* Update recognition_en.md (#10059) ic15_dict.txt only have 36 digits * Update ocr_rec.h (#9469) It is enough to include preprocess_op.h, we do not need to include ocr_cls.h. * 补充num_classes注释说明 (#10073) ser_vi_layoutxlm_xfund_zh.yml中的Architecture.Backbone.num_classes所赋值会设置给Loss.num_classes, 由于采用BIO标注,假设字典中包含n个字段(包含other)时,则类别数为2n-1;假设字典中包含n个字段(不含other)时,则类别数为2n+1。 * Update algorithm_overview_en.md (#9747) Fix links to super-resolution algorithm docs * 改进文档`deploy/hubserving/readme.md`和`doc/doc_ch/models_list.md` (#9110) * Update readme.md * Update readme.md * Update readme.md * Update models_list.md * trim trailling spaces @ `deploy/hubserving/readme_en.md` * `s/shell/bash/` @ `deploy/hubserving/readme_en.md` * Update `deploy/hubserving/readme_en.md` to sync with `deploy/hubserving/readme.md` * Update deploy/hubserving/readme_en.md to sync with `deploy/hubserving/readme.md` * Update deploy/hubserving/readme_en.md to sync with `deploy/hubserving/readme.md` * Update `doc/doc_en/models_list_en.md` to sync with `doc/doc_ch/models_list_en.md` * using Grammarly to weak `deploy/hubserving/readme_en.md` * using Grammarly to tweak `doc/doc_en/models_list_en.md` * `ocr_system` module will return with values of field `confidence` * Update README_CN.md * 修复测试服务中图片转Base64的引用地址错误。 (#8334) * Update application.md * [Doc] Fix 404 link. (#10318) * Update PP-OCRv3_det_train.md * Update knowledge_distillation.md * Update config.md * Fix fitz camelCase deprecation and .PDF not being recognized as pdf file (#10181) * Fix fitz camelCase deprecation and .PDF not being recognized as pdf file * refactor get_image_file_list function * Update customize.md (#10325) * Update FAQ.md (#10345) * Update FAQ.md (#10349) * Don't break overall processing on a bad image (#10216) * Add preprocessing common to OCR tasks (#10217) Add preprocessing to options * [MLU] add mlu device for infer (#10249) * Create newfeature.md * Update newfeature.md * remove unused imported module, so can avoid PyInstaller packaged binary's start-time not found module error. (#10502) * CV套件建设专项活动 - 文字识别返回单字识别坐标 (#10515) * modification of return word box * update_implements * Update rec_postprocess.py * Update utility.py * Update README_ch.md * revert README_ch.md update * Fixed Layout recovery README file (#10493) Co-authored-by: Shubham Chambhare <shubhamchambhare@zoop.one> * update_doc * bugfix --------- Co-authored-by: ChuongLoc <89434232+ChuongLoc@users.noreply.github.com> Co-authored-by: Wang Xin <xinwang614@gmail.com> Co-authored-by: tanjh <dtdhinjapan@gmail.com> Co-authored-by: Louis Maddox <lmmx@users.noreply.github.com> Co-authored-by: n0099 <n@n0099.net> Co-authored-by: zhenliang li <37922155+shouyong@users.noreply.github.com> Co-authored-by: itasli <ilyas.tasli@outlook.fr> Co-authored-by: UserUnknownFactor <63057995+UserUnknownFactor@users.noreply.github.com> Co-authored-by: PeiyuLau <135964669+PeiyuLau@users.noreply.github.com> Co-authored-by: kerneltravel <kjpioo2006@gmail.com> Co-authored-by: ToddBear <43341135+ToddBear@users.noreply.github.com> Co-authored-by: Ligoml <39876205+Ligoml@users.noreply.github.com> Co-authored-by: Shubham Chambhare <59397280+Shubham654@users.noreply.github.com> Co-authored-by: Shubham Chambhare <shubhamchambhare@zoop.one> Co-authored-by: andyj <87074272+andyjpaddle@users.noreply.github.com> |
||
---|---|---|
.. | ||
README.md | ||
README_ch.md | ||
__init__.py | ||
recovery_to_doc.py | ||
requirements.txt | ||
table_process.py |
README.md
English | 简体中文
Layout Recovery
- 1. Introduction
- 2. Install
- 3. Quick Start using standard PDF parse
- 4. Quick Start using image format PDF parse
- 5. More
1. Introduction
The layout recovery module is used to restore the image or pdf to an editable Word file consistent with the original image layout.
Two layout recovery methods are provided, you can choose by PDF format:
-
Standard PDF parse(the input is standard PDF): Python based PDF to word library [pdf2docx] (https://github.com/dothinking/pdf2docx) is optimized, the method extracts data from PDF with PyMuPDF, then parse layout with rule, finally, generate docx with python-docx.
