mirror of https://github.com/open-mmlab/mmocr.git
parent
73df26d749
commit
59d89e10c7
|
@ -1,3 +1,491 @@
|
|||
# Datasets\[coming soon\]
|
||||
# Dataset
|
||||
|
||||
Coming Soon!
|
||||
## Overview
|
||||
|
||||
In MMOCR, all the datasets are processed via different Dataset classes based on [mmengine.BaseDataset](mmengine.dataset.BaseDataset). Dataset classes are responsible for loading the data and performing initial parsing, then fed to [data pipeline](./transforms.md) for data preprocessing, augmentation, formatting, etc.
|
||||
|
||||
<div align="center">
|
||||
|
||||
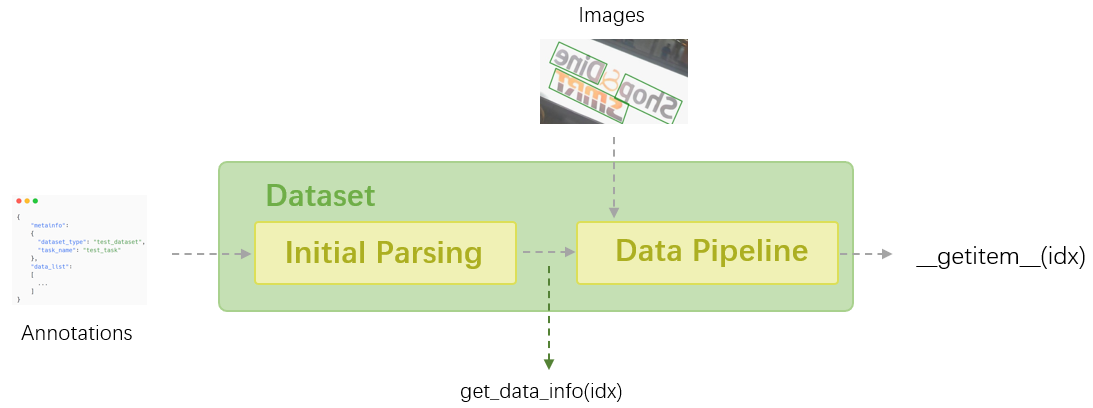
|
||||
|
||||
</div>
|
||||
|
||||
In this tutorial, we will introduce some common interfaces of the Dataset class, and the usage of Dataset implementations in MMOCR as well as the annotation types they support.
|
||||
|
||||
```{tip}
|
||||
Dataset class supports some advanced features, such as lazy initialization and data serialization, and takes advantage of various dataset wrappers to perform data concatenation, repeating, and category balancing. These content will not be covered in this tutorial, but you can read {external+mmengine:doc}`MMEngine: BaseDataset <advanced_tutorials/basedataset>` for more details.
|
||||
```
|
||||
|
||||
## Common Interfaces
|
||||
|
||||
Now, let's look at a concrete example and learn some typical interfaces of a Dataset class.
|
||||
`OCRDataset` is a widely used Dataset implementation in MMOCR, and is suggested as a default Dataset type in MMOCR as its associated annotation format is flexible enough to support *all* the OCR tasks ([more info](#ocrdataset)). Now we will instantiate an `OCRDataset` object wherein the toy dataset in `tests/data/det_toy_dataset` will be loaded.
