182 lines
8.1 KiB
Markdown
182 lines
8.1 KiB
Markdown
# Runner
|
|
|
|
Deep learning algorithms usually share similar pipelines for training, validation and testing.
|
|
Therefore, MMengine designed `Runner` to simplify the construction of these pipelines.
|
|
In most cases, users can use our default `Runner` directly.
|
|
If you find it not feasible to implement your ideas, you can also modify it or customize your own runner.
|
|
|
|
Before introducing the design of `Runner`, let's walk through some examples to better understand why we should use runner.
|
|
Below is a few lines of pseudo codes for training models in PyTorch:
|
|
|
|
```python
|
|
model = ResNet()
|
|
optimizer = SGD(model.parameters(), lr=0.01, momentum=0.9)
|
|
train_dataset = ImageNetDataset(...)
|
|
train_dataloader = DataLoader(train_dataset, ...)
|
|
|
|
for i in range(max_epochs):
|
|
for data_batch in train_dataloader:
|
|
optimizer.zero_grad()
|
|
outputs = model(data_batch)
|
|
loss = loss_func(outputs, data_batch)
|
|
loss.backward()
|
|
optimizer.step()
|
|
```
|
|
|
|
Pseudo codes for model validation in PyTorch:
|
|
|
|
```python
|
|
model = ResNet()
|
|
model.load_state_dict(torch.load(CKPT_PATH))
|
|
model.eval()
|
|
|
|
test_dataset = ImageNetDataset(...)
|
|
test_dataloader = DataLoader(test_dataset, ...)
|
|
|
|
for data_batch in test_dataloader:
|
|
outputs = model(data_batch)
|
|
acc = calculate_acc(outputs, data_batch)
|
|
```
|
|
|
|
Pseudo codes for model inference in PyTorch:
|
|
|
|
```python
|
|
model = ResNet()
|
|
model.load_state_dict(torch.load(CKPT_PATH))
|
|
model.eval()
|
|
|
|
for img in imgs:
|
|
prediction = model(img)
|
|
```
|
|
|
|
The observation from the above 3 pieces of codes is that they are similar.
|
|
They can all be divided into some distinct steps, such as model construction, data loading and loop iterations.
|
|
Although the above examples are based on image classification tasks, the same holds for many other tasks as well, including object detection, image segmentation, etc.
|
|
Based on the observation above, we propose runner, which structures the training, validation and testing pipeline.
|
|
With runner, the only thing you need to do is to prepare necessary components (models, data, etc.) of your pipeline, and leave the schedule and execution to `Runner`.
|
|
You are free of constructing similar pipelines one and another time.
|
|
You are free of annoying details like the differences between distributed and non-distributed training.
|
|
You can focus on your own awesome ideas.
|
|
These are all achieved by runner and various practical modules in MMEngine.
|
|
|
|
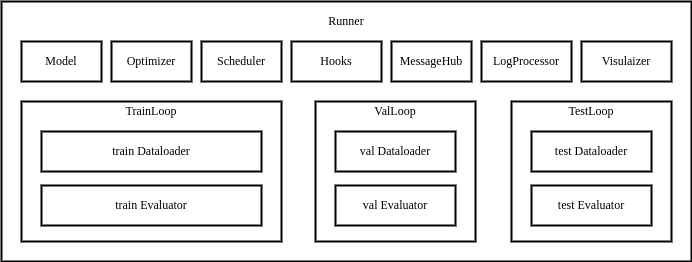
|
|
|
|
The `Runner` in MMEngine contains various modules required for training, testing and validation, as well as loop controllers(`Loop`) and [Hook](../tutorials/hook.md), as shown in the figure above.
|
|
It provides 3 APIs for users: `train`, `val` and `test`, each correspond to a specific `Loop`.
|
|
You can use `Runner` either by providing a config file, or by providing manually constructed modules.
|
|
Once activated, the `Runner` will automatically setup the runtime environment, build/compose your modules, execute the loop iterations in `Loop` and call registered hooks during iterations.
|
|
|
|
The execution order of `Runner` is as follows:
|
|
|
|
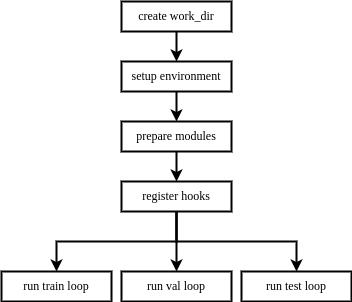
|
|
|
|
A feature of `Runner` is that it will always lazily initialize modules managed by itself.
