调优:
|
||||
|
||||
|
||||
- 修改 schema
|
||||
- 添加正则方法
|
||||
- 标注小样本微调模型
|
||||
|
||||
**修改schema**
|
||||
|
||||
Prompt和原文描述越像,抽取效果越好,例如
|
||||
```
|
||||
三:合同价格:总价为人民币大写:参拾玖万捌仟伍佰
|
||||
元,小写:398500.00元。总价中包括站房工程建设、安装
|
||||
及相关避雷、消防、接地、电力、材料费、检验费、安全、
|
||||
验收等所需费用及其他相关费用和税金。
|
||||
```
|
||||
schema = ["总金额"] 时无法准确抽取,与原文描述差异较大。 修改 schema = ["总价"] 再次尝试:
|
||||
|
||||
```
|
||||
from paddlenlp import Taskflow
|
||||
# schema = ["总金额"]
|
||||
schema = ["总价"]
|
||||
ie = Taskflow('information_extraction', schema=schema)
|
||||
ie.set_schema(schema)
|
||||
ie(all_context)
|
||||
```
|
||||
|
||||
|
||||
**模型微调**
|
||||
|
||||
UIE的建模方式主要是通过 `Prompt` 方式来建模, `Prompt` 在小样本上进行微调效果非常有效。详细的数据标注+模型微调步骤可以参考项目:
|
||||
|
||||
[PaddleNLP信息抽取技术重磅升级!](https://aistudio.baidu.com/aistudio/projectdetail/3914778?channelType=0&channel=0)
|
||||
|
||||
[工单信息抽取](https://aistudio.baidu.com/aistudio/projectdetail/3914778?contributionType=1)
|
||||
|
||||
[快递单信息抽取](https://aistudio.baidu.com/aistudio/projectdetail/4038499?contributionType=1)
|
||||
|
||||
|
||||
## 总结
|
||||
|
||||
扫描合同的关键信息提取可以使用 PaddleOCR + PaddleNLP 组合实现,两个工具均有以下优势:
|
||||
|
||||
* 使用简单:whl包一键安装,3行命令调用
|
||||
* 效果领先:优秀的模型效果可覆盖几乎全部的应用场景
|
||||
* 调优成本低:OCR模型可通过后处理参数的调整适配略有偏差的扫描文本, UIE模型可以通过极少的标注样本微调,成本很低。
|
||||
|
||||
## 作业
|
||||
|
||||
尝试自己解析出 `test_img/homework.png` 扫描合同中的 [甲方、乙方] 关键词:
|
||||
|
||||
|
||||
|
||||
<img src=https://ai-studio-static-online.cdn.bcebos.com/50a49a3c9f8348bfa04e8c8b97d3cce0d0dd6b14040f43939268d120688ef7ca width=300 hight=400>
|
||||
|
||||
|
||||
|
||||
更多场景下的垂类模型获取,请扫下图二维码填写问卷,加入PaddleOCR官方交流群获取模型下载链接、《动手学OCR》电子书等全套OCR学习资料🎁
|
||||
|
||||
<img src=https://ai-studio-static-online.cdn.bcebos.com/606538b59ea845cb99943b1dec6efe724e78f75c1e9c49228c7bf7da9f8837f5 width=300 hight=300>
|
|
@ -68,6 +68,7 @@ Train:
|
|||
- VQAReTokenRelation:
|
||||
- VQAReTokenChunk:
|
||||
max_seq_len: *max_seq_len
|
||||
- TensorizeEntitiesRelations:
|
||||
- Resize:
|
||||
size: [224,224]
|
||||
- NormalizeImage:
|
||||
|
@ -83,7 +84,6 @@ Train:
|
|||
drop_last: False
|
||||
batch_size_per_card: 2
|
||||
num_workers: 8
|
||||
collate_fn: ListCollator
|
||||
|
||||
Eval:
|
||||
dataset:
|
||||
|
@ -105,6 +105,7 @@ Eval:
|
|||
- VQAReTokenRelation:
|
||||
- VQAReTokenChunk:
|
||||
max_seq_len: *max_seq_len
|
||||
- TensorizeEntitiesRelations:
|
||||
- Resize:
|
||||
size: [224,224]
|
||||
- NormalizeImage:
|
||||
|
@ -120,4 +121,3 @@ Eval:
|
|||
drop_last: False
|
||||
batch_size_per_card: 8
|
||||
num_workers: 8
|
||||
collate_fn: ListCollator
|
||||
|
|
|
@ -73,6 +73,7 @@ Train:
|
|||
- VQAReTokenRelation:
|
||||
- VQAReTokenChunk:
|
||||
max_seq_len: *max_seq_len
|
||||
- TensorizeEntitiesRelations:
|
||||
- Resize:
|
||||
size: [224,224]
|
||||
- NormalizeImage:
|
||||
|
@ -82,13 +83,12 @@ Train:
|
|||
order: 'hwc'
|
||||
- ToCHWImage:
|
||||
- KeepKeys:
|
||||
keep_keys: [ 'input_ids', 'bbox','attention_mask', 'token_type_ids', 'image', 'entities', 'relations'] # dataloader will return list in this order
|
||||
keep_keys: [ 'input_ids', 'bbox','attention_mask', 'token_type_ids', 'entities', 'relations'] # dataloader will return list in this order
|
||||
loader:
|
||||
shuffle: True
|
||||
drop_last: False
|
||||
batch_size_per_card: 2
|
||||
num_workers: 4
|
||||
collate_fn: ListCollator
|
||||
|
||||
Eval:
|
||||
dataset:
|
||||
|
@ -112,6 +112,7 @@ Eval:
|
|||
- VQAReTokenRelation:
|
||||
- VQAReTokenChunk:
|
||||
max_seq_len: *max_seq_len
|
||||
- TensorizeEntitiesRelations:
|
||||
- Resize:
|
||||
size: [224,224]
|
||||
- NormalizeImage:
|
||||
|
@ -121,11 +122,9 @@ Eval:
|
|||
order: 'hwc'
|
||||
- ToCHWImage:
|
||||
- KeepKeys:
|
||||
keep_keys: [ 'input_ids', 'bbox', 'attention_mask', 'token_type_ids', 'image', 'entities', 'relations'] # dataloader will return list in this order
|
||||
keep_keys: [ 'input_ids', 'bbox', 'attention_mask', 'token_type_ids', 'entities', 'relations'] # dataloader will return list in this order
|
||||
loader:
|
||||
shuffle: False
|
||||
drop_last: False
|
||||
batch_size_per_card: 8
|
||||
num_workers: 8
|
||||
collate_fn: ListCollator
|
||||
|
||||
|
|
|
@ -57,14 +57,16 @@ Loss:
|
|||
mode: "l2"
|
||||
model_name_pairs:
|
||||
- ["Student", "Teacher"]
|
||||
key: hidden_states_5
|
||||
key: hidden_states
|
||||
index: 5
|
||||
name: "loss_5"
|
||||
- DistillationVQADistanceLoss:
|
||||
weight: 0.5
|
||||
mode: "l2"
|
||||
model_name_pairs:
|
||||
- ["Student", "Teacher"]
|
||||
key: hidden_states_8
|
||||
key: hidden_states
|
||||
index: 8
|
||||
name: "loss_8"
|
||||
|
||||
|
||||
|
@ -116,6 +118,7 @@ Train:
|
|||
- VQAReTokenRelation:
|
||||
- VQAReTokenChunk:
|
||||
max_seq_len: *max_seq_len
|
||||
- TensorizeEntitiesRelations:
|
||||
- Resize:
|
||||
size: [224,224]
|
||||
- NormalizeImage:
|
||||
|
@ -125,13 +128,12 @@ Train:
|
|||
order: 'hwc'
|
||||
- ToCHWImage:
|
||||
- KeepKeys:
|
||||
keep_keys: [ 'input_ids', 'bbox','attention_mask', 'token_type_ids', 'image', 'entities', 'relations'] # dataloader will return list in this order
|
||||
keep_keys: [ 'input_ids', 'bbox','attention_mask', 'token_type_ids', 'entities', 'relations'] # dataloader will return list in this order
|
||||
loader:
|
||||
shuffle: True
|
||||
drop_last: False
|
||||
batch_size_per_card: 2
|
||||
num_workers: 4
|
||||
collate_fn: ListCollator
|
||||
|
||||
Eval:
|
||||
dataset:
|
||||
|
@ -155,6 +157,7 @@ Eval:
|
|||
- VQAReTokenRelation:
|
||||
- VQAReTokenChunk:
|
||||
max_seq_len: *max_seq_len
|
||||
- TensorizeEntitiesRelations:
|
||||
- Resize:
|
||||
size: [224,224]
|
||||
- NormalizeImage:
|
||||
|
@ -164,12 +167,11 @@ Eval:
|
|||
order: 'hwc'
|
||||
- ToCHWImage:
|
||||
- KeepKeys:
|
||||
keep_keys: [ 'input_ids', 'bbox', 'attention_mask', 'token_type_ids', 'image', 'entities', 'relations'] # dataloader will return list in this order
|
||||
keep_keys: [ 'input_ids', 'bbox', 'attention_mask', 'token_type_ids', 'entities', 'relations'] # dataloader will return list in this order
|
||||
loader:
|
||||
shuffle: False
|
||||
drop_last: False
|
||||
batch_size_per_card: 8
|
||||
num_workers: 8
|
||||
collate_fn: ListCollator
|
||||
|
||||
|
||||
|
|
|
@ -70,14 +70,16 @@ Loss:
|
|||
mode: "l2"
|
||||
model_name_pairs:
|
||||
- ["Student", "Teacher"]
|
||||
key: hidden_states_5
|
||||
key: hidden_states
|
||||
index: 5
|
||||
name: "loss_5"
|
||||
- DistillationVQADistanceLoss:
|
||||
weight: 0.5
|
||||
mode: "l2"
|
||||
model_name_pairs:
|
||||
- ["Student", "Teacher"]
|
||||
key: hidden_states_8
|
||||
key: hidden_states
|
||||
index: 8
|
||||
name: "loss_8"
|
||||
|
||||
|
||||
|
|
|
@ -49,6 +49,11 @@ DECLARE_int32(rec_batch_num);
|
|||
DECLARE_string(rec_char_dict_path);
|
||||
DECLARE_int32(rec_img_h);
|
||||
DECLARE_int32(rec_img_w);
|
||||
// layout model related
|
||||
DECLARE_string(layout_model_dir);
|
||||
DECLARE_string(layout_dict_path);
|
||||
DECLARE_double(layout_score_threshold);
|
||||
DECLARE_double(layout_nms_threshold);
|
||||
// structure model related
|
||||
DECLARE_string(table_model_dir);
|
||||
DECLARE_int32(table_max_len);
|
||||
|
@ -59,4 +64,5 @@ DECLARE_bool(merge_no_span_structure);
|
|||
DECLARE_bool(det);
|
||||
DECLARE_bool(rec);
|
||||
DECLARE_bool(cls);
|
||||
DECLARE_bool(table);
|
||||
DECLARE_bool(table);
|
||||
DECLARE_bool(layout);
|
|
@ -14,26 +14,12 @@
|
|||
|
||||
#pragma once
|
||||
|
||||
#include "opencv2/core.hpp"
|
||||
#include "opencv2/imgcodecs.hpp"
|
||||
#include "opencv2/imgproc.hpp"
|
||||
#include "paddle_api.h"
|
||||
#include "paddle_inference_api.h"
|
||||
#include <chrono>
|
||||
#include <iomanip>
|
||||
#include <iostream>
|
||||
#include <ostream>
|
||||
#include <vector>
|
||||
|
||||
#include <cstring>
|
||||
#include <fstream>
|
||||
#include <numeric>
|
||||
|
||||
#include <include/preprocess_op.h>
|
||||
#include <include/utility.h>
|
||||
|
||||
using namespace paddle_infer;
|
||||
|
||||
namespace PaddleOCR {
|
||||
|
||||
class Classifier {
|
||||
|
@ -66,7 +52,7 @@ public:
|
|||
std::vector<float> &cls_scores, std::vector<double> ×);
|
||||
|
||||
private:
|
||||
std::shared_ptr<Predictor> predictor_;
|
||||
std::shared_ptr<paddle_infer::Predictor> predictor_;
|
||||
|
||||
bool use_gpu_ = false;
|
||||
int gpu_id_ = 0;
|
||||
|
|
|
@ -14,26 +14,12 @@
|
|||
|
||||
#pragma once
|
||||
|
||||
#include "opencv2/core.hpp"
|
||||
#include "opencv2/imgcodecs.hpp"
|
||||
#include "opencv2/imgproc.hpp"
|
||||
#include "paddle_api.h"
|
||||
#include "paddle_inference_api.h"
|
||||
#include <chrono>
|
||||
#include <iomanip>
|
||||
#include <iostream>
|
||||
#include <ostream>
|
||||
#include <vector>
|
||||
|
||||
#include <cstring>
|
||||
#include <fstream>
|
||||
#include <numeric>
|
||||
|
||||
#include <include/postprocess_op.h>
|
||||
#include <include/preprocess_op.h>
|
||||
|
||||
using namespace paddle_infer;
|
||||
|
||||
namespace PaddleOCR {
|
||||
|
||||
class DBDetector {
|
||||
|
@ -41,7 +27,7 @@ public:
|
|||
explicit DBDetector(const std::string &model_dir, const bool &use_gpu,
|
||||
const int &gpu_id, const int &gpu_mem,
|
||||
const int &cpu_math_library_num_threads,
|
||||
const bool &use_mkldnn, const string &limit_type,
|
||||
const bool &use_mkldnn, const std::string &limit_type,
|
||||
const int &limit_side_len, const double &det_db_thresh,
|
||||
const double &det_db_box_thresh,
|
||||
const double &det_db_unclip_ratio,
|
||||
|
@ -77,7 +63,7 @@ public:
|
|||
std::vector<double> ×);
|
||||
|
||||
private:
|
||||
std::shared_ptr<Predictor> predictor_;
|
||||
std::shared_ptr<paddle_infer::Predictor> predictor_;
|
||||
|
||||
bool use_gpu_ = false;
|
||||
int gpu_id_ = 0;
|
||||
|
@ -85,7 +71,7 @@ private:
|
|||
int cpu_math_library_num_threads_ = 4;
|
||||
bool use_mkldnn_ = false;
|
||||
|
||||
string limit_type_ = "max";
|
||||
std::string limit_type_ = "max";
|
||||
int limit_side_len_ = 960;
|
||||
|
||||
double det_db_thresh_ = 0.3;
|
||||
|
|
|
@ -14,27 +14,12 @@
|
|||
|
||||
#pragma once
|
||||
|
||||
#include "opencv2/core.hpp"
|
||||
#include "opencv2/imgcodecs.hpp"
|
||||
#include "opencv2/imgproc.hpp"
|
||||
#include "paddle_api.h"
|
||||
#include "paddle_inference_api.h"
|
||||
#include <chrono>
|
||||
#include <iomanip>
|
||||
#include <iostream>
|
||||
#include <ostream>
|
||||
#include <vector>
|
||||
|
||||
#include <cstring>
|
||||
#include <fstream>
|
||||
#include <numeric>
|
||||
|
||||
#include <include/ocr_cls.h>
|
||||
#include <include/preprocess_op.h>
|
||||
#include <include/utility.h>
|
||||
|
||||
using namespace paddle_infer;
|
||||
|
||||
namespace PaddleOCR {
|
||||
|
||||
class CRNNRecognizer {
|
||||
|
@ -42,7 +27,7 @@ public:
|
|||
explicit CRNNRecognizer(const std::string &model_dir, const bool &use_gpu,
|
||||
const int &gpu_id, const int &gpu_mem,
|
||||
const int &cpu_math_library_num_threads,
|
||||
const bool &use_mkldnn, const string &label_path,
|
||||
const bool &use_mkldnn, const std::string &label_path,
|
||||
const bool &use_tensorrt,
|
||||
const std::string &precision,
|
||||
const int &rec_batch_num, const int &rec_img_h,
|
||||
|
@ -75,7 +60,7 @@ public:
|
|||
std::vector<float> &rec_text_scores, std::vector<double> ×);
|
||||
|
||||
private:
|
||||
std::shared_ptr<Predictor> predictor_;
|
||||
std::shared_ptr<paddle_infer::Predictor> predictor_;
|
||||
|
||||
bool use_gpu_ = false;
|
||||
int gpu_id_ = 0;
|
||||
|
|
|
@ -14,28 +14,9 @@
|
|||
|
||||
#pragma once
|
||||
|
||||
#include "opencv2/core.hpp"
|
||||
#include "opencv2/imgcodecs.hpp"
|
||||
#include "opencv2/imgproc.hpp"
|
||||
#include "paddle_api.h"
|
||||
#include "paddle_inference_api.h"
|
||||
#include <chrono>
|
||||
#include <iomanip>
|
||||
#include <iostream>
|
||||
#include <ostream>
|
||||
#include <vector>
|
||||
|
||||
#include <cstring>
|
||||
#include <fstream>
|
||||
#include <numeric>
|
||||
|
||||
#include <include/ocr_cls.h>
|
||||
#include <include/ocr_det.h>
|
||||
#include <include/ocr_rec.h>
|
||||
#include <include/preprocess_op.h>
|
||||
#include <include/utility.h>
|
||||
|
||||
using namespace paddle_infer;
|
||||
|
||||
namespace PaddleOCR {
|
||||
|
||||
|
@ -43,21 +24,27 @@ class PPOCR {
|
|||
public:
|
||||
explicit PPOCR();
|
||||
~PPOCR();
|
||||
std::vector<std::vector<OCRPredictResult>>
|
||||
ocr(std::vector<cv::String> cv_all_img_names, bool det = true,
|
||||
bool rec = true, bool cls = true);
|
||||
|
||||
std::vector<std::vector<OCRPredictResult>> ocr(std::vector<cv::Mat> img_list,
|
||||
bool det = true,
|
||||
bool rec = true,
|
||||
bool cls = true);
|
||||
std::vector<OCRPredictResult> ocr(cv::Mat img, bool det = true,
|
||||
bool rec = true, bool cls = true);
|
||||
|
||||
void reset_timer();
|
||||
void benchmark_log(int img_num);
|
||||
|
||||
protected:
|
||||
void det(cv::Mat img, std::vector<OCRPredictResult> &ocr_results,
|
||||
std::vector<double> ×);
|
||||
std::vector<double> time_info_det = {0, 0, 0};
|
||||
std::vector<double> time_info_rec = {0, 0, 0};
|
||||
std::vector<double> time_info_cls = {0, 0, 0};
|
||||
|
||||
void det(cv::Mat img, std::vector<OCRPredictResult> &ocr_results);
|
||||
void rec(std::vector<cv::Mat> img_list,
|
||||
std::vector<OCRPredictResult> &ocr_results,
|
||||
std::vector<double> ×);
|
||||
std::vector<OCRPredictResult> &ocr_results);
|
||||
void cls(std::vector<cv::Mat> img_list,
|
||||
std::vector<OCRPredictResult> &ocr_results,
|
||||
std::vector<double> ×);
|
||||
void log(std::vector<double> &det_times, std::vector<double> &rec_times,
|
||||
std::vector<double> &cls_times, int img_num);
|
||||
std::vector<OCRPredictResult> &ocr_results);
|
||||
|
||||
private:
|
||||
DBDetector *detector_ = nullptr;
|
||||
|
|
|
@ -14,27 +14,9 @@
|
|||
|
||||
#pragma once
|
||||
|
||||
#include "opencv2/core.hpp"
|
||||
#include "opencv2/imgcodecs.hpp"
|
||||
#include "opencv2/imgproc.hpp"
|
||||
#include "paddle_api.h"
|
||||
#include "paddle_inference_api.h"
|
||||
#include <chrono>
|
||||
#include <iomanip>
|
||||
#include <iostream>
|
||||
#include <ostream>
|
||||
#include <vector>
|
||||
|
||||
#include <cstring>
|
||||
#include <fstream>
|
||||
#include <numeric>
|
||||
|
||||
#include <include/paddleocr.h>
|
||||
#include <include/preprocess_op.h>
|
||||
#include <include/structure_layout.h>
|
||||
#include <include/structure_table.h>
|
||||
#include <include/utility.h>
|
||||
|
||||
using namespace paddle_infer;
|
||||
|
||||
namespace PaddleOCR {
|
||||
|
||||
|
@ -42,23 +24,31 @@ class PaddleStructure : public PPOCR {
|
|||
public:
|
||||
explicit PaddleStructure();
|
||||
~PaddleStructure();
|
||||
std::vector<std::vector<StructurePredictResult>>
|
||||
structure(std::vector<cv::String> cv_all_img_names, bool layout = false,
|
||||
bool table = true);
|
||||
|
||||
std::vector<StructurePredictResult> structure(cv::Mat img,
|
||||
bool layout = false,
|
||||
bool table = true,
|
||||
bool ocr = false);
|
||||
|
||||
void reset_timer();
|
||||
void benchmark_log(int img_num);
|
||||
|
||||
private:
|
||||
StructureTableRecognizer *recognizer_ = nullptr;
|
||||
std::vector<double> time_info_table = {0, 0, 0};
|
||||
std::vector<double> time_info_layout = {0, 0, 0};
|
||||
|
||||
StructureTableRecognizer *table_model_ = nullptr;
|
||||
StructureLayoutRecognizer *layout_model_ = nullptr;
|
||||
|
||||
void layout(cv::Mat img,
|
||||
std::vector<StructurePredictResult> &structure_result);
|
||||
|
||||
void table(cv::Mat img, StructurePredictResult &structure_result);
|
||||
|
||||
void table(cv::Mat img, StructurePredictResult &structure_result,
|
||||
std::vector<double> &time_info_table,
|
||||
std::vector<double> &time_info_det,
|
||||
std::vector<double> &time_info_rec,
|
||||
std::vector<double> &time_info_cls);
|
||||
std::string rebuild_table(std::vector<std::string> rec_html_tags,
|
||||
std::vector<std::vector<int>> rec_boxes,
|
||||
std::vector<OCRPredictResult> &ocr_result);
|
||||
|
||||
float iou(std::vector<int> &box1, std::vector<int> &box2);
|
||||
float dis(std::vector<int> &box1, std::vector<int> &box2);
|
||||
|
||||
static bool comparison_dis(const std::vector<float> &dis1,
|
||||
|
|
|
@ -14,24 +14,9 @@
|
|||
|
||||
#pragma once
|
||||
|
||||
#include "opencv2/core.hpp"
|
||||
#include "opencv2/imgcodecs.hpp"
|
||||
#include "opencv2/imgproc.hpp"
|
||||
#include <chrono>
|
||||
#include <iomanip>
|
||||
#include <iostream>
|
||||
#include <ostream>
|
||||
#include <vector>
|
||||
|
||||
#include <cstring>
|
||||
#include <fstream>
|
||||
#include <numeric>
|
||||
|
||||
#include "include/clipper.h"
|
||||
#include "include/utility.h"
|
||||
|
||||
using namespace std;
|
||||
|
||||
namespace PaddleOCR {
|
||||
|
||||
class DBPostProcessor {
|
||||
|
@ -106,4 +91,27 @@ private:
|
|||
std::string beg = "sos";
|
||||
};
|
||||
|
||||
class PicodetPostProcessor {
|
||||
public:
|
||||
void init(std::string label_path, const double score_threshold = 0.4,
|
||||
const double nms_threshold = 0.5,
|
||||
const std::vector<int> &fpn_stride = {8, 16, 32, 64});
|
||||
void Run(std::vector<StructurePredictResult> &results,
|
||||
std::vector<std::vector<float>> outs, std::vector<int> ori_shape,
|
||||
std::vector<int> resize_shape, int eg_max);
|
||||
std::vector<int> fpn_stride_ = {8, 16, 32, 64};
|
||||
|
||||
private:
|
||||
StructurePredictResult disPred2Bbox(std::vector<float> bbox_pred, int label,
|
||||
float score, int x, int y, int stride,
|
||||
std::vector<int> im_shape, int reg_max);
|
||||
void nms(std::vector<StructurePredictResult> &input_boxes,
|
||||
float nms_threshold);
|
||||
|
||||
std::vector<std::string> label_list_;
|
||||
double score_threshold_ = 0.4;
|
||||
double nms_threshold_ = 0.5;
|
||||
int num_class_ = 5;
|
||||
};
|
||||
|
||||
} // namespace PaddleOCR
|
||||
|
|
|
@ -14,21 +14,12 @@
|
|||
|
||||
#pragma once
|
||||
|
||||
#include <iostream>
|
||||
#include <vector>
|
||||
|
||||
#include "opencv2/core.hpp"
|
||||
#include "opencv2/imgcodecs.hpp"
|
||||
#include "opencv2/imgproc.hpp"
|
||||
#include <chrono>
|
||||
#include <iomanip>
|
||||
#include <iostream>
|
||||
#include <ostream>
|
||||
#include <vector>
|
||||
|
||||
#include <cstring>
|
||||
#include <fstream>
|
||||
#include <numeric>
|
||||
|
||||
using namespace std;
|
||||
using namespace paddle;
|
||||
|
||||
namespace PaddleOCR {
|
||||
|
||||
|
@ -51,9 +42,9 @@ public:
|
|||
|
||||
class ResizeImgType0 {
|
||||
public:
|
||||
virtual void Run(const cv::Mat &img, cv::Mat &resize_img, string limit_type,
|
||||
int limit_side_len, float &ratio_h, float &ratio_w,
|
||||
bool use_tensorrt);
|
||||
virtual void Run(const cv::Mat &img, cv::Mat &resize_img,
|
||||
std::string limit_type, int limit_side_len, float &ratio_h,
|
||||
float &ratio_w, bool use_tensorrt);
|
||||
};
|
||||
|
||||
class CrnnResizeImg {
|
||||
|
@ -82,4 +73,10 @@ public:
|
|||
const int max_len = 488);
|
||||
};
|
||||
|
||||
class Resize {
|
||||
public:
|
||||
virtual void Run(const cv::Mat &img, cv::Mat &resize_img, const int h,
|
||||
const int w);
|
||||
};
|
||||
|
||||
} // namespace PaddleOCR
|
|
@ -0,0 +1,78 @@
|
|||
// Copyright (c) 2020 PaddlePaddle Authors. All Rights Reserved.
|
||||
//
|
||||
// Licensed under the Apache License, Version 2.0 (the "License");
|
||||
// you may not use this file except in compliance with the License.
|
||||
// You may obtain a copy of the License at
|
||||
//
|
||||
// http://www.apache.org/licenses/LICENSE-2.0
|
||||
//
|
||||
// Unless required by applicable law or agreed to in writing, software
|
||||
// distributed under the License is distributed on an "AS IS" BASIS,
|
||||
// WITHOUT WARRANTIES OR CONDITIONS OF ANY KIND, either express or implied.
|
||||
// See the License for the specific language governing permissions and
|
||||
// limitations under the License.