|
||||
|
||||
```python
|
||||
from mmocr.datasets import OCRDataset
|
||||
from mmengine.registry import init_default_scope
|
||||
init_default_scope('mmocr')
|
||||
|
||||
train_pipeline = [
|
||||
dict(
|
||||
type='LoadImageFromFile'),
|
||||
dict(
|
||||
type='LoadOCRAnnotations',
|
||||
with_polygon=True,
|
||||
with_bbox=True,
|
||||
with_label=True,
|
||||
),
|
||||
dict(type='RandomCrop', min_side_ratio=0.1),
|
||||
dict(type='Resize', scale=(640, 640), keep_ratio=True),
|
||||
dict(type='Pad', size=(640, 640)),
|
||||
dict(
|
||||
type='PackTextDetInputs',
|
||||
meta_keys=('img_path', 'ori_shape', 'img_shape'))
|
||||
]
|
||||
dataset = OCRDataset(
|
||||
data_root='tests/data/det_toy_dataset',
|
||||
ann_file='textdet_test.json',
|
||||
test_mode=False,
|
||||
pipeline=train_pipeline)
|
||||
|
||||
```
|
||||
|
||||
Let's peek the size of this dataset:
|
||||
|
||||
```python
|
||||
>>> print(len(dataset))
|
||||
|
||||
10
|
||||
```
|
||||
|
||||
Typically, a Dataset class loads and stores two types of information: (1) **meta information**: Some meta descriptors of the dataset's property, such as available object categories in this dataset. (2) **annotation**: The path to images, and their labels. We can access the meta information in `dataset.metainfo`:
|
||||
|
||||
```python
|
||||
>>> from pprint import pprint
|
||||
>>> pprint(dataset.metainfo)
|
||||
|
||||
{'category': [{'id': 0, 'name': 'text'}],
|
||||
'dataset_type': 'TextDetDataset',
|
||||
'task_name': 'textdet'}
|
||||
```
|
||||
|
||||
As for the annotations, we can access them via `dataset.get_data_info(idx)`, which returns a dictionary containing the information of the `idx`-th sample in the dataset that is initially parsed, but not yet processed by [data pipeline](./transforms.md).
|
||||
|
||||
```python
|
||||
>>> from pprint import pprint
|
||||
>>> pprint(dataset.get_data_info(0))
|
||||
|
||||
{'height': 720,
|
||||
'img_path': 'tests/data/det_toy_dataset/test/img_10.jpg',
|
||||
'instances': [{'bbox': [260.0, 138.0, 284.0, 158.0],
|
||||
'bbox_label': 0,
|
||||
'ignore': True,
|
||||
'polygon': [261, 138, 284, 140, 279, 158, 260, 158]},
|
||||
...,
|
||||
{'bbox': [1011.0, 157.0, 1079.0, 173.0],
|
||||
'bbox_label': 0,
|
||||
'ignore': True,
|
||||
'polygon': [1011, 157, 1079, 160, 1076, 173, 1011, 170]}],
|
||||
'sample_idx': 0,
|
||||
'seg_map': 'test/gt_img_10.txt',
|
||||
'width': 1280}
|
||||
|
||||
```
|
||||
|
||||
On the other hand, we can get the sample fully processed by data pipeline via `dataset[idx]` or `dataset.__getitem__(idx)`, which is directly feedable to models and perform a full train/test cycle. It has two fields:
|
||||
|
||||
- `inputs`: The image after data augmentation;
|
||||
- `data_samples`: The [DataSample](./structures.md) that contains the augmented annotations, and meta information appended by some data transforms to keep track of some key properties of this sample.
|
||||
|
||||
```python
|
||||
>>> pprint(dataset[0])
|
||||
|
||||
{'data_samples': <TextDetDataSample(
|
||||
|
||||
META INFORMATION
|
||||
ori_shape: (720, 1280)
|
||||
img_path: 'tests/data/det_toy_dataset/imgs/test/img_10.jpg'
|
||||
img_shape: (640, 640)
|
||||
|
||||
DATA FIELDS
|
||||
gt_instances: <InstanceData(
|
||||
|
||||
META INFORMATION
|
||||
|
||||
DATA FIELDS
|
||||
labels: tensor([0, 0, 0])
|
||||
polygons: [array([207.33984 , 104.65409 , 208.34634 , 84.528305, 231.49594 ,
|
||||
86.54088 , 226.46341 , 104.65409 , 207.33984 , 104.65409 ],
|
||||
dtype=float32), array([237.53496 , 103.6478 , 235.52196 , 84.528305, 365.36096 ,
|
||||
86.54088 , 364.35446 , 107.67296 , 237.53496 , 103.6478 ],
|
||||
dtype=float32), array([105.68293, 166.03773, 105.68293, 151.94969, 177.14471, 150.94339,
|
||||
178.15121, 165.03145, 105.68293, 166.03773], dtype=float32)]
|
||||
ignored: tensor([ True, False, True])
|
||||
bboxes: tensor([[207.3398, 84.5283, 231.4959, 104.6541],
|
||||
[235.5220, 84.5283, 365.3610, 107.6730],
|
||||
[105.6829, 150.9434, 178.1512, 166.0377]])
|
||||
) at 0x7f7359f04fa0>
|
||||
) at 0x7f735a0508e0>,
|
||||
'inputs': tensor([[[129, 111, 131, ..., 0, 0, 0], ...
