* resolve conflicts add heads and config for multilabel tasks * minor change * remove evaluating mAP in head * add baseline config * add configs * reserve only one config * minor change * fix minor bug * minor change * minor change * add unittests and fix docstrings |
||
---|---|---|
.github | ||
configs | ||
demo | ||
docs | ||
mmcls | ||
requirements | ||
resources | ||
tests | ||
tools | ||
.gitignore | ||
.pre-commit-config.yaml | ||
.readthedocs.yml | ||
LICENSE | ||
README.md | ||
requirements.txt | ||
setup.cfg | ||
setup.py |
README.md
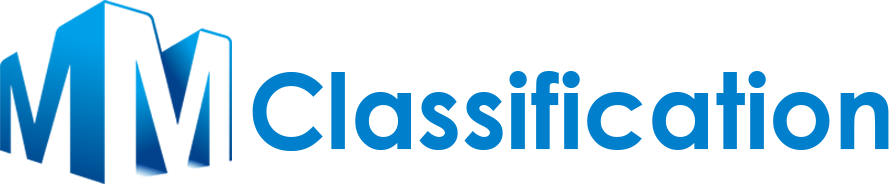
Introduction
MMClassification is an open source image classification toolbox based on PyTorch. It is a part of the OpenMMLab project.
Documentation: https://mmclassification.readthedocs.io/en/latest/
Major features
- Various backbones and pretrained models
- Bag of training tricks
- Large-scale training configs
- High efficiency and extensibility
License
This project is released under the Apache 2.0 license.
Changelog
v0.7.0 was released in 31/12/2020. Please refer to changelog.md for details and release history.
Benchmark and model zoo
Results and models are available in the model zoo.
Supported backbones:
- ResNet
- ResNeXt
- SE-ResNet
- SE-ResNeXt
- RegNet
- ShuffleNetV1
- ShuffleNetV2
- MobileNetV2
- MobileNetV3
Installation
Please refer to install.md for installation and dataset preparation.
Getting Started
Please see getting_started.md for the basic usage of MMClassification. There are also tutorials for finetuning models, adding new dataset, designing data pipeline, and adding new modules.
Contributing
We appreciate all contributions to improve MMClassification. Please refer to CONTRUBUTING.md for the contributing guideline.
Acknowledgement
MMClassification is an open source project that is contributed by researchers and engineers from various colleges and companies. We appreciate all the contributors who implement their methods or add new features, as well as users who give valuable feedbacks. We wish that the toolbox and benchmark could serve the growing research community by providing a flexible toolkit to reimplement existing methods and develop their own new classifiers.