-
Image format PDF parse(the input can be standard PDF or image format PDF): Layout recovery combines layout analysis、table recognition to better recover images, tables, titles, etc. supports input files in PDF and document image formats in Chinese and English.
The input formats and application scenarios of the two methods are as follows:
method | input formats | application scenarios/problem |
---|---|---|
Standard PDF parse | Advantages: Better recovery for non-paper documents, each page remains on the same page after restoration Disadvantages: English characters in some Chinese documents are garbled, some contents are still beyond the current page, the whole page content is restored to the table format, and the recovery effect of some pictures is not good |
|
Image format PDF parse( | pdf、picture | Advantages: More suitable for paper document content recovery, OCR recognition effect is more good Disadvantages: Currently, the recovery is based on rules, the effect of content typesetting (spacing, fonts, etc.) need to be further improved, and the effect of layout recovery depends on layout analysis |
The following figure shows the effect of restoring the layout of documents by using PDF parse:
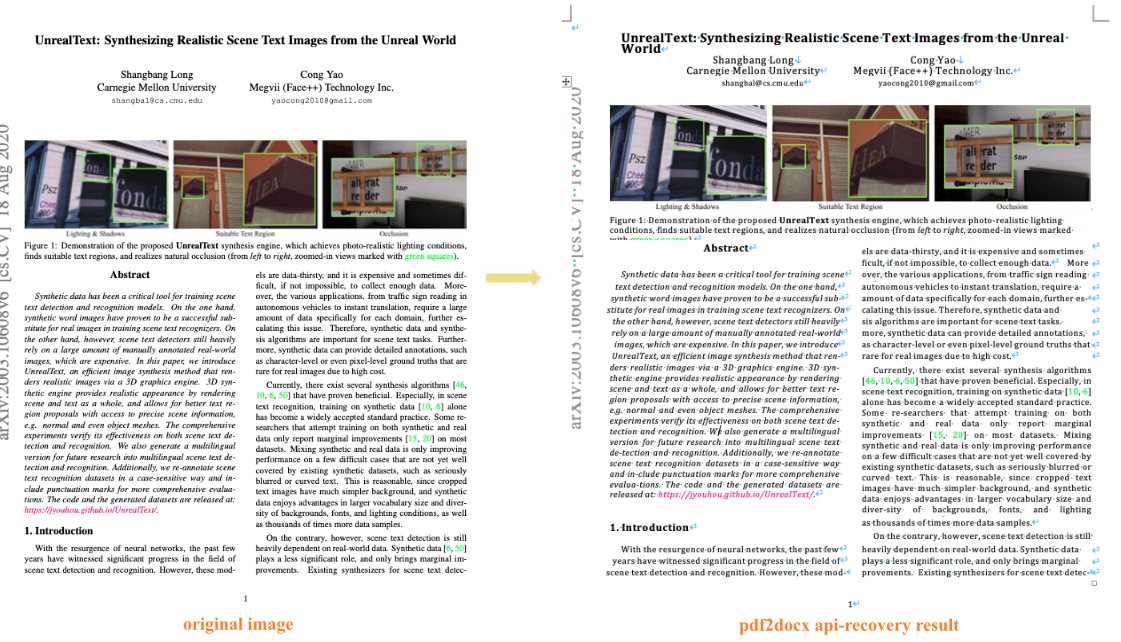
The following figures show the effect of restoring the layout of English and Chinese documents by using OCR technique:


2. Install
2.1 Install PaddlePaddle
python3 -m pip install --upgrade pip
# If you have cuda9 or cuda10 installed on your machine, please run the following command to install
python3 -m pip install "paddlepaddle-gpu" -i https://mirror.baidu.com/pypi/simple
# CPU installation
python3 -m pip install "paddlepaddle" -i https://mirror.baidu.com/pypi/simple
For more requirements, please refer to the instructions in Installation Documentation.
2.2 Install PaddleOCR
- (1) Download source code
[Recommended] git clone https://github.com/PaddlePaddle/PaddleOCR
# If the pull cannot be successful due to network problems, you can also choose to use the hosting on the code cloud:
git clone https://gitee.com/paddlepaddle/PaddleOCR
# Note: Code cloud hosting code may not be able to synchronize the update of this github project in real time, there is a delay of 3 to 5 days, please use the recommended method first.
- (2) Install recovery
requirements
The layout restoration is exported as docx files, so python-docx API need to be installed, and PyMuPDF api(requires Python >= 3.7) need to be installed to process the input files in pdf format.