|
||||
[ 19, 18, 15, ..., 0, 0, 0]]], dtype=torch.uint8)}
|
||||
```
|
||||
|
||||
## Dataset Classes and Annotation Formats
|
||||
|
||||
Each Dataset implementation can only load datasets in a specific annotation format. Here lists all supported Dataset classes and their compatible annotation formats, as well as an example config that showcases how to use them in practice.
|
||||
|
||||
```{note}
|
||||
If you are not familiar with the config system, you may find [Dataset Configuration](../user_guides/dataset_prepare.md#dataset-configuration) helpful.
|
||||
```
|
||||
|
||||
### OCRDataset
|
||||
|
||||
Usually, there are many different types of annotations in OCR datasets, and the formats often vary between different subtasks, such as text detection and text recognition. These differences can result in the need for different data loading code when using different datasets, increasing the learning and maintenance costs for users.
|
||||
|
||||
In MMOCR, we propose a unified dataset format that can adapt to all three subtasks of OCR: text detection, text recognition, and text spotting. This design maximizes the uniformity of the dataset, allows for the reuse of data annotations across different tasks, and makes dataset management more convenient. Considering that popular dataset formats are still inconsistent, MMOCR provides [Dataset Preparer](../user_guides/data_prepare/dataset_preparer.md) to help users convert their datasets to MMOCR format. We also strongly encourage researchers to develop their own datasets based on this data format.
|
||||
|
||||
#### Annotation Format
|
||||
|
||||
This annotation file is a `.json` file that stores a `dict`, containing both `metainfo` and `data_list`, where the former includes basic information about the dataset and the latter consists of the label item of each target instance. Here presents an extensive list of all the fields in the annotation file, but some fields are used in a subset of tasks and can be ignored in other tasks.
|
||||
|
||||
```python
|
||||
{
|
||||
"metainfo":
|
||||
{
|
||||
"dataset_type": "TextDetDataset", # Options: TextDetDataset/TextRecogDataset/TextSpotterDataset
|
||||
"task_name": "textdet", # Options: textdet/textspotter/textrecog
|
||||
"category": [{"id": 0, "name": "text"}] # Used in textdet/textspotter
|
||||
},
|
||||
"data_list":
|
||||
[
|
||||
{
|
||||
"img_path": "test_img.jpg",
|
||||
"height": 604,
|
||||
"width": 640,
|
||||
"instances": # multiple instances in one image
|
||||
[
|
||||
{
|
||||
"bbox": [0, 0, 10, 20], # in textdet/textspotter, [x1, y1, x2, y2].
|
||||
"bbox_label": 0, # The object category, always 0 (text) in MMOCR
|
||||
"polygon": [0, 0, 0, 10, 10, 20, 20, 0], # in textdet/textspotter. [x1, y1, x2, y2, ....]
|
||||
"text": "mmocr", # in textspotter/textrecog
|
||||
"ignore": False # in textspotter/textdet. Whether to ignore this sample during training
|
||||
},
|
||||
#...
|
||||
],
|
||||
}
|
||||
#... multiple images
|
||||
]
|
||||
}
|
||||
```
|
||||
|
||||
#### Example Config
|
||||
|
||||
Here is a part of config example where we make `train_dataloader` use `OCRDataset` to load the ICDAR2015 dataset for a text detection model. Keep in mind that `OCRDataset` can load any OCR datasets prepared by Dataset Preparer regardless of its task. That is, you can use it for text recognition and text spotting, but you still have to modify the transform types in `pipeline` according to the needs of different tasks.
