|
||
---|---|---|
configs | ||
demo | ||
docker | ||
docs | ||
local_configs | ||
mmseg | ||
requirements | ||
resources | ||
tests | ||
tools | ||
.gitignore | ||
LICENSE | ||
README.md | ||
pytest.ini | ||
requirements.txt | ||
setup.cfg | ||
setup.py |
README.md
SegFormer: Simple and Efficient Design for Semantic Segmentation with Transformers
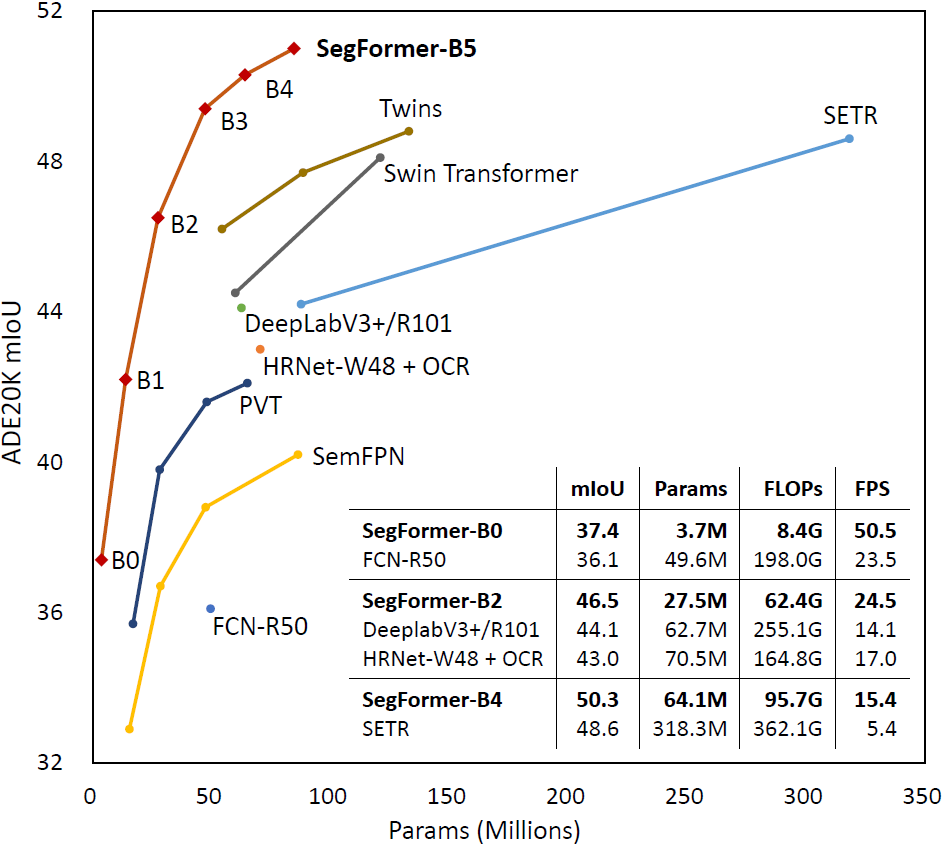
Figure 1: Performance of SegFormer-B0 to SegFormer-B5.
Project page | Paper | Demo (Youtube) | Demo (Bilibili)
SegFormer: Simple and Efficient Design for Semantic Segmentation with Transformers.
Enze Xie, Wenhai Wang, Zhiding Yu, Anima Anandkumar, Jose M. Alvarez, and Ping Luo.
Technical Report 2021.
This repository contains the PyTorch training/evaluation code and the pretrained models for SegFormer.
SegFormer is a simple, efficient and powerful semantic segmentation method, as shown in Figure 1.
We use MMSegmentation v0.13.0 as the codebase.
Installation
For install and data preparation, please refer to the guidelines in MMSegmentation v0.13.0.
Other requirements:
pip install timm==0.3.2
An example (works for me): CUDA 10.1
and pytorch 1.7.1
pip install torchvision==0.8.2
pip install timm==0.3.2
pip install mmcv-full==1.2.7
pip install opencv-python==4.5.1.48
cd SegFormer && pip install -e . --user
Evaluation
Download trained weights.
Example: evaluate SegFormer-B1
on ADE20K
:
# Single-gpu testing
python tools/test.py local_configs/segformer/B1/segformer.b1.512x512.ade.160k.py /path/to/checkpoint_file
# Multi-gpu testing
./tools/dist_test.sh local_configs/segformer/B1/segformer.b1.512x512.ade.160k.py /path/to/checkpoint_file <GPU_NUM>
# Multi-gpu, multi-scale testing
tools/dist_test.sh local_configs/segformer/B1/segformer.b1.512x512.ade.160k.py /path/to/checkpoint_file <GPU_NUM> --aug-test
Training
Download weights pretrained on ImageNet-1K, and put them in a folder pretrained/
.
Example: train SegFormer-B1
on ADE20K
:
# Single-gpu training
python tools/train.py local_configs/segformer/B1/segformer.b1.512x512.ade.160k.py
# Multi-gpu training
./tools/dist_train.sh local_configs/segformer/B1/segformer.b1.512x512.ade.160k.py <GPU_NUM>
License
Please check the LICENSE file. SegFormer may be used non-commercially, meaning for research or evaluation purposes only. For business inquiries, please contact researchinquiries@nvidia.com.
Citation
@article{xie2021segformer,
title={SegFormer: Simple and Efficient Design for Semantic Segmentation with Transformers},
author={Xie, Enze and Wang, Wenhai and Yu, Zhiding and Anandkumar, Anima and Alvarez, Jose M and Luo, Ping},
journal={arXiv preprint arXiv:2105.15203},
year={2021}
}