|
|
To be specific, `Runner` won't build every module on initialization, and it won't build a module until it is needed in current `Loop`.
|
|
Therefore, if you are running only one of the `train`, `val`, or `test` pipelines, you only need to provide the relevant configs/modules.
|
|
|
|
## Loop
|
|
|
|
In MMEngine, we abstract the execution process of the task into `Loop`, based on the observation that most deep learning tasks can be summarized as a model iterating over datasets.
|
|
We provide 4 built-in loops in MMEngine:
|
|
|
|
- EpochBasedTrainLoop
|
|
- IterBasedTrainLoop
|
|
- ValLoop
|
|
- TestLoop
|
|
|
|
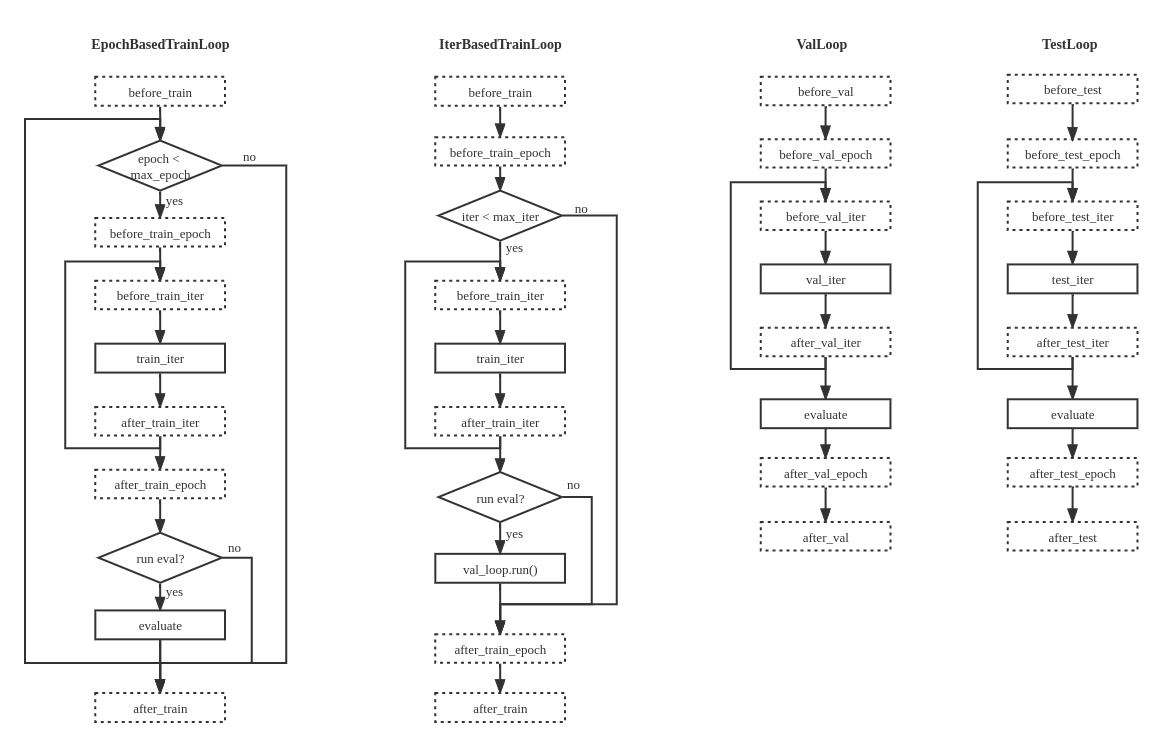
|
|
|
|
The built-in runner and loops are capable of most deep learning tasks, but surely not all.
|
|
Some tasks need extra modifications and refactorizations.
|
|
Therefore, we make it possible for users to customize their own pipelines for model training, validation and testing.
|
|
|
|
You can write your own pipeline by subclassing [BaseLoop](mmengine.runner.BaseLoop), which needs 2 arguments for initialization: 1) `runner` the Runner instance, and 2) `dataloader` the dataloader used in this loop.