|
||||
|
||||
```python
|
||||
pipeline = [
|
||||
dict(
|
||||
type='LoadImageFromFile'),
|
||||
dict(
|
||||
type='LoadOCRAnnotations',
|
||||
with_polygon=True,
|
||||
with_bbox=True,
|
||||
with_label=True,
|
||||
),
|
||||
dict(
|
||||
type='PackTextDetInputs',
|
||||
meta_keys=('img_path', 'ori_shape', 'img_shape'))
|
||||
]
|
||||
|
||||
icdar2015_textdet_train = dict(
|
||||
type='OCRDataset',
|
||||
data_root='data/icdar2015',
|
||||
ann_file='textdet_train.json',
|
||||
filter_cfg=dict(filter_empty_gt=True, min_size=32),
|
||||
pipeline=pipeline)
|
||||
|
||||
train_dataloader = dict(
|
||||
batch_size=16,
|
||||
num_workers=8,
|
||||
persistent_workers=True,
|
||||
sampler=dict(type='DefaultSampler', shuffle=True),
|
||||
dataset=icdar2015_textdet_train)
|
||||
```
|
||||
|
||||
### RecogLMDBDataset
|
||||
|
||||
Reading images or labels from files can be slow when data are excessive, e.g. on a scale of millions. Besides, in academia, most of the scene text recognition datasets are stored in lmdb format, including images and labels. ([Example](https://github.com/clovaai/deep-text-recognition-benchmark))
|
||||
|
||||
To get closer to the mainstream practice and enhance the data storage efficiency, MMOCR supports loading images and labels from lmdb datasets via `RecogLMDBDataset`.
|
||||
|
||||
#### Annotation Format
|
||||
|
||||
MMOCR requires the following keys for LMDB datasets:
|
||||
|
||||
- `num_samples`: The parameter describing the data volume of the dataset.
|
||||
- The keys of images and labels are in the
|
||||
format of `image-000000001` and `label-000000001`, respectively. The index starts from 1.
|
||||
|
||||
MMOCR has a toy LMDB dataset in `tests/data/rec_toy_dataset/imgs.lmdb`.
|
||||
You can get a sense of the format with the following code snippet.
|
||||
|
||||
```python
|
||||
>>> import lmdb
|
||||
>>>
|
||||
>>> env = lmdb.open('tests/data/rec_toy_dataset/imgs.lmdb')
|
||||
>>> txn = env.begin()
|
||||
>>> for k, v in txn.cursor():
|
||||
>>> print(k, v)
|
||||
|
||||
b'image-000000001' b'\xff...'
|
||||
b'image-000000002' b'\xff...'
|
||||
b'image-000000003' b'\xff...'
|
||||
b'image-000000004' b'\xff...'
|
||||
b'image-000000005' b'\xff...'
|
||||
b'image-000000006' b'\xff...'
|
||||
b'image-000000007' b'\xff...'
|
||||
b'image-000000008' b'\xff...'
|
||||
b'image-000000009' b'\xff...'
|
||||
b'image-000000010' b'\xff...'
|
||||
b'label-000000001' b'GRAND'
|
||||
b'label-000000002' b'HOTEL'
|
||||
b'label-000000003' b'HOTEL'
|
||||
b'label-000000004' b'PACIFIC'
|
||||
b'label-000000005' b'03/09/2009'
|
||||
b'label-000000006' b'ANING'
|
||||
b'label-000000007' b'Virgin'
|
||||
b'label-000000008' b'america'
|
||||
b'label-000000009' b'ATTACK'
|
||||
b'label-000000010' b'DAVIDSON'
|
||||
b'num-samples' b'10'
|
||||
```
|
||||
|
||||
#### Example Config
|
||||
|
||||
Here is a part of config example where we make `train_dataloader` use `RecogLMDBDataset` to load the toy dataset. Since `RecogLMDBDataset` loads images as numpy arrays, don't forget to use `LoadImageFromNDArray` instead of `LoadImageFromFile` in the pipeline for successful loading.