|
||||
|
||||
#pragma once
|
||||
|
||||
#include "paddle_api.h"
|
||||
#include "paddle_inference_api.h"
|
||||
|
||||
#include <include/postprocess_op.h>
|
||||
#include <include/preprocess_op.h>
|
||||
|
||||
namespace PaddleOCR {
|
||||
|
||||
class StructureLayoutRecognizer {
|
||||
public:
|
||||
explicit StructureLayoutRecognizer(
|
||||
const std::string &model_dir, const bool &use_gpu, const int &gpu_id,
|
||||
const int &gpu_mem, const int &cpu_math_library_num_threads,
|
||||
const bool &use_mkldnn, const std::string &label_path,
|
||||
const bool &use_tensorrt, const std::string &precision,
|
||||
const double &layout_score_threshold,
|
||||
const double &layout_nms_threshold) {
|
||||
this->use_gpu_ = use_gpu;
|
||||
this->gpu_id_ = gpu_id;
|
||||
this->gpu_mem_ = gpu_mem;
|
||||
this->cpu_math_library_num_threads_ = cpu_math_library_num_threads;
|
||||
this->use_mkldnn_ = use_mkldnn;
|
||||
this->use_tensorrt_ = use_tensorrt;
|
||||
this->precision_ = precision;
|
||||
|
||||
this->post_processor_.init(label_path, layout_score_threshold,
|
||||
layout_nms_threshold);
|
||||
LoadModel(model_dir);
|
||||
}
|
||||
|
||||
// Load Paddle inference model
|
||||
void LoadModel(const std::string &model_dir);
|
||||
|
||||
void Run(cv::Mat img, std::vector<StructurePredictResult> &result,
|
||||
std::vector<double> ×);
|
||||
|
||||
private:
|
||||
std::shared_ptr<paddle_infer::Predictor> predictor_;
|
||||
|
||||
bool use_gpu_ = false;
|
||||
int gpu_id_ = 0;
|
||||
int gpu_mem_ = 4000;
|
||||
int cpu_math_library_num_threads_ = 4;
|
||||
bool use_mkldnn_ = false;
|
||||
|
||||
std::vector<float> mean_ = {0.485f, 0.456f, 0.406f};
|
||||
std::vector<float> scale_ = {1 / 0.229f, 1 / 0.224f, 1 / 0.225f};
|
||||
bool is_scale_ = true;
|
||||
|
||||
bool use_tensorrt_ = false;
|
||||
std::string precision_ = "fp32";
|
||||
|
||||
// pre-process
|
||||
Resize resize_op_;
|
||||
Normalize normalize_op_;
|
||||
Permute permute_op_;
|
||||
|
||||
// post-process
|
||||
PicodetPostProcessor post_processor_;
|
||||
};
|
||||
|
||||
} // namespace PaddleOCR
|
|
@ -14,26 +14,11 @@
|
|||
|
||||
#pragma once
|
||||
|
||||
#include "opencv2/core.hpp"
|
||||
#include "opencv2/imgcodecs.hpp"
|
||||
#include "opencv2/imgproc.hpp"
|
||||
#include "paddle_api.h"
|
||||
#include "paddle_inference_api.h"
|
||||
#include <chrono>
|
||||
#include <iomanip>
|
||||
#include <iostream>
|
||||
#include <ostream>
|
||||
#include <vector>
|
||||
|
||||
#include <cstring>
|
||||
#include <fstream>
|
||||
#include <numeric>
|
||||
|
||||
#include <include/postprocess_op.h>
|
||||
#include <include/preprocess_op.h>
|
||||
#include <include/utility.h>
|
||||
|
||||
using namespace paddle_infer;
|
||||
|
||||
namespace PaddleOCR {
|
||||
|
||||
|
@ -42,7 +27,7 @@ public:
|
|||
explicit StructureTableRecognizer(
|
||||
const std::string &model_dir, const bool &use_gpu, const int &gpu_id,
|
||||
const int &gpu_mem, const int &cpu_math_library_num_threads,
|
||||
const bool &use_mkldnn, const string &label_path,
|
||||
const bool &use_mkldnn, const std::string &label_path,
|
||||
const bool &use_tensorrt, const std::string &precision,
|
||||
const int &table_batch_num, const int &table_max_len,
|
||||
const bool &merge_no_span_structure) {
|
||||
|
@ -70,7 +55,7 @@ public:
|
|||
std::vector<double> ×);
|
||||
|
||||
private:
|
||||
std::shared_ptr<Predictor> predictor_;
|
||||
std::shared_ptr<paddle_infer::Predictor> predictor_;
|
||||
|
||||
bool use_gpu_ = false;
|
||||
int gpu_id_ = 0;
|
||||
|
|
|
@ -41,12 +41,13 @@ struct OCRPredictResult {
|
|||
};
|
||||
|
||||
struct StructurePredictResult {
|
||||
std::vector<int> box;
|
||||
std::vector<float> box;
|
||||
std::vector<std::vector<int>> cell_box;
|
||||
std::string type;
|
||||
std::vector<OCRPredictResult> text_res;
|
||||
std::string html;
|
||||
float html_score = -1;
|
||||
float confidence;
|
||||
};
|
||||
|
||||
class Utility {
|
||||
|
@ -82,13 +83,20 @@ public:
|
|||
|
||||
static void print_result(const std::vector<OCRPredictResult> &ocr_result);
|
||||
|
||||
static cv::Mat crop_image(cv::Mat &img, std::vector<int> &area);
|
||||
static cv::Mat crop_image(cv::Mat &img, const std::vector<int> &area);
|
||||
static cv::Mat crop_image(cv::Mat &img, const std::vector<float> &area);
|
||||
|
||||
static void sorted_boxes(std::vector<OCRPredictResult> &ocr_result);
|
||||
|
||||
static std::vector<int> xyxyxyxy2xyxy(std::vector<std::vector<int>> &box);
|
||||
static std::vector<int> xyxyxyxy2xyxy(std::vector<int> &box);
|
||||
|
||||
static float fast_exp(float x);
|
||||
static std::vector<float>
|
||||
activation_function_softmax(std::vector<float> &src);
|
||||
static float iou(std::vector<int> &box1, std::vector<int> &box2);
|
||||
static float iou(std::vector<float> &box1, std::vector<float> &box2);
|
||||
|
||||
private:
|
||||
static bool comparison_box(const OCRPredictResult &result1,
|
||||
const OCRPredictResult &result2) {
|
||||
|
|
|
@ -174,6 +174,9 @@ inference/
|
|||
|-- table
|
||||
| |--inference.pdiparams
|
||||
| |--inference.pdmodel
|
||||
|-- layout
|
||||
| |--inference.pdiparams
|
||||
| |--inference.pdmodel
|
||||
```
|
||||
|
||||
|
||||
|
@ -278,8 +281,30 @@ Specifically,
|
|||
--cls=true \
|
||||
```
|
||||
|
||||
##### 7. layout+table
|
||||
```shell
|
||||
./build/ppocr --det_model_dir=inference/det_db \
|
||||
--rec_model_dir=inference/rec_rcnn \
|
||||
--table_model_dir=inference/table \
|
||||
--image_dir=../../ppstructure/docs/table/table.jpg \
|
||||
--layout_model_dir=inference/layout \
|
||||
--type=structure \
|
||||
--table=true \
|
||||
--layout=true
|
||||
```
|
||||
|
||||
##### 7. table
|
||||
##### 8. layout
|
||||
```shell
|
||||
./build/ppocr --layout_model_dir=inference/layout \
|
||||
--image_dir=../../ppstructure/docs/table/1.png \
|
||||
--type=structure \
|
||||
--table=false \
|
||||
--layout=true \
|
||||
--det=false \
|
||||
--rec=false
|
||||
```
|
||||
|
||||
##### 9. table
|
||||
```shell
|
||||
./build/ppocr --det_model_dir=inference/det_db \
|
||||
--rec_model_dir=inference/rec_rcnn \
|
||||
|
@ -343,6 +368,16 @@ More parameters are as follows,
|
|||
|rec_img_h|int|48|image height of recognition|
|
||||
|rec_img_w|int|320|image width of recognition|
|
||||
|
||||
- Layout related parameters
|
||||
|
||||
|parameter|data type|default|meaning|
|
||||
| :---: | :---: | :---: | :---: |
|
||||
|layout_model_dir|string|-| Address of layout inference model|
|
||||
|layout_dict_path|string|../../ppocr/utils/dict/layout_dict/layout_publaynet_dict.txt|dictionary file|
|
||||
|layout_score_threshold|float|0.5|Threshold of score.|
|
||||
|layout_nms_threshold|float|0.5|Threshold of nms.|
|
||||
|
||||
|
||||
- Table recognition related parameters
|
||||
|
||||
|parameter|data type|default|meaning|
|
||||
|
@ -368,11 +403,51 @@ predict img: ../../doc/imgs/12.jpg
|
|||
The detection visualized image saved in ./output//12.jpg
|
||||
```
|
||||
|
||||
- table
|
||||
- layout+table
|
||||
|
||||
```bash
|
||||
predict img: ../../ppstructure/docs/table/table.jpg
|
||||
0 type: table, region: [0,0,371,293], res: <html><body><table><thead><tr><td>Methods</td><td>R</td><td>P</td><td>F</td><td>FPS</td></tr></thead><tbody><tr><td>SegLink [26]</td><td>70.0</td><td>86.0</td><td>77.0</td><td>8.9</td></tr><tr><td>PixelLink [4]</td><td>73.2</td><td>83.0</td><td>77.8</td><td>-</td></tr><tr><td>TextSnake [18]</td><td>73.9</td><td>83.2</td><td>78.3</td><td>1.1</td></tr><tr><td>TextField [37]</td><td>75.9</td><td>87.4</td><td>81.3</td><td>5.2 </td></tr><tr><td>MSR[38]</td><td>76.7</td><td>87.4</td><td>81.7</td><td>-</td></tr><tr><td>FTSN [3]</td><td>77.1</td><td>87.6</td><td>82.0</td><td>-</td></tr><tr><td>LSE[30]</td><td>81.7</td><td>84.2</td><td>82.9</td><td>-</td></tr><tr><td>CRAFT [2]</td><td>78.2</td><td>88.2</td><td>82.9</td><td>8.6</td></tr><tr><td>MCN [16]</td><td>79</td><td>88</td><td>83</td><td>-</td></tr><tr><td>ATRR[35]</td><td>82.1</td><td>85.2</td><td>83.6</td><td>-</td></tr><tr><td>PAN [34]</td><td>83.8</td><td>84.4</td><td>84.1</td><td>30.2</td></tr><tr><td>DB[12]</td><td>79.2</td><td>91.5</td><td>84.9</td><td>32.0</td></tr><tr><td>DRRG [41]</td><td>82.30</td><td>88.05</td><td>85.08</td><td>-</td></tr><tr><td>Ours (SynText)</td><td>80.68</td><td>85.40</td><td>82.97</td><td>12.68</td></tr><tr><td>Ours (MLT-17)</td><td>84.54</td><td>86.62</td><td>85.57</td><td>12.31</td></tr></tbody></table></body></html>
|
||||
predict img: ../../ppstructure/docs/table/1.png
|
||||
0 type: text, region: [12,729,410,848], score: 0.781044, res: count of ocr result is : 7
|
||||
********** print ocr result **********
|
||||
0 det boxes: [[4,1],[79,1],[79,12],[4,12]] rec text: CTW1500. rec score: 0.769472
|
||||
...
|
||||
6 det boxes: [[4,99],[391,99],[391,112],[4,112]] rec text: sate-of-the-artmethods[12.34.36l.ourapproachachieves rec score: 0.90414
|
||||
********** end print ocr result **********
|
||||
1 type: text, region: [69,342,342,359], score: 0.703666, res: count of ocr result is : 1
|
||||
********** print ocr result **********
|
||||
0 det boxes: [[8,2],[269,2],[269,13],[8,13]] rec text: Table6.Experimentalresults on CTW-1500 rec score: 0.890454
|
||||
********** end print ocr result **********
|
||||
2 type: text, region: [70,316,706,332], score: 0.659738, res: count of ocr result is : 2
|
||||
********** print ocr result **********
|
||||
0 det boxes: [[373,2],[630,2],[630,11],[373,11]] rec text: oroposals.andthegreencontoursarefinal rec score: 0.919729
|
||||
1 det boxes: [[8,3],[357,3],[357,11],[8,11]] rec text: Visualexperimentalresultshebluecontoursareboundar rec score: 0.915963
|
||||
********** end print ocr result **********
|
||||
3 type: text, region: [489,342,789,359], score: 0.630538, res: count of ocr result is : 1
|
||||
********** print ocr result **********
|
||||
0 det boxes: [[8,2],[294,2],[294,14],[8,14]] rec text: Table7.Experimentalresults onMSRA-TD500 rec score: 0.942251
|
||||
********** end print ocr result **********
|
||||
4 type: text, region: [444,751,841,848], score: 0.607345, res: count of ocr result is : 5
|
||||
********** print ocr result **********
|
||||
0 det boxes: [[19,3],[389,3],[389,17],[19,17]] rec text: Inthispaper,weproposeanovel adaptivebound rec score: 0.941031
|
||||
1 det boxes: [[4,22],[390,22],[390,36],[4,36]] rec text: aryproposalnetworkforarbitraryshapetextdetection rec score: 0.960172
|
||||
2 det boxes: [[4,42],[392,42],[392,56],[4,56]] rec text: whichadoptanboundaryproposalmodeltogeneratecoarse rec score: 0.934647
|
||||
3 det boxes: [[4,61],[389,61],[389,75],[4,75]] rec text: ooundaryproposals,andthenadoptanadaptiveboundary rec score: 0.946296
|
||||
4 det boxes: [[5,80],[387,80],[387,93],[5,93]] rec text: leformationmodelcombinedwithGCNandRNNtoper rec score: 0.952401
|
||||
********** end print ocr result **********
|
||||
5 type: title, region: [444,705,564,724], score: 0.785429, res: count of ocr result is : 1
|
||||
********** print ocr result **********
|
||||
0 det boxes: [[6,2],[113,2],[113,14],[6,14]] rec text: 5.Conclusion rec score: 0.856903
|
||||
********** end print ocr result **********
|
||||
6 type: table, region: [14,360,402,711], score: 0.963643, res: <html><body><table><thead><tr><td>Methods</td><td>Ext</td><td>R</td><td>P</td><td>F</td><td>FPS</td></tr></thead><tbody><tr><td>TextSnake [18]</td><td>Syn</td><td>85.3</td><td>67.9</td><td>75.6</td><td></td></tr><tr><td>CSE [17]</td><td>MiLT</td><td>76.1</td><td>78.7</td><td>77.4</td><td>0.38</td></tr><tr><td>LOMO[40]</td><td>Syn</td><td>76.5</td><td>85.7</td><td>80.8</td><td>4.4</td></tr><tr><td>ATRR[35]</td><td>Sy-</td><td>80.2</td><td>80.1</td><td>80.1</td><td>-</td></tr><tr><td>SegLink++ [28]</td><td>Syn</td><td>79.8</td><td>82.8</td><td>81.3</td><td>-</td></tr><tr><td>TextField [37]</td><td>Syn</td><td>79.8</td><td>83.0</td><td>81.4</td><td>6.0</td></tr><tr><td>MSR[38]</td><td>Syn</td><td>79.0</td><td>84.1</td><td>81.5</td><td>4.3</td></tr><tr><td>PSENet-1s [33]</td><td>MLT</td><td>79.7</td><td>84.8</td><td>82.2</td><td>3.9</td></tr><tr><td>DB [12]</td><td>Syn</td><td>80.2</td><td>86.9</td><td>83.4</td><td>22.0</td></tr><tr><td>CRAFT [2]</td><td>Syn</td><td>81.1</td><td>86.0</td><td>83.5</td><td>-</td></tr><tr><td>TextDragon [5]</td><td>MLT+</td><td>82.8</td><td>84.5</td><td>83.6</td><td></td></tr><tr><td>PAN [34]</td><td>Syn</td><td>81.2</td><td>86.4</td><td>83.7</td><td>39.8</td></tr><tr><td>ContourNet [36]</td><td></td><td>84.1</td><td>83.7</td><td>83.9</td><td>4.5</td></tr><tr><td>DRRG [41]</td><td>MLT</td><td>83.02</td><td>85.93</td><td>84.45</td><td>-</td></tr><tr><td>TextPerception[23]</td><td>Syn</td><td>81.9</td><td>87.5</td><td>84.6</td><td></td></tr><tr><td>Ours</td><td> Syn</td><td>80.57</td><td>87.66</td><td>83.97</td><td>12.08</td></tr><tr><td>Ours</td><td></td><td>81.45</td><td>87.81</td><td>84.51</td><td>12.15</td></tr><tr><td>Ours</td><td>MLT</td><td>83.60</td><td>86.45</td><td>85.00</td><td>12.21</td></tr></tbody></table></body></html>
|
||||
The table visualized image saved in ./output//6_1.png
|
||||
7 type: table, region: [462,359,820,657], score: 0.953917, res: <html><body><table><thead><tr><td>Methods</td><td>R</td><td>P</td><td>F</td><td>FPS</td></tr></thead><tbody><tr><td>SegLink [26]</td><td>70.0</td><td>86.0</td><td>77.0</td><td>8.9</td></tr><tr><td>PixelLink [4]</td><td>73.2</td><td>83.0</td><td>77.8</td><td>-</td></tr><tr><td>TextSnake [18]</td><td>73.9</td><td>83.2</td><td>78.3</td><td>1.1</td></tr><tr><td>TextField [37]</td><td>75.9</td><td>87.4</td><td>81.3</td><td>5.2 </td></tr><tr><td>MSR[38]</td><td>76.7</td><td>87.4</td><td>81.7</td><td>-</td></tr><tr><td>FTSN[3]</td><td>77.1</td><td>87.6</td><td>82.0</td><td>:</td></tr><tr><td>LSE[30]</td><td>81.7</td><td>84.2</td><td>82.9</td><td></td></tr><tr><td>CRAFT [2]</td><td>78.2</td><td>88.2</td><td>82.9</td><td>8.6</td></tr><tr><td>MCN [16]</td><td>79</td><td>88</td><td>83</td><td>-</td></tr><tr><td>ATRR[35]</td><td>82.1</td><td>85.2</td><td>83.6</td><td>-</td></tr><tr><td>PAN [34]</td><td>83.8</td><td>84.4</td><td>84.1</td><td>30.2</td></tr><tr><td>DB[12]</td><td>79.2</td><td>91.5</td><td>84.9</td><td>32.0</td></tr><tr><td>DRRG [41]</td><td>82.30</td><td>88.05</td><td>85.08</td><td>-</td></tr><tr><td>Ours (SynText)</td><td>80.68</td><td>85.40</td><td>82.97</td><td>12.68</td></tr><tr><td>Ours (MLT-17)</td><td>84.54</td><td>86.62</td><td>85.57</td><td>12.31</td></tr></tbody></table></body></html>
|
||||
The table visualized image saved in ./output//7_1.png
|
||||
8 type: figure, region: [14,3,836,310], score: 0.969443, res: count of ocr result is : 26
|
||||
********** print ocr result **********
|
||||
0 det boxes: [[506,14],[539,15],[539,22],[506,21]] rec text: E rec score: 0.318073
|
||||
...
|
||||
25 det boxes: [[680,290],[759,288],[759,303],[680,305]] rec text: (d) CTW1500 rec score: 0.95911
|
||||
********** end print ocr result **********
|
||||
```
|
||||
|
||||
<a name="3"></a>
|
||||
|
|
|
@ -184,6 +184,9 @@ inference/
|
|||
|-- table
|
||||
| |--inference.pdiparams
|
||||
| |--inference.pdmodel
|
||||
|-- layout
|
||||
| |--inference.pdiparams
|
||||
| |--inference.pdmodel
|
||||
```
|
||||
|
||||
<a name="22"></a>
|
||||
|
@ -288,7 +291,30 @@ CUDNN_LIB_DIR=/your_cudnn_lib_dir
|
|||
--cls=true \
|
||||
```
|
||||
|
||||
##### 7. 表格识别
|
||||
##### 7. 版面分析+表格识别
|
||||
```shell
|
||||
./build/ppocr --det_model_dir=inference/det_db \
|
||||
--rec_model_dir=inference/rec_rcnn \
|
||||
--table_model_dir=inference/table \
|
||||
--image_dir=../../ppstructure/docs/table/table.jpg \
|
||||
--layout_model_dir=inference/layout \
|
||||
--type=structure \
|
||||
--table=true \
|
||||
--layout=true
|
||||
```
|
||||
|
||||
##### 8. 版面分析
|
||||
```shell
|
||||
./build/ppocr --layout_model_dir=inference/layout \
|
||||
--image_dir=../../ppstructure/docs/table/1.png \
|
||||
--type=structure \
|
||||
--table=false \
|
||||
--layout=true \
|
||||
--det=false \
|
||||
--rec=false
|
||||
```
|
||||
|
||||
##### 9. 表格识别
|
||||
```shell
|
||||
./build/ppocr --det_model_dir=inference/det_db \
|
||||
--rec_model_dir=inference/rec_rcnn \
|
||||
|
@ -352,12 +378,22 @@ CUDNN_LIB_DIR=/your_cudnn_lib_dir
|
|||
|rec_img_w|int|320|文字识别模型输入图像宽度|
|
||||
|
||||
|
||||
- 版面分析模型相关
|
||||
|
||||
|参数名称|类型|默认参数|意义|
|
||||
| :---: | :---: | :---: | :---: |
|
||||
|layout_model_dir|string|-|版面分析模型inference model地址|
|
||||
|layout_dict_path|string|../../ppocr/utils/dict/layout_dict/layout_publaynet_dict.txt|字典文件|
|
||||
|layout_score_threshold|float|0.5|检测框的分数阈值|
|
||||
|layout_nms_threshold|float|0.5|nms的阈值|
|
||||
|
||||
|
||||
- 表格识别模型相关
|
||||
|
||||
|参数名称|类型|默认参数|意义|
|
||||
| :---: | :---: | :---: | :---: |
|
||||
|table_model_dir|string|-|表格识别模型inference model地址|
|
||||
|table_char_dict_path|string|../../ppocr/utils/dict/table_structure_dict.txt|字典文件|
|
||||
|table_char_dict_path|string|../../ppocr/utils/dict/table_structure_dict_ch.txt|字典文件|
|
||||
|table_max_len|int|488|表格识别模型输入图像长边大小,最终网络输入图像大小为(table_max_len,table_max_len)|
|
||||
|merge_no_span_structure|bool|true|是否合并<td> 和 </td> 为<td></td>|
|
||||
|
||||
|
@ -378,11 +414,51 @@ predict img: ../../doc/imgs/12.jpg
|
|||
The detection visualized image saved in ./output//12.jpg
|
||||
```
|
||||
|
||||
- table
|
||||
- layout+table
|
||||
|
||||
```bash
|
||||
predict img: ../../ppstructure/docs/table/table.jpg
|
||||
0 type: table, region: [0,0,371,293], res: <html><body><table><thead><tr><td>Methods</td><td>R</td><td>P</td><td>F</td><td>FPS</td></tr></thead><tbody><tr><td>SegLink [26]</td><td>70.0</td><td>86.0</td><td>77.0</td><td>8.9</td></tr><tr><td>PixelLink [4]</td><td>73.2</td><td>83.0</td><td>77.8</td><td>-</td></tr><tr><td>TextSnake [18]</td><td>73.9</td><td>83.2</td><td>78.3</td><td>1.1</td></tr><tr><td>TextField [37]</td><td>75.9</td><td>87.4</td><td>81.3</td><td>5.2 </td></tr><tr><td>MSR[38]</td><td>76.7</td><td>87.4</td><td>81.7</td><td>-</td></tr><tr><td>FTSN [3]</td><td>77.1</td><td>87.6</td><td>82.0</td><td>-</td></tr><tr><td>LSE[30]</td><td>81.7</td><td>84.2</td><td>82.9</td><td>-</td></tr><tr><td>CRAFT [2]</td><td>78.2</td><td>88.2</td><td>82.9</td><td>8.6</td></tr><tr><td>MCN [16]</td><td>79</td><td>88</td><td>83</td><td>-</td></tr><tr><td>ATRR[35]</td><td>82.1</td><td>85.2</td><td>83.6</td><td>-</td></tr><tr><td>PAN [34]</td><td>83.8</td><td>84.4</td><td>84.1</td><td>30.2</td></tr><tr><td>DB[12]</td><td>79.2</td><td>91.5</td><td>84.9</td><td>32.0</td></tr><tr><td>DRRG [41]</td><td>82.30</td><td>88.05</td><td>85.08</td><td>-</td></tr><tr><td>Ours (SynText)</td><td>80.68</td><td>85.40</td><td>82.97</td><td>12.68</td></tr><tr><td>Ours (MLT-17)</td><td>84.54</td><td>86.62</td><td>85.57</td><td>12.31</td></tr></tbody></table></body></html>
|
||||
predict img: ../../ppstructure/docs/table/1.png
|
||||
0 type: text, region: [12,729,410,848], score: 0.781044, res: count of ocr result is : 7
|
||||
********** print ocr result **********
|
||||
0 det boxes: [[4,1],[79,1],[79,12],[4,12]] rec text: CTW1500. rec score: 0.769472
|
||||
...
|
||||
6 det boxes: [[4,99],[391,99],[391,112],[4,112]] rec text: sate-of-the-artmethods[12.34.36l.ourapproachachieves rec score: 0.90414
|
||||
********** end print ocr result **********
|
||||
1 type: text, region: [69,342,342,359], score: 0.703666, res: count of ocr result is : 1
|
||||
********** print ocr result **********
|
||||
0 det boxes: [[8,2],[269,2],[269,13],[8,13]] rec text: Table6.Experimentalresults on CTW-1500 rec score: 0.890454
|
||||
********** end print ocr result **********
|
||||
2 type: text, region: [70,316,706,332], score: 0.659738, res: count of ocr result is : 2
|
||||
********** print ocr result **********
|
||||
0 det boxes: [[373,2],[630,2],[630,11],[373,11]] rec text: oroposals.andthegreencontoursarefinal rec score: 0.919729
|
||||
1 det boxes: [[8,3],[357,3],[357,11],[8,11]] rec text: Visualexperimentalresultshebluecontoursareboundar rec score: 0.915963
|
||||
********** end print ocr result **********
|
||||
3 type: text, region: [489,342,789,359], score: 0.630538, res: count of ocr result is : 1
|
||||
********** print ocr result **********
|
||||
0 det boxes: [[8,2],[294,2],[294,14],[8,14]] rec text: Table7.Experimentalresults onMSRA-TD500 rec score: 0.942251
|
||||
********** end print ocr result **********
|
||||
4 type: text, region: [444,751,841,848], score: 0.607345, res: count of ocr result is : 5
|
||||
********** print ocr result **********
|
||||
0 det boxes: [[19,3],[389,3],[389,17],[19,17]] rec text: Inthispaper,weproposeanovel adaptivebound rec score: 0.941031
|
||||
1 det boxes: [[4,22],[390,22],[390,36],[4,36]] rec text: aryproposalnetworkforarbitraryshapetextdetection rec score: 0.960172
|
||||
2 det boxes: [[4,42],[392,42],[392,56],[4,56]] rec text: whichadoptanboundaryproposalmodeltogeneratecoarse rec score: 0.934647
|
||||
3 det boxes: [[4,61],[389,61],[389,75],[4,75]] rec text: ooundaryproposals,andthenadoptanadaptiveboundary rec score: 0.946296
|
||||
4 det boxes: [[5,80],[387,80],[387,93],[5,93]] rec text: leformationmodelcombinedwithGCNandRNNtoper rec score: 0.952401
|
||||
********** end print ocr result **********
|
||||
5 type: title, region: [444,705,564,724], score: 0.785429, res: count of ocr result is : 1
|
||||
********** print ocr result **********
|
||||
0 det boxes: [[6,2],[113,2],[113,14],[6,14]] rec text: 5.Conclusion rec score: 0.856903
|
||||
********** end print ocr result **********
|
||||
6 type: table, region: [14,360,402,711], score: 0.963643, res: <html><body><table><thead><tr><td>Methods</td><td>Ext</td><td>R</td><td>P</td><td>F</td><td>FPS</td></tr></thead><tbody><tr><td>TextSnake [18]</td><td>Syn</td><td>85.3</td><td>67.9</td><td>75.6</td><td></td></tr><tr><td>CSE [17]</td><td>MiLT</td><td>76.1</td><td>78.7</td><td>77.4</td><td>0.38</td></tr><tr><td>LOMO[40]</td><td>Syn</td><td>76.5</td><td>85.7</td><td>80.8</td><td>4.4</td></tr><tr><td>ATRR[35]</td><td>Sy-</td><td>80.2</td><td>80.1</td><td>80.1</td><td>-</td></tr><tr><td>SegLink++ [28]</td><td>Syn</td><td>79.8</td><td>82.8</td><td>81.3</td><td>-</td></tr><tr><td>TextField [37]</td><td>Syn</td><td>79.8</td><td>83.0</td><td>81.4</td><td>6.0</td></tr><tr><td>MSR[38]</td><td>Syn</td><td>79.0</td><td>84.1</td><td>81.5</td><td>4.3</td></tr><tr><td>PSENet-1s [33]</td><td>MLT</td><td>79.7</td><td>84.8</td><td>82.2</td><td>3.9</td></tr><tr><td>DB [12]</td><td>Syn</td><td>80.2</td><td>86.9</td><td>83.4</td><td>22.0</td></tr><tr><td>CRAFT [2]</td><td>Syn</td><td>81.1</td><td>86.0</td><td>83.5</td><td>-</td></tr><tr><td>TextDragon [5]</td><td>MLT+</td><td>82.8</td><td>84.5</td><td>83.6</td><td></td></tr><tr><td>PAN [34]</td><td>Syn</td><td>81.2</td><td>86.4</td><td>83.7</td><td>39.8</td></tr><tr><td>ContourNet [36]</td><td></td><td>84.1</td><td>83.7</td><td>83.9</td><td>4.5</td></tr><tr><td>DRRG [41]</td><td>MLT</td><td>83.02</td><td>85.93</td><td>84.45</td><td>-</td></tr><tr><td>TextPerception[23]</td><td>Syn</td><td>81.9</td><td>87.5</td><td>84.6</td><td></td></tr><tr><td>Ours</td><td> Syn</td><td>80.57</td><td>87.66</td><td>83.97</td><td>12.08</td></tr><tr><td>Ours</td><td></td><td>81.45</td><td>87.81</td><td>84.51</td><td>12.15</td></tr><tr><td>Ours</td><td>MLT</td><td>83.60</td><td>86.45</td><td>85.00</td><td>12.21</td></tr></tbody></table></body></html>
|
||||
The table visualized image saved in ./output//6_1.png
|
||||
7 type: table, region: [462,359,820,657], score: 0.953917, res: <html><body><table><thead><tr><td>Methods</td><td>R</td><td>P</td><td>F</td><td>FPS</td></tr></thead><tbody><tr><td>SegLink [26]</td><td>70.0</td><td>86.0</td><td>77.0</td><td>8.9</td></tr><tr><td>PixelLink [4]</td><td>73.2</td><td>83.0</td><td>77.8</td><td>-</td></tr><tr><td>TextSnake [18]</td><td>73.9</td><td>83.2</td><td>78.3</td><td>1.1</td></tr><tr><td>TextField [37]</td><td>75.9</td><td>87.4</td><td>81.3</td><td>5.2 </td></tr><tr><td>MSR[38]</td><td>76.7</td><td>87.4</td><td>81.7</td><td>-</td></tr><tr><td>FTSN[3]</td><td>77.1</td><td>87.6</td><td>82.0</td><td>:</td></tr><tr><td>LSE[30]</td><td>81.7</td><td>84.2</td><td>82.9</td><td></td></tr><tr><td>CRAFT [2]</td><td>78.2</td><td>88.2</td><td>82.9</td><td>8.6</td></tr><tr><td>MCN [16]</td><td>79</td><td>88</td><td>83</td><td>-</td></tr><tr><td>ATRR[35]</td><td>82.1</td><td>85.2</td><td>83.6</td><td>-</td></tr><tr><td>PAN [34]</td><td>83.8</td><td>84.4</td><td>84.1</td><td>30.2</td></tr><tr><td>DB[12]</td><td>79.2</td><td>91.5</td><td>84.9</td><td>32.0</td></tr><tr><td>DRRG [41]</td><td>82.30</td><td>88.05</td><td>85.08</td><td>-</td></tr><tr><td>Ours (SynText)</td><td>80.68</td><td>85.40</td><td>82.97</td><td>12.68</td></tr><tr><td>Ours (MLT-17)</td><td>84.54</td><td>86.62</td><td>85.57</td><td>12.31</td></tr></tbody></table></body></html>
|
||||
The table visualized image saved in ./output//7_1.png
|
||||
8 type: figure, region: [14,3,836,310], score: 0.969443, res: count of ocr result is : 26
|
||||
********** print ocr result **********
|
||||
0 det boxes: [[506,14],[539,15],[539,22],[506,21]] rec text: E rec score: 0.318073
|
||||
...
