mirror of
https://github.com/JDAI-CV/fast-reid.git
synced 2025-06-03 14:50:47 +08:00
Merge c7ced7a5d1571a8d99b28a2ff3fa9e6f4e2ec8e6 into 31d99b793fe0937461b9c9bc8a8a11f88bf5642c
This commit is contained in:
commit
2c1f44ef4a
@ -8,3 +8,11 @@ You can run this command to get cosine similarites between different images
|
|||||||
cd demo/
|
cd demo/
|
||||||
sh run_demo.sh
|
sh run_demo.sh
|
||||||
```
|
```
|
||||||
|
|
||||||
|
What is more, you can use this command to make thing more interesting
|
||||||
|
```bash
|
||||||
|
export CUDA_VISIBLE_DEVICES=0
|
||||||
|
python3 demo/visualize_result.py --config-file ./configs/VeRi/sbs_R50-ibn.yml --actmap --dataset-name 'VeRi' --output logs/veri/sbs_R50-ibn/eval --opts MODEL.WEIGHTS logs/veri/sbs_R50-ibn/model_best.pth
|
||||||
|
```
|
||||||
|
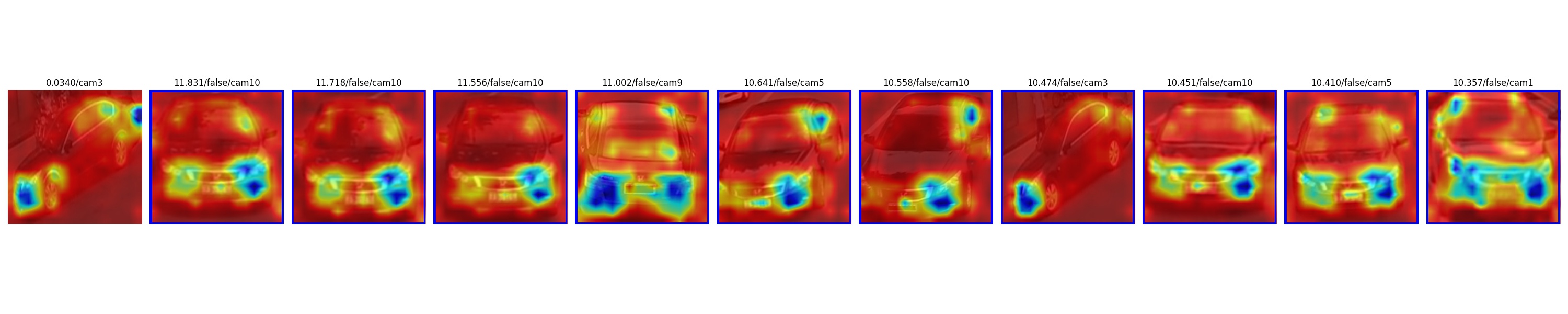
|
||||||
|
where `--actmap` is used to add activation map upon the original image.
|
||||||
|
@ -9,6 +9,9 @@ import bisect
|
|||||||
from collections import deque
|
from collections import deque
|
||||||
|
|
||||||
import cv2
|
import cv2
|
||||||
|
import numpy as np
|
||||||
|
import torch.nn.functional as F
|
||||||
|
|
||||||
import torch
|
import torch
|
||||||
import torch.multiprocessing as mp
|
import torch.multiprocessing as mp
|
||||||
|
|
||||||
@ -57,6 +60,31 @@ class FeatureExtractionDemo(object):
|
|||||||
predictions = self.predictor(image)
|
predictions = self.predictor(image)
|
||||||
return predictions
|
return predictions
|
||||||
|
|
||||||
|
|
||||||
|
def get_actmap(self, features, sz):
|
||||||
|
"""
|
||||||
|
:param features: (1, 2048, 16, 8) activation map
|
||||||
|
:return:
|
||||||
|
"""
|
||||||
|
features = (features ** 2).sum(1) # (1, 16, 8)
|
||||||
|
b, h, w = features.size()
|
||||||
|
features = features.view(b, h * w)
|
||||||
|
features = F.normalize(features, p=2, dim=1)
|
||||||
|
acts = features.view(b, h, w)
|
||||||
|
all_acts = []
|
||||||
|
for i in range(b):
|
||||||
|
act = acts[i].numpy()
|
||||||
|
act = cv2.resize(act, (sz[1], sz[0]))
|
||||||
|
# act = 255 * (act - act.max()) / (act.max() - act.min() + 1e-12)
|
||||||
|
act = 255 * (act - act.min()) / (act.max() - act.min() + 1e-12)
|
||||||
|
|
||||||
|
act = np.uint8(np.floor(act))
|
||||||
|
act = cv2.applyColorMap(act, cv2.COLORMAP_JET)
|
||||||
|
|
||||||
|
all_acts.append(act)
|
||||||
|
return all_acts
|
||||||
|
|
||||||
|
|
||||||
def run_on_loader(self, data_loader):
|
def run_on_loader(self, data_loader):
|
||||||
if self.parallel:
|
if self.parallel:
|
||||||
buffer_size = self.predictor.default_buffer_size
|
buffer_size = self.predictor.default_buffer_size
|
||||||
@ -78,8 +106,22 @@ class FeatureExtractionDemo(object):
|
|||||||
yield predictions, batch["targets"].cpu().numpy(), batch["camids"].cpu().numpy()
|
yield predictions, batch["targets"].cpu().numpy(), batch["camids"].cpu().numpy()
|
||||||
else:
|
else:
|
||||||
for batch in data_loader:
|
for batch in data_loader:
|
||||||
|
# add hook here to get features: start
|