|
||||
|
||||
```python
|
||||
pipeline = [
|
||||
dict(
|
||||
type='LoadImageFromNDArray'),
|
||||
dict(
|
||||
type='LoadOCRAnnotations',
|
||||
with_text=True,
|
||||
),
|
||||
dict(
|
||||
type='PackTextRecogInputs',
|
||||
meta_keys=('img_path', 'ori_shape', 'img_shape'))
|
||||
]
|
||||
|
||||
toy_textrecog_train = dict(
|
||||
type='RecogLMDBDataset',
|
||||
data_root='tests/data/rec_toy_dataset/',
|
||||
ann_file='imgs.lmdb',
|
||||
pipeline=pipeline)
|
||||
|
||||
train_dataloader = dict(
|
||||
batch_size=16,
|
||||
num_workers=8,
|
||||
persistent_workers=True,
|
||||
sampler=dict(type='DefaultSampler', shuffle=True),
|
||||
dataset=toy_textrecog_train)
|
||||
```
|
||||
|
||||
### RecogTextDataset
|
||||
|
||||
Prior to MMOCR 1.0, MMOCR 0.x takes text files as input for text recognition. These formats has been deprecated in MMOCR 1.0, and this class could be removed anytime in the future. [More info](../migration/dataset.md)
|
||||
|
||||
#### Annotation Format
|
||||
|
||||
Text files can either be in `txt` format or `jsonl` format. The simple `.txt` annotations separate image name and word annotation by a blank space, which cannot handle the case when spaces are included in a text instance.
|
||||
|
||||
```text
|
||||
img1.jpg OpenMMLab
|
||||
img2.jpg MMOCR
|
||||
```
|
||||
|
||||
The JSON Line format uses a dictionary-like structure to represent the annotations, where the keys `filename` and `text` store the image name and word label, respectively.
|
||||
|
||||
```json
|
||||
{"filename": "img1.jpg", "text": "OpenMMLab"}
|
||||
{"filename": "img2.jpg", "text": "MMOCR"}
|
||||
```
|
||||
|
||||
#### Example Config
|
||||
|
||||
Here is a part of config example where we use `RecogTextDataset` to load the old txt labels in training, and the old jsonl labels in testing.
|
||||
|
||||
```python
|
||||
pipeline = [
|
||||
dict(
|
||||
type='LoadImageFromFile'),
|
||||
dict(
|
||||
type='LoadOCRAnnotations',
|
||||
with_polygon=True,
|
||||
with_bbox=True,
|
||||
with_label=True,
|
||||
),
|
||||
dict(
|
||||
type='PackTextDetInputs',
|
||||
meta_keys=('img_path', 'ori_shape', 'img_shape'))
|
||||
]
|
||||
|
||||
# loading 0.x txt format annos
|
||||
txt_dataset = dict(
|
||||
type='RecogTextDataset',
|
||||
data_root=data_root,
|
||||
ann_file='old_label.txt',
|
||||
data_prefix=dict(img_path='imgs'),
|
||||
parser_cfg=dict(
|
||||
type='LineStrParser',
|
||||
keys=['filename', 'text'],
|
||||
keys_idx=[0, 1]),
|
||||
pipeline=pipeline)
|
||||
|
||||
|
||||
train_dataloader = dict(
|
||||
batch_size=16,
|
||||
num_workers=8,
|
||||
persistent_workers=True,
|
||||
sampler=dict(type='DefaultSampler', shuffle=True),
|
||||
dataset=txt_dataset)
|
||||
|
||||
# loading 0.x json line format annos
|
||||
jsonl_dataset = dict(
|
||||
type='RecogTextDataset',
|
||||
data_root=data_root,
|
||||
ann_file='old_label.jsonl',
|
||||
data_prefix=dict(img_path='imgs'),
|
||||
parser_cfg=dict(
|
||||
type='LineJsonParser',
|
||||
keys=['filename', 'text'],
|
||||
pipeline=pipeline))
|
||||
|
||||
test_dataloader = dict(
|
||||
batch_size=16,
|
||||
num_workers=8,
|
||||
persistent_workers=True,
|
||||
sampler=dict(type='DefaultSampler', shuffle=False),
|
||||
dataset=jsonl_dataset)
|
||||
```
|
||||
|
||||
### IcdarDataset
|
||||
|
||||
Prior to MMOCR 1.0, MMOCR 0.x takes COCO-like format annotations as input for text detection. These formats has been deprecated in MMOCR 1.0, and this class could be removed anytime in the future. [More info](../migration/dataset.md)
|
||||
|
||||
#### Annotation Format
|
||||
|
||||
```json
|
||||
{
|
||||
"images": [
|
||||
{
|
||||
"id": 1,
|
||||
"width": 800,
|
||||
"height": 600,
|
||||
"file_name": "test.jpg"
|
||||
}
|
||||
],
|
||||
"annotations": [
|
||||
{
|
||||
"id": 1,
|
||||
"image_id": 1,
|
||||
"category_id": 1,
|
||||
"bbox": [0,0,10,10],
|
||||
"segmentation": [
|
||||
[0,0,10,0,10,10,0,10]
|
||||
],
|
||||
"area": 100,
|
||||
"iscrowd": 0
|
||||
}
|
||||
]
|
||||
}
|
||||
```
|
||||
|
||||
#### Example Config
|
||||
|
||||
Here is a part of config example where we make `train_dataloader` use `IcdarDataset` to load the old labels.