|
||||
25 det boxes: [[680,290],[759,288],[759,303],[680,305]] rec text: (d) CTW1500 rec score: 0.95911
|
||||
********** end print ocr result **********
|
||||
```
|
||||
|
||||
<a name="3"></a>
|
||||
|
|
|
@ -51,6 +51,13 @@ DEFINE_string(rec_char_dict_path, "../../ppocr/utils/ppocr_keys_v1.txt",
|
|||
DEFINE_int32(rec_img_h, 48, "rec image height");
|
||||
DEFINE_int32(rec_img_w, 320, "rec image width");
|
||||
|
||||
// layout model related
|
||||
DEFINE_string(layout_model_dir, "", "Path of table layout inference model.");
|
||||
DEFINE_string(layout_dict_path,
|
||||
"../../ppocr/utils/dict/layout_dict/layout_publaynet_dict.txt",
|
||||
"Path of dictionary.");
|
||||
DEFINE_double(layout_score_threshold, 0.5, "Threshold of score.");
|
||||
DEFINE_double(layout_nms_threshold, 0.5, "Threshold of nms.");
|
||||
// structure model related
|
||||
DEFINE_string(table_model_dir, "", "Path of table struture inference model.");
|
||||
DEFINE_int32(table_max_len, 488, "max len size of input image.");
|
||||
|
@ -65,4 +72,5 @@ DEFINE_string(table_char_dict_path,
|
|||
DEFINE_bool(det, true, "Whether use det in forward.");
|
||||
DEFINE_bool(rec, true, "Whether use rec in forward.");
|
||||
DEFINE_bool(cls, false, "Whether use cls in forward.");
|
||||
DEFINE_bool(table, false, "Whether use table structure in forward.");
|
||||
DEFINE_bool(table, false, "Whether use table structure in forward.");
|
||||
DEFINE_bool(layout, false, "Whether use layout analysis in forward.");
|
|
@ -65,9 +65,18 @@ void check_params() {
|
|||
exit(1);
|
||||
}
|
||||
}
|
||||
if (FLAGS_layout) {
|
||||
if (FLAGS_layout_model_dir.empty() || FLAGS_image_dir.empty()) {
|
||||
std::cout << "Usage[layout]: ./ppocr "
|
||||
<< "--layout_model_dir=/PATH/TO/LAYOUT_INFERENCE_MODEL/ "
|
||||
<< "--image_dir=/PATH/TO/INPUT/IMAGE/" << std::endl;
|
||||
exit(1);
|
||||
}
|
||||
}
|
||||
if (FLAGS_precision != "fp32" && FLAGS_precision != "fp16" &&
|
||||
FLAGS_precision != "int8") {
|
||||
cout << "precison should be 'fp32'(default), 'fp16' or 'int8'. " << endl;
|
||||
std::cout << "precison should be 'fp32'(default), 'fp16' or 'int8'. "
|
||||
<< std::endl;
|
||||
exit(1);
|
||||
}
|
||||
}
|
||||
|
@ -75,71 +84,94 @@ void check_params() {
|
|||
void ocr(std::vector<cv::String> &cv_all_img_names) {
|
||||
PPOCR ocr = PPOCR();
|
||||
|
||||
std::vector<std::vector<OCRPredictResult>> ocr_results =
|
||||
ocr.ocr(cv_all_img_names, FLAGS_det, FLAGS_rec, FLAGS_cls);
|
||||
if (FLAGS_benchmark) {
|
||||
ocr.reset_timer();
|
||||
}
|
||||
|
||||
std::vector<cv::Mat> img_list;
|
||||
std::vector<cv::String> img_names;
|
||||
for (int i = 0; i < cv_all_img_names.size(); ++i) {
|
||||
if (FLAGS_benchmark) {
|
||||
cout << cv_all_img_names[i] << '\t';
|
||||
if (FLAGS_rec && FLAGS_det) {
|
||||
Utility::print_result(ocr_results[i]);
|
||||
} else if (FLAGS_det) {
|
||||
for (int n = 0; n < ocr_results[i].size(); n++) {
|
||||
for (int m = 0; m < ocr_results[i][n].box.size(); m++) {
|
||||
cout << ocr_results[i][n].box[m][0] << ' '
|
||||
<< ocr_results[i][n].box[m][1] << ' ';
|
||||
}
|
||||
}
|
||||
cout << endl;
|
||||
} else {
|
||||
Utility::print_result(ocr_results[i]);
|
||||
}
|
||||
} else {
|
||||
cout << cv_all_img_names[i] << "\n";
|
||||
Utility::print_result(ocr_results[i]);
|
||||
if (FLAGS_visualize && FLAGS_det) {
|
||||
cv::Mat srcimg = cv::imread(cv_all_img_names[i], cv::IMREAD_COLOR);
|
||||
if (!srcimg.data) {
|
||||
std::cerr << "[ERROR] image read failed! image path: "
|
||||
<< cv_all_img_names[i] << endl;
|
||||
exit(1);
|
||||
}
|
||||
std::string file_name = Utility::basename(cv_all_img_names[i]);
|
||||
|
||||
Utility::VisualizeBboxes(srcimg, ocr_results[i],
|
||||
FLAGS_output + "/" + file_name);
|
||||
}
|
||||
cout << "***************************" << endl;
|
||||
cv::Mat img = cv::imread(cv_all_img_names[i], cv::IMREAD_COLOR);
|
||||
if (!img.data) {
|
||||
std::cerr << "[ERROR] image read failed! image path: "
|
||||
<< cv_all_img_names[i] << std::endl;
|
||||
continue;
|
||||
}
|
||||
img_list.push_back(img);
|
||||
img_names.push_back(cv_all_img_names[i]);
|
||||
}
|
||||
|
||||
std::vector<std::vector<OCRPredictResult>> ocr_results =
|
||||
ocr.ocr(img_list, FLAGS_det, FLAGS_rec, FLAGS_cls);
|
||||
|
||||
for (int i = 0; i < img_names.size(); ++i) {
|
||||
std::cout << "predict img: " << cv_all_img_names[i] << std::endl;
|
||||
Utility::print_result(ocr_results[i]);
|
||||
if (FLAGS_visualize && FLAGS_det) {
|
||||
std::string file_name = Utility::basename(img_names[i]);
|
||||
cv::Mat srcimg = img_list[i];
|
||||
Utility::VisualizeBboxes(srcimg, ocr_results[i],
|
||||
FLAGS_output + "/" + file_name);
|
||||
}
|
||||
}
|
||||
if (FLAGS_benchmark) {
|
||||
ocr.benchmark_log(cv_all_img_names.size());
|
||||
}
|
||||
}
|
||||
|
||||
void structure(std::vector<cv::String> &cv_all_img_names) {
|
||||
PaddleOCR::PaddleStructure engine = PaddleOCR::PaddleStructure();
|
||||
std::vector<std::vector<StructurePredictResult>> structure_results =
|
||||
engine.structure(cv_all_img_names, false, FLAGS_table);
|
||||
for (int i = 0; i < cv_all_img_names.size(); i++) {
|
||||
cout << "predict img: " << cv_all_img_names[i] << endl;
|
||||
cv::Mat srcimg = cv::imread(cv_all_img_names[i], cv::IMREAD_COLOR);
|
||||
for (int j = 0; j < structure_results[i].size(); j++) {
|
||||
std::cout << j << "\ttype: " << structure_results[i][j].type
|
||||
<< ", region: [";
|
||||
std::cout << structure_results[i][j].box[0] << ","
|
||||
<< structure_results[i][j].box[1] << ","
|
||||
<< structure_results[i][j].box[2] << ","
|
||||
<< structure_results[i][j].box[3] << "], res: ";
|
||||
if (structure_results[i][j].type == "table") {
|
||||
std::cout << structure_results[i][j].html << std::endl;
|
||||
std::string file_name = Utility::basename(cv_all_img_names[i]);
|
||||
|
||||
Utility::VisualizeBboxes(srcimg, structure_results[i][j],
|
||||
FLAGS_output + "/" + std::to_string(j) + "_" +
|
||||
file_name);
|
||||
if (FLAGS_benchmark) {
|
||||
engine.reset_timer();
|
||||
}
|
||||
|
||||
for (int i = 0; i < cv_all_img_names.size(); i++) {
|
||||
std::cout << "predict img: " << cv_all_img_names[i] << std::endl;
|
||||
cv::Mat img = cv::imread(cv_all_img_names[i], cv::IMREAD_COLOR);
|
||||
if (!img.data) {
|
||||
std::cerr << "[ERROR] image read failed! image path: "
|
||||
<< cv_all_img_names[i] << std::endl;
|
||||
continue;
|
||||
}
|
||||
|
||||
std::vector<StructurePredictResult> structure_results = engine.structure(
|
||||
img, FLAGS_layout, FLAGS_table, FLAGS_det && FLAGS_rec);
|
||||
|
||||
for (int j = 0; j < structure_results.size(); j++) {
|
||||
std::cout << j << "\ttype: " << structure_results[j].type
|
||||
<< ", region: [";
|
||||
std::cout << structure_results[j].box[0] << ","
|
||||
<< structure_results[j].box[1] << ","
|
||||
<< structure_results[j].box[2] << ","
|
||||
<< structure_results[j].box[3] << "], score: ";
|
||||
std::cout << structure_results[j].confidence << ", res: ";
|
||||
|
||||
if (structure_results[j].type == "table") {
|
||||
std::cout << structure_results[j].html << std::endl;
|
||||
if (structure_results[j].cell_box.size() > 0 && FLAGS_visualize) {
|
||||
std::string file_name = Utility::basename(cv_all_img_names[i]);
|
||||
|
||||
Utility::VisualizeBboxes(img, structure_results[j],
|
||||
FLAGS_output + "/" + std::to_string(j) +
|
||||
"_" + file_name);
|
||||
}
|
||||
} else {
|
||||
Utility::print_result(structure_results[i][j].text_res);
|
||||
std::cout << "count of ocr result is : "
|
||||
<< structure_results[j].text_res.size() << std::endl;
|
||||
if (structure_results[j].text_res.size() > 0) {
|
||||
std::cout << "********** print ocr result "
|
||||
<< "**********" << std::endl;
|
||||
Utility::print_result(structure_results[j].text_res);
|
||||
std::cout << "********** end print ocr result "
|
||||
<< "**********" << std::endl;
|
||||
}
|
||||
}
|
||||
}
|
||||
}
|
||||
if (FLAGS_benchmark) {
|
||||
engine.benchmark_log(cv_all_img_names.size());
|
||||
}
|
||||
}
|
||||
|
||||
int main(int argc, char **argv) {
|
||||
|
@ -149,19 +181,22 @@ int main(int argc, char **argv) {
|
|||
|
||||
if (!Utility::PathExists(FLAGS_image_dir)) {
|
||||
std::cerr << "[ERROR] image path not exist! image_dir: " << FLAGS_image_dir
|
||||
<< endl;
|
||||
<< std::endl;
|
||||
exit(1);
|
||||
}
|
||||
|
||||
std::vector<cv::String> cv_all_img_names;
|
||||
cv::glob(FLAGS_image_dir, cv_all_img_names);
|
||||
std::cout << "total images num: " << cv_all_img_names.size() << endl;
|
||||
std::cout << "total images num: " << cv_all_img_names.size() << std::endl;
|
||||
|
||||
if (!Utility::PathExists(FLAGS_output)) {
|
||||
Utility::CreateDir(FLAGS_output);
|
||||
}
|
||||
if (FLAGS_type == "ocr") {
|
||||
ocr(cv_all_img_names);
|
||||
} else if (FLAGS_type == "structure") {
|
||||
structure(cv_all_img_names);
|
||||
} else {
|
||||
std::cout << "only value in ['ocr','structure'] is supported" << endl;
|
||||
std::cout << "only value in ['ocr','structure'] is supported" << std::endl;
|
||||
}
|
||||
}
|
||||
|
|
|
@ -32,7 +32,7 @@ void Classifier::Run(std::vector<cv::Mat> img_list,
|
|||
for (int beg_img_no = 0; beg_img_no < img_num;
|
||||
beg_img_no += this->cls_batch_num_) {
|
||||
auto preprocess_start = std::chrono::steady_clock::now();
|
||||
int end_img_no = min(img_num, beg_img_no + this->cls_batch_num_);
|
||||
int end_img_no = std::min(img_num, beg_img_no + this->cls_batch_num_);
|
||||
int batch_num = end_img_no - beg_img_no;
|
||||
// preprocess
|
||||
std::vector<cv::Mat> norm_img_batch;
|
||||
|
@ -97,7 +97,7 @@ void Classifier::Run(std::vector<cv::Mat> img_list,
|
|||
}
|
||||
|
||||
void Classifier::LoadModel(const std::string &model_dir) {
|
||||
AnalysisConfig config;
|
||||
paddle_infer::Config config;
|
||||
config.SetModel(model_dir + "/inference.pdmodel",
|
||||
model_dir + "/inference.pdiparams");
|
||||
|
||||
|
@ -112,9 +112,9 @@ void Classifier::LoadModel(const std::string &model_dir) {
|
|||
precision = paddle_infer::Config::Precision::kInt8;
|
||||
}
|
||||
config.EnableTensorRtEngine(1 << 20, 10, 3, precision, false, false);
|
||||
if (!Utility::PathExists("./trt_cls_shape.txt")){
|
||||
if (!Utility::PathExists("./trt_cls_shape.txt")) {
|
||||
config.CollectShapeRangeInfo("./trt_cls_shape.txt");
|
||||
} else {
|
||||
} else {
|
||||
config.EnableTunedTensorRtDynamicShape("./trt_cls_shape.txt", true);
|
||||
}
|
||||
}
|
||||
|
@ -136,6 +136,6 @@ void Classifier::LoadModel(const std::string &model_dir) {
|
|||
config.EnableMemoryOptim();
|
||||
config.DisableGlogInfo();
|
||||
|
||||
this->predictor_ = CreatePredictor(config);
|
||||
this->predictor_ = paddle_infer::CreatePredictor(config);
|
||||
}
|
||||
} // namespace PaddleOCR
|
||||
|
|
|
@ -33,12 +33,11 @@ void DBDetector::LoadModel(const std::string &model_dir) {
|
|||
precision = paddle_infer::Config::Precision::kInt8;
|
||||
}
|
||||
config.EnableTensorRtEngine(1 << 30, 1, 20, precision, false, false);
|
||||
if (!Utility::PathExists("./trt_det_shape.txt")){
|
||||
if (!Utility::PathExists("./trt_det_shape.txt")) {
|
||||
config.CollectShapeRangeInfo("./trt_det_shape.txt");
|
||||
} else {
|
||||
} else {
|
||||
config.EnableTunedTensorRtDynamicShape("./trt_det_shape.txt", true);
|
||||
}
|
||||
|
||||
}
|
||||
} else {
|
||||
config.DisableGpu();
|
||||
|
@ -59,7 +58,7 @@ void DBDetector::LoadModel(const std::string &model_dir) {
|
|||
config.EnableMemoryOptim();
|
||||
// config.DisableGlogInfo();
|
||||
|
||||
this->predictor_ = CreatePredictor(config);
|
||||
this->predictor_ = paddle_infer::CreatePredictor(config);
|
||||
}
|
||||
|
||||
void DBDetector::Run(cv::Mat &img,
|
||||
|
|
|
@ -37,7 +37,7 @@ void CRNNRecognizer::Run(std::vector<cv::Mat> img_list,
|
|||
for (int beg_img_no = 0; beg_img_no < img_num;
|
||||
beg_img_no += this->rec_batch_num_) {
|
||||
auto preprocess_start = std::chrono::steady_clock::now();
|
||||
int end_img_no = min(img_num, beg_img_no + this->rec_batch_num_);
|
||||
int end_img_no = std::min(img_num, beg_img_no + this->rec_batch_num_);
|
||||
int batch_num = end_img_no - beg_img_no;
|
||||
int imgH = this->rec_image_shape_[1];
|
||||
int imgW = this->rec_image_shape_[2];
|
||||
|
@ -46,7 +46,7 @@ void CRNNRecognizer::Run(std::vector<cv::Mat> img_list,
|
|||
int h = img_list[indices[ino]].rows;
|
||||
int w = img_list[indices[ino]].cols;
|
||||
float wh_ratio = w * 1.0 / h;
|
||||
max_wh_ratio = max(max_wh_ratio, wh_ratio);
|
||||
max_wh_ratio = std::max(max_wh_ratio, wh_ratio);
|
||||
}
|
||||
|
||||
int batch_width = imgW;
|
||||
|
@ -60,7 +60,7 @@ void CRNNRecognizer::Run(std::vector<cv::Mat> img_list,
|
|||
this->normalize_op_.Run(&resize_img, this->mean_, this->scale_,
|
||||
this->is_scale_);
|
||||
norm_img_batch.push_back(resize_img);
|
||||
batch_width = max(resize_img.cols, batch_width);
|
||||
batch_width = std::max(resize_img.cols, batch_width);
|
||||
}
|
||||
|
||||
std::vector<float> input(batch_num * 3 * imgH * batch_width, 0.0f);
|
||||
|
@ -115,7 +115,7 @@ void CRNNRecognizer::Run(std::vector<cv::Mat> img_list,
|
|||
last_index = argmax_idx;
|
||||
}
|
||||
score /= count;
|
||||
if (isnan(score)) {
|
||||
if (std::isnan(score)) {
|
||||
continue;
|
||||
}
|
||||
rec_texts[indices[beg_img_no + m]] = str_res;
|
||||
|
@ -130,7 +130,6 @@ void CRNNRecognizer::Run(std::vector<cv::Mat> img_list,
|
|||
}
|
||||
|
||||
void CRNNRecognizer::LoadModel(const std::string &model_dir) {
|
||||
// AnalysisConfig config;
|
||||
paddle_infer::Config config;
|
||||
config.SetModel(model_dir + "/inference.pdmodel",
|
||||
model_dir + "/inference.pdiparams");
|
||||
|
@ -147,12 +146,11 @@ void CRNNRecognizer::LoadModel(const std::string &model_dir) {
|
|||
if (this->precision_ == "int8") {
|
||||
precision = paddle_infer::Config::Precision::kInt8;
|
||||
}
|
||||
if (!Utility::PathExists("./trt_rec_shape.txt")){
|
||||
if (!Utility::PathExists("./trt_rec_shape.txt")) {
|
||||
config.CollectShapeRangeInfo("./trt_rec_shape.txt");
|
||||
} else {
|
||||
} else {
|
||||
config.EnableTunedTensorRtDynamicShape("./trt_rec_shape.txt", true);
|
||||
}
|
||||
|
||||
}
|
||||
} else {
|
||||
config.DisableGpu();
|
||||
|
@ -177,7 +175,7 @@ void CRNNRecognizer::LoadModel(const std::string &model_dir) {
|
|||
config.EnableMemoryOptim();
|
||||
// config.DisableGlogInfo();
|
||||
|
||||
this->predictor_ = CreatePredictor(config);
|
||||
this->predictor_ = paddle_infer::CreatePredictor(config);
|
||||
}
|
||||
|
||||
} // namespace PaddleOCR
|
||||
|
|
|
@ -16,7 +16,7 @@
|
|||
#include <include/paddleocr.h>
|
||||
|
||||
#include "auto_log/autolog.h"
|
||||
#include <numeric>
|
||||
|
||||
namespace PaddleOCR {
|
||||
|
||||
PPOCR::PPOCR() {
|
||||
|
@ -44,8 +44,71 @@ PPOCR::PPOCR() {
|
|||
}
|
||||
};
|
||||
|
||||
void PPOCR::det(cv::Mat img, std::vector<OCRPredictResult> &ocr_results,
|
||||
std::vector<double> ×) {
|
||||
std::vector<std::vector<OCRPredictResult>>
|
||||
PPOCR::ocr(std::vector<cv::Mat> img_list, bool det, bool rec, bool cls) {
|
||||
std::vector<std::vector<OCRPredictResult>> ocr_results;
|
||||
|
||||
if (!det) {
|
||||
std::vector<OCRPredictResult> ocr_result;
|
||||
ocr_result.resize(img_list.size());
|
||||
if (cls && this->classifier_ != nullptr) {
|
||||
this->cls(img_list, ocr_result);
|
||||
for (int i = 0; i < img_list.size(); i++) {
|
||||
if (ocr_result[i].cls_label % 2 == 1 &&
|
||||
ocr_result[i].cls_score > this->classifier_->cls_thresh) {
|
||||
cv::rotate(img_list[i], img_list[i], 1);
|
||||
}
|
||||
}
|
||||
}
|
||||
if (rec) {
|
||||
this->rec(img_list, ocr_result);
|
||||
}
|
||||
for (int i = 0; i < ocr_result.size(); ++i) {
|
||||
std::vector<OCRPredictResult> ocr_result_tmp;
|
||||
ocr_result_tmp.push_back(ocr_result[i]);
|
||||
ocr_results.push_back(ocr_result_tmp);
|
||||
}
|
||||
} else {
|
||||
for (int i = 0; i < img_list.size(); ++i) {
|
||||
std::vector<OCRPredictResult> ocr_result =
|
||||
this->ocr(img_list[i], true, rec, cls);
|
||||
ocr_results.push_back(ocr_result);
|
||||
}
|
||||
}
|
||||
return ocr_results;
|
||||
}
|
||||
|
||||
std::vector<OCRPredictResult> PPOCR::ocr(cv::Mat img, bool det, bool rec,
|
||||
bool cls) {
|
||||
|
||||
std::vector<OCRPredictResult> ocr_result;
|
||||
// det
|
||||
this->det(img, ocr_result);
|
||||
// crop image
|
||||
std::vector<cv::Mat> img_list;
|
||||
for (int j = 0; j < ocr_result.size(); j++) {
|
||||
cv::Mat crop_img;
|
||||
crop_img = Utility::GetRotateCropImage(img, ocr_result[j].box);
|
||||
img_list.push_back(crop_img);
|
||||
}
|
||||
// cls
|
||||
if (cls && this->classifier_ != nullptr) {
|
||||
this->cls(img_list, ocr_result);
|
||||
for (int i = 0; i < img_list.size(); i++) {
|
||||
if (ocr_result[i].cls_label % 2 == 1 &&
|
||||
ocr_result[i].cls_score > this->classifier_->cls_thresh) {
|
||||
cv::rotate(img_list[i], img_list[i], 1);
|
||||
}
|
||||
}
|
||||
}
|
||||
// rec
|
||||
if (rec) {
|
||||
this->rec(img_list, ocr_result);
|
||||
}
|
||||
return ocr_result;
|
||||
}
|
||||
|
||||
void PPOCR::det(cv::Mat img, std::vector<OCRPredictResult> &ocr_results) {
|
||||
std::vector<std::vector<std::vector<int>>> boxes;
|
||||
std::vector<double> det_times;
|
||||
|
||||
|
@ -58,14 +121,13 @@ void PPOCR::det(cv::Mat img, std::vector<OCRPredictResult> &ocr_results,
|
|||
}
|
||||
// sort boex from top to bottom, from left to right
|
||||
Utility::sorted_boxes(ocr_results);
|
||||
times[0] += det_times[0];
|
||||
times[1] += det_times[1];
|
||||
times[2] += det_times[2];
|
||||
this->time_info_det[0] += det_times[0];
|
||||
this->time_info_det[1] += det_times[1];
|
||||
this->time_info_det[2] += det_times[2];
|
||||
}
|
||||
|
||||
void PPOCR::rec(std::vector<cv::Mat> img_list,
|
||||
std::vector<OCRPredictResult> &ocr_results,
|
||||
std::vector<double> ×) {
|
||||
std::vector<OCRPredictResult> &ocr_results) {
|
||||
std::vector<std::string> rec_texts(img_list.size(), "");
|
||||
std::vector<float> rec_text_scores(img_list.size(), 0);
|
||||
std::vector<double> rec_times;
|
||||
|
@ -75,14 +137,13 @@ void PPOCR::rec(std::vector<cv::Mat> img_list,
|
|||
ocr_results[i].text = rec_texts[i];
|
||||
ocr_results[i].score = rec_text_scores[i];
|
||||
}
|
||||
times[0] += rec_times[0];
|
||||
times[1] += rec_times[1];
|
||||
times[2] += rec_times[2];
|
||||
this->time_info_rec[0] += rec_times[0];
|
||||
this->time_info_rec[1] += rec_times[1];
|
||||
this->time_info_rec[2] += rec_times[2];
|
||||
}
|
||||
|
||||
void PPOCR::cls(std::vector<cv::Mat> img_list,
|
||||
std::vector<OCRPredictResult> &ocr_results,
|
||||
std::vector<double> ×) {
|
||||
std::vector<OCRPredictResult> &ocr_results) {
|
||||
std::vector<int> cls_labels(img_list.size(), 0);
|
||||
std::vector<float> cls_scores(img_list.size(), 0);
|
||||
std::vector<double> cls_times;
|
||||
|
@ -92,125 +153,43 @@ void PPOCR::cls(std::vector<cv::Mat> img_list,
|
|||
ocr_results[i].cls_label = cls_labels[i];
|
||||
ocr_results[i].cls_score = cls_scores[i];
|
||||
}
|
||||
times[0] += cls_times[0];
|
||||
times[1] += cls_times[1];
|
||||
times[2] += cls_times[2];
|
||||
this->time_info_cls[0] += cls_times[0];
|
||||
this->time_info_cls[1] += cls_times[1];
|
||||
this->time_info_cls[2] += cls_times[2];
|
||||
}
|
||||
|
||||
std::vector<std::vector<OCRPredictResult>>
|
||||
PPOCR::ocr(std::vector<cv::String> cv_all_img_names, bool det, bool rec,
|
||||
bool cls) {
|
||||
std::vector<double> time_info_det = {0, 0, 0};
|
||||
std::vector<double> time_info_rec = {0, 0, 0};
|
||||
std::vector<double> time_info_cls = {0, 0, 0};
|
||||
std::vector<std::vector<OCRPredictResult>> ocr_results;
|
||||
void PPOCR::reset_timer() {
|
||||
this->time_info_det = {0, 0, 0};
|
||||
this->time_info_rec = {0, 0, 0};
|
||||
this->time_info_cls = {0, 0, 0};
|
||||
}
|
||||
|
||||
if (!det) {
|
||||
std::vector<OCRPredictResult> ocr_result;
|
||||
// read image
|
||||
std::vector<cv::Mat> img_list;
|
||||
for (int i = 0; i < cv_all_img_names.size(); ++i) {
|
||||
cv::Mat srcimg = cv::imread(cv_all_img_names[i], cv::IMREAD_COLOR);
|
||||
if (!srcimg.data) {
|
||||
std::cerr << "[ERROR] image read failed! image path: "
|
||||
<< cv_all_img_names[i] << endl;
|
||||
exit(1);
|
||||
}
|
||||
img_list.push_back(srcimg);
|
||||
OCRPredictResult res;
|
||||
ocr_result.push_back(res);
|
||||
}
|
||||
if (cls && this->classifier_ != nullptr) {
|
||||
this->cls(img_list, ocr_result, time_info_cls);
|
||||
for (int i = 0; i < img_list.size(); i++) {
|
||||
if (ocr_result[i].cls_label % 2 == 1 &&
|
||||
ocr_result[i].cls_score > this->classifier_->cls_thresh) {
|
||||
cv::rotate(img_list[i], img_list[i], 1);
|
||||
}
|
||||
}
|
||||
}
|
||||
if (rec) {
|
||||
this->rec(img_list, ocr_result, time_info_rec);
|
||||
}
|
||||
for (int i = 0; i < cv_all_img_names.size(); ++i) {
|
||||
std::vector<OCRPredictResult> ocr_result_tmp;
|
||||
ocr_result_tmp.push_back(ocr_result[i]);
|
||||
ocr_results.push_back(ocr_result_tmp);
|
||||
}
|
||||
} else {
|
||||
if (!Utility::PathExists(FLAGS_output) && FLAGS_det) {
|
||||
Utility::CreateDir(FLAGS_output);
|
||||
}
|
||||
|
||||
for (int i = 0; i < cv_all_img_names.size(); ++i) {
|
||||
std::vector<OCRPredictResult> ocr_result;
|
||||
if (!FLAGS_benchmark) {
|
||||
cout << "predict img: " << cv_all_img_names[i] << endl;
|
||||
}
|
||||
|
||||
cv::Mat srcimg = cv::imread(cv_all_img_names[i], cv::IMREAD_COLOR);
|
||||
if (!srcimg.data) {
|
||||
std::cerr << "[ERROR] image read failed! image path: "
|
||||
<< cv_all_img_names[i] << endl;
|
||||
exit(1);
|
||||
}
|
||||
// det
|
||||
this->det(srcimg, ocr_result, time_info_det);
|
||||
// crop image
|
||||
std::vector<cv::Mat> img_list;
|
||||
for (int j = 0; j < ocr_result.size(); j++) {
|
||||
cv::Mat crop_img;
|
||||
crop_img = Utility::GetRotateCropImage(srcimg, ocr_result[j].box);
|
||||
img_list.push_back(crop_img);
|
||||
}
|
||||
|
||||
// cls
|
||||
if (cls && this->classifier_ != nullptr) {
|
||||
this->cls(img_list, ocr_result, time_info_cls);
|
||||
for (int i = 0; i < img_list.size(); i++) {
|
||||
if (ocr_result[i].cls_label % 2 == 1 &&
|
||||
ocr_result[i].cls_score > this->classifier_->cls_thresh) {
|
||||
cv::rotate(img_list[i], img_list[i], 1);
|
||||
}
|
||||
}
|
||||
}
|
||||
// rec
|
||||
if (rec) {
|
||||
this->rec(img_list, ocr_result, time_info_rec);
|
||||
}
|
||||
ocr_results.push_back(ocr_result);
|
||||
}
|
||||
}
|
||||
if (FLAGS_benchmark) {
|
||||
this->log(time_info_det, time_info_rec, time_info_cls,
|
||||
cv_all_img_names.size());
|
||||
}
|
||||
return ocr_results;
|
||||
} // namespace PaddleOCR
|
||||
|
||||
void PPOCR::log(std::vector<double> &det_times, std::vector<double> &rec_times,
|
||||
std::vector<double> &cls_times, int img_num) {
|
||||
if (det_times[0] + det_times[1] + det_times[2] > 0) {
|
||||
void PPOCR::benchmark_log(int img_num) {
|
||||
if (this->time_info_det[0] + this->time_info_det[1] + this->time_info_det[2] >
|
||||
0) {
|
||||