||||||
|
act_outputs = []
|
||||||
|
def hook_fns_forward(module, input, output):
|
||||||
|
act_outputs.append(output.cpu())
|
||||||
|
handle = self.predictor.model.backbone.register_forward_hook(hook_fns_forward)
|
||||||
|
# add hook here to get features: end
|
||||||
|
|
||||||
predictions = self.predictor(batch["images"])
|
predictions = self.predictor(batch["images"])
|
||||||
yield predictions, batch["targets"].cpu().numpy(), batch["camids"].cpu().numpy()
|
|
||||||
|
# add hook here to get features: start
|
||||||
|
handle.remove()
|
||||||
|
sz = list(batch["images"].shape[-2:])
|
||||||
|
acts = self.get_actmap(act_outputs[0], sz)
|
||||||
|
# add hook here to get features: end
|
||||||
|
|
||||||
|
yield predictions, batch["targets"].cpu().numpy(), batch["camids"].cpu().numpy(), acts
|
||||||
|
|
||||||
|
|
||||||
class AsyncPredictor:
|
class AsyncPredictor:
|
||||||
|
@ -55,6 +55,11 @@ def get_parser():
|
|||||||
action='store_true',
|
action='store_true',
|
||||||
help='if use multiprocess for feature extraction.'
|
help='if use multiprocess for feature extraction.'
|
||||||
)
|
)
|
||||||
|
parser.add_argument(
|
||||||
|
'--actmap',
|
||||||
|
action='store_true',
|
||||||
|
help='if use activation map to overlap the image.'
|
||||||
|
)
|
||||||
parser.add_argument(
|
parser.add_argument(
|
||||||
"--dataset-name",
|
"--dataset-name",
|
||||||
help="a test dataset name for visualizing ranking list."
|
help="a test dataset name for visualizing ranking list."
|
||||||
@ -72,6 +77,7 @@ def get_parser():
|
|||||||
)
|
)
|
||||||
parser.add_argument(
|
parser.add_argument(
|
||||||
"--num-vis",
|
"--num-vis",
|
||||||
|
type=int,
|
||||||
default=100,
|
default=100,
|
||||||
help="number of query images to be visualized",
|
help="number of query images to be visualized",
|
||||||
)
|
)
|
||||||
@ -87,6 +93,7 @@ def get_parser():
|
|||||||
)
|
)
|
||||||
parser.add_argument(
|
parser.add_argument(
|
||||||
"--max-rank",
|
"--max-rank",
|
||||||
|
type=int,
|
||||||
default=10,
|
default=10,
|
||||||
help="maximum number of rank list to be visualized",
|
help="maximum number of rank list to be visualized",
|
||||||
)
|
)
|
||||||
@ -109,10 +116,12 @@ if __name__ == '__main__':
|
|||||||
feats = []
|
feats = []
|
||||||
pids = []
|
pids = []
|
||||||
camids = []
|
camids = []
|
||||||
for (feat, pid, camid) in tqdm.tqdm(demo.run_on_loader(test_loader), total=len(test_loader)):
|
acts_list = []
|
||||||
|
for (feat, pid, camid, acts) in tqdm.tqdm(demo.run_on_loader(test_loader), total=len(test_loader)):
|
||||||
feats.append(feat)
|
feats.append(feat)
|
||||||
pids.extend(pid)
|
pids.extend(pid)
|
||||||
camids.extend(camid)
|
camids.extend(camid)
|
||||||
|
acts_list.extend(acts)
|
||||||
|
|
||||||
feats = torch.cat(feats, dim=0)
|
feats = torch.cat(feats, dim=0)
|
||||||
q_feat = feats[:num_query]
|
q_feat = feats[:num_query]
|
||||||
@ -131,7 +140,7 @@ if __name__ == '__main__':
|
|||||||
logger.info("Finish computing APs for all query images!")