|
|
You are free to add more arguments to your own loop subclass.
|
|
After defining your own loop subclass, you should register it to LOOPS(mmengine.registry.LOOPS), and specify it in config files by `type` field in `train_cfg`, `val_cfg` and `test_cfg`.
|
|
In fact, you can write any execution order, any hook position in your own loop.
|
|
However, built-in hooks may not work if you change hook positions, which may lead to inconsistent behavior during training.
|
|
Therefore, we strongly recommend you to implement you subclass with similar execution order illustrated in the figure above, and with the same hook positions defined in [hook documentation](../tutorials/hook.md).
|
|
|
|
```python
|
|
from mmengine.registry import LOOPS, HOOKS
|
|
from mmengine.runner import BaseLoop
|
|
from mmengine.hooks import Hook
|
|
|
|
|
|
# Customized validation loop
|
|
@LOOPS.register_module()
|
|
class CustomValLoop(BaseLoop):
|
|
def __init__(self, runner, dataloader, evaluator, dataloader2):
|
|
super().__init__(runner, dataloader, evaluator)
|
|
self.dataloader2 = runner.build_dataloader(dataloader2)
|
|
|
|
def run(self):
|
|
self.runner.call_hooks('before_val_epoch')
|
|
for idx, data_batch in enumerate(self.dataloader):
|
|
self.runner.call_hooks(
|
|
'before_val_iter', batch_idx=idx, data_batch=data_batch)
|
|
outputs = self.run_iter(idx, data_batch)
|
|
self.runner.call_hooks(
|
|
'after_val_iter', batch_idx=idx, data_batch=data_batch, outputs=outputs)
|
|
metric = self.evaluator.evaluate()
|
|
|
|
# add extra loop for validation purpose
|
|
for idx, data_batch in enumerate(self.dataloader2):
|
|
# add new hooks
|
|
self.runner.call_hooks(
|
|
'before_valloader2_iter', batch_idx=idx, data_batch=data_batch)
|
|
self.run_iter(idx, data_batch)
|
|
# add new hooks
|
|
self.runner.call_hooks(
|
|
'after_valloader2_iter', batch_idx=idx, data_batch=data_batch, outputs=outputs)
|
|
metric2 = self.evaluator.evaluate()
|
|
|
|
...
|
|
|
|
self.runner.call_hooks('after_val_epoch')
|
|
|
|
|
|
# Define a hook with extra hook positions
|
|
@HOOKS.register_module()
|
|
class CustomValHook(Hook):
|
|
def before_valloader2_iter(self, batch_idx, data_batch):
|
|
...
|
|
|
|
def after_valloader2_iter(self, batch_idx, data_batch, outputs):
|
|
...
|
|
|
|
```
|
|
|
|
The example above shows how to implement a different validation loop.
|
|
The new loop validates on two different validation datasets.
|
|
It also defines a new hook position in the second validation.
|
|
You can easily use it by setting `type='CustomValLoop'` in `val_cfg` in your config file.
|
|
|
|
```python
|
|
# Customized validation loop
|
|
val_cfg = dict(type='CustomValLoop', dataloader2=dict(dataset=dict(type='ValDataset2'), ...))
|
|
# Customized hook with extra hook position
|
|
custom_hooks = [dict(type='CustomValHook')]
|
|
```
|
|
|
|
## Customize Runner
|
|
|
|
Moreover, you can write your own runner by subclassing `Runner` if the built-in `Runner` is not feasible.
|
|
The method is similar to writing other modules: write your subclass inherited from `Runner`, overrides some functions, register it to `mmengine.registry.RUNNERS` and access it by assigning `runner_type` in your config file.
|
|
|
|
```python
|
|
from mmengine.registry import RUNNERS
|
|
from mmengine.runner import Runner
|
|
|
|
@RUNNERS.register_module()
|
|
class CustomRunner(Runner):
|
|
|
|
def setup_env(self):
|
|
...
|
|
```
|
|
|
|
The example above shows how to implement a customized runner which overrides the `setup_env` function and is registered to RUNNERS.
|
|
Now `CustomRunner` is prepared to be used by setting `runner_type='CustomRunner'` in your config file.
|
|
|
|
Further readings: [Runner tutorial](../tutorials/runner.md) and [Runner API documentations](mmengine.runner.Runner)
|