AutoLogger autolog_det("ocr_det", FLAGS_use_gpu, FLAGS_use_tensorrt,
|
||||
FLAGS_enable_mkldnn, FLAGS_cpu_threads, 1, "dynamic",
|
||||
FLAGS_precision, det_times, img_num);
|
||||
FLAGS_precision, this->time_info_det, img_num);
|
||||
autolog_det.report();
|
||||
}
|
||||
if (rec_times[0] + rec_times[1] + rec_times[2] > 0) {
|
||||
if (this->time_info_rec[0] + this->time_info_rec[1] + this->time_info_rec[2] >
|
||||
0) {
|
||||
AutoLogger autolog_rec("ocr_rec", FLAGS_use_gpu, FLAGS_use_tensorrt,
|
||||
FLAGS_enable_mkldnn, FLAGS_cpu_threads,
|
||||
FLAGS_rec_batch_num, "dynamic", FLAGS_precision,
|
||||
rec_times, img_num);
|
||||
this->time_info_rec, img_num);
|
||||
autolog_rec.report();
|
||||
}
|
||||
if (cls_times[0] + cls_times[1] + cls_times[2] > 0) {
|
||||
if (this->time_info_cls[0] + this->time_info_cls[1] + this->time_info_cls[2] >
|
||||
0) {
|
||||
AutoLogger autolog_cls("ocr_cls", FLAGS_use_gpu, FLAGS_use_tensorrt,
|
||||
FLAGS_enable_mkldnn, FLAGS_cpu_threads,
|
||||
FLAGS_cls_batch_num, "dynamic", FLAGS_precision,
|
||||
cls_times, img_num);
|
||||
this->time_info_cls, img_num);
|
||||
autolog_cls.report();
|
||||
}
|
||||
}
|
||||
|
||||
PPOCR::~PPOCR() {
|
||||
if (this->detector_ != nullptr) {
|
||||
delete this->detector_;
|
||||
|
|
|
@ -16,14 +16,19 @@
|
|||
#include <include/paddlestructure.h>
|
||||
|
||||
#include "auto_log/autolog.h"
|
||||
#include <numeric>
|
||||
#include <sys/stat.h>
|
||||
|
||||
namespace PaddleOCR {
|
||||
|
||||
PaddleStructure::PaddleStructure() {
|
||||
if (FLAGS_layout) {
|
||||
this->layout_model_ = new StructureLayoutRecognizer(
|
||||
FLAGS_layout_model_dir, FLAGS_use_gpu, FLAGS_gpu_id, FLAGS_gpu_mem,
|
||||
FLAGS_cpu_threads, FLAGS_enable_mkldnn, FLAGS_layout_dict_path,
|
||||
FLAGS_use_tensorrt, FLAGS_precision, FLAGS_layout_score_threshold,
|
||||
FLAGS_layout_nms_threshold);
|
||||
}
|
||||
if (FLAGS_table) {
|
||||
this->recognizer_ = new StructureTableRecognizer(
|
||||
this->table_model_ = new StructureTableRecognizer(
|
||||
FLAGS_table_model_dir, FLAGS_use_gpu, FLAGS_gpu_id, FLAGS_gpu_mem,
|
||||
FLAGS_cpu_threads, FLAGS_enable_mkldnn, FLAGS_table_char_dict_path,
|
||||
FLAGS_use_tensorrt, FLAGS_precision, FLAGS_table_batch_num,
|
||||
|
@ -31,68 +36,63 @@ PaddleStructure::PaddleStructure() {
|
|||
}
|
||||
};
|
||||
|
||||
std::vector<std::vector<StructurePredictResult>>
|
||||
PaddleStructure::structure(std::vector<cv::String> cv_all_img_names,
|
||||
bool layout, bool table) {
|
||||
std::vector<double> time_info_det = {0, 0, 0};
|
||||
std::vector<double> time_info_rec = {0, 0, 0};
|
||||
std::vector<double> time_info_cls = {0, 0, 0};
|
||||
std::vector<double> time_info_table = {0, 0, 0};
|
||||
std::vector<StructurePredictResult>
|
||||
PaddleStructure::structure(cv::Mat srcimg, bool layout, bool table, bool ocr) {
|
||||
cv::Mat img;
|
||||
srcimg.copyTo(img);
|
||||
|
||||
std::vector<std::vector<StructurePredictResult>> structure_results;
|
||||
std::vector<StructurePredictResult> structure_results;
|
||||
|
||||
if (!Utility::PathExists(FLAGS_output) && FLAGS_det) {
|
||||
Utility::CreateDir(FLAGS_output);
|
||||
if (layout) {
|
||||
this->layout(img, structure_results);
|
||||
} else {
|
||||
StructurePredictResult res;
|
||||
res.type = "table";
|
||||
res.box = std::vector<float>(4, 0.0);
|
||||
res.box[2] = img.cols;
|
||||
res.box[3] = img.rows;
|
||||
structure_results.push_back(res);
|
||||
}
|
||||
for (int i = 0; i < cv_all_img_names.size(); ++i) {
|
||||
std::vector<StructurePredictResult> structure_result;
|
||||
cv::Mat srcimg = cv::imread(cv_all_img_names[i], cv::IMREAD_COLOR);
|
||||
if (!srcimg.data) {
|
||||
std::cerr << "[ERROR] image read failed! image path: "
|
||||
<< cv_all_img_names[i] << endl;
|
||||
exit(1);
|
||||
cv::Mat roi_img;
|
||||
for (int i = 0; i < structure_results.size(); i++) {
|
||||
// crop image
|
||||
roi_img = Utility::crop_image(img, structure_results[i].box);
|
||||
if (structure_results[i].type == "table" && table) {
|
||||
this->table(roi_img, structure_results[i]);
|
||||
} else if (ocr) {
|
||||
structure_results[i].text_res = this->ocr(roi_img, true, true, false);
|
||||
}
|
||||
if (layout) {
|
||||
} else {
|
||||
StructurePredictResult res;
|
||||
res.type = "table";
|
||||
res.box = std::vector<int>(4, 0);
|
||||
res.box[2] = srcimg.cols;
|
||||
res.box[3] = srcimg.rows;
|
||||
structure_result.push_back(res);
|
||||
}
|
||||
cv::Mat roi_img;
|
||||
for (int i = 0; i < structure_result.size(); i++) {
|
||||
// crop image
|
||||
roi_img = Utility::crop_image(srcimg, structure_result[i].box);
|
||||
if (structure_result[i].type == "table") {
|
||||
this->table(roi_img, structure_result[i], time_info_table,
|
||||
time_info_det, time_info_rec, time_info_cls);
|
||||
}
|
||||
}
|
||||
structure_results.push_back(structure_result);
|
||||
}
|
||||
|
||||
return structure_results;
|
||||
};
|
||||
|
||||
void PaddleStructure::layout(
|
||||
cv::Mat img, std::vector<StructurePredictResult> &structure_result) {
|
||||
std::vector<double> layout_times;
|
||||
this->layout_model_->Run(img, structure_result, layout_times);
|
||||
|
||||
this->time_info_layout[0] += layout_times[0];
|
||||
this->time_info_layout[1] += layout_times[1];
|
||||
this->time_info_layout[2] += layout_times[2];
|
||||
}
|
||||
|
||||
void PaddleStructure::table(cv::Mat img,
|
||||
StructurePredictResult &structure_result,
|
||||
std::vector<double> &time_info_table,
|
||||
std::vector<double> &time_info_det,
|
||||
std::vector<double> &time_info_rec,
|
||||
std::vector<double> &time_info_cls) {
|
||||
StructurePredictResult &structure_result) {
|
||||
// predict structure
|
||||
std::vector<std::vector<std::string>> structure_html_tags;
|
||||
std::vector<float> structure_scores(1, 0);
|
||||
std::vector<std::vector<std::vector<int>>> structure_boxes;
|
||||
std::vector<double> structure_imes;
|
||||
std::vector<double> structure_times;
|
||||
std::vector<cv::Mat> img_list;
|
||||
img_list.push_back(img);
|
||||
this->recognizer_->Run(img_list, structure_html_tags, structure_scores,
|
||||
structure_boxes, structure_imes);
|
||||
time_info_table[0] += structure_imes[0];
|
||||
time_info_table[1] += structure_imes[1];
|
||||
time_info_table[2] += structure_imes[2];
|
||||
|
||||
this->table_model_->Run(img_list, structure_html_tags, structure_scores,
|
||||
structure_boxes, structure_times);
|
||||
|
||||
this->time_info_table[0] += structure_times[0];
|
||||
this->time_info_table[1] += structure_times[1];
|
||||
this->time_info_table[2] += structure_times[2];
|
||||
|
||||
std::vector<OCRPredictResult> ocr_result;
|
||||
std::string html;
|
||||
|
@ -100,22 +100,22 @@ void PaddleStructure::table(cv::Mat img,
|
|||
|
||||
for (int i = 0; i < img_list.size(); i++) {
|
||||
// det
|
||||
this->det(img_list[i], ocr_result, time_info_det);
|
||||
this->det(img_list[i], ocr_result);
|
||||
// crop image
|
||||
std::vector<cv::Mat> rec_img_list;
|
||||
std::vector<int> ocr_box;
|
||||
for (int j = 0; j < ocr_result.size(); j++) {
|
||||
ocr_box = Utility::xyxyxyxy2xyxy(ocr_result[j].box);
|
||||
ocr_box[0] = max(0, ocr_box[0] - expand_pixel);
|
||||
ocr_box[1] = max(0, ocr_box[1] - expand_pixel),
|
||||
ocr_box[2] = min(img_list[i].cols, ocr_box[2] + expand_pixel);
|
||||
ocr_box[3] = min(img_list[i].rows, ocr_box[3] + expand_pixel);
|
||||
ocr_box[0] = std::max(0, ocr_box[0] - expand_pixel);
|
||||
ocr_box[1] = std::max(0, ocr_box[1] - expand_pixel),
|
||||
ocr_box[2] = std::min(img_list[i].cols, ocr_box[2] + expand_pixel);
|
||||
ocr_box[3] = std::min(img_list[i].rows, ocr_box[3] + expand_pixel);
|
||||
|
||||
cv::Mat crop_img = Utility::crop_image(img_list[i], ocr_box);
|
||||
rec_img_list.push_back(crop_img);
|
||||
}
|
||||
// rec
|
||||
this->rec(rec_img_list, ocr_result, time_info_rec);
|
||||
this->rec(rec_img_list, ocr_result);
|
||||
// rebuild table
|
||||
html = this->rebuild_table(structure_html_tags[i], structure_boxes[i],
|
||||
ocr_result);
|
||||
|
@ -130,8 +130,8 @@ PaddleStructure::rebuild_table(std::vector<std::string> structure_html_tags,
|
|||
std::vector<std::vector<int>> structure_boxes,
|
||||
std::vector<OCRPredictResult> &ocr_result) {
|
||||
// match text in same cell
|
||||
std::vector<std::vector<string>> matched(structure_boxes.size(),
|
||||
std::vector<std::string>());
|
||||
std::vector<std::vector<std::string>> matched(structure_boxes.size(),
|
||||
std::vector<std::string>());
|
||||
|
||||
std::vector<int> ocr_box;
|
||||
std::vector<int> structure_box;
|
||||
|
@ -150,7 +150,7 @@ PaddleStructure::rebuild_table(std::vector<std::string> structure_html_tags,
|
|||
structure_box = structure_boxes[j];
|
||||
}
|
||||
dis_list[j][0] = this->dis(ocr_box, structure_box);
|
||||
dis_list[j][1] = 1 - this->iou(ocr_box, structure_box);
|
||||
dis_list[j][1] = 1 - Utility::iou(ocr_box, structure_box);
|
||||
dis_list[j][2] = j;
|
||||
}
|
||||
// find min dis idx
|
||||
|
@ -216,28 +216,6 @@ PaddleStructure::rebuild_table(std::vector<std::string> structure_html_tags,
|
|||
return html_str;
|
||||
}
|
||||
|
||||
float PaddleStructure::iou(std::vector<int> &box1, std::vector<int> &box2) {
|
||||
int area1 = max(0, box1[2] - box1[0]) * max(0, box1[3] - box1[1]);
|
||||
int area2 = max(0, box2[2] - box2[0]) * max(0, box2[3] - box2[1]);
|
||||
|
||||
// computing the sum_area
|
||||
int sum_area = area1 + area2;
|
||||
|
||||
// find the each point of intersect rectangle
|
||||
int x1 = max(box1[0], box2[0]);
|
||||
int y1 = max(box1[1], box2[1]);
|
||||
int x2 = min(box1[2], box2[2]);
|
||||
int y2 = min(box1[3], box2[3]);
|
||||
|
||||
// judge if there is an intersect
|
||||
if (y1 >= y2 || x1 >= x2) {
|
||||
return 0.0;
|
||||
} else {
|
||||
int intersect = (x2 - x1) * (y2 - y1);
|
||||
return intersect / (sum_area - intersect + 0.00000001);
|
||||
}
|
||||
}
|
||||
|
||||
float PaddleStructure::dis(std::vector<int> &box1, std::vector<int> &box2) {
|
||||
int x1_1 = box1[0];
|
||||
int y1_1 = box1[1];
|
||||
|
@ -253,12 +231,64 @@ float PaddleStructure::dis(std::vector<int> &box1, std::vector<int> &box2) {
|
|||
abs(x1_2 - x1_1) + abs(y1_2 - y1_1) + abs(x2_2 - x2_1) + abs(y2_2 - y2_1);
|
||||
float dis_2 = abs(x1_2 - x1_1) + abs(y1_2 - y1_1);
|
||||
float dis_3 = abs(x2_2 - x2_1) + abs(y2_2 - y2_1);
|
||||
return dis + min(dis_2, dis_3);
|
||||
return dis + std::min(dis_2, dis_3);
|
||||
}
|
||||
|
||||
void PaddleStructure::reset_timer() {
|
||||
this->time_info_det = {0, 0, 0};
|
||||
this->time_info_rec = {0, 0, 0};
|
||||
this->time_info_cls = {0, 0, 0};
|
||||
this->time_info_table = {0, 0, 0};
|
||||
this->time_info_layout = {0, 0, 0};
|
||||
}
|
||||
|
||||
void PaddleStructure::benchmark_log(int img_num) {
|
||||
if (this->time_info_det[0] + this->time_info_det[1] + this->time_info_det[2] >
|
||||
0) {
|
||||
AutoLogger autolog_det("ocr_det", FLAGS_use_gpu, FLAGS_use_tensorrt,
|
||||
FLAGS_enable_mkldnn, FLAGS_cpu_threads, 1, "dynamic",
|
||||
FLAGS_precision, this->time_info_det, img_num);
|
||||
autolog_det.report();
|
||||
}
|
||||
if (this->time_info_rec[0] + this->time_info_rec[1] + this->time_info_rec[2] >
|
||||
0) {
|
||||
AutoLogger autolog_rec("ocr_rec", FLAGS_use_gpu, FLAGS_use_tensorrt,
|
||||
FLAGS_enable_mkldnn, FLAGS_cpu_threads,
|
||||
FLAGS_rec_batch_num, "dynamic", FLAGS_precision,
|
||||
this->time_info_rec, img_num);
|
||||
autolog_rec.report();
|
||||
}
|
||||
if (this->time_info_cls[0] + this->time_info_cls[1] + this->time_info_cls[2] >
|
||||
0) {
|
||||
AutoLogger autolog_cls("ocr_cls", FLAGS_use_gpu, FLAGS_use_tensorrt,
|
||||
FLAGS_enable_mkldnn, FLAGS_cpu_threads,
|
||||
FLAGS_cls_batch_num, "dynamic", FLAGS_precision,
|
||||
this->time_info_cls, img_num);
|
||||
autolog_cls.report();
|
||||
}
|
||||
if (this->time_info_table[0] + this->time_info_table[1] +
|
||||
this->time_info_table[2] >
|
||||
0) {
|
||||
AutoLogger autolog_table("table", FLAGS_use_gpu, FLAGS_use_tensorrt,
|
||||
FLAGS_enable_mkldnn, FLAGS_cpu_threads,
|
||||
FLAGS_cls_batch_num, "dynamic", FLAGS_precision,
|
||||
this->time_info_table, img_num);
|
||||
autolog_table.report();
|
||||
}
|
||||
if (this->time_info_layout[0] + this->time_info_layout[1] +
|
||||
this->time_info_layout[2] >
|
||||
0) {
|
||||
AutoLogger autolog_layout("layout", FLAGS_use_gpu, FLAGS_use_tensorrt,
|
||||
FLAGS_enable_mkldnn, FLAGS_cpu_threads,
|
||||
FLAGS_cls_batch_num, "dynamic", FLAGS_precision,
|
||||
this->time_info_layout, img_num);
|
||||
autolog_layout.report();
|
||||
}
|
||||
}
|
||||
|
||||
PaddleStructure::~PaddleStructure() {
|
||||
if (this->recognizer_ != nullptr) {
|
||||
delete this->recognizer_;
|
||||
if (this->table_model_ != nullptr) {
|
||||
delete this->table_model_;
|
||||
}
|
||||
};
|
||||
|
||||
|
|
|
@ -12,7 +12,6 @@
|
|||
// See the License for the specific language governing permissions and
|
||||
// limitations under the License.
|
||||
|
||||
#include <include/clipper.h>
|
||||
#include <include/postprocess_op.h>
|
||||
|
||||
namespace PaddleOCR {
|
||||
|
@ -431,7 +430,7 @@ void TablePostProcessor::Run(
|
|||
}
|
||||
}
|
||||
score /= count;
|
||||
if (isnan(score) || rec_boxes.size() == 0) {
|
||||
if (std::isnan(score) || rec_boxes.size() == 0) {
|
||||
score = -1;
|
||||
}
|
||||
rec_scores.push_back(score);
|
||||
|
@ -440,4 +439,137 @@ void TablePostProcessor::Run(
|
|||
}
|
||||
}
|
||||
|
||||
void PicodetPostProcessor::init(std::string label_path,
|
||||
const double score_threshold,
|
||||
const double nms_threshold,
|
||||
const std::vector<int> &fpn_stride) {
|
||||
this->label_list_ = Utility::ReadDict(label_path);
|
||||
this->score_threshold_ = score_threshold;
|
||||
this->nms_threshold_ = nms_threshold;
|
||||
this->num_class_ = label_list_.size();
|
||||
this->fpn_stride_ = fpn_stride;
|
||||
}
|
||||
|
||||
void PicodetPostProcessor::Run(std::vector<StructurePredictResult> &results,
|
||||
std::vector<std::vector<float>> outs,
|
||||
std::vector<int> ori_shape,
|
||||
std::vector<int> resize_shape, int reg_max) {
|
||||
int in_h = resize_shape[0];
|
||||
int in_w = resize_shape[1];
|
||||
float scale_factor_h = resize_shape[0] / float(ori_shape[0]);
|
||||
float scale_factor_w = resize_shape[1] / float(ori_shape[1]);
|
||||
|
||||
std::vector<std::vector<StructurePredictResult>> bbox_results;
|
||||
bbox_results.resize(this->num_class_);
|
||||
for (int i = 0; i < this->fpn_stride_.size(); ++i) {
|
||||
int feature_h = std::ceil((float)in_h / this->fpn_stride_[i]);
|
||||
int feature_w = std::ceil((float)in_w / this->fpn_stride_[i]);
|
||||
for (int idx = 0; idx < feature_h * feature_w; idx++) {
|
||||
// score and label
|
||||
float score = 0;
|
||||
int cur_label = 0;
|
||||
for (int label = 0; label < this->num_class_; label++) {
|
||||
if (outs[i][idx * this->num_class_ + label] > score) {
|
||||
score = outs[i][idx * this->num_class_ + label];
|
||||
cur_label = label;
|
||||
}
|
||||
}
|
||||
// bbox
|
||||
if (score > this->score_threshold_) {
|
||||
int row = idx / feature_w;
|
||||
int col = idx % feature_w;
|
||||
std::vector<float> bbox_pred(
|
||||
outs[i + this->fpn_stride_.size()].begin() + idx * 4 * reg_max,
|
||||
outs[i + this->fpn_stride_.size()].begin() +
|
||||
(idx + 1) * 4 * reg_max);
|
||||
bbox_results[cur_label].push_back(
|
||||
this->disPred2Bbox(bbox_pred, cur_label, score, col, row,
|
||||
this->fpn_stride_[i], resize_shape, reg_max));
|
||||
}
|
||||
}
|
||||
}
|
||||
for (int i = 0; i < bbox_results.size(); i++) {
|
||||
bool flag = bbox_results[i].size() <= 0;
|
||||
}
|
||||
for (int i = 0; i < bbox_results.size(); i++) {
|
||||
bool flag = bbox_results[i].size() <= 0;
|
||||
if (bbox_results[i].size() <= 0) {
|
||||
continue;
|
||||
}
|
||||
this->nms(bbox_results[i], this->nms_threshold_);
|
||||
for (auto box : bbox_results[i]) {
|
||||
box.box[0] = box.box[0] / scale_factor_w;
|
||||
box.box[2] = box.box[2] / scale_factor_w;
|
||||
box.box[1] = box.box[1] / scale_factor_h;
|
||||
box.box[3] = box.box[3] / scale_factor_h;
|
||||
results.push_back(box);
|
||||
}
|
||||
}
|
||||
}
|
||||
|
||||
StructurePredictResult
|
||||
PicodetPostProcessor::disPred2Bbox(std::vector<float> bbox_pred, int label,
|
||||
float score, int x, int y, int stride,
|
||||
std::vector<int> im_shape, int reg_max) {
|
||||
float ct_x = (x + 0.5) * stride;
|
||||
float ct_y = (y + 0.5) * stride;
|
||||
std::vector<float> dis_pred;
|
||||
dis_pred.resize(4);
|
||||
for (int i = 0; i < 4; i++) {
|
||||
float dis = 0;
|
||||
std::vector<float> bbox_pred_i(bbox_pred.begin() + i * reg_max,
|
||||
bbox_pred.begin() + (i + 1) * reg_max);
|
||||
std::vector<float> dis_after_sm =
|
||||
Utility::activation_function_softmax(bbox_pred_i);
|
||||
for (int j = 0; j < reg_max; j++) {
|
||||
dis += j * dis_after_sm[j];
|
||||
}
|
||||
dis *= stride;
|
||||
dis_pred[i] = dis;
|
||||
}
|
||||
|
||||
float xmin = (std::max)(ct_x - dis_pred[0], .0f);
|
||||
float ymin = (std::max)(ct_y - dis_pred[1], .0f);
|
||||
float xmax = (std::min)(ct_x + dis_pred[2], (float)im_shape[1]);
|
||||
float ymax = (std::min)(ct_y + dis_pred[3], (float)im_shape[0]);
|
||||
|
||||
StructurePredictResult result_item;
|
||||
result_item.box = {xmin, ymin, xmax, ymax};
|
||||
result_item.type = this->label_list_[label];
|
||||
result_item.confidence = score;
|
||||
|
||||
return result_item;
|
||||
}
|
||||
|
||||
void PicodetPostProcessor::nms(std::vector<StructurePredictResult> &input_boxes,
|
||||
float nms_threshold) {
|
||||
std::sort(input_boxes.begin(), input_boxes.end(),
|
||||
[](StructurePredictResult a, StructurePredictResult b) {
|
||||
return a.confidence > b.confidence;
|
||||
});
|
||||
std::vector<int> picked(input_boxes.size(), 1);
|
||||
|
||||
for (int i = 0; i < input_boxes.size(); ++i) {
|
||||
if (picked[i] == 0) {
|
||||
continue;
|
||||
}
|
||||
for (int j = i + 1; j < input_boxes.size(); ++j) {
|
||||
if (picked[j] == 0) {
|
||||
continue;
|
||||
}
|
||||
float iou = Utility::iou(input_boxes[i].box, input_boxes[j].box);
|
||||
if (iou > nms_threshold) {
|
||||
picked[j] = 0;
|
||||
}
|
||||
}
|
||||
}
|
||||
std::vector<StructurePredictResult> input_boxes_nms;
|
||||
for (int i = 0; i < input_boxes.size(); ++i) {
|
||||
if (picked[i] == 1) {
|
||||
input_boxes_nms.push_back(input_boxes[i]);
|
||||
}
|
||||
}
|
||||
input_boxes = input_boxes_nms;
|
||||
}
|
||||
|
||||
} // namespace PaddleOCR
|
||||
|
|
|
@ -12,21 +12,6 @@
|
|||
// See the License for the specific language governing permissions and
|
||||
// limitations under the License.
|
||||
|
||||
#include "opencv2/core.hpp"
|
||||
#include "opencv2/imgcodecs.hpp"
|
||||
#include "opencv2/imgproc.hpp"
|
||||
#include "paddle_api.h"
|
||||
#include "paddle_inference_api.h"
|
||||
#include <chrono>
|
||||
#include <iomanip>
|
||||
#include <iostream>
|
||||
#include <ostream>
|
||||
#include <vector>
|
||||
|
||||
#include <cstring>
|
||||
#include <fstream>
|
||||
#include <numeric>
|
||||
|
||||
#include <include/preprocess_op.h>
|
||||
|
||||
namespace PaddleOCR {
|
||||
|
@ -69,13 +54,13 @@ void Normalize::Run(cv::Mat *im, const std::vector<float> &mean,
|
|||
}
|
||||
|
||||
void ResizeImgType0::Run(const cv::Mat &img, cv::Mat &resize_img,
|
||||
string limit_type, int limit_side_len, float &ratio_h,
|
||||
float &ratio_w, bool use_tensorrt) {
|
||||
std::string limit_type, int limit_side_len,
|
||||
float &ratio_h, float &ratio_w, bool use_tensorrt) {
|
||||
int w = img.cols;
|
||||
int h = img.rows;
|
||||
float ratio = 1.f;
|
||||
if (limit_type == "min") {
|
||||
int min_wh = min(h, w);
|
||||
int min_wh = std::min(h, w);
|
||||
if (min_wh < limit_side_len) {
|
||||
if (h < w) {
|
||||
ratio = float(limit_side_len) / float(h);
|
||||
|
@ -84,7 +69,7 @@ void ResizeImgType0::Run(const cv::Mat &img, cv::Mat &resize_img,
|
|||
}
|
||||
}
|
||||
} else {
|
||||
int max_wh = max(h, w);
|
||||
int max_wh = std::max(h, w);
|
||||
if (max_wh > limit_side_len) {
|
||||
if (h > w) {
|
||||
ratio = float(limit_side_len) / float(h);
|
||||
|
@ -97,8 +82,8 @@ void ResizeImgType0::Run(const cv::Mat &img, cv::Mat &resize_img,
|
|||
int resize_h = int(float(h) * ratio);
|
||||
int resize_w = int(float(w) * ratio);
|
||||
|
||||
resize_h = max(int(round(float(resize_h) / 32) * 32), 32);
|
||||
resize_w = max(int(round(float(resize_w) / 32) * 32), 32);
|
||||
resize_h = std::max(int(round(float(resize_h) / 32) * 32), 32);
|
||||
resize_w = std::max(int(round(float(resize_w) / 32) * 32), 32);
|
||||
|
||||
cv::resize(img, resize_img, cv::Size(resize_w, resize_h));
|
||||
ratio_h = float(resize_h) / float(h);
|
||||
|
@ -175,4 +160,9 @@ void TablePadImg::Run(const cv::Mat &img, cv::Mat &resize_img,
|
|||
cv::BORDER_CONSTANT, cv::Scalar(0, 0, 0));
|
||||
}
|
||||
|
||||
void Resize::Run(const cv::Mat &img, cv::Mat &resize_img, const int h,
|
||||
const int w) {
|
||||
cv::resize(img, resize_img, cv::Size(w, h));
|
||||
}
|
||||
|
||||
} // namespace PaddleOCR
|
||||
|
|
|
@ -0,0 +1,149 @@
|
|||
// Copyright (c) 2020 PaddlePaddle Authors. All Rights Reserved.
|
||||
//
|
||||
// Licensed under the Apache License, Version 2.0 (the "License");
|
||||
// you may not use this file except in compliance with the License.
|
||||
// You may obtain a copy of the License at
|
||||
//
|
||||
// http://www.apache.org/licenses/LICENSE-2.0
|
||||
//
|
||||
// Unless required by applicable law or agreed to in writing, software
|
||||
// distributed under the License is distributed on an "AS IS" BASIS,
|
||||
// WITHOUT WARRANTIES OR CONDITIONS OF ANY KIND, either express or implied.
|
||||
// See the License for the specific language governing permissions and
|
||||
// limitations under the License.
|
||||
|
||||
#include <include/structure_layout.h>
|
||||
|
||||
namespace PaddleOCR {
|
||||
|
||||
void StructureLayoutRecognizer::Run(cv::Mat img,
|
||||
std::vector<StructurePredictResult> &result,
|
||||
std::vector<double> ×) {
|
||||
std::chrono::duration<float> preprocess_diff =
|
||||
std::chrono::steady_clock::now() - std::chrono::steady_clock::now();
|
||||
std::chrono::duration<float> inference_diff =
|
||||
std::chrono::steady_clock::now() - std::chrono::steady_clock::now();
|
||||
std::chrono::duration<float> postprocess_diff =
|
||||
std::chrono::steady_clock::now() - std::chrono::steady_clock::now();
|
||||
|
||||
// preprocess
|
||||
auto preprocess_start = std::chrono::steady_clock::now();
|
||||
|
||||
cv::Mat srcimg;
|
||||
img.copyTo(srcimg);
|
||||
cv::Mat resize_img;
|
||||
this->resize_op_.Run(srcimg, resize_img, 800, 608);
|
||||
this->normalize_op_.Run(&resize_img, this->mean_, this->scale_,
|
||||
this->is_scale_);
|
||||
|
||||
std::vector<float> input(1 * 3 * resize_img.rows * resize_img.cols, 0.0f);
|
||||
this->permute_op_.Run(&resize_img, input.data());
|
||||
auto preprocess_end = std::chrono::steady_clock::now();
|
||||
preprocess_diff += preprocess_end - preprocess_start;
|
||||
|
||||
// inference.