|
logger.info("Finish computing APs for all query images!")
|
||||||
|
|
||||||
visualizer = Visualizer(test_loader.dataset)
|
visualizer = Visualizer(test_loader.dataset)
|
||||||
visualizer.get_model_output(all_ap, distmat, q_pids, g_pids, q_camids, g_camids)
|
visualizer.get_model_output(all_ap, distmat, q_pids, g_pids, q_camids, g_camids, acts_list)
|
||||||
|
|
||||||
logger.info("Start saving ROC curve ...")
|
logger.info("Start saving ROC curve ...")
|
||||||
fpr, tpr, pos, neg = visualizer.vis_roc_curve(args.output)
|
fpr, tpr, pos, neg = visualizer.vis_roc_curve(args.output)
|
||||||
@ -140,5 +149,5 @@ if __name__ == '__main__':
|
|||||||
|
|
||||||
logger.info("Saving rank list result ...")
|
logger.info("Saving rank list result ...")
|
||||||
query_indices = visualizer.vis_rank_list(args.output, args.vis_label, args.num_vis,
|
query_indices = visualizer.vis_rank_list(args.output, args.vis_label, args.num_vis,
|
||||||
args.rank_sort, args.label_sort, args.max_rank)
|
args.rank_sort, args.label_sort, args.max_rank, args.actmap)
|
||||||
logger.info("Finish saving rank list results!")
|
logger.info("Finish saving rank list results!")
|
||||||
|
@ -7,6 +7,8 @@
|
|||||||
import os
|
import os
|
||||||
import pickle
|
import pickle
|
||||||
import random
|
import random
|
||||||
|
import cv2
|
||||||
|
import torch.nn.functional as F
|
||||||
|
|
||||||
import matplotlib.pyplot as plt
|
import matplotlib.pyplot as plt
|
||||||
import numpy as np
|
import numpy as np
|
||||||
@ -23,7 +25,7 @@ class Visualizer:
|
|||||||
def __init__(self, dataset):
|
def __init__(self, dataset):
|
||||||
self.dataset = dataset
|
self.dataset = dataset
|
||||||
|
|
||||||
def get_model_output(self, all_ap, dist, q_pids, g_pids, q_camids, g_camids):
|
def get_model_output(self, all_ap, dist, q_pids, g_pids, q_camids, g_camids, acts=None):
|
||||||
self.all_ap = all_ap
|
self.all_ap = all_ap
|
||||||
self.dist = dist
|
self.dist = dist
|
||||||
self.sim = 1 - dist
|
self.sim = 1 - dist
|
||||||
@ -37,6 +39,8 @@ class Visualizer:
|
|||||||
|
|
||||||
self.num_query = len(q_pids)
|
self.num_query = len(q_pids)
|
||||||
|
|
||||||
|
if acts: self.acts = acts
|
||||||
|
|
||||||
def get_matched_result(self, q_index):
|
def get_matched_result(self, q_index):
|
||||||
q_pid = self.q_pids[q_index]
|
q_pid = self.q_pids[q_index]
|
||||||
q_camid = self.q_camids[q_index]
|
q_camid = self.q_camids[q_index]
|
||||||
@ -65,7 +69,19 @@ class Visualizer:
|
|||||||
query_img = np.rollaxis(np.asarray(query_img.numpy(), dtype=np.uint8), 0, 3)
|
query_img = np.rollaxis(np.asarray(query_img.numpy(), dtype=np.uint8), 0, 3)
|
||||||
plt.clf()
|
plt.clf()
|
||||||
ax = fig.add_subplot(1, max_rank + 1, 1)
|
ax = fig.add_subplot(1, max_rank + 1, 1)
|
||||||
ax.imshow(query_img)
|
|
||||||
|
# ax.imshow(query_img)
|
||||||
|
# added: show acts
|
||||||
|
if actmap:
|
||||||
|
query_acts = self.acts[q_idx]
|
||||||
|
overlapped = query_img*0.3 + query_acts*0.7
|
||||||
|
overlapped[overlapped > 255] = 255
|
||||||
|
overlapped = overlapped.astype(np.uint8)
|
||||||
|
ax.imshow(overlapped)
|
||||||
|
# added: show acts
|
||||||
|
else:
|
||||||
|
ax.imshow(query_img)
|
||||||
|
|
||||||
ax.set_title('{:.4f}/cam{}'.format(self.all_ap[q_idx], cam_id))
|