Install all the libraries by running the following command:
python3 -m pip install -r ppstructure/recovery/requirements.txt
And if using pdf parse method, we need to install pdf2docx api.
wget https://paddleocr.bj.bcebos.com/whl/pdf2docx-0.0.0-py3-none-any.whl
pip3 install pdf2docx-0.0.0-py3-none-any.whl
3. Quick Start using standard PDF parse
use_pdf2docx_api
use PDF parse for layout recovery, The whl package is also provided for quick use, follow the above code, for more infomation please refer to quickstart for details.
# install paddleocr
pip3 install "paddleocr>=2.6"
paddleocr --image_dir=ppstructure/recovery/UnrealText.pdf --type=structure --recovery=true --use_pdf2docx_api=true
Command line:
python3 predict_system.py \
--image_dir=ppstructure/recovery/UnrealText.pdf \
--recovery=True \
--use_pdf2docx_api=True \
--output=../output/
4. Quick Start using image format PDF parse
Through layout analysis, we divided the image/PDF documents into regions, located the key regions, such as text, table, picture, etc., and recorded the location, category, and regional pixel value information of each region. Different regions are processed separately, where:
-
OCR detection and recognition is performed in the text area, and the coordinates of the OCR detection box and the text content information are added on the basis of the previous information
-
The table area identifies tables and records html and text information of tables
-
Save the image directly
We can restore the test picture through the layout information, OCR detection and recognition structure, table information, and saved pictures.
The whl package is also provided for quick use, follow the above code, for more infomation please refer to quickstart for details.
paddleocr --image_dir=ppstructure/docs/table/1.png --type=structure --recovery=true --lang='en'
4.1 Download models
If input is English document, download English models:
cd PaddleOCR/ppstructure
# download model
mkdir inference && cd inference
# Download the detection model of the ultra-lightweight English PP-OCRv3 model and unzip it
wget https://paddleocr.bj.bcebos.com/PP-OCRv3/english/en_PP-OCRv3_det_infer.tar && tar xf en_PP-OCRv3_det_infer.tar
# Download the recognition model of the ultra-lightweight English PP-OCRv3 model and unzip it
wget https://paddleocr.bj.bcebos.com/PP-OCRv3/english/en_PP-OCRv3_rec_infer.tar && tar xf en_PP-OCRv3_rec_infer.tar
# Download the ultra-lightweight English table inch model and unzip it
wget https://paddleocr.bj.bcebos.com/ppstructure/models/slanet/en_ppstructure_mobile_v2.0_SLANet_infer.tar
tar xf en_ppstructure_mobile_v2.0_SLANet_infer.tar
# Download the layout model of publaynet dataset and unzip it
wget https://paddleocr.bj.bcebos.com/ppstructure/models/layout/picodet_lcnet_x1_0_fgd_layout_infer.tar
tar xf picodet_lcnet_x1_0_fgd_layout_infer.tar
cd ..
If input is Chinese document,download Chinese models: Chinese and English ultra-lightweight PP-OCRv3 model、table recognition model、layout analysis model
4.2 Layout recovery
python3 predict_system.py \
--image_dir=./docs/table/1.png \
--det_model_dir=inference/en_PP-OCRv3_det_infer \
--rec_model_dir=inference/en_PP-OCRv3_rec_infer \
--rec_char_dict_path=../ppocr/utils/en_dict.txt \
--table_model_dir=inference/en_ppstructure_mobile_v2.0_SLANet_infer \
--table_char_dict_path=../ppocr/utils/dict/table_structure_dict.txt \
--layout_model_dir=inference/picodet_lcnet_x1_0_fgd_layout_infer \
--layout_dict_path=../ppocr/utils/dict/layout_dict/layout_publaynet_dict.txt \
--vis_font_path=../doc/fonts/simfang.ttf \
--recovery=True \
--output=../output/
After running, the docx of each picture will be saved in the directory specified by the output field
Field:
- image_dir:test file, can be picture, picture directory, pdf file, pdf file directory
- det_model_dir:OCR detection model path
- rec_model_dir:OCR recognition model path
- rec_char_dict_path:OCR recognition dict path. If the Chinese model is used, change to "../ppocr/utils/ppocr_keys_v1.txt". And if you trained the model on your own dataset, change to the trained dictionary
- table_model_dir:tabel recognition model path
- table_char_dict_path:tabel recognition dict path. If the Chinese model is used, no need to change
- layout_model_dir:layout analysis model path
- layout_dict_path:layout analysis dict path. If the Chinese model is used, change to "../ppocr/utils/dict/layout_dict/layout_cdla_dict.txt"
- recovery:whether to enable layout of recovery, default False
- output:save the recovery result path
5. More
For training, evaluation and inference tutorial for text detection models, please refer to text detection doc.
For training, evaluation and inference tutorial for text recognition models, please refer to text recognition doc.
For training, evaluation and inference tutorial for layout analysis models, please refer to layout analysis doc
For training, evaluation and inference tutorial for table recognition models, please refer to table recognition doc