|
||||
auto input_names = this->predictor_->GetInputNames();
|
||||
auto input_t = this->predictor_->GetInputHandle(input_names[0]);
|
||||
input_t->Reshape({1, 3, resize_img.rows, resize_img.cols});
|
||||
auto inference_start = std::chrono::steady_clock::now();
|
||||
input_t->CopyFromCpu(input.data());
|
||||
|
||||
this->predictor_->Run();
|
||||
|
||||
// Get output tensor
|
||||
std::vector<std::vector<float>> out_tensor_list;
|
||||
std::vector<std::vector<int>> output_shape_list;
|
||||
auto output_names = this->predictor_->GetOutputNames();
|
||||
for (int j = 0; j < output_names.size(); j++) {
|
||||
auto output_tensor = this->predictor_->GetOutputHandle(output_names[j]);
|
||||
std::vector<int> output_shape = output_tensor->shape();
|
||||
int out_num = std::accumulate(output_shape.begin(), output_shape.end(), 1,
|
||||
std::multiplies<int>());
|
||||
output_shape_list.push_back(output_shape);
|
||||
|
||||
std::vector<float> out_data;
|
||||
out_data.resize(out_num);
|
||||
output_tensor->CopyToCpu(out_data.data());
|
||||
out_tensor_list.push_back(out_data);
|
||||
}
|
||||
auto inference_end = std::chrono::steady_clock::now();
|
||||
inference_diff += inference_end - inference_start;
|
||||
|
||||
// postprocess
|
||||
auto postprocess_start = std::chrono::steady_clock::now();
|
||||
|
||||
std::vector<int> bbox_num;
|
||||
int reg_max = 0;
|
||||
for (int i = 0; i < out_tensor_list.size(); i++) {
|
||||
if (i == this->post_processor_.fpn_stride_.size()) {
|
||||
reg_max = output_shape_list[i][2] / 4;
|
||||
break;
|
||||
}
|
||||
}
|
||||
std::vector<int> ori_shape = {srcimg.rows, srcimg.cols};
|
||||
std::vector<int> resize_shape = {resize_img.rows, resize_img.cols};
|
||||
this->post_processor_.Run(result, out_tensor_list, ori_shape, resize_shape,
|
||||
reg_max);
|
||||
bbox_num.push_back(result.size());
|
||||
|
||||
auto postprocess_end = std::chrono::steady_clock::now();
|
||||
postprocess_diff += postprocess_end - postprocess_start;
|
||||
times.push_back(double(preprocess_diff.count() * 1000));
|
||||
times.push_back(double(inference_diff.count() * 1000));
|
||||
times.push_back(double(postprocess_diff.count() * 1000));
|
||||
}
|
||||
|
||||
void StructureLayoutRecognizer::LoadModel(const std::string &model_dir) {
|
||||
paddle_infer::Config config;
|
||||
if (Utility::PathExists(model_dir + "/inference.pdmodel") &&
|
||||
Utility::PathExists(model_dir + "/inference.pdiparams")) {
|
||||
config.SetModel(model_dir + "/inference.pdmodel",
|
||||
model_dir + "/inference.pdiparams");
|
||||
} else if (Utility::PathExists(model_dir + "/model.pdmodel") &&
|
||||
Utility::PathExists(model_dir + "/model.pdiparams")) {
|
||||
config.SetModel(model_dir + "/model.pdmodel",
|
||||
model_dir + "/model.pdiparams");
|
||||
} else {
|
||||
std::cerr << "[ERROR] not find model.pdiparams or inference.pdiparams in "
|
||||
<< model_dir << std::endl;
|
||||
exit(1);
|
||||
}
|
||||
|
||||
if (this->use_gpu_) {
|
||||
config.EnableUseGpu(this->gpu_mem_, this->gpu_id_);
|
||||
if (this->use_tensorrt_) {
|
||||
auto precision = paddle_infer::Config::Precision::kFloat32;
|
||||
if (this->precision_ == "fp16") {
|
||||
precision = paddle_infer::Config::Precision::kHalf;
|
||||
}
|
||||
if (this->precision_ == "int8") {
|
||||
precision = paddle_infer::Config::Precision::kInt8;
|
||||
}
|
||||
config.EnableTensorRtEngine(1 << 20, 10, 3, precision, false, false);
|
||||
if (!Utility::PathExists("./trt_layout_shape.txt")) {
|
||||
config.CollectShapeRangeInfo("./trt_layout_shape.txt");
|
||||
} else {
|
||||
config.EnableTunedTensorRtDynamicShape("./trt_layout_shape.txt", true);
|
||||
}
|
||||
}
|
||||
} else {
|
||||
config.DisableGpu();
|
||||
if (this->use_mkldnn_) {
|
||||
config.EnableMKLDNN();
|
||||
}
|
||||
config.SetCpuMathLibraryNumThreads(this->cpu_math_library_num_threads_);
|
||||
}
|
||||
|
||||
// false for zero copy tensor
|
||||
config.SwitchUseFeedFetchOps(false);
|
||||
// true for multiple input
|
||||
config.SwitchSpecifyInputNames(true);
|
||||
|
||||
config.SwitchIrOptim(true);
|
||||
|
||||
config.EnableMemoryOptim();
|
||||
config.DisableGlogInfo();
|
||||
|
||||
this->predictor_ = paddle_infer::CreatePredictor(config);
|
||||
}
|
||||
} // namespace PaddleOCR
|
|
@ -34,7 +34,7 @@ void StructureTableRecognizer::Run(
|
|||
beg_img_no += this->table_batch_num_) {
|
||||
// preprocess
|
||||
auto preprocess_start = std::chrono::steady_clock::now();
|
||||
int end_img_no = min(img_num, beg_img_no + this->table_batch_num_);
|
||||
int end_img_no = std::min(img_num, beg_img_no + this->table_batch_num_);
|
||||
int batch_num = end_img_no - beg_img_no;
|
||||
std::vector<cv::Mat> norm_img_batch;
|
||||
std::vector<int> width_list;
|
||||
|
@ -118,7 +118,7 @@ void StructureTableRecognizer::Run(
|
|||
}
|
||||
|
||||
void StructureTableRecognizer::LoadModel(const std::string &model_dir) {
|
||||
AnalysisConfig config;
|
||||
paddle_infer::Config config;
|
||||
config.SetModel(model_dir + "/inference.pdmodel",
|
||||
model_dir + "/inference.pdiparams");
|
||||
|
||||
|
@ -133,6 +133,11 @@ void StructureTableRecognizer::LoadModel(const std::string &model_dir) {
|
|||
precision = paddle_infer::Config::Precision::kInt8;
|
||||
}
|
||||
config.EnableTensorRtEngine(1 << 20, 10, 3, precision, false, false);
|
||||
if (!Utility::PathExists("./trt_table_shape.txt")) {
|
||||
config.CollectShapeRangeInfo("./trt_table_shape.txt");
|
||||
} else {
|
||||
config.EnableTunedTensorRtDynamicShape("./trt_table_shape.txt", true);
|
||||
}
|
||||
}
|
||||
} else {
|
||||
config.DisableGpu();
|
||||
|
@ -152,6 +157,6 @@ void StructureTableRecognizer::LoadModel(const std::string &model_dir) {
|
|||
config.EnableMemoryOptim();
|
||||
config.DisableGlogInfo();
|
||||
|
||||
this->predictor_ = CreatePredictor(config);
|
||||
this->predictor_ = paddle_infer::CreatePredictor(config);
|
||||
}
|
||||
} // namespace PaddleOCR
|
||||
|
|
|
@ -70,6 +70,7 @@ void Utility::VisualizeBboxes(const cv::Mat &srcimg,
|
|||
const std::string &save_path) {
|
||||
cv::Mat img_vis;
|
||||
srcimg.copyTo(img_vis);
|
||||
img_vis = crop_image(img_vis, structure_result.box);
|
||||
for (int n = 0; n < structure_result.cell_box.size(); n++) {
|
||||
if (structure_result.cell_box[n].size() == 8) {
|
||||
cv::Point rook_points[4];
|
||||
|
@ -280,23 +281,29 @@ void Utility::print_result(const std::vector<OCRPredictResult> &ocr_result) {
|
|||
}
|
||||
}
|
||||
|
||||
cv::Mat Utility::crop_image(cv::Mat &img, std::vector<int> &area) {
|
||||
cv::Mat Utility::crop_image(cv::Mat &img, const std::vector<int> &box) {
|
||||
cv::Mat crop_im;
|
||||
int crop_x1 = std::max(0, area[0]);
|
||||
int crop_y1 = std::max(0, area[1]);
|
||||
int crop_x2 = std::min(img.cols - 1, area[2] - 1);
|
||||
int crop_y2 = std::min(img.rows - 1, area[3] - 1);
|
||||
int crop_x1 = std::max(0, box[0]);
|
||||
int crop_y1 = std::max(0, box[1]);
|
||||
int crop_x2 = std::min(img.cols - 1, box[2] - 1);
|
||||
int crop_y2 = std::min(img.rows - 1, box[3] - 1);
|
||||
|
||||
crop_im = cv::Mat::zeros(area[3] - area[1], area[2] - area[0], 16);
|
||||
crop_im = cv::Mat::zeros(box[3] - box[1], box[2] - box[0], 16);
|
||||
cv::Mat crop_im_window =
|
||||
crop_im(cv::Range(crop_y1 - area[1], crop_y2 + 1 - area[1]),
|
||||
cv::Range(crop_x1 - area[0], crop_x2 + 1 - area[0]));
|
||||
crop_im(cv::Range(crop_y1 - box[1], crop_y2 + 1 - box[1]),
|
||||
cv::Range(crop_x1 - box[0], crop_x2 + 1 - box[0]));
|
||||
cv::Mat roi_img =
|
||||
img(cv::Range(crop_y1, crop_y2 + 1), cv::Range(crop_x1, crop_x2 + 1));
|
||||
crop_im_window += roi_img;
|
||||
return crop_im;
|
||||
}
|
||||
|
||||
cv::Mat Utility::crop_image(cv::Mat &img, const std::vector<float> &box) {
|
||||
std::vector<int> box_int = {(int)box[0], (int)box[1], (int)box[2],
|
||||
(int)box[3]};
|
||||
return crop_image(img, box_int);
|
||||
}
|
||||
|
||||
void Utility::sorted_boxes(std::vector<OCRPredictResult> &ocr_result) {
|
||||
std::sort(ocr_result.begin(), ocr_result.end(), Utility::comparison_box);
|
||||
if (ocr_result.size() > 0) {
|
||||
|
@ -341,4 +348,78 @@ std::vector<int> Utility::xyxyxyxy2xyxy(std::vector<int> &box) {
|
|||
return box1;
|
||||
}
|
||||
|
||||
float Utility::fast_exp(float x) {
|
||||
union {
|
||||
uint32_t i;
|
||||
float f;
|
||||
} v{};
|
||||
v.i = (1 << 23) * (1.4426950409 * x + 126.93490512f);
|
||||
return v.f;
|
||||
}
|
||||
|
||||
std::vector<float>
|
||||
Utility::activation_function_softmax(std::vector<float> &src) {
|
||||
int length = src.size();
|
||||
std::vector<float> dst;
|
||||
dst.resize(length);
|
||||
const float alpha = float(*std::max_element(&src[0], &src[0 + length]));
|
||||
float denominator{0};
|
||||
|
||||
for (int i = 0; i < length; ++i) {
|
||||
dst[i] = fast_exp(src[i] - alpha);
|
||||
denominator += dst[i];
|
||||
}
|
||||
|
||||
for (int i = 0; i < length; ++i) {
|
||||
dst[i] /= denominator;
|
||||
}
|
||||
return dst;
|
||||
}
|
||||
|
||||
float Utility::iou(std::vector<int> &box1, std::vector<int> &box2) {
|
||||
int area1 = std::max(0, box1[2] - box1[0]) * std::max(0, box1[3] - box1[1]);
|
||||
int area2 = std::max(0, box2[2] - box2[0]) * std::max(0, box2[3] - box2[1]);
|
||||
|
||||
// computing the sum_area
|
||||
int sum_area = area1 + area2;
|
||||
|
||||
// find the each point of intersect rectangle
|
||||
int x1 = std::max(box1[0], box2[0]);
|
||||
int y1 = std::max(box1[1], box2[1]);
|
||||
int x2 = std::min(box1[2], box2[2]);
|
||||
int y2 = std::min(box1[3], box2[3]);
|
||||
|
||||
// judge if there is an intersect
|
||||
if (y1 >= y2 || x1 >= x2) {
|
||||
return 0.0;
|
||||
} else {
|
||||
int intersect = (x2 - x1) * (y2 - y1);
|
||||
return intersect / (sum_area - intersect + 0.00000001);
|
||||
}
|
||||
}
|
||||
|
||||
float Utility::iou(std::vector<float> &box1, std::vector<float> &box2) {
|
||||
float area1 = std::max((float)0.0, box1[2] - box1[0]) *
|
||||
std::max((float)0.0, box1[3] - box1[1]);
|
||||
float area2 = std::max((float)0.0, box2[2] - box2[0]) *
|
||||
std::max((float)0.0, box2[3] - box2[1]);
|
||||
|
||||
// computing the sum_area
|
||||
float sum_area = area1 + area2;
|
||||
|
||||
// find the each point of intersect rectangle
|
||||
float x1 = std::max(box1[0], box2[0]);
|
||||
float y1 = std::max(box1[1], box2[1]);
|
||||
float x2 = std::min(box1[2], box2[2]);
|
||||
float y2 = std::min(box1[3], box2[3]);
|
||||
|
||||
// judge if there is an intersect
|
||||
if (y1 >= y2 || x1 >= x2) {
|
||||
return 0.0;
|
||||
} else {
|
||||
float intersect = (x2 - x1) * (y2 - y1);
|
||||
return intersect / (sum_area - intersect + 0.00000001);
|
||||
}
|
||||
}
|
||||
|
||||
} // namespace PaddleOCR
|
|
@ -30,7 +30,7 @@
|
|||
|模型|骨干网络|任务|配置文件|hmean|下载链接|
|
||||
| --- | --- |--|--- | --- | --- |
|
||||
|LayoutXLM|LayoutXLM-base|SER |[ser_layoutxlm_xfund_zh.yml](../../configs/kie/layoutlm_series/ser_layoutxlm_xfund_zh.yml)|90.38%|[训练模型](https://paddleocr.bj.bcebos.com/pplayout/ser_LayoutXLM_xfun_zh.tar)/[推理模型](https://paddleocr.bj.bcebos.com/pplayout/ser_LayoutXLM_xfun_zh_infer.tar)|
|
||||
|LayoutXLM|LayoutXLM-base|RE | [re_layoutxlm_xfund_zh.yml](../../configs/kie/layoutlm_series/re_layoutxlm_xfund_zh.yml)|74.83%|[训练模型](https://paddleocr.bj.bcebos.com/pplayout/re_LayoutXLM_xfun_zh.tar)/[推理模型(coming soon)]()|
|
||||
|LayoutXLM|LayoutXLM-base|RE | [re_layoutxlm_xfund_zh.yml](../../configs/kie/layoutlm_series/re_layoutxlm_xfund_zh.yml)|74.83%|[训练模型](https://paddleocr.bj.bcebos.com/pplayout/re_LayoutXLM_xfun_zh.tar)/[推理模型](https://paddleocr.bj.bcebos.com/pplayout/re_LayoutXLM_xfun_zh_infer.tar)|
|
||||
|
||||
<a name="2"></a>
|
||||
|
||||
|
@ -52,14 +52,14 @@
|
|||
|
||||
### 4.1 Python推理
|
||||
|
||||
**注:** 目前RE任务推理过程仍在适配中,下面以SER任务为例,介绍基于LayoutXLM模型的关键信息抽取过程。
|
||||
- SER
|
||||
|
||||
首先将训练得到的模型转换成inference model。LayoutXLM模型在XFUND_zh数据集上训练的模型为例([模型下载地址](https://paddleocr.bj.bcebos.com/pplayout/ser_LayoutXLM_xfun_zh.tar)),可以使用下面的命令进行转换。
|
||||
|
||||
``` bash
|
||||
wget https://paddleocr.bj.bcebos.com/pplayout/ser_LayoutXLM_xfun_zh.tar
|
||||
tar -xf ser_LayoutXLM_xfun_zh.tar
|
||||
python3 tools/export_model.py -c configs/kie/layoutlm_series/ser_layoutxlm_xfund_zh.yml -o Architecture.Backbone.checkpoints=./ser_LayoutXLM_xfun_zh/best_accuracy Global.save_inference_dir=./inference/ser_layoutxlm
|
||||
python3 tools/export_model.py -c configs/kie/layoutlm_series/ser_layoutxlm_xfund_zh.yml -o Architecture.Backbone.checkpoints=./ser_LayoutXLM_xfun_zh Global.save_inference_dir=./inference/ser_layoutxlm_infer
|
||||
```
|
||||
|
||||
LayoutXLM模型基于SER任务进行推理,可以执行如下命令:
|
||||
|
@ -80,6 +80,34 @@ SER可视化结果默认保存到`./output`文件夹里面,结果示例如下
|
|||
<img src="../../ppstructure/docs/kie/result_ser/zh_val_42_ser.jpg" width="800">
|
||||
</div>
|
||||
|
||||
- RE
|
||||
|
||||
首先将训练得到的模型转换成inference model。LayoutXLM模型在XFUND_zh数据集上训练的模型为例([模型下载地址](https://paddleocr.bj.bcebos.com/pplayout/re_LayoutXLM_xfun_zh.tar)),可以使用下面的命令进行转换。
|
||||
|
||||
``` bash
|
||||
wget https://paddleocr.bj.bcebos.com/pplayout/re_LayoutXLM_xfun_zh.tar
|
||||
tar -xf re_LayoutXLM_xfun_zh.tar
|
||||
python3 tools/export_model.py -c configs/kie/layoutlm_series/re_layoutxlm_xfund_zh.yml -o Architecture.Backbone.checkpoints=./re_LayoutXLM_xfun_zh Global.save_inference_dir=./inference/ser_layoutxlm_infer
|
||||
```
|
||||
|
||||
LayoutXLM模型基于RE任务进行推理,可以执行如下命令:
|
||||
|
||||
```bash
|
||||
cd ppstructure
|
||||
python3 kie/predict_kie_token_ser_re.py \
|
||||
--kie_algorithm=LayoutXLM \
|
||||
--re_model_dir=../inference/re_layoutxlm_infer \
|
||||
--ser_model_dir=../inference/ser_layoutxlm_infer \
|
||||
--image_dir=./docs/kie/input/zh_val_42.jpg \
|
||||
--ser_dict_path=../train_data/XFUND/class_list_xfun.txt \
|
||||
--vis_font_path=../doc/fonts/simfang.ttf
|
||||
```
|
||||
|
||||
RE可视化结果默认保存到`./output`文件夹里面,结果示例如下:
|
||||
|
||||
<div align="center">
|
||||
<img src="../../ppstructure/docs/kie/result_re/zh_val_42_re.jpg" width="800">
|
||||
</div>
|
||||
|
||||
<a name="4-2"></a>
|
||||
### 4.2 C++推理部署
|
||||
|
|
|
@ -23,7 +23,7 @@ VI-LayoutXLM基于LayoutXLM进行改进,在下游任务训练过程中,去
|
|||
|模型|骨干网络|任务|配置文件|hmean|下载链接|
|
||||
| --- | --- |---| --- | --- | --- |
|
||||
|VI-LayoutXLM |VI-LayoutXLM-base | SER |[ser_vi_layoutxlm_xfund_zh_udml.yml](../../configs/kie/vi_layoutxlm/ser_vi_layoutxlm_xfund_zh_udml.yml)|93.19%|[训练模型](https://paddleocr.bj.bcebos.com/ppstructure/models/vi_layoutxlm/ser_vi_layoutxlm_xfund_pretrained.tar)/[推理模型](https://paddleocr.bj.bcebos.com/ppstructure/models/vi_layoutxlm/ser_vi_layoutxlm_xfund_infer.tar)|
|
||||
|VI-LayoutXLM |VI-LayoutXLM-base |RE | [re_vi_layoutxlm_xfund_zh_udml.yml](../../configs/kie/vi_layoutxlm/re_vi_layoutxlm_xfund_zh_udml.yml)|83.92%|[训练模型](https://paddleocr.bj.bcebos.com/ppstructure/models/vi_layoutxlm/re_vi_layoutxlm_xfund_pretrained.tar)/[推理模型(coming soon)]()|
|
||||
|VI-LayoutXLM |VI-LayoutXLM-base |RE | [re_vi_layoutxlm_xfund_zh_udml.yml](../../configs/kie/vi_layoutxlm/re_vi_layoutxlm_xfund_zh_udml.yml)|83.92%|[训练模型](https://paddleocr.bj.bcebos.com/ppstructure/models/vi_layoutxlm/re_vi_layoutxlm_xfund_pretrained.tar)/[推理模型](https://paddleocr.bj.bcebos.com/ppstructure/models/vi_layoutxlm/re_vi_layoutxlm_xfund_infer.tar)|
|
||||
|
||||
<a name="2"></a>
|
||||
|
||||
|
@ -45,7 +45,7 @@ VI-LayoutXLM基于LayoutXLM进行改进,在下游任务训练过程中,去
|
|||
|
||||
### 4.1 Python推理
|
||||
|
||||
**注:** 目前RE任务推理过程仍在适配中,下面以SER任务为例,介绍基于VI-LayoutXLM模型的关键信息抽取过程。
|
||||
- SER
|
||||
|
||||
首先将训练得到的模型转换成inference model。以VI-LayoutXLM模型在XFUND_zh数据集上训练的模型为例([模型下载地址](https://paddleocr.bj.bcebos.com/ppstructure/models/vi_layoutxlm/ser_vi_layoutxlm_xfund_pretrained.tar)),可以使用下面的命令进行转换。
|
||||
|
||||
|
@ -74,6 +74,36 @@ SER可视化结果默认保存到`./output`文件夹里面,结果示例如下
|
|||
<img src="../../ppstructure/docs/kie/result_ser/zh_val_42_ser.jpg" width="800">
|
||||
</div>
|
||||
|
||||
- RE
|
||||
|
||||
首先将训练得到的模型转换成inference model。以VI-LayoutXLM模型在XFUND_zh数据集上训练的模型为例([模型下载地址](https://paddleocr.bj.bcebos.com/ppstructure/models/vi_layoutxlm/re_vi_layoutxlm_xfund_pretrained.tar)),可以使用下面的命令进行转换。
|
||||
|
||||
``` bash
|
||||
wget https://paddleocr.bj.bcebos.com/ppstructure/models/vi_layoutxlm/re_vi_layoutxlm_xfund_pretrained.tar
|
||||
tar -xf re_vi_layoutxlm_xfund_pretrained.tar
|
||||
python3 tools/export_model.py -c configs/kie/vi_layoutxlm/re_vi_layoutxlm_xfund_zh.yml -o Architecture.Backbone.checkpoints=./re_vi_layoutxlm_xfund_pretrained/best_accuracy Global.save_inference_dir=./inference/re_vi_layoutxlm_infer
|
||||
```
|
||||
|
||||
VI-LayoutXLM模型基于RE任务进行推理,可以执行如下命令:
|
||||
|
||||
```bash
|
||||
cd ppstructure
|
||||
python3 kie/predict_kie_token_ser_re.py \
|
||||
--kie_algorithm=LayoutXLM \
|
||||
--re_model_dir=../inference/re_vi_layoutxlm_infer \
|
||||
--ser_model_dir=../inference/ser_vi_layoutxlm_infer \
|
||||
--use_visual_backbone=False \
|
||||
--image_dir=./docs/kie/input/zh_val_42.jpg \
|
||||
--ser_dict_path=../train_data/XFUND/class_list_xfun.txt \
|
||||
--vis_font_path=../doc/fonts/simfang.ttf \
|
||||
--ocr_order_method="tb-yx"
|
||||
```
|
||||
|
||||
RE可视化结果默认保存到`./output`文件夹里面,结果示例如下:
|
||||
|
||||
<div align="center">
|
||||
<img src="../../ppstructure/docs/kie/result_re/zh_val_42_re.jpg" width="800">
|
||||
</div>
|
||||
|
||||
<a name="4-2"></a>
|
||||
### 4.2 C++推理部署
|
||||
|
|
|
@ -28,7 +28,7 @@ On XFUND_zh dataset, the algorithm reproduction Hmean is as follows.
|
|||
|Model|Backbone|Task |Cnnfig|Hmean|Download link|
|
||||
| --- | --- |--|--- | --- | --- |
|
||||
|LayoutXLM|LayoutXLM-base|SER |[ser_layoutxlm_xfund_zh.yml](../../configs/kie/layoutlm_series/ser_layoutxlm_xfund_zh.yml)|90.38%|[trained model](https://paddleocr.bj.bcebos.com/pplayout/ser_LayoutXLM_xfun_zh.tar)/[inference model](https://paddleocr.bj.bcebos.com/pplayout/ser_LayoutXLM_xfun_zh_infer.tar)|
|
||||
|LayoutXLM|LayoutXLM-base|RE | [re_layoutxlm_xfund_zh.yml](../../configs/kie/layoutlm_series/re_layoutxlm_xfund_zh.yml)|74.83%|[trained model](https://paddleocr.bj.bcebos.com/pplayout/re_LayoutXLM_xfun_zh.tar)/[inference model(coming soon)]()|
|
||||
|LayoutXLM|LayoutXLM-base|RE | [re_layoutxlm_xfund_zh.yml](../../configs/kie/layoutlm_series/re_layoutxlm_xfund_zh.yml)|74.83%|[trained model](https://paddleocr.bj.bcebos.com/pplayout/re_LayoutXLM_xfun_zh.tar)/[inference model](https://paddleocr.bj.bcebos.com/pplayout/re_LayoutXLM_xfun_zh_infer.tar)|
|
||||
|
||||
|
||||
## 2. Environment
|
||||
|
@ -46,7 +46,7 @@ Please refer to [KIE tutorial](./kie_en.md)。PaddleOCR has modularized the code
|
|||
|
||||
### 4.1 Python Inference
|
||||
|
||||
**Note:** Currently, the RE model inference process is still in the process of adaptation. We take SER model as an example to introduce the KIE process based on LayoutXLM model.
|
||||
- SER
|
||||
|
||||
First, we need to export the trained model into inference model. Take LayoutXLM model trained on XFUND_zh as an example ([trained model download link](https://paddleocr.bj.bcebos.com/pplayout/ser_LayoutXLM_xfun_zh.tar)). Use the following command to export.
|
||||
|
||||
|
@ -54,7 +54,7 @@ First, we need to export the trained model into inference model. Take LayoutXLM
|
|||
``` bash
|
||||
wget https://paddleocr.bj.bcebos.com/pplayout/ser_LayoutXLM_xfun_zh.tar
|
||||
tar -xf ser_LayoutXLM_xfun_zh.tar
|
||||
python3 tools/export_model.py -c configs/kie/layoutlm_series/ser_layoutxlm_xfund_zh.yml -o Architecture.Backbone.checkpoints=./ser_LayoutXLM_xfun_zh/best_accuracy Global.save_inference_dir=./inference/ser_layoutxlm
|
||||
python3 tools/export_model.py -c configs/kie/layoutlm_series/ser_layoutxlm_xfund_zh.yml -o Architecture.Backbone.checkpoints=./ser_LayoutXLM_xfun_zh Global.save_inference_dir=./inference/ser_layoutxlm_infer
|
||||
```
|
||||
|
||||
Use the following command to infer using LayoutXLM SER model.
|
||||
|
@ -77,6 +77,38 @@ The SER visualization results are saved in the `./output` directory by default.
|
|||
</div>
|
||||
|
||||
|
||||
- RE
|
||||
|
||||
First, we need to export the trained model into inference model. Take LayoutXLM model trained on XFUND_zh as an example ([trained model download link](https://paddleocr.bj.bcebos.com/pplayout/re_LayoutXLM_xfun_zh.tar)). Use the following command to export.
|
||||
|
||||
|
||||
``` bash
|
||||
wget https://paddleocr.bj.bcebos.com/pplayout/re_LayoutXLM_xfun_zh.tar
|
||||
tar -xf re_LayoutXLM_xfun_zh.tar
|
||||
python3 tools/export_model.py -c configs/kie/layoutlm_series/re_layoutxlm_xfund_zh.yml -o Architecture.Backbone.checkpoints=./re_LayoutXLM_xfun_zh Global.save_inference_dir=./inference/re_layoutxlm_infer
|
||||
```
|
||||
|
||||
Use the following command to infer using LayoutXLM RE model.
|
||||
|
||||
|
||||
```bash
|
||||
cd ppstructure
|
||||
python3 kie/predict_kie_token_ser_re.py \
|
||||
--kie_algorithm=LayoutXLM \
|
||||
--re_model_dir=../inference/re_layoutxlm_infer \
|
||||
--ser_model_dir=../inference/ser_layoutxlm_infer \
|
||||
--image_dir=./docs/kie/input/zh_val_42.jpg \
|
||||
--ser_dict_path=../train_data/XFUND/class_list_xfun.txt \
|
||||
--vis_font_path=../doc/fonts/simfang.ttf
|
||||
```
|
||||
The RE visualization results are saved in the `./output` directory by default. The results are as follows.
|
||||
|
||||
|
||||
<div align="center">
|
||||
<img src="../../ppstructure/docs/kie/result_re/zh_val_42_re.jpg" width="800">
|
||||
</div>
|
||||
|
||||
|
||||
### 4.2 C++ Inference
|
||||
|
||||
Not supported
|
||||
|
|
|
@ -22,7 +22,7 @@ On XFUND_zh dataset, the algorithm reproduction Hmean is as follows.
|
|||
|Model|Backbone|Task |Cnnfig|Hmean|Download link|
|
||||
| --- | --- |---| --- | --- | --- |
|
||||
|VI-LayoutXLM |VI-LayoutXLM-base | SER |[ser_vi_layoutxlm_xfund_zh_udml.yml](../../configs/kie/vi_layoutxlm/ser_vi_layoutxlm_xfund_zh_udml.yml)|93.19%|[trained model](https://paddleocr.bj.bcebos.com/ppstructure/models/vi_layoutxlm/ser_vi_layoutxlm_xfund_pretrained.tar)/[inference model](https://paddleocr.bj.bcebos.com/ppstructure/models/vi_layoutxlm/ser_vi_layoutxlm_xfund_infer.tar)|
|
||||
|VI-LayoutXLM |VI-LayoutXLM-base |RE | [re_vi_layoutxlm_xfund_zh_udml.yml](../../configs/kie/vi_layoutxlm/re_vi_layoutxlm_xfund_zh_udml.yml)|83.92%|[trained model](https://paddleocr.bj.bcebos.com/ppstructure/models/vi_layoutxlm/re_vi_layoutxlm_xfund_pretrained.tar)/[inference model(coming soon)]()|
|
||||
|VI-LayoutXLM |VI-LayoutXLM-base |RE | [re_vi_layoutxlm_xfund_zh_udml.yml](../../configs/kie/vi_layoutxlm/re_vi_layoutxlm_xfund_zh_udml.yml)|83.92%|[trained model](https://paddleocr.bj.bcebos.com/ppstructure/models/vi_layoutxlm/re_vi_layoutxlm_xfund_pretrained.tar)/[inference model](https://paddleocr.bj.bcebos.com/ppstructure/models/vi_layoutxlm/re_vi_layoutxlm_xfund_infer.tar)|
|
||||
|
||||
|
||||
Please refer to ["Environment Preparation"](./environment_en.md) to configure the PaddleOCR environment, and refer to ["Project Clone"](./clone_en.md) to clone the project code.
|
||||
|
@ -37,7 +37,7 @@ Please refer to [KIE tutorial](./kie_en.md)。PaddleOCR has modularized the code
|
|||
|
||||
### 4.1 Python Inference
|
||||
|
||||
**Note:** Currently, the RE model inference process is still in the process of adaptation. We take SER model as an example to introduce the KIE process based on VI-LayoutXLM model.
|
||||
- SER
|
||||
|
||||
First, we need to export the trained model into inference model. Take VI-LayoutXLM model trained on XFUND_zh as an example ([trained model download link](https://paddleocr.bj.bcebos.com/ppstructure/models/vi_layoutxlm/ser_vi_layoutxlm_xfund_pretrained.tar)). Use the following command to export.
|
||||
|
||||
|
@ -70,6 +70,41 @@ The SER visualization results are saved in the `./output` folder by default. The
|
|||
</div>
|
||||
|
||||
|
||||
- RE
|
||||
|
||||
First, we need to export the trained model into inference model. Take VI-LayoutXLM model trained on XFUND_zh as an example ([trained model download link](https://paddleocr.bj.bcebos.com/ppstructure/models/vi_layoutxlm/re_vi_layoutxlm_xfund_pretrained.tar)). Use the following command to export.
|
||||
|
||||
|
||||
``` bash
|
||||
wget https://paddleocr.bj.bcebos.com/ppstructure/models/vi_layoutxlm/re_vi_layoutxlm_xfund_pretrained.tar
|
||||
tar -xf re_vi_layoutxlm_xfund_pretrained.tar
|
||||
python3 tools/export_model.py -c configs/kie/vi_layoutxlm/re_vi_layoutxlm_xfund_zh.yml -o Architecture.Backbone.checkpoints=./re_vi_layoutxlm_xfund_pretrained/best_accuracy Global.save_inference_dir=./inference/re_vi_layoutxlm_infer
|
||||
```
|
||||
|
||||
Use the following command to infer using VI-LayoutXLM RE model.
|
||||
|
||||
|
||||
```bash
|
||||
cd ppstructure
|
||||
python3 kie/predict_kie_token_ser_re.py \
|
||||
--kie_algorithm=LayoutXLM \
|
||||
--re_model_dir=../inference/re_vi_layoutxlm_infer \
|
||||
--ser_model_dir=../inference/ser_vi_layoutxlm_infer \
|
||||
--use_visual_backbone=False \
|
||||
--image_dir=./docs/kie/input/zh_val_42.jpg \
|
||||
--ser_dict_path=../train_data/XFUND/class_list_xfun.txt \
|
||||
--vis_font_path=../doc/fonts/simfang.ttf \
|
||||
--ocr_order_method="tb-yx"
|
||||
```
|
||||
|
||||
The RE visualization results are saved in the `./output` folder by default. The results are as follows.