ax.set_title('{:.4f}/cam{}'.format(self.all_ap[q_idx], cam_id))
|
||||||
ax.axis("off")
|
ax.axis("off")
|
||||||
for i in range(max_rank):
|
for i in range(max_rank):
|
||||||
@ -89,27 +105,21 @@ class Visualizer:
|
|||||||
ax.add_patch(plt.Rectangle(xy=(0, 0), width=gallery_img.shape[1] - 1,
|
ax.add_patch(plt.Rectangle(xy=(0, 0), width=gallery_img.shape[1] - 1,
|
||||||
height=gallery_img.shape[0] - 1,
|
height=gallery_img.shape[0] - 1,
|
||||||
edgecolor=(0, 0, 1), fill=False, linewidth=5))
|
edgecolor=(0, 0, 1), fill=False, linewidth=5))
|
||||||
ax.imshow(gallery_img)
|
|
||||||
|
# added: show acts
|
||||||
|
if actmap:
|
||||||
|
gallery_acts = self.acts[g_idx]
|
||||||
|
overlapped = gallery_img*0.3 + gallery_acts*0.7
|
||||||
|
overlapped[overlapped > 255] = 255
|
||||||
|
overlapped = overlapped.astype(np.uint8)
|
||||||
|
ax.imshow(overlapped)
|
||||||
|
# added: show acts
|
||||||
|
else:
|
||||||
|
ax.imshow(gallery_img)
|
||||||
|
|
||||||
ax.set_title(f'{self.sim[q_idx, sort_idx[i]]:.3f}/{label}/cam{cam_id}')
|
ax.set_title(f'{self.sim[q_idx, sort_idx[i]]:.3f}/{label}/cam{cam_id}')
|
||||||
ax.axis("off")
|
ax.axis("off")
|
||||||
# if actmap:
|
|
||||||
# act_outputs = []
|
|
||||||
#
|
|
||||||
# def hook_fns_forward(module, input, output):
|
|
||||||
# act_outputs.append(output.cpu())
|
|
||||||
#
|
|
||||||
# all_imgs = np.stack(all_imgs, axis=0) # (b, 3, h, w)
|
|
||||||
# all_imgs = torch.from_numpy(all_imgs).float()
|
|
||||||
# # normalize
|
|
||||||
# all_imgs = all_imgs.sub_(self.mean).div_(self.std)
|
|
||||||
# sz = list(all_imgs.shape[-2:])
|
|
||||||
# handle = m.base.register_forward_hook(hook_fns_forward)
|
|
||||||
# with torch.no_grad():
|
|
||||||
# _ = m(all_imgs.cuda())
|
|
||||||
# handle.remove()
|
|
||||||
# acts = self.get_actmap(act_outputs[0], sz)
|
|
||||||
# for i in range(top + 1):
|
|
||||||
# axes.flat[i].imshow(acts[i], alpha=0.3, cmap='jet')
|
|
||||||
if vis_label:
|
if vis_label:
|
||||||
label_indice = np.where(cmc == 1)[0]
|
label_indice = np.where(cmc == 1)[0]
|
||||||
if label_sort == "ascending": label_indice = label_indice[::-1]
|
if label_sort == "ascending": label_indice = label_indice[::-1]
|
||||||
@ -257,22 +267,3 @@ class Visualizer:
|
|||||||
# plt.xticks(np.arange(0.1, 1.0, 0.1))
|
# plt.xticks(np.arange(0.1, 1.0, 0.1))
|
||||||
# plt.title('positive and negative pair distribution')
|
# plt.title('positive and negative pair distribution')
|
||||||
# return fig
|
# return fig
|
||||||
|
|
||||||
# def get_actmap(self, features, sz):
|
|
||||||
# """
|
|
||||||
# :param features: (1, 2048, 16, 8) activation map
|
|
||||||
# :return:
|
|
||||||
# """
|
|
||||||
# features = (features ** 2).sum(1) # (1, 16, 8)
|
|
||||||
# b, h, w = features.size()
|
|
||||||
# features = features.view(b, h * w)
|
|
||||||
# features = nn.functional.normalize(features, p=2, dim=1)
|
|
||||||
# acts = features.view(b, h, w)
|
|
||||||
# all_acts = []
|
|
||||||
# for i in range(b):
|
|
||||||
# act = acts[i].numpy()
|
|
||||||
# act = cv2.resize(act, (sz[1], sz[0]))
|
|
||||||
# act = 255 * (act - act.max()) / (act.max() - act.min() + 1e-12)
|
|
||||||
# act = np.uint8(np.floor(act))
|
|
||||||
# all_acts.append(act)
|
|
||||||
# return all_acts
|
|
||||||
|
Loading…
x
Reference in New Issue
Block a user