|
||||
|
||||
|
||||
<div align="center">
|
||||
<img src="../../ppstructure/docs/kie/result_re/zh_val_42_re.jpg" width="800">
|
||||
</div>
|
||||
|
||||
|
||||
### 4.2 C++ Inference
|
||||
|
||||
Not supported
|
||||
|
|
|
@ -12,11 +12,9 @@
|
|||
# See the License for the specific language governing permissions and
|
||||
# limitations under the License.
|
||||
|
||||
from .token import VQATokenPad, VQASerTokenChunk, VQAReTokenChunk, VQAReTokenRelation
|
||||
from .token import VQATokenPad, VQASerTokenChunk, VQAReTokenChunk, VQAReTokenRelation, TensorizeEntitiesRelations
|
||||
|
||||
__all__ = [
|
||||
'VQATokenPad',
|
||||
'VQASerTokenChunk',
|
||||
'VQAReTokenChunk',
|
||||
'VQAReTokenRelation',
|
||||
'VQATokenPad', 'VQASerTokenChunk', 'VQAReTokenChunk', 'VQAReTokenRelation',
|
||||
'TensorizeEntitiesRelations'
|
||||
]
|
||||
|
|
|
@ -15,3 +15,4 @@
|
|||
from .vqa_token_chunk import VQASerTokenChunk, VQAReTokenChunk
|
||||
from .vqa_token_pad import VQATokenPad
|
||||
from .vqa_token_relation import VQAReTokenRelation
|
||||
from .vqa_re_convert import TensorizeEntitiesRelations
|
|
@ -0,0 +1,51 @@
|
|||
# copyright (c) 2022 PaddlePaddle Authors. All Rights Reserve.
|
||||
#
|
||||
# Licensed under the Apache License, Version 2.0 (the "License");
|
||||
# you may not use this file except in compliance with the License.
|
||||
# You may obtain a copy of the License at
|
||||
#
|
||||
# http://www.apache.org/licenses/LICENSE-2.0
|
||||
#
|
||||
# Unless required by applicable law or agreed to in writing, software
|
||||
# distributed under the License is distributed on an "AS IS" BASIS,
|
||||
# WITHOUT WARRANTIES OR CONDITIONS OF ANY KIND, either express or implied.
|
||||
# See the License for the specific language governing permissions and
|
||||
# limitations under the License.
|
||||
|
||||
import numpy as np
|
||||
|
||||
|
||||
class TensorizeEntitiesRelations(object):
|
||||
def __init__(self, max_seq_len=512, infer_mode=False, **kwargs):
|
||||
self.max_seq_len = max_seq_len
|
||||
self.infer_mode = infer_mode
|
||||
|
||||
def __call__(self, data):
|
||||
entities = data['entities']
|
||||
relations = data['relations']
|
||||
|
||||
entities_new = np.full(
|
||||
shape=[self.max_seq_len + 1, 3], fill_value=-1, dtype='int64')
|
||||
entities_new[0, 0] = len(entities['start'])
|
||||
entities_new[0, 1] = len(entities['end'])
|
||||
entities_new[0, 2] = len(entities['label'])
|
||||
entities_new[1:len(entities['start']) + 1, 0] = np.array(entities[
|
||||
'start'])
|
||||
entities_new[1:len(entities['end']) + 1, 1] = np.array(entities['end'])
|
||||
entities_new[1:len(entities['label']) + 1, 2] = np.array(entities[
|
||||
'label'])
|
||||
|
||||
relations_new = np.full(
|
||||
shape=[self.max_seq_len * self.max_seq_len + 1, 2],
|
||||
fill_value=-1,
|
||||
dtype='int64')
|
||||
relations_new[0, 0] = len(relations['head'])
|
||||
relations_new[0, 1] = len(relations['tail'])
|
||||
relations_new[1:len(relations['head']) + 1, 0] = np.array(relations[
|
||||
'head'])
|
||||
relations_new[1:len(relations['tail']) + 1, 1] = np.array(relations[
|
||||
'tail'])
|
||||
|
||||
data['entities'] = entities_new
|
||||
data['relations'] = relations_new
|
||||
return data
|
|
@ -417,11 +417,13 @@ class DistillationVQADistanceLoss(DistanceLoss):
|
|||
mode="l2",
|
||||
model_name_pairs=[],
|
||||
key=None,
|
||||
index=None,
|
||||
name="loss_distance",
|
||||
**kargs):
|
||||
super().__init__(mode=mode, **kargs)
|
||||
assert isinstance(model_name_pairs, list)
|
||||
self.key = key
|
||||
self.index = index
|
||||
self.model_name_pairs = model_name_pairs
|
||||
self.name = name + "_l2"
|
||||
|
||||
|
@ -434,6 +436,9 @@ class DistillationVQADistanceLoss(DistanceLoss):
|
|||
if self.key is not None:
|
||||
out1 = out1[self.key]
|
||||
out2 = out2[self.key]
|
||||
if self.index is not None:
|
||||
out1 = out1[:, self.index, :, :]
|
||||
out2 = out2[:, self.index, :, :]
|
||||
if attention_mask is not None:
|
||||
max_len = attention_mask.shape[-1]
|
||||
out1 = out1[:, :max_len]
|
||||
|
|
|
@ -37,23 +37,25 @@ class VQAReTokenMetric(object):
|
|||
gt_relations = []
|
||||
for b in range(len(self.relations_list)):
|
||||
rel_sent = []
|
||||
if "head" in self.relations_list[b]:
|
||||
for head, tail in zip(self.relations_list[b]["head"],
|
||||
self.relations_list[b]["tail"]):
|
||||
relation_list = self.relations_list[b]
|
||||
entitie_list = self.entities_list[b]
|
||||
head_len = relation_list[0, 0]
|
||||
if head_len > 0:
|
||||
entitie_start_list = entitie_list[1:entitie_list[0, 0] + 1, 0]
|
||||
entitie_end_list = entitie_list[1:entitie_list[0, 1] + 1, 1]
|
||||
entitie_label_list = entitie_list[1:entitie_list[0, 2] + 1, 2]
|
||||
for head, tail in zip(relation_list[1:head_len + 1, 0],
|
||||
relation_list[1:head_len + 1, 1]):
|
||||
rel = {}
|
||||
rel["head_id"] = head
|
||||
rel["head"] = (
|
||||
self.entities_list[b]["start"][rel["head_id"]],
|
||||
self.entities_list[b]["end"][rel["head_id"]])
|
||||
rel["head_type"] = self.entities_list[b]["label"][rel[
|
||||
"head_id"]]
|
||||
rel["head"] = (entitie_start_list[head],
|
||||
entitie_end_list[head])
|
||||
rel["head_type"] = entitie_label_list[head]
|
||||
|
||||
rel["tail_id"] = tail
|
||||
rel["tail"] = (
|
||||
self.entities_list[b]["start"][rel["tail_id"]],
|
||||
self.entities_list[b]["end"][rel["tail_id"]])
|
||||
rel["tail_type"] = self.entities_list[b]["label"][rel[
|
||||
"tail_id"]]
|
||||
rel["tail"] = (entitie_start_list[tail],
|
||||
entitie_end_list[tail])
|
||||
rel["tail_type"] = entitie_label_list[tail]
|
||||
|
||||
rel["type"] = 1
|
||||
rel_sent.append(rel)
|
||||
|
|
|
@ -218,8 +218,12 @@ class LayoutXLMForRe(NLPBaseModel):
|
|||
def forward(self, x):
|
||||
if self.use_visual_backbone is True:
|
||||
image = x[4]
|
||||
entities = x[5]
|
||||
relations = x[6]
|
||||
else:
|
||||
image = None
|
||||
entities = x[4]
|
||||
relations = x[5]
|
||||
x = self.model(
|
||||
input_ids=x[0],
|
||||
bbox=x[1],
|
||||
|
@ -229,6 +233,6 @@ class LayoutXLMForRe(NLPBaseModel):
|
|||
position_ids=None,
|
||||
head_mask=None,
|
||||
labels=None,
|
||||
entities=x[5],
|
||||
relations=x[6])
|
||||
entities=entities,
|
||||
relations=relations)
|
||||
return x
|
||||
|
|
|
@ -21,18 +21,22 @@ class VQAReTokenLayoutLMPostProcess(object):
|
|||
super(VQAReTokenLayoutLMPostProcess, self).__init__()
|
||||
|
||||
def __call__(self, preds, label=None, *args, **kwargs):
|
||||
pred_relations = preds['pred_relations']
|
||||
if isinstance(preds['pred_relations'], paddle.Tensor):
|
||||
pred_relations = pred_relations.numpy()
|
||||
pred_relations = self.decode_pred(pred_relations)
|
||||
|
||||
if label is not None:
|
||||
return self._metric(preds, label)
|
||||
return self._metric(pred_relations, label)
|
||||
else:
|
||||
return self._infer(preds, *args, **kwargs)
|
||||
return self._infer(pred_relations, *args, **kwargs)
|
||||
|
||||
def _metric(self, preds, label):
|
||||
return preds['pred_relations'], label[6], label[5]
|
||||
def _metric(self, pred_relations, label):
|
||||
return pred_relations, label[-1], label[-2]
|
||||
|
||||
def _infer(self, preds, *args, **kwargs):
|
||||
def _infer(self, pred_relations, *args, **kwargs):
|
||||
ser_results = kwargs['ser_results']
|
||||
entity_idx_dict_batch = kwargs['entity_idx_dict_batch']
|
||||
pred_relations = preds['pred_relations']
|
||||
|
||||
# merge relations and ocr info
|
||||
results = []
|
||||
|
@ -50,6 +54,24 @@ class VQAReTokenLayoutLMPostProcess(object):
|
|||
results.append(result)
|
||||
return results
|
||||
|
||||
def decode_pred(self, pred_relations):
|
||||
pred_relations_new = []
|
||||
for pred_relation in pred_relations:
|
||||
pred_relation_new = []
|
||||
pred_relation = pred_relation[1:pred_relation[0, 0, 0] + 1]
|
||||
for relation in pred_relation:
|
||||
relation_new = dict()
|
||||
relation_new['head_id'] = relation[0, 0]
|
||||
relation_new['head'] = tuple(relation[1])
|
||||
relation_new['head_type'] = relation[2, 0]
|
||||
relation_new['tail_id'] = relation[3, 0]
|
||||
relation_new['tail'] = tuple(relation[4])
|
||||
relation_new['tail_type'] = relation[5, 0]
|
||||
relation_new['type'] = relation[6, 0]
|
||||
pred_relation_new.append(relation_new)
|
||||
pred_relations_new.append(pred_relation_new)
|
||||
return pred_relations_new
|
||||
|
||||
|
||||
class DistillationRePostProcess(VQAReTokenLayoutLMPostProcess):
|
||||
"""
|
||||
|
|
Binary file not shown.
Before Width: | Height: | Size: 179 KiB After Width: | Height: | Size: 1.2 MiB |
|
@ -51,9 +51,9 @@
|
|||
|模型名称|模型简介 | 推理模型大小| 精度(hmean) | 预测耗时(ms) | 下载地址|
|
||||
| --- | --- | --- |--- |--- | --- |
|
||||
|ser_VI-LayoutXLM_xfund_zh|基于VI-LayoutXLM在xfund中文数据集上训练的SER模型|1.1G| 93.19% | 15.49 | [推理模型](https://paddleocr.bj.bcebos.com/ppstructure/models/vi_layoutxlm/ser_vi_layoutxlm_xfund_infer.tar) / [训练模型](https://paddleocr.bj.bcebos.com/ppstructure/models/vi_layoutxlm/ser_vi_layoutxlm_xfund_pretrained.tar) |
|
||||
|re_VI-LayoutXLM_xfund_zh|基于VI-LayoutXLM在xfund中文数据集上训练的RE模型|1.1G| 83.92% | 15.49 |[推理模型 coming soon]() / [训练模型](https://paddleocr.bj.bcebos.com/ppstructure/models/vi_layoutxlm/re_vi_layoutxlm_xfund_pretrained.tar) |
|
||||
|re_VI-LayoutXLM_xfund_zh|基于VI-LayoutXLM在xfund中文数据集上训练的RE模型|1.1G| 83.92% | 15.49 |[推理模型](https://paddleocr.bj.bcebos.com/ppstructure/models/vi_layoutxlm/re_vi_layoutxlm_xfund_infer.tar) / [训练模型](https://paddleocr.bj.bcebos.com/ppstructure/models/vi_layoutxlm/re_vi_layoutxlm_xfund_pretrained.tar) |
|
||||
|ser_LayoutXLM_xfund_zh|基于LayoutXLM在xfund中文数据集上训练的SER模型|1.4G| 90.38% | 19.49 |[推理模型](https://paddleocr.bj.bcebos.com/pplayout/ser_LayoutXLM_xfun_zh_infer.tar) / [训练模型](https://paddleocr.bj.bcebos.com/pplayout/ser_LayoutXLM_xfun_zh.tar) |
|
||||
|re_LayoutXLM_xfund_zh|基于LayoutXLM在xfund中文数据集上训练的RE模型|1.4G| 74.83% | 19.49 |[推理模型 coming soon]() / [训练模型](https://paddleocr.bj.bcebos.com/pplayout/re_LayoutXLM_xfun_zh.tar) |
|
||||
|re_LayoutXLM_xfund_zh|基于LayoutXLM在xfund中文数据集上训练的RE模型|1.4G| 74.83% | 19.49 |[推理模型](https://paddleocr.bj.bcebos.com/pplayout/re_LayoutXLM_xfun_zh_infer.tar) / [训练模型](https://paddleocr.bj.bcebos.com/pplayout/re_LayoutXLM_xfun_zh.tar) |
|
||||
|ser_LayoutLMv2_xfund_zh|基于LayoutLMv2在xfund中文数据集上训练的SER模型|778M| 85.44% | 31.46 |[推理模型](https://paddleocr.bj.bcebos.com/pplayout/ser_LayoutLMv2_xfun_zh_infer.tar) / [训练模型](https://paddleocr.bj.bcebos.com/pplayout/ser_LayoutLMv2_xfun_zh.tar) |
|
||||
|re_LayoutLMv2_xfund_zh|基于LayoutLMv2在xfun中文数据集上训练的RE模型|765M| 67.77% | 31.46 |[推理模型 coming soon]() / [训练模型](https://paddleocr.bj.bcebos.com/pplayout/re_LayoutLMv2_xfun_zh.tar) |
|
||||
|ser_LayoutLM_xfund_zh|基于LayoutLM在xfund中文数据集上训练的SER模型|430M| 77.31% | - |[推理模型](https://paddleocr.bj.bcebos.com/pplayout/ser_LayoutLM_xfun_zh_infer.tar) / [训练模型](https://paddleocr.bj.bcebos.com/pplayout/ser_LayoutLM_xfun_zh.tar) |
|
||||
|
|
|
@ -209,17 +209,18 @@ python3 ./tools/infer_kie_token_ser_re.py \
|
|||
|
||||
#### 4.2.3 Inference using PaddleInference
|
||||
|
||||
At present, only SER model supports inference using PaddleInference.
|
||||
|
||||
Firstly, download the inference SER inference model.
|
||||
|
||||
|
||||
```bash
|
||||
mkdir inference
|
||||
cd inference
|
||||
wget https://paddleocr.bj.bcebos.com/ppstructure/models/vi_layoutxlm/ser_vi_layoutxlm_xfund_infer.tar && tar -xf ser_vi_layoutxlm_xfund_infer.tar
|
||||
wget https://paddleocr.bj.bcebos.com/ppstructure/models/vi_layoutxlm/re_vi_layoutxlm_xfund_infer.tar && tar -xf re_vi_layoutxlm_xfund_infer.tar
|
||||
cd ..
|
||||
```
|
||||
|
||||
- SER
|
||||
|
||||
Use the following command for inference.
|
||||
|
||||
|
||||
|
@ -236,6 +237,26 @@ python3 kie/predict_kie_token_ser.py \
|
|||
|
||||
The visual results and text file will be saved in directory `output`.
|
||||
|
||||
- RE
|
||||
|
||||
Use the following command for inference.
|
||||
|
||||
|
||||
```bash
|
||||
cd ppstructure
|
||||
python3 kie/predict_kie_token_ser_re.py \
|
||||
--kie_algorithm=LayoutXLM \
|
||||
--re_model_dir=../inference/re_vi_layoutxlm_xfund_infer \
|
||||
--ser_model_dir=../inference/ser_vi_layoutxlm_xfund_infer \
|
||||
--use_visual_backbone=False \
|
||||
--image_dir=./docs/kie/input/zh_val_42.jpg \
|
||||
--ser_dict_path=../train_data/XFUND/class_list_xfun.txt \
|
||||
--vis_font_path=../doc/fonts/simfang.ttf \
|
||||
--ocr_order_method="tb-yx"
|
||||
```
|
||||
|
||||
The visual results and text file will be saved in directory `output`.
|
||||
|
||||
|
||||
### 4.3 More
|
||||
|
||||
|
|
|
@ -193,17 +193,18 @@ python3 ./tools/infer_kie_token_ser_re.py \
|
|||
|
||||
#### 4.2.3 基于PaddleInference的预测
|
||||
|
||||
目前仅SER模型支持PaddleInference推理。
|
||||
|
||||
首先下载SER的推理模型。
|
||||
|
||||
首先下载SER和RE的推理模型。
|
||||
|
||||
```bash
|
||||
mkdir inference
|
||||
cd inference
|
||||
wget https://paddleocr.bj.bcebos.com/ppstructure/models/vi_layoutxlm/ser_vi_layoutxlm_xfund_infer.tar && tar -xf ser_vi_layoutxlm_xfund_infer.tar
|
||||
wget https://paddleocr.bj.bcebos.com/ppstructure/models/vi_layoutxlm/re_vi_layoutxlm_xfund_infer.tar && tar -xf re_vi_layoutxlm_xfund_infer.tar
|
||||
cd ..
|
||||
```
|
||||
|
||||
- SER
|
||||
|
||||
执行下面的命令进行预测。
|
||||
|
||||
```bash
|
||||
|
@ -219,6 +220,26 @@ python3 kie/predict_kie_token_ser.py \
|
|||
|
||||
可视化结果保存在`output`目录下。
|
||||
|
||||
- RE
|
||||
|
||||
执行下面的命令进行预测。
|
||||
|
||||
```bash
|
||||
cd ppstructure
|
||||
python3 kie/predict_kie_token_ser_re.py \
|
||||
--kie_algorithm=LayoutXLM \
|
||||
--re_model_dir=../inference/re_vi_layoutxlm_xfund_infer \
|
||||
--ser_model_dir=../inference/ser_vi_layoutxlm_xfund_infer \
|
||||
--use_visual_backbone=False \
|
||||
--image_dir=./docs/kie/input/zh_val_42.jpg \
|
||||
--ser_dict_path=../train_data/XFUND/class_list_xfun.txt \
|
||||
--vis_font_path=../doc/fonts/simfang.ttf \
|
||||
--ocr_order_method="tb-yx"
|
||||
```
|
||||
|
||||
可视化结果保存在`output`目录下。
|
||||
|
||||
|
||||
### 4.3 更多
|
||||
|
||||
关于KIE模型的训练评估与推理,请参考:[关键信息抽取教程](../../doc/doc_ch/kie.md)。
|
||||
|
|
|
@ -102,16 +102,18 @@ class SerPredictor(object):
|
|||
ori_im = img.copy()
|
||||
data = {'image': img}
|
||||
data = transform(data, self.preprocess_op)
|
||||
img = data[0]
|
||||
if img is None:
|
||||
if data[0] is None:
|
||||
return None, 0
|
||||
img = np.expand_dims(img, axis=0)
|
||||
img = img.copy()
|
||||
starttime = time.time()
|
||||
|
||||
for idx in range(len(data)):
|
||||
if isinstance(data[idx], np.ndarray):
|
||||
data[idx] = np.expand_dims(data[idx], axis=0)
|
||||
else:
|
||||
data[idx] = [data[idx]]
|
||||
|
||||
for idx in range(len(self.input_tensor)):
|
||||
expand_input = np.expand_dims(data[idx], axis=0)
|
||||
self.input_tensor[idx].copy_from_cpu(expand_input)
|
||||
self.input_tensor[idx].copy_from_cpu(data[idx])
|
||||
|
||||
self.predictor.run()
|
||||
|
||||
|
@ -122,9 +124,9 @@ class SerPredictor(object):
|
|||
preds = outputs[0]
|
||||
|
||||
post_result = self.postprocess_op(
|
||||
preds, segment_offset_ids=[data[6]], ocr_infos=[data[7]])
|
||||
preds, segment_offset_ids=data[6], ocr_infos=data[7])
|
||||
elapse = time.time() - starttime
|
||||
return post_result, elapse
|
||||
return post_result, data, elapse
|
||||
|
||||
|
||||
def main(args):
|
||||
|
@ -145,7 +147,7 @@ def main(args):
|
|||
if img is None:
|
||||
logger.info("error in loading image:{}".format(image_file))
|
||||
continue
|
||||
ser_res, elapse = ser_predictor(img)
|
||||
ser_res, _, elapse = ser_predictor(img)
|
||||
ser_res = ser_res[0]
|
||||
|
||||
res_str = '{}\t{}\n'.format(
|
||||
|
|
|
@ -0,0 +1,127 @@
|
|||
# Copyright (c) 2022 PaddlePaddle Authors. All Rights Reserved.
|
||||
#
|
||||
# Licensed under the Apache License, Version 2.0 (the "License");
|
||||
# you may not use this file except in compliance with the License.
|
||||
# You may obtain a copy of the License at
|
||||
#
|
||||
# http://www.apache.org/licenses/LICENSE-2.0
|
||||
#
|
||||
# Unless required by applicable law or agreed to in writing, software
|
||||
# distributed under the License is distributed on an "AS IS" BASIS,
|
||||
# WITHOUT WARRANTIES OR CONDITIONS OF ANY KIND, either express or implied.
|
||||
# See the License for the specific language governing permissions and
|
||||
# limitations under the License.
|
||||
import os
|
||||
import sys
|
||||
|
||||
__dir__ = os.path.dirname(os.path.abspath(__file__))
|
||||
sys.path.append(__dir__)
|
||||
sys.path.insert(0, os.path.abspath(os.path.join(__dir__, '../..')))
|
||||
|
||||
os.environ["FLAGS_allocator_strategy"] = 'auto_growth'
|
||||
|
||||
import cv2
|
||||
import json
|
||||
import numpy as np
|
||||
import time
|
||||
|
||||
import tools.infer.utility as utility
|
||||
from tools.infer_kie_token_ser_re import make_input
|
||||
from ppocr.postprocess import build_post_process
|
||||
from ppocr.utils.logging import get_logger
|
||||
from ppocr.utils.visual import draw_re_results
|
||||
from ppocr.utils.utility import get_image_file_list, check_and_read
|
||||
from ppstructure.utility import parse_args
|
||||
from ppstructure.kie.predict_kie_token_ser import SerPredictor
|
||||
|
||||
from paddleocr import PaddleOCR
|
||||
|
||||
logger = get_logger()
|
||||
|
||||
|
||||
class SerRePredictor(object):
|
||||
def __init__(self, args):
|
||||
self.use_visual_backbone = args.use_visual_backbone
|
||||
self.ser_engine = SerPredictor(args)
|
||||
|
||||
postprocess_params = {'name': 'VQAReTokenLayoutLMPostProcess'}
|
||||
self.postprocess_op = build_post_process(postprocess_params)
|
||||
self.predictor, self.input_tensor, self.output_tensors, self.config = \
|
||||
utility.create_predictor(args, 're', logger)
|
||||
|
||||
def __call__(self, img):
|
||||
ori_im = img.copy()
|
||||
starttime = time.time()
|
||||
ser_results, ser_inputs, _ = self.ser_engine(img)
|
||||
re_input, entity_idx_dict_batch = make_input(ser_inputs, ser_results)
|
||||
if self.use_visual_backbone == False:
|
||||
re_input.pop(4)
|
||||
for idx in range(len(self.input_tensor)):
|
||||
self.input_tensor[idx].copy_from_cpu(re_input[idx])
|
||||
|
||||
self.predictor.run()
|
||||
outputs = []
|
||||
for output_tensor in self.output_tensors:
|
||||
output = output_tensor.copy_to_cpu()
|
||||
outputs.append(output)
|
||||
preds = dict(
|
||||
loss=outputs[1],
|
||||
pred_relations=outputs[2],
|
||||
hidden_states=outputs[0], )
|
||||
|
||||
post_result = self.postprocess_op(
|
||||
preds,
|
||||
ser_results=ser_results,
|
||||
entity_idx_dict_batch=entity_idx_dict_batch)
|
||||
|
||||
elapse = time.time() - starttime
|
||||
return post_result, elapse
|
||||
|
||||
|
||||
def main(args):
|
||||
image_file_list = get_image_file_list(args.image_dir)
|
||||
ser_predictor = SerRePredictor(args)
|
||||
count = 0
|
||||
total_time = 0
|
||||
|
||||
os.makedirs(args.output, exist_ok=True)
|
||||
with open(
|
||||
os.path.join(args.output, 'infer.txt'), mode='w',
|
||||
encoding='utf-8') as f_w:
|
||||
for image_file in image_file_list:
|
||||
img, flag, _ = check_and_read(image_file)
|
||||
if not flag:
|
||||
img = cv2.imread(image_file)
|
||||
img = img[:, :, ::-1]
|
||||
if img is None:
|
||||
logger.info("error in loading image:{}".format(image_file))
|
||||
continue
|
||||
re_res, elapse = ser_predictor(img)
|
||||
re_res = re_res[0]
|
||||
|
||||
res_str = '{}\t{}\n'.format(
|
||||
image_file,
|
||||
json.dumps(
|
||||
{
|
||||
"ocr_info": re_res,
|
||||
}, ensure_ascii=False))
|
||||
f_w.write(res_str)
|
||||
|
||||
img_res = draw_re_results(
|
||||
image_file, re_res, font_path=args.vis_font_path)
|
||||
|
||||
img_save_path = os.path.join(
|
||||
args.output,
|
||||
os.path.splitext(os.path.basename(image_file))[0] +
|
||||
"_ser_re.jpg")
|
||||
|
||||
cv2.imwrite(img_save_path, img_res)
|
||||
logger.info("save vis result to {}".format(img_save_path))
|
||||
if count > 0:
|
||||
total_time += elapse
|
||||
count += 1
|
||||
logger.info("Predict time of {}: {}".format(image_file, elapse))
|
||||
|
||||
|
||||
if __name__ == "__main__":
|
||||
main(parse_args())
|
|
@ -23,7 +23,7 @@ English | [简体中文](README_ch.md)
|
|||
|
||||
## 1. Introduction
|
||||
|
||||
Layout analysis refers to the regional division of documents in the form of pictures and the positioning of key areas, such as text, title, table, picture, etc. The layout analysis algorithm is based on the lightweight model PP-picodet of [PaddleDetection]( https://github.com/PaddlePaddle/PaddleDetection )
|
||||
Layout analysis refers to the regional division of documents in the form of pictures and the positioning of key areas, such as text, title, table, picture, etc. The layout analysis algorithm is based on the lightweight model PP-picodet of [PaddleDetection]( https://github.com/PaddlePaddle/PaddleDetection ), including English layout analysis, Chinese layout analysis and table layout analysis models. English layout analysis models can detect document layout elements such as text, title, table, figure, list. Chinese layout analysis models can detect document layout elements such as text, figure, figure caption, table, table caption, header, footer, reference, and equation. Table layout analysis models can detect table regions.
|
||||
|
||||
<div align="center">
|
||||
<img src="../docs/layout/layout.png" width="800">
|
||||
|
@ -152,7 +152,7 @@ We provide CDLA(Chinese layout analysis), TableBank(Table layout analysis)etc. d
|
|||
| [cTDaR2019_cTDaR](https://cndplab-founder.github.io/cTDaR2019/) | For form detection (TRACKA) and form identification (TRACKB).Image types include historical data sets (beginning with cTDaR_t0, such as CTDAR_T00872.jpg) and modern data sets (beginning with cTDaR_t1, CTDAR_T10482.jpg). |
|
||||
| [IIIT-AR-13K](http://cvit.iiit.ac.in/usodi/iiitar13k.php) | Data sets constructed by manually annotating figures or pages from publicly available annual reports, containing 5 categories:table, figure, natural image, logo, and signature. |
|
||||
| [TableBank](https://github.com/doc-analysis/TableBank) | For table detection and recognition of large datasets, including Word and Latex document formats |
|
||||
| [CDLA](https://github.com/buptlihang/CDLA) | Chinese document layout analysis data set, for Chinese literature (paper) scenarios, including 10 categories:Table, Figure, Figure caption, Table, Table caption, Header, Footer, Reference, Equation |
|
||||
| [CDLA](https://github.com/buptlihang/CDLA) | Chinese document layout analysis data set, for Chinese literature (paper) scenarios, including 10 categories:Text, Title, Figure, Figure caption, Table, Table caption, Header, Footer, Reference, Equation |
|
||||
| [DocBank](https://github.com/doc-analysis/DocBank) | Large-scale dataset (500K document pages) constructed using weakly supervised methods for document layout analysis, containing 12 categories:Author, Caption, Date, Equation, Figure, Footer, List, Paragraph, Reference, Section, Table, Title |
|
||||
|
||||
|
||||
|
@ -175,7 +175,7 @@ If the test image is Chinese, the pre-trained model of Chinese CDLA dataset can
|
|||
|
||||
### 5.1. Train
|
||||
|
||||
Train:
|
||||
Start training with the PaddleDetection [layout analysis profile](https://github.com/PaddlePaddle/PaddleDetection/tree/release/2.5/configs/picodet/legacy_model/application/layout_analysis)
|
||||
|
||||
* Modify Profile
|
||||
|
||||
|
|
|
@ -22,7 +22,7 @@
|
|||
|
||||
## 1. 简介
|
||||
|
||||
版面分析指的是对图片形式的文档进行区域划分,定位其中的关键区域,如文字、标题、表格、图片等。版面分析算法基于[PaddleDetection](https://github.com/PaddlePaddle/PaddleDetection)的轻量模型PP-PicoDet进行开发。
|
||||
版面分析指的是对图片形式的文档进行区域划分,定位其中的关键区域,如文字、标题、表格、图片等。版面分析算法基于[PaddleDetection](https://github.com/PaddlePaddle/PaddleDetection)的轻量模型PP-PicoDet进行开发,包含英文、中文、表格版面分析3类模型。其中,英文模型支持Text、Title、Tale、Figure、List5类区域的检测,中文模型支持Text、Title、Figure、Figure caption、Table、Table caption、Header、Footer、Reference、Equation10类区域的检测,表格版面分析支持Table区域的检测,版面分析效果如下图所示:
|
||||
|
||||
<div align="center">
|
||||
<img src="../docs/layout/layout.png" width="800">
|
||||
|
@ -152,7 +152,7 @@ json文件包含所有图像的标注,数据以字典嵌套的方式存放,
|
|||
| ------------------------------------------------------------ | ------------------------------------------------------------ |
|
||||
| [cTDaR2019_cTDaR](https://cndplab-founder.github.io/cTDaR2019/) | 用于表格检测(TRACKA)和表格识别(TRACKB)。图片类型包含历史数据集(以cTDaR_t0开头,如cTDaR_t00872.jpg)和现代数据集(以cTDaR_t1开头,cTDaR_t10482.jpg)。 |
|
||||
| [IIIT-AR-13K](http://cvit.iiit.ac.in/usodi/iiitar13k.php) | 手动注释公开的年度报告中的图形或页面而构建的数据集,包含5类:table, figure, natural image, logo, and signature |
|
||||
| [CDLA](https://github.com/buptlihang/CDLA) | 中文文档版面分析数据集,面向中文文献类(论文)场景,包含10类:Table、Figure、Figure caption、Table、Table caption、Header、Footer、Reference、Equation |
|
||||
| [CDLA](https://github.com/buptlihang/CDLA) | 中文文档版面分析数据集,面向中文文献类(论文)场景,包含10类:Text、Title、Figure、Figure caption、Table、Table caption、Header、Footer、Reference、Equation |
|
||||
| [TableBank](https://github.com/doc-analysis/TableBank) | 用于表格检测和识别大型数据集,包含Word和Latex2种文档格式 |
|
||||
| [DocBank](https://github.com/doc-analysis/DocBank) | 使用弱监督方法构建的大规模数据集(500K文档页面),用于文档布局分析,包含12类:Author、Caption、Date、Equation、Figure、Footer、List、Paragraph、Reference、Section、Table、Title |
|
||||
|
||||
|
@ -161,7 +161,7 @@ json文件包含所有图像的标注,数据以字典嵌套的方式存放,
|
|||
|
||||
提供了训练脚本、评估脚本和预测脚本,本节将以PubLayNet预训练模型为例进行讲解。
|
||||
|
||||
如果不希望训练,直接体验后面的模型评估、预测、动转静、推理的流程,可以下载提供的预训练模型(PubLayNet数据集),并跳过本部分。
|
||||
如果不希望训练,直接体验后面的模型评估、预测、动转静、推理的流程,可以下载提供的预训练模型(PubLayNet数据集),并跳过5.1和5.2。
|
||||
|
||||
```
|
||||
mkdir pretrained_model
|
||||
|
@ -176,7 +176,7 @@ wget https://paddleocr.bj.bcebos.com/ppstructure/models/layout/picodet_lcnet_x1_
|
|||
|
||||
### 5.1. 启动训练
|
||||
|
||||
开始训练:
|
||||
使用PaddleDetection[版面分析配置文件](https://github.com/PaddlePaddle/PaddleDetection/tree/release/2.5/configs/picodet/legacy_model/application/layout_analysis)启动训练
|
||||
|
||||
* 修改配置文件
|
||||
|
||||
|
|
|
@ -100,7 +100,8 @@ class StructureSystem(object):
|
|||
'180': cv2.ROTATE_180,
|
||||
'270': cv2.ROTATE_90_CLOCKWISE
|
||||
}
|
||||
img = cv2.rotate(img, cv_rotate_code[angle])
|
||||
if angle in cv_rotate_code:
|
||||
img = cv2.rotate(img, cv_rotate_code[angle])
|
||||
toc = time.time()
|
||||
time_dict['image_orientation'] = toc - tic
|
||||
if self.mode == 'structure':
|
||||
|
@ -254,8 +255,7 @@ def main(args):
|
|||
|
||||
if args.recovery and all_res != []:
|
||||
try:
|
||||
convert_info_docx(img, all_res, save_folder, img_name,
|
||||
args.save_pdf)
|
||||
convert_info_docx(img, all_res, save_folder, img_name)
|
||||
except Exception as ex:
|
||||
logger.error("error in layout recovery image:{}, err msg: {}".
|
||||
format(image_file, ex))
|
||||
|
|
|
@ -82,8 +82,11 @@ Through layout analysis, we divided the image/PDF documents into regions, locate
|
|||
|
||||
We can restore the test picture through the layout information, OCR detection and recognition structure, table information, and saved pictures.
|
||||
|
||||
The whl package is also provided for quick use, see [quickstart](../docs/quickstart_en.md) for details.
|
||||
The whl package is also provided for quick use, follow the above code, for more infomation please refer to [quickstart](../docs/quickstart_en.md) for details.
|
||||
|
||||
```bash
|
||||
paddleocr --image_dir=ppstructure/docs/table/1.png --type=structure --recovery=true --lang='en'
|
||||
```
|
||||
|
||||
<a name="3.1"></a>
|
||||
### 3.1 Download models
|
||||
|
|
|
@ -83,7 +83,16 @@ python3 -m pip install -r ppstructure/recovery/requirements.txt
|
|||
|
||||
我们通过版面信息、OCR检测和识别结构、表格信息、保存的图片,对测试图片进行恢复即可。
|
||||
|
||||
提供如下代码实现版面恢复,也提供了whl包的形式方便快速使用,详见 [quickstart](../docs/quickstart.md)。
|
||||
提供如下代码实现版面恢复,也提供了whl包的形式方便快速使用,代码如下,更多信息详见 [quickstart](../docs/quickstart.md)。
|
||||
|
||||
```bash
|
||||
# 中文测试图
|
||||
paddleocr --image_dir=ppstructure/docs/table/1.png --type=structure --recovery=true
|
||||
# 英文测试图
|
||||
paddleocr --image_dir=ppstructure/docs/table/1.png --type=structure --recovery=true --lang='en'
|
||||
# pdf测试文件
|
||||
paddleocr --image_dir=ppstructure/recovery/UnrealText.pdf --type=structure --recovery=true --lang='en'
|
||||
```
|
||||
|
||||
<a name="3.1"></a>
|
||||
|
||||
|
|
|
@ -28,7 +28,7 @@ from ppocr.utils.logging import get_logger
|
|||
logger = get_logger()
|
||||
|
||||
|
||||
def convert_info_docx(img, res, save_folder, img_name, save_pdf=False):
|
||||
def convert_info_docx(img, res, save_folder, img_name):
|
||||
doc = Document()
|
||||
doc.styles['Normal'].font.name = 'Times New Roman'
|
||||
doc.styles['Normal']._element.rPr.rFonts.set(qn('w:eastAsia'), u'宋体')
|
||||
|
@ -60,14 +60,9 @@ def convert_info_docx(img, res, save_folder, img_name, save_pdf=False):
|
|||
elif region['type'].lower() == 'title':
|
||||
doc.add_heading(region['res'][0]['text'])
|
||||
elif region['type'].lower() == 'table':
|
||||
paragraph = doc.add_paragraph()
|
||||
new_parser = HtmlToDocx()
|
||||
new_parser.table_style = 'TableGrid'
|
||||
table = new_parser.handle_table(html=region['res']['html'])
|
||||
new_table = deepcopy(table)
|
||||
new_table.alignment = WD_TABLE_ALIGNMENT.CENTER
|
||||
paragraph.add_run().element.addnext(new_table._tbl)
|
||||
|
||||
parser = HtmlToDocx()
|
||||
parser.table_style = 'TableGrid'
|
||||
parser.handle_table(region['res']['html'], doc)
|
||||
else:
|
||||
paragraph = doc.add_paragraph()
|
||||
paragraph_format = paragraph.paragraph_format
|
||||
|
@ -82,13 +77,6 @@ def convert_info_docx(img, res, save_folder, img_name, save_pdf=False):
|
|||
doc.save(docx_path)
|
||||
logger.info('docx save to {}'.format(docx_path))
|
||||
|
||||
# save to pdf
|
||||
if save_pdf:
|
||||
pdf_path = os.path.join(save_folder, '{}.pdf'.format(img_name))
|
||||
from docx2pdf import convert
|
||||
convert(docx_path, pdf_path)
|
||||
logger.info('pdf save to {}'.format(pdf_path))
|
||||
|
||||
|
||||
def sorted_layout_boxes(res, w):
|
||||
"""
|
||||
|
|
|
@ -1,4 +1,3 @@
|
|||
python-docx
|
||||
docx2pdf
|
||||
PyMuPDF
|
||||
beautifulsoup4
|
|
@ -1,4 +1,3 @@
|
|||
|
||||
# Copyright (c) 2022 PaddlePaddle Authors. All Rights Reserved.
|
||||
#
|
||||
# Licensed under the Apache License, Version 2.0 (the "License");
|
||||
|
@ -13,62 +12,59 @@
|
|||
# See the License for the specific language governing permissions and
|
||||
# limitations under the License.
|
||||
"""
|
||||
This code is refer from:https://github.com/pqzx/html2docx/blob/8f6695a778c68befb302e48ac0ed5201ddbd4524/htmldocx/h2d.py
|
||||
|
||||
This code is refer from: https://github.com/weizwx/html2docx/blob/master/htmldocx/h2d.py
|
||||
"""
|
||||
import re, argparse
|
||||
import io, os
|
||||
import urllib.request
|
||||
from urllib.parse import urlparse
|
||||
|
||||
import re
|
||||
import docx
|
||||
from docx import Document
|
||||
from bs4 import BeautifulSoup
|
||||
from html.parser import HTMLParser
|
||||
|
||||
import docx, docx.table
|
||||
from docx import Document
|
||||
from docx.shared import RGBColor, Pt, Inches
|
||||
from docx.enum.text import WD_COLOR, WD_ALIGN_PARAGRAPH
|
||||
from docx.oxml import OxmlElement
|
||||
from docx.oxml.ns import qn
|
||||
|
||||
from bs4 import BeautifulSoup
|
||||
|
||||
# values in inches
|
||||
INDENT = 0.25
|
||||
LIST_INDENT = 0.5
|
||||
MAX_INDENT = 5.5 # To stop indents going off the page
|
||||
|
||||
# Style to use with tables. By default no style is used.
|
||||
DEFAULT_TABLE_STYLE = None
|
||||
|
||||
# Style to use with paragraphs. By default no style is used.
|
||||
DEFAULT_PARAGRAPH_STYLE = None
|
||||
def get_table_rows(table_soup):
|
||||
table_row_selectors = [
|
||||
'table > tr', 'table > thead > tr', 'table > tbody > tr',
|
||||
'table > tfoot > tr'
|
||||
]
|
||||
# If there's a header, body, footer or direct child tr tags, add row dimensions from there
|
||||
return table_soup.select(', '.join(table_row_selectors), recursive=False)
|
||||
|
||||
|
||||
def get_filename_from_url(url):
|
||||
return os.path.basename(urlparse(url).path)
|
||||
def get_table_columns(row):
|
||||
# Get all columns for the specified row tag.
|
||||
return row.find_all(['th', 'td'], recursive=False) if row else []
|
||||
|
||||
def is_url(url):
|
||||
"""
|
||||
Not to be used for actually validating a url, but in our use case we only
|
||||
care if it's a url or a file path, and they're pretty distinguishable
|
||||
"""
|
||||
parts = urlparse(url)
|
||||
return all([parts.scheme, parts.netloc, parts.path])
|
||||
|
||||
def fetch_image(url):
|
||||
"""
|
||||
Attempts to fetch an image from a url.
|
||||
If successful returns a bytes object, else returns None
|
||||
:return:
|
||||
"""
|
||||
try:
|
||||
with urllib.request.urlopen(url) as response:
|
||||
# security flaw?
|
||||
return io.BytesIO(response.read())
|
||||
except urllib.error.URLError:
|
||||
return None
|
||||
def get_table_dimensions(table_soup):
|
||||
# Get rows for the table
|
||||
rows = get_table_rows(table_soup)
|
||||
# Table is either empty or has non-direct children between table and tr tags
|
||||
# Thus the row dimensions and column dimensions are assumed to be 0
|
||||
|
||||
cols = get_table_columns(rows[0]) if rows else []
|
||||
# Add colspan calculation column number
|
||||
col_count = 0
|
||||
for col in cols:
|
||||
colspan = col.attrs.get('colspan', 1)
|
||||
col_count += int(colspan)
|
||||
|
||||
return rows, col_count
|
||||
|
||||
|
||||
def get_cell_html(soup):
|
||||
# Returns string of td element with opening and closing <td> tags removed
|
||||
# Cannot use find_all as it only finds element tags and does not find text which
|
||||
# is not inside an element
|
||||
return ' '.join([str(i) for i in soup.contents])
|
||||
|
||||
|
||||
def delete_paragraph(paragraph):
|
||||
# https://github.com/python-openxml/python-docx/issues/33#issuecomment-77661907
|
||||
p = paragraph._element
|
||||
p.getparent().remove(p)
|
||||
p._p = p._element = None
|
||||
|
||||
def remove_last_occurence(ls, x):
|
||||
ls.pop(len(ls) - ls[::-1].index(x) - 1)
|
||||
|
||||
def remove_whitespace(string, leading=False, trailing=False):
|
||||
"""Remove white space from a string.
|
||||
|
@ -122,11 +118,6 @@ def remove_whitespace(string, leading=False, trailing=False):
|
|||
# TODO need some way to get rid of extra spaces in e.g. text <span> </span> text
|
||||
return re.sub(r'\s+', ' ', string)
|
||||
|
||||
def delete_paragraph(paragraph):
|
||||
# https://github.com/python-openxml/python-docx/issues/33#issuecomment-77661907
|
||||
p = paragraph._element
|
||||
p.getparent().remove(p)
|
||||
p._p = p._element = None
|
||||
|
||||
font_styles = {
|
||||
'b': 'bold',
|
||||
|
@ -145,13 +136,8 @@ font_names = {
|
|||
'pre': 'Courier',
|
||||
}
|
||||
|
||||
styles = {
|
||||
'LIST_BULLET': 'List Bullet',
|
||||
'LIST_NUMBER': 'List Number',
|
||||
}
|
||||
|
||||
class HtmlToDocx(HTMLParser):
|
||||
|
||||
def __init__(self):
|
||||
super().__init__()
|
||||
self.options = {
|
||||
|
@ -161,13 +147,11 @@ class HtmlToDocx(HTMLParser):
|
|||
'styles': True,
|
||||
}
|
||||
self.table_row_selectors = [
|
||||
'table > tr',
|
||||
'table > thead > tr',
|
||||
'table > tbody > tr',
|
||||
'table > tr', 'table > thead > tr', 'table > tbody > tr',
|
||||
'table > tfoot > tr'
|
||||
]
|
||||
self.table_style = DEFAULT_TABLE_STYLE
|
||||
self.paragraph_style = DEFAULT_PARAGRAPH_STYLE
|
||||
self.table_style = None
|
||||
self.paragraph_style = None
|
||||
|
||||
def set_initial_attrs(self, document=None):
|
||||
self.tags = {
|
||||
|
@ -178,9 +162,10 @@ class HtmlToDocx(HTMLParser):
|
|||
self.doc = document
|
||||
else:
|
||||
self.doc = Document()
|
||||
self.bs = self.options['fix-html'] # whether or not to clean with BeautifulSoup
|
||||
self.bs = self.options[
|
||||
'fix-html'] # whether or not to clean with BeautifulSoup
|
||||
self.document = self.doc
|
||||
self.include_tables = True #TODO add this option back in?
|
||||
self.include_tables = True #TODO add this option back in?
|
||||
self.include_images = self.options['images']
|
||||
self.include_styles = self.options['styles']
|
||||
self.paragraph = None
|
||||
|
@ -193,55 +178,52 @@ class HtmlToDocx(HTMLParser):
|
|||
self.table_style = other.table_style
|
||||
self.paragraph_style = other.paragraph_style
|
||||
|
||||
def get_cell_html(self, soup):
|
||||
# Returns string of td element with opening and closing <td> tags removed
|
||||
# Cannot use find_all as it only finds element tags and does not find text which
|
||||
# is not inside an element
|
||||
return ' '.join([str(i) for i in soup.contents])
|
||||
def ignore_nested_tables(self, tables_soup):
|
||||
"""
|
||||
Returns array containing only the highest level tables
|
||||
Operates on the assumption that bs4 returns child elements immediately after
|
||||
the parent element in `find_all`. If this changes in the future, this method will need to be updated
|
||||
:return:
|
||||
"""
|
||||
new_tables = []
|
||||
nest = 0
|
||||
for table in tables_soup:
|
||||
if nest:
|
||||
nest -= 1
|
||||
continue
|
||||
new_tables.append(table)
|
||||
nest = len(table.find_all('table'))
|
||||
return new_tables
|
||||
|
||||
def add_styles_to_paragraph(self, style):
|
||||
if 'text-align' in style:
|
||||
align = style['text-align']
|
||||
if align == 'center':
|
||||
self.paragraph.paragraph_format.alignment = WD_ALIGN_PARAGRAPH.CENTER
|
||||
elif align == 'right':
|
||||
self.paragraph.paragraph_format.alignment = WD_ALIGN_PARAGRAPH.RIGHT
|
||||
elif align == 'justify':
|
||||
self.paragraph.paragraph_format.alignment = WD_ALIGN_PARAGRAPH.JUSTIFY
|
||||
if 'margin-left' in style:
|
||||
margin = style['margin-left']
|
||||
units = re.sub(r'[0-9]+', '', margin)
|
||||
margin = int(float(re.sub(r'[a-z]+', '', margin)))
|
||||
if units == 'px':
|
||||
self.paragraph.paragraph_format.left_indent = Inches(min(margin // 10 * INDENT, MAX_INDENT))
|
||||
# TODO handle non px units
|
||||
def get_tables(self):
|
||||
if not hasattr(self, 'soup'):
|
||||
self.include_tables = False
|
||||
return
|
||||
# find other way to do it, or require this dependency?
|
||||
self.tables = self.ignore_nested_tables(self.soup.find_all('table'))
|
||||
self.table_no = 0
|
||||
|
||||
def add_styles_to_run(self, style):
|
||||
if 'color' in style:
|
||||
if 'rgb' in style['color']:
|
||||
color = re.sub(r'[a-z()]+', '', style['color'])
|
||||
colors = [int(x) for x in color.split(',')]
|
||||
elif '#' in style['color']:
|
||||
color = style['color'].lstrip('#')
|
||||
colors = tuple(int(color[i:i+2], 16) for i in (0, 2, 4))
|
||||
else:
|
||||
colors = [0, 0, 0]
|
||||
# TODO map colors to named colors (and extended colors...)
|
||||
# For now set color to black to prevent crashing
|
||||
self.run.font.color.rgb = RGBColor(*colors)
|
||||
|
||||
if 'background-color' in style:
|
||||
if 'rgb' in style['background-color']:
|
||||
color = color = re.sub(r'[a-z()]+', '', style['background-color'])
|
||||
colors = [int(x) for x in color.split(',')]
|
||||
elif '#' in style['background-color']:
|
||||
color = style['background-color'].lstrip('#')
|
||||
colors = tuple(int(color[i:i+2], 16) for i in (0, 2, 4))
|
||||
else:
|
||||
colors = [0, 0, 0]
|
||||
# TODO map colors to named colors (and extended colors...)
|
||||
# For now set color to black to prevent crashing
|
||||
self.run.font.highlight_color = WD_COLOR.GRAY_25 #TODO: map colors
|
||||
def run_process(self, html):
|
||||
if self.bs and BeautifulSoup:
|
||||
self.soup = BeautifulSoup(html, 'html.parser')
|
||||
html = str(self.soup)
|
||||
if self.include_tables:
|
||||
self.get_tables()
|
||||
self.feed(html)
|
||||
|
||||
def add_html_to_cell(self, html, cell):
|
||||
if not isinstance(cell, docx.table._Cell):
|
||||
raise ValueError('Second argument needs to be a %s' %
|
||||
docx.table._Cell)
|
||||
unwanted_paragraph = cell.paragraphs[0]
|
||||
if unwanted_paragraph.text == "":
|
||||
delete_paragraph(unwanted_paragraph)
|
||||
self.set_initial_attrs(cell)
|
||||
self.run_process(html)
|
||||
# cells must end with a paragraph or will get message about corrupt file
|
||||
# https://stackoverflow.com/a/29287121
|
||||
if not self.doc.paragraphs:
|
||||
self.doc.add_paragraph('')
|
||||
|
||||
def apply_paragraph_style(self, style=None):
|
||||
try:
|
||||
|
@ -250,69 +232,10 @@ class HtmlToDocx(HTMLParser):
|
|||
elif self.paragraph_style:
|
||||
self.paragraph.style = self.paragraph_style
|
||||
except KeyError as e:
|
||||
raise ValueError(f"Unable to apply style {self.paragraph_style}.") from e
|
||||
raise ValueError(
|
||||
f"Unable to apply style {self.paragraph_style}.") from e
|
||||
|
||||
def parse_dict_string(self, string, separator=';'):
|
||||
new_string = string.replace(" ", '').split(separator)
|
||||
string_dict = dict([x.split(':') for x in new_string if ':' in x])
|
||||
return string_dict
|
||||
|
||||
def handle_li(self):
|
||||
# check list stack to determine style and depth
|
||||
list_depth = len(self.tags['list'])
|
||||
if list_depth:
|
||||
list_type = self.tags['list'][-1]
|
||||
else:
|
||||
list_type = 'ul' # assign unordered if no tag
|
||||
|
||||
if list_type == 'ol':
|
||||
list_style = styles['LIST_NUMBER']
|
||||
else:
|
||||
list_style = styles['LIST_BULLET']
|
||||
|
||||
self.paragraph = self.doc.add_paragraph(style=list_style)
|
||||
self.paragraph.paragraph_format.left_indent = Inches(min(list_depth * LIST_INDENT, MAX_INDENT))
|
||||
self.paragraph.paragraph_format.line_spacing = 1
|
||||
|
||||
def add_image_to_cell(self, cell, image):
|
||||
# python-docx doesn't have method yet for adding images to table cells. For now we use this
|
||||
paragraph = cell.add_paragraph()
|
||||
run = paragraph.add_run()
|
||||
run.add_picture(image)
|
||||
|
||||
def handle_img(self, current_attrs):
|
||||
if not self.include_images:
|
||||
self.skip = True
|
||||
self.skip_tag = 'img'
|
||||
return
|
||||
src = current_attrs['src']
|
||||
# fetch image
|
||||
src_is_url = is_url(src)
|
||||
if src_is_url:
|
||||
try:
|
||||
image = fetch_image(src)
|
||||
except urllib.error.URLError:
|
||||
image = None
|
||||
else:
|
||||
image = src
|
||||
# add image to doc
|
||||
if image:
|
||||
try:
|
||||
if isinstance(self.doc, docx.document.Document):
|
||||
self.doc.add_picture(image)
|
||||
else:
|
||||
self.add_image_to_cell(self.doc, image)
|
||||
except FileNotFoundError:
|
||||
image = None
|
||||
if not image:
|
||||
if src_is_url:
|
||||
self.doc.add_paragraph("<image: %s>" % src)
|
||||
else:
|
||||
# avoid exposing filepaths in document
|
||||
self.doc.add_paragraph("<image: %s>" % get_filename_from_url(src))
|
||||
|
||||
|
||||
def handle_table(self, html):
|
||||
def handle_table(self, html, doc):
|
||||
"""
|
||||
To handle nested tables, we will parse tables manually as follows:
|
||||
Get table soup
|
||||
|
@ -320,194 +243,42 @@ class HtmlToDocx(HTMLParser):
|
|||
Iterate over soup and fill docx table with new instances of this parser
|
||||
Tell HTMLParser to ignore any tags until the corresponding closing table tag
|
||||
"""
|
||||
doc = Document()
|
||||
table_soup = BeautifulSoup(html, 'html.parser')
|
||||
rows, cols_len = self.get_table_dimensions(table_soup)
|
||||
rows, cols_len = get_table_dimensions(table_soup)
|
||||
table = doc.add_table(len(rows), cols_len)
|
||||
table.style = doc.styles['Table Grid']
|
||||
|
||||
cell_row = 0
|
||||
for index, row in enumerate(rows):
|
||||
cols = self.get_table_columns(row)
|
||||
cols = get_table_columns(row)
|
||||
cell_col = 0
|
||||
for col in cols:
|
||||
colspan = int(col.attrs.get('colspan', 1))
|
||||
rowspan = int(col.attrs.get('rowspan', 1))
|
||||
|
||||
cell_html = self.get_cell_html(col)
|
||||
|
||||
cell_html = get_cell_html(col)
|
||||
if col.name == 'th':
|
||||
cell_html = "<b>%s</b>" % cell_html
|
||||
|
||||
docx_cell = table.cell(cell_row, cell_col)
|
||||
|
||||
while docx_cell.text != '': # Skip the merged cell
|
||||
cell_col += 1
|
||||
docx_cell = table.cell(cell_row, cell_col)
|
||||
|
||||
cell_to_merge = table.cell(cell_row + rowspan - 1, cell_col + colspan - 1)
|
||||
cell_to_merge = table.cell(cell_row + rowspan - 1,
|
||||
cell_col + colspan - 1)
|
||||
if docx_cell != cell_to_merge:
|
||||
docx_cell.merge(cell_to_merge)
|
||||
|
||||
child_parser = HtmlToDocx()
|
||||
child_parser.copy_settings_from(self)
|
||||
|
||||
child_parser.add_html_to_cell(cell_html or ' ', docx_cell) # occupy the position
|
||||
child_parser.add_html_to_cell(cell_html or ' ', docx_cell)
|
||||
|
||||
cell_col += colspan
|
||||
cell_row += 1
|
||||
|
||||
# skip all tags until corresponding closing tag
|
||||
self.instances_to_skip = len(table_soup.find_all('table'))
|
||||
self.skip_tag = 'table'
|
||||
self.skip = True
|
||||
self.table = None
|
||||
return table
|
||||
|
||||
def handle_link(self, href, text):
|
||||
# Link requires a relationship
|
||||
is_external = href.startswith('http')
|
||||
rel_id = self.paragraph.part.relate_to(
|
||||
href,
|
||||
docx.opc.constants.RELATIONSHIP_TYPE.HYPERLINK,
|
||||
is_external=True # don't support anchor links for this library yet
|
||||
)
|
||||
|
||||
# Create the w:hyperlink tag and add needed values
|
||||
hyperlink = docx.oxml.shared.OxmlElement('w:hyperlink')
|
||||
hyperlink.set(docx.oxml.shared.qn('r:id'), rel_id)
|
||||
|
||||
|
||||
# Create sub-run
|
||||
subrun = self.paragraph.add_run()
|
||||
rPr = docx.oxml.shared.OxmlElement('w:rPr')
|
||||
|
||||
# add default color
|
||||
c = docx.oxml.shared.OxmlElement('w:color')
|
||||
c.set(docx.oxml.shared.qn('w:val'), "0000EE")
|
||||
rPr.append(c)
|
||||
|
||||
# add underline
|
||||
u = docx.oxml.shared.OxmlElement('w:u')
|
||||
u.set(docx.oxml.shared.qn('w:val'), 'single')
|
||||
rPr.append(u)
|
||||
|
||||
subrun._r.append(rPr)
|
||||
subrun._r.text = text
|
||||
|
||||
# Add subrun to hyperlink
|
||||
hyperlink.append(subrun._r)
|
||||
|
||||
# Add hyperlink to run
|
||||
self.paragraph._p.append(hyperlink)
|
||||
|
||||
def handle_starttag(self, tag, attrs):
|
||||
if self.skip:
|
||||
return
|
||||
if tag == 'head':
|
||||
self.skip = True
|
||||
self.skip_tag = tag
|
||||
self.instances_to_skip = 0
|
||||
return
|
||||
elif tag == 'body':
|
||||
return
|
||||
|
||||
current_attrs = dict(attrs)
|
||||
|
||||
if tag == 'span':
|
||||
self.tags['span'].append(current_attrs)
|
||||
return
|
||||
elif tag == 'ol' or tag == 'ul':
|
||||
self.tags['list'].append(tag)
|
||||
return # don't apply styles for now
|
||||
elif tag == 'br':
|
||||
self.run.add_break()
|
||||
return
|
||||
|
||||
self.tags[tag] = current_attrs
|
||||
if tag in ['p', 'pre']:
|
||||
self.paragraph = self.doc.add_paragraph()
|
||||
self.apply_paragraph_style()
|
||||
|
||||
elif tag == 'li':
|
||||
self.handle_li()
|
||||
|
||||
elif tag == "hr":
|
||||
|
||||
# This implementation was taken from:
|
||||
# https://github.com/python-openxml/python-docx/issues/105#issuecomment-62806373
|
||||
|
||||
self.paragraph = self.doc.add_paragraph()
|
||||
pPr = self.paragraph._p.get_or_add_pPr()
|
||||
pBdr = OxmlElement('w:pBdr')
|
||||
pPr.insert_element_before(pBdr,
|
||||
'w:shd', 'w:tabs', 'w:suppressAutoHyphens', 'w:kinsoku', 'w:wordWrap',
|
||||
'w:overflowPunct', 'w:topLinePunct', 'w:autoSpaceDE', 'w:autoSpaceDN',
|
||||
'w:bidi', 'w:adjustRightInd', 'w:snapToGrid', 'w:spacing', 'w:ind',
|
||||
'w:contextualSpacing', 'w:mirrorIndents', 'w:suppressOverlap', 'w:jc',
|
||||
'w:textDirection', 'w:textAlignment', 'w:textboxTightWrap',
|
||||
'w:outlineLvl', 'w:divId', 'w:cnfStyle', 'w:rPr', 'w:sectPr',
|
||||
'w:pPrChange'
|
||||
)
|
||||
bottom = OxmlElement('w:bottom')
|
||||
bottom.set(qn('w:val'), 'single')
|
||||
bottom.set(qn('w:sz'), '6')
|
||||
bottom.set(qn('w:space'), '1')
|
||||
bottom.set(qn('w:color'), 'auto')
|
||||
pBdr.append(bottom)
|
||||
|
||||
elif re.match('h[1-9]', tag):
|
||||
if isinstance(self.doc, docx.document.Document):
|
||||
h_size = int(tag[1])
|
||||
self.paragraph = self.doc.add_heading(level=min(h_size, 9))
|
||||
else:
|
||||
self.paragraph = self.doc.add_paragraph()
|
||||
|
||||
elif tag == 'img':
|
||||
self.handle_img(current_attrs)
|
||||
return
|
||||
|
||||
elif tag == 'table':
|
||||
self.handle_table()
|
||||
return
|
||||
|
||||
# set new run reference point in case of leading line breaks
|
||||
if tag in ['p', 'li', 'pre']:
|
||||
self.run = self.paragraph.add_run()
|
||||
|
||||
# add style
|
||||
if not self.include_styles:
|
||||
return
|
||||
if 'style' in current_attrs and self.paragraph:
|
||||
style = self.parse_dict_string(current_attrs['style'])
|
||||
self.add_styles_to_paragraph(style)
|
||||
|
||||
def handle_endtag(self, tag):
|
||||
if self.skip:
|
||||
if not tag == self.skip_tag:
|
||||
return
|
||||
|
||||
if self.instances_to_skip > 0:
|
||||
self.instances_to_skip -= 1
|
||||
return
|
||||
|
||||
self.skip = False
|
||||
self.skip_tag = None
|
||||
self.paragraph = None
|
||||
|
||||
if tag == 'span':
|
||||
if self.tags['span']:
|
||||
self.tags['span'].pop()
|
||||
return
|
||||
elif tag == 'ol' or tag == 'ul':
|
||||
remove_last_occurence(self.tags['list'], tag)
|
||||
return
|
||||
elif tag == 'table':
|
||||
self.table_no += 1
|
||||
self.table = None
|
||||
self.doc = self.document
|
||||
self.paragraph = None
|
||||
|
||||
if tag in self.tags:
|
||||
self.tags.pop(tag)
|
||||
# maybe set relevant reference to None?
|
||||
doc.save('1.docx')
|
||||
|
||||
def handle_data(self, data):
|
||||
if self.skip:
|
||||
|
@ -546,87 +317,3 @@ class HtmlToDocx(HTMLParser):
|
|||
if tag in font_names:
|
||||
font_name = font_names[tag]
|
||||
self.run.font.name = font_name
|
||||
|
||||
def ignore_nested_tables(self, tables_soup):
|
||||
"""
|
||||
Returns array containing only the highest level tables
|
||||
Operates on the assumption that bs4 returns child elements immediately after
|
||||
the parent element in `find_all`. If this changes in the future, this method will need to be updated
|
||||
:return:
|
||||
"""
|
||||
new_tables = []
|
||||
nest = 0
|
||||
for table in tables_soup:
|
||||
if nest:
|
||||
nest -= 1
|
||||
continue
|
||||
new_tables.append(table)
|
||||
nest = len(table.find_all('table'))
|
||||
return new_tables
|
||||
|
||||
def get_table_rows(self, table_soup):
|
||||
# If there's a header, body, footer or direct child tr tags, add row dimensions from there
|
||||
return table_soup.select(', '.join(self.table_row_selectors), recursive=False)
|
||||
|
||||
def get_table_columns(self, row):
|
||||
# Get all columns for the specified row tag.
|
||||
return row.find_all(['th', 'td'], recursive=False) if row else []
|
||||
|
||||
def get_table_dimensions(self, table_soup):
|
||||
# Get rows for the table
|
||||
rows = self.get_table_rows(table_soup)
|
||||
# Table is either empty or has non-direct children between table and tr tags
|
||||
# Thus the row dimensions and column dimensions are assumed to be 0
|
||||
|
||||
cols = self.get_table_columns(rows[0]) if rows else []
|
||||
# Add colspan calculation column number
|
||||
col_count = 0
|
||||
for col in cols:
|
||||
colspan = col.attrs.get('colspan', 1)
|
||||
col_count += int(colspan)
|
||||
|
||||
# return len(rows), col_count
|
||||
return rows, col_count
|
||||
|
||||
def get_tables(self):
|
||||
if not hasattr(self, 'soup'):
|
||||
self.include_tables = False
|
||||
return
|
||||
# find other way to do it, or require this dependency?
|
||||
self.tables = self.ignore_nested_tables(self.soup.find_all('table'))
|
||||
self.table_no = 0
|
||||
|
||||
def run_process(self, html):
|
||||
if self.bs and BeautifulSoup:
|
||||
self.soup = BeautifulSoup(html, 'html.parser')
|
||||
html = str(self.soup)
|
||||
if self.include_tables:
|
||||
self.get_tables()
|
||||
self.feed(html)
|
||||
|
||||
def add_html_to_document(self, html, document):
|
||||
if not isinstance(html, str):
|
||||
raise ValueError('First argument needs to be a %s' % str)
|
||||
elif not isinstance(document, docx.document.Document) and not isinstance(document, docx.table._Cell):
|
||||
raise ValueError('Second argument needs to be a %s' % docx.document.Document)
|
||||
self.set_initial_attrs(document)
|
||||
self.run_process(html)
|
||||
|
||||
def add_html_to_cell(self, html, cell):
|
||||
self.set_initial_attrs(cell)
|
||||
self.run_process(html)
|
||||
|
||||
def parse_html_file(self, filename_html, filename_docx=None):
|
||||
with open(filename_html, 'r') as infile:
|
||||
html = infile.read()
|
||||
self.set_initial_attrs()
|
||||
self.run_process(html)
|
||||
if not filename_docx:
|
||||
path, filename = os.path.split(filename_html)
|
||||
filename_docx = '%s/new_docx_file_%s' % (path, filename)
|
||||
self.doc.save('%s.docx' % filename_docx)
|
||||
|
||||
def parse_html_string(self, html):
|
||||
self.set_initial_attrs()
|
||||
self.run_process(html)
|
||||
return self.doc
|
|
@ -114,7 +114,7 @@ python3 table/eval_table.py \
|
|||
--det_model_dir=path/to/det_model_dir \
|
||||
--rec_model_dir=path/to/rec_model_dir \
|
||||
--table_model_dir=path/to/table_model_dir \
|
||||
--image_dir=../doc/table/1.png \
|
||||
--image_dir=docs/table/table.jpg \
|
||||
--rec_char_dict_path=../ppocr/utils/dict/table_dict.txt \
|
||||
--table_char_dict_path=../ppocr/utils/dict/table_structure_dict.txt \
|
||||
--det_limit_side_len=736 \
|
||||
|
@ -145,6 +145,7 @@ python3 table/eval_table.py \
|
|||
--table_char_dict_path=../ppocr/utils/dict/table_structure_dict.txt \
|
||||
--det_limit_side_len=736 \
|
||||
--det_limit_type=min \
|
||||
--rec_image_shape=3,32,320 \
|
||||
--gt_path=path/to/gt.txt
|
||||
```
|
||||
|
||||
|
|
|
@ -118,7 +118,7 @@ python3 table/eval_table.py \
|
|||
--det_model_dir=path/to/det_model_dir \
|
||||
--rec_model_dir=path/to/rec_model_dir \
|
||||
--table_model_dir=path/to/table_model_dir \
|
||||
--image_dir=../doc/table/1.png \
|
||||
--image_dir=docs/table/table.jpg \
|
||||
--rec_char_dict_path=../ppocr/utils/dict/table_dict.txt \
|
||||
--table_char_dict_path=../ppocr/utils/dict/table_structure_dict.txt \
|
||||
--det_limit_side_len=736 \
|
||||
|
@ -149,6 +149,7 @@ python3 table/eval_table.py \
|
|||
--table_char_dict_path=../ppocr/utils/dict/table_structure_dict.txt \
|
||||
--det_limit_side_len=736 \
|
||||
--det_limit_type=min \
|
||||
--rec_image_shape=3,32,320 \
|
||||
--gt_path=path/to/gt.txt
|
||||
```
|
||||
|
||||
|
|
|
@ -52,6 +52,8 @@ def init_args():
|
|||
# params for kie
|
||||
parser.add_argument("--kie_algorithm", type=str, default='LayoutXLM')
|
||||
parser.add_argument("--ser_model_dir", type=str)
|
||||
parser.add_argument("--re_model_dir", type=str)
|
||||
parser.add_argument("--use_visual_backbone", type=str2bool, default=True)
|
||||
parser.add_argument(
|
||||
"--ser_dict_path",
|
||||
type=str,
|
||||
|
@ -90,11 +92,6 @@ def init_args():
|
|||
type=str2bool,
|
||||
default=False,
|
||||
help='Whether to enable layout of recovery')
|
||||
parser.add_argument(
|
||||
"--save_pdf",
|
||||
type=str2bool,
|
||||
default=False,
|
||||
help='Whether to save pdf file')
|
||||
|
||||
return parser
|
||||
|
||||
|
@ -108,7 +105,38 @@ def draw_structure_result(image, result, font_path):
|
|||
if isinstance(image, np.ndarray):
|
||||
image = Image.fromarray(image)
|
||||
boxes, txts, scores = [], [], []
|
||||
|
||||
img_layout = image.copy()
|
||||
draw_layout = ImageDraw.Draw(img_layout)
|
||||
text_color = (255, 255, 255)
|
||||
text_background_color = (80, 127, 255)
|
||||
catid2color = {}
|
||||
font_size = 15
|
||||
font = ImageFont.truetype(font_path, font_size, encoding="utf-8")
|
||||
|
||||
for region in result:
|
||||
if region['type'] not in catid2color:
|
||||
box_color = (random.randint(0, 255), random.randint(0, 255),
|
||||
random.randint(0, 255))
|
||||
catid2color[region['type']] = box_color
|
||||
else:
|
||||
box_color = catid2color[region['type']]
|
||||
box_layout = region['bbox']
|
||||
draw_layout.rectangle(
|
||||
[(box_layout[0], box_layout[1]), (box_layout[2], box_layout[3])],
|
||||
outline=box_color,
|
||||
width=3)
|
||||
text_w, text_h = font.getsize(region['type'])
|
||||
draw_layout.rectangle(
|
||||
[(box_layout[0], box_layout[1]),
|
||||
(box_layout[0] + text_w, box_layout[1] + text_h)],
|
||||
fill=text_background_color)
|
||||
draw_layout.text(
|
||||
(box_layout[0], box_layout[1]),
|
||||
region['type'],
|
||||
fill=text_color,
|
||||
font=font)
|
||||
|
||||
if region['type'] == 'table':
|
||||
pass
|
||||
else:
|
||||
|
@ -116,6 +144,7 @@ def draw_structure_result(image, result, font_path):
|
|||
boxes.append(np.array(text_result['text_region']))
|
||||
txts.append(text_result['text'])
|
||||
scores.append(text_result['confidence'])
|
||||
|
||||
im_show = draw_ocr_box_txt(
|
||||
image, boxes, txts, scores, font_path=font_path, drop_score=0)
|
||||
img_layout, boxes, txts, scores, font_path=font_path, drop_score=0)
|
||||
return im_show
|
||||
|
|
|
@ -0,0 +1,20 @@
|
|||
===========================cpp_infer_params===========================
|
||||
model_name:en_table_structure_PACT
|
||||
use_opencv:True
|
||||
infer_model:./inference/en_ppocr_mobile_v2.0_table_structure_slim_infer/
|
||||
infer_quant:False
|
||||
inference:./deploy/cpp_infer/build/ppocr --rec_img_h=32 --det_model_dir=./inference/en_ppocr_mobile_v2.0_table_det_infer --rec_model_dir=./inference/en_ppocr_mobile_v2.0_table_rec_infer --rec_char_dict_path=./ppocr/utils/dict/table_dict.txt --table_char_dict_path=./ppocr/utils/dict/table_structure_dict.txt --limit_side_len=736 --limit_type=min --output=./output/table --merge_no_span_structure=False --type=structure --table=True
|
||||
--use_gpu:True|False
|
||||
--enable_mkldnn:False
|
||||
--cpu_threads:6
|
||||
--rec_batch_num:6
|
||||
--use_tensorrt:False
|
||||
--precision:fp32
|
||||
--table_model_dir:
|
||||
--image_dir:./ppstructure/docs/table/table.jpg
|
||||
null:null
|
||||
--benchmark:True
|
||||
--det:True
|
||||
--rec:True
|
||||
--cls:False
|
||||
--use_angle_cls:False
|
|
@ -0,0 +1,53 @@
|
|||
===========================train_params===========================
|
||||
model_name:layoutxlm_ser
|
||||
python:python3.7
|
||||
gpu_list:192.168.0.1,192.168.0.2;0,1
|
||||
Global.use_gpu:True
|
||||
Global.auto_cast:fp32
|
||||
Global.epoch_num:lite_train_lite_infer=1|whole_train_whole_infer=17
|
||||
Global.save_model_dir:./output/
|
||||
Train.loader.batch_size_per_card:lite_train_lite_infer=4|whole_train_whole_infer=8
|
||||
Architecture.Backbone.checkpoints:null
|
||||
train_model_name:latest
|
||||
train_infer_img_dir:ppstructure/docs/kie/input/zh_val_42.jpg
|
||||
null:null
|
||||
##
|
||||
trainer:norm_train
|
||||
norm_train:tools/train.py -c test_tipc/configs/layoutxlm_ser/ser_layoutxlm_xfund_zh.yml
|
||||
pact_train:null
|
||||
fpgm_train:null
|
||||
distill_train:null
|
||||
null:null
|
||||
null:null
|
||||
##
|
||||
===========================eval_params===========================
|
||||
eval:null
|
||||
null:null
|
||||
##
|
||||
===========================infer_params===========================
|
||||
Global.save_inference_dir:./output/
|
||||
Architecture.Backbone.checkpoints:
|
||||
norm_export:tools/export_model.py -c test_tipc/configs/layoutxlm_ser/ser_layoutxlm_xfund_zh.yml -o
|
||||
quant_export:
|
||||
fpgm_export:
|
||||
distill_export:null
|
||||
export1:null
|
||||
export2:null
|
||||
##
|
||||
infer_model:null
|
||||
infer_export:null
|
||||
infer_quant:False
|
||||
inference:ppstructure/kie/predict_kie_token_ser.py --kie_algorithm=LayoutXLM --ser_dict_path=train_data/XFUND/class_list_xfun.txt --output=output
|
||||
--use_gpu:True|False
|
||||
--enable_mkldnn:False
|
||||
--cpu_threads:6
|
||||
--rec_batch_num:1
|
||||
--use_tensorrt:False
|
||||
--precision:fp32
|
||||
--ser_model_dir:
|
||||
--image_dir:./ppstructure/docs/kie/input/zh_val_42.jpg
|
||||
null:null
|
||||
--benchmark:False
|
||||
null:null
|
||||
===========================infer_benchmark_params==========================
|
||||
random_infer_input:[{float32,[3,224,224]}]
|
|
@ -0,0 +1,53 @@
|
|||
===========================train_params===========================
|
||||
model_name:layoutxlm_ser
|
||||
python:python3.7
|
||||
gpu_list:0|0,1
|
||||
Global.use_gpu:True|True
|
||||
Global.auto_cast:amp
|
||||
Global.epoch_num:lite_train_lite_infer=1|whole_train_whole_infer=17
|
||||
Global.save_model_dir:./output/
|
||||
Train.loader.batch_size_per_card:lite_train_lite_infer=4|whole_train_whole_infer=8
|
||||
Architecture.Backbone.checkpoints:null
|
||||
train_model_name:latest
|
||||
train_infer_img_dir:ppstructure/docs/kie/input/zh_val_42.jpg
|
||||
null:null
|
||||
##
|
||||
trainer:norm_train
|
||||
norm_train:tools/train.py -c test_tipc/configs/layoutxlm_ser/ser_layoutxlm_xfund_zh.yml -o Global.print_batch_step=1 Global.eval_batch_step=[1000,1000] Train.loader.shuffle=false
|
||||
pact_train:null
|
||||
fpgm_train:null
|
||||
distill_train:null
|
||||
null:null
|
||||
null:null
|
||||
##
|
||||
===========================eval_params===========================
|
||||
eval:null
|
||||
null:null
|
||||
##
|
||||
===========================infer_params===========================
|
||||
Global.save_inference_dir:./output/
|
||||
Architecture.Backbone.checkpoints:
|
||||
norm_export:tools/export_model.py -c test_tipc/configs/layoutxlm_ser/ser_layoutxlm_xfund_zh.yml -o
|
||||
quant_export:
|
||||
fpgm_export:
|
||||
distill_export:null
|
||||
export1:null
|
||||
export2:null
|
||||
##
|
||||
infer_model:null
|
||||
infer_export:null
|
||||
infer_quant:False
|
||||
inference:ppstructure/kie/predict_kie_token_ser.py --kie_algorithm=LayoutXLM --ser_dict_path=train_data/XFUND/class_list_xfun.txt --output=output
|
||||
--use_gpu:True|False
|
||||
--enable_mkldnn:False
|
||||
--cpu_threads:6
|
||||
--rec_batch_num:1
|
||||
--use_tensorrt:False
|
||||
--precision:fp32
|
||||
--ser_model_dir:
|
||||
--image_dir:./ppstructure/docs/kie/input/zh_val_42.jpg
|
||||
null:null
|
||||
--benchmark:False
|
||||
null:null
|
||||
===========================infer_benchmark_params==========================
|
||||
random_infer_input:[{float32,[3,224,224]}]
|
|
@ -350,8 +350,8 @@ elif [ ${MODE} = "whole_infer" ];then
|
|||
python_name_list=$(func_parser_value "${lines[2]}")
|
||||
array=(${python_name_list})
|
||||
python_name=${array[0]}
|
||||
${python_name} -m pip install paddleslim
|
||||
${python_name} -m pip install -r requirements.txt
|
||||
${python_name} -m pip install paddleslim --force-reinstall
|
||||
${python_name} -m pip install -r requirements.txt
|
||||
wget -nc -P ./inference https://paddleocr.bj.bcebos.com/dygraph_v2.0/test/ch_det_data_50.tar --no-check-certificate
|
||||
wget -nc -P ./inference/ https://paddleocr.bj.bcebos.com/dygraph_v2.0/test/rec_inference.tar --no-check-certificate
|
||||
cd ./inference && tar xf rec_inference.tar && tar xf ch_det_data_50.tar && cd ../
|
||||
|
@ -519,10 +519,18 @@ elif [ ${MODE} = "whole_infer" ];then
|
|||
cd ./inference/ && tar xf det_r50_dcn_fce_ctw_v2.0_train.tar & cd ../
|
||||
fi
|
||||
if [[ ${model_name} =~ "en_table_structure" ]];then
|
||||
wget -nc -P ./inference/ https://paddleocr.bj.bcebos.com/dygraph_v2.0/table/en_ppocr_mobile_v2.0_table_structure_infer.tar --no-check-certificate
|
||||
wget -nc -P ./inference/ https://paddleocr.bj.bcebos.com/dygraph_v2.0/table/en_ppocr_mobile_v2.0_table_det_infer.tar --no-check-certificate
|
||||
wget -nc -P ./inference/ https://paddleocr.bj.bcebos.com/dygraph_v2.0/table/en_ppocr_mobile_v2.0_table_rec_infer.tar --no-check-certificate
|
||||
cd ./inference/ && tar xf en_ppocr_mobile_v2.0_table_structure_infer.tar && tar xf en_ppocr_mobile_v2.0_table_det_infer.tar && tar xf en_ppocr_mobile_v2.0_table_rec_infer.tar && cd ../
|
||||
|
||||
cd ./inference/ && tar xf en_ppocr_mobile_v2.0_table_det_infer.tar && tar xf en_ppocr_mobile_v2.0_table_rec_infer.tar
|
||||
if [ ${model_name} == "en_table_structure" ]; then
|
||||
wget -nc https://paddleocr.bj.bcebos.com/dygraph_v2.0/table/en_ppocr_mobile_v2.0_table_structure_infer.tar --no-check-certificate
|
||||
tar xf en_ppocr_mobile_v2.0_table_structure_infer.tar
|
||||
elif [ ${model_name} == "en_table_structure_PACT" ]; then
|
||||
wget -nc https://paddleocr.bj.bcebos.com/dygraph_v2.0/table/en_ppocr_mobile_v2.0_table_structure_slim_infer.tar --no-check-certificate
|
||||
tar xf en_ppocr_mobile_v2.0_table_structure_slim_infer.tar
|
||||
fi
|
||||
cd ../
|
||||
fi
|
||||
if [[ ${model_name} =~ "layoutxlm_ser" ]]; then
|
||||
${python_name} -m pip install -r ppstructure/kie/requirements.txt
|
||||
|
@ -691,12 +699,16 @@ if [ ${MODE} = "cpp_infer" ];then
|
|||
wget -nc -P ./inference https://paddleocr.bj.bcebos.com/dygraph_v2.0/test/ch_det_data_50.tar --no-check-certificate
|
||||
wget -nc -P ./inference https://paddleocr.bj.bcebos.com/PP-OCRv3/chinese/ch_PP-OCRv3_rec_infer.tar --no-check-certificate
|
||||
cd ./inference && tar xf ch_PP-OCRv3_det_infer.tar && tar xf ch_PP-OCRv3_rec_infer.tar && tar xf ch_det_data_50.tar && cd ../
|
||||
fi
|
||||
elif [ ${model_name} = "en_table_structure_KL" ];then
|
||||
elif [[ ${model_name} =~ "en_table_structure" ]];then
|
||||
wget -nc -P ./inference/ https://paddleocr.bj.bcebos.com/dygraph_v2.0/table/en_ppocr_mobile_v2.0_table_structure_infer.tar --no-check-certificate
|
||||
wget -nc -P ./inference/ https://paddleocr.bj.bcebos.com/dygraph_v2.0/table/en_ppocr_mobile_v2.0_table_det_infer.tar --no-check-certificate
|
||||
wget -nc -P ./inference/ https://paddleocr.bj.bcebos.com/dygraph_v2.0/table/en_ppocr_mobile_v2.0_table_rec_infer.tar --no-check-certificate
|
||||
cd ./inference/ && tar xf en_ppocr_mobile_v2.0_table_structure_infer.tar && tar xf en_ppocr_mobile_v2.0_table_det_infer.tar && tar xf en_ppocr_mobile_v2.0_table_rec_infer.tar && cd ../
|
||||
elif [[ ${model_name} =~ "slanet" ]];then
|
||||
wget -nc -P ./inference/ https://paddleocr.bj.bcebos.com/ppstructure/models/slanet/ch_ppstructure_mobile_v2.0_SLANet_infer.tar --no-check-certificate
|
||||
wget -nc -P ./inference/ https://paddleocr.bj.bcebos.com/PP-OCRv3/chinese/ch_PP-OCRv3_det_infer.tar --no-check-certificate
|
||||
wget -nc -P ./inference/ https://paddleocr.bj.bcebos.com/PP-OCRv3/chinese/ch_PP-OCRv3_rec_infer.tar --no-check-certificate
|
||||
cd ./inference/ && tar xf ch_ppstructure_mobile_v2.0_SLANet_infer.tar && tar xf ch_PP-OCRv3_det_infer.tar && tar xf ch_PP-OCRv3_rec_infer.tar && cd ../
|
||||
fi
|
||||
fi
|
||||
|
||||
|
|
|
@ -115,16 +115,12 @@ def export_single_model(model,
|
|||
max_text_length = arch_config["Head"]["max_text_length"]
|
||||
other_shape = [
|
||||
paddle.static.InputSpec(
|
||||
shape=[None, 3, 48, 160], dtype="float32"),
|
||||
|
||||
[
|
||||
paddle.static.InputSpec(
|
||||
shape=[None, ],
|
||||
dtype="float32"),
|
||||
paddle.static.InputSpec(
|
||||
shape=[None, max_text_length],
|
||||
dtype="int64")
|
||||
]
|
||||
shape=[None, 3, 48, 160], dtype="float32"), [
|
||||
paddle.static.InputSpec(
|
||||
shape=[None, ], dtype="float32"),
|
||||
paddle.static.InputSpec(
|
||||
shape=[None, max_text_length], dtype="int64")
|
||||
]
|
||||
]
|
||||
model = to_static(model, input_spec=other_shape)
|
||||
elif arch_config["algorithm"] in ["LayoutLM", "LayoutLMv2", "LayoutXLM"]:
|
||||
|
@ -140,6 +136,13 @@ def export_single_model(model,
|
|||
paddle.static.InputSpec(
|
||||
shape=[None, 3, 224, 224], dtype="int64"), # image
|
||||
]
|
||||
if 'Re' in arch_config['Backbone']['name']:
|
||||
input_spec.extend([
|
||||
paddle.static.InputSpec(
|
||||
shape=[None, 512, 3], dtype="int64"), # entities
|
||||
paddle.static.InputSpec(
|
||||
shape=[None, None, 2], dtype="int64"), # relations
|
||||
])
|
||||
if model.backbone.use_visual_backbone is False:
|
||||
input_spec.pop(4)
|
||||
model = to_static(model, input_spec=[input_spec])
|
||||
|
|
|
@ -40,7 +40,6 @@ def init_args():
|
|||
parser.add_argument("--ir_optim", type=str2bool, default=True)
|
||||
parser.add_argument("--use_tensorrt", type=str2bool, default=False)
|
||||
parser.add_argument("--min_subgraph_size", type=int, default=15)
|
||||
parser.add_argument("--shape_info_filename", type=str, default=None)
|
||||
parser.add_argument("--precision", type=str, default="fp32")
|
||||
parser.add_argument("--gpu_mem", type=int, default=500)
|
||||
|
||||
|
@ -163,6 +162,8 @@ def create_predictor(args, mode, logger):
|
|||
model_dir = args.table_model_dir
|
||||
elif mode == 'ser':
|
||||
model_dir = args.ser_model_dir
|
||||
elif mode == 're':
|
||||
model_dir = args.re_model_dir
|
||||
elif mode == "sr":
|
||||
model_dir = args.sr_model_dir
|
||||
elif mode == 'layout':
|
||||
|
@ -228,22 +229,19 @@ def create_predictor(args, mode, logger):
|
|||
use_calib_mode=False)
|
||||
|
||||
# collect shape
|
||||
trt_shape_f = f"{os.path.dirname(args.shape_info_filename)}/{mode}_{os.path.basename(args.shape_info_filename)}"
|
||||
if trt_shape_f is not None:
|
||||
if not os.path.exists(trt_shape_f):
|
||||
config.collect_shape_range_info(trt_shape_f)
|
||||
logger.info(
|
||||
f"collect dynamic shape info into : {trt_shape_f}"
|
||||
)
|
||||
else:
|
||||
logger.info(
|
||||
f"dynamic shape info file( {trt_shape_f} ) already exists, not need to generate again."
|
||||
)
|
||||
config.enable_tuned_tensorrt_dynamic_shape(trt_shape_f, True)
|
||||
else:
|
||||
trt_shape_f = os.path.join(model_dir,
|
||||
f"{mode}_trt_dynamic_shape.txt")
|
||||
|
||||
if not os.path.exists(trt_shape_f):
|
||||
config.collect_shape_range_info(trt_shape_f)
|
||||
logger.info(
|
||||
f"when using tensorrt, dynamic shape is a suggested option, you can use '--shape_info_filename=shape.txt' for offline dygnamic shape tuning"
|
||||
)
|
||||
f"collect dynamic shape info into : {trt_shape_f}")
|
||||
try:
|
||||
config.enable_tuned_tensorrt_dynamic_shape(trt_shape_f,
|
||||
True)
|
||||
except Exception as E:
|
||||
logger.info(E)
|
||||
logger.info("Please keep your paddlepaddle-gpu >= 2.3.0!")
|
||||
|
||||
elif args.use_npu:
|
||||
config.enable_npu()
|
||||
|
@ -267,6 +265,8 @@ def create_predictor(args, mode, logger):
|
|||
config.disable_glog_info()
|
||||
config.delete_pass("conv_transpose_eltwiseadd_bn_fuse_pass")
|
||||
config.delete_pass("matmul_transpose_reshape_fuse_pass")
|
||||
if mode == 're':
|
||||
config.delete_pass("simplify_with_basic_ops_pass")
|
||||
if mode == 'table':
|
||||
config.delete_pass("fc_fuse_pass") # not supported for table
|
||||
config.switch_use_feed_fetch_ops(False)
|
||||
|
|
|
@ -63,7 +63,7 @@ class ReArgsParser(ArgsParser):
|
|||
|
||||
def make_input(ser_inputs, ser_results):
|
||||
entities_labels = {'HEADER': 0, 'QUESTION': 1, 'ANSWER': 2}
|
||||
|
||||
batch_size, max_seq_len = ser_inputs[0].shape[:2]
|
||||
entities = ser_inputs[8][0]
|
||||
ser_results = ser_results[0]
|
||||
assert len(entities) == len(ser_results)
|
||||
|
@ -80,34 +80,44 @@ def make_input(ser_inputs, ser_results):
|
|||
start.append(entity['start'])
|
||||
end.append(entity['end'])
|
||||
label.append(entities_labels[res['pred']])
|
||||
entities = dict(start=start, end=end, label=label)
|
||||
|
||||
entities = np.full([max_seq_len + 1, 3], fill_value=-1)
|
||||
entities[0, 0] = len(start)
|
||||
entities[1:len(start) + 1, 0] = start
|
||||
entities[0, 1] = len(end)
|
||||
entities[1:len(end) + 1, 1] = end
|
||||
entities[0, 2] = len(label)
|
||||
entities[1:len(label) + 1, 2] = label
|
||||
|
||||
# relations
|
||||
head = []
|
||||
tail = []
|
||||
for i in range(len(entities["label"])):
|
||||
for j in range(len(entities["label"])):
|
||||
if entities["label"][i] == 1 and entities["label"][j] == 2:
|
||||
for i in range(len(label)):
|
||||
for j in range(len(label)):
|
||||
if label[i] == 1 and label[j] == 2:
|
||||
head.append(i)
|
||||
tail.append(j)
|
||||
|
||||
relations = dict(head=head, tail=tail)
|
||||
relations = np.full([len(head) + 1, 2], fill_value=-1)
|
||||
relations[0, 0] = len(head)
|
||||
relations[1:len(head) + 1, 0] = head
|
||||
relations[0, 1] = len(tail)
|
||||
relations[1:len(tail) + 1, 1] = tail
|
||||
|
||||
entities = np.expand_dims(entities, axis=0)
|
||||
entities = np.repeat(entities, batch_size, axis=0)
|
||||
relations = np.expand_dims(relations, axis=0)
|
||||
relations = np.repeat(relations, batch_size, axis=0)
|
||||
|
||||
# remove ocr_info segment_offset_id and label in ser input
|
||||
if isinstance(ser_inputs[0], paddle.Tensor):
|
||||
entities = paddle.to_tensor(entities)
|
||||
relations = paddle.to_tensor(relations)
|
||||
ser_inputs = ser_inputs[:5] + [entities, relations]
|
||||
|
||||
batch_size = ser_inputs[0].shape[0]
|
||||
entities_batch = []
|
||||
relations_batch = []
|
||||
entity_idx_dict_batch = []
|
||||
for b in range(batch_size):
|
||||
entities_batch.append(entities)
|
||||
relations_batch.append(relations)
|
||||
entity_idx_dict_batch.append(entity_idx_dict)
|
||||
|
||||
ser_inputs[8] = entities_batch
|
||||
ser_inputs.append(relations_batch)
|
||||
# remove ocr_info segment_offset_id and label in ser input
|
||||
ser_inputs.pop(7)
|
||||
ser_inputs.pop(6)
|
||||
ser_inputs.pop(5)
|
||||
return ser_inputs, entity_idx_dict_batch
|
||||
|
||||
|
||||
|
@ -136,6 +146,8 @@ class SerRePredictor(object):
|
|||
def __call__(self, data):
|
||||
ser_results, ser_inputs = self.ser_engine(data)
|
||||
re_input, entity_idx_dict_batch = make_input(ser_inputs, ser_results)
|
||||
if self.model.backbone.use_visual_backbone is False:
|
||||
re_input.pop(4)
|
||||
preds = self.model(re_input)
|
||||
post_result = self.post_process_class(
|
||||
preds,
|
||||
|
|
Loading…
Reference in New Issue