|
||||
|
||||
```python
|
||||
pipeline = [
|
||||
dict(
|
||||
type='LoadImageFromFile'),
|
||||
dict(
|
||||
type='LoadOCRAnnotations',
|
||||
with_polygon=True,
|
||||
with_bbox=True,
|
||||
with_label=True,
|
||||
),
|
||||
dict(
|
||||
type='PackTextDetInputs',
|
||||
meta_keys=('img_path', 'ori_shape', 'img_shape'))
|
||||
]
|
||||
|
||||
icdar2015_textdet_train = dict(
|
||||
type='IcdarDatasetDataset',
|
||||
data_root='data/det/icdar2015',
|
||||
ann_file='instances_training.json',
|
||||
filter_cfg=dict(filter_empty_gt=True, min_size=32),
|
||||
pipeline=pipeline)
|
||||
|
||||
train_dataloader = dict(
|
||||
batch_size=16,
|
||||
num_workers=8,
|
||||
persistent_workers=True,
|
||||
sampler=dict(type='DefaultSampler', shuffle=True),
|
||||
dataset=icdar2015_textdet_train)
|
||||
```
|
||||
|
||||
### WildReceiptDataset
|
||||
|
||||
It's customized for [WildReceipt](https://mmocr.readthedocs.io/en/dev-1.x/user_guides/data_prepare/datasetzoo.html#wildreceipt) dataset only.
|
||||
|
||||
#### Annotation Format
|
||||
|
||||
```json
|
||||
// Close Set
|
||||
{
|
||||
"file_name": "image_files/Image_16/11/d5de7f2a20751e50b84c747c17a24cd98bed3554.jpeg",
|
||||
"height": 1200,
|
||||
"width": 1600,
|
||||
"annotations":
|
||||
[
|
||||
{
|
||||
"box": [550.0, 190.0, 937.0, 190.0, 937.0, 104.0, 550.0, 104.0],
|
||||
"text": "SAFEWAY",
|
||||
"label": 1
|
||||
},
|
||||
{
|
||||
"box": [1048.0, 211.0, 1074.0, 211.0, 1074.0, 196.0, 1048.0, 196.0],
|
||||
"text": "TM",
|
||||
"label": 25
|
||||
}
|
||||
], //...
|
||||
}
|
||||
|
||||
// Open Set
|
||||
{
|
||||
"file_name": "image_files/Image_12/10/845be0dd6f5b04866a2042abd28d558032ef2576.jpeg",
|
||||
"height": 348,
|
||||
"width": 348,
|
||||
"annotations":
|
||||
[
|
||||
{
|
||||
"box": [114.0, 19.0, 230.0, 19.0, 230.0, 1.0, 114.0, 1.0],
|
||||
"text": "CHOEUN",
|
||||
"label": 2,
|
||||
"edge": 1
|
||||
},
|
||||
{
|
||||
"box": [97.0, 35.0, 236.0, 35.0, 236.0, 19.0, 97.0, 19.0],
|
||||
"text": "KOREANRESTAURANT",
|
||||
"label": 2,
|
||||
"edge": 1
|
||||
}
|
||||
]
|
||||
}
|
||||
```
|
||||
|
||||
#### Example Config
|
||||
|
||||
Please refer to [SDMGR's config](https://github.com/open-mmlab/mmocr/blob/f30c16ce96bd2393570c04eeb9cf48a7916315cc/configs/kie/sdmgr/sdmgr_novisual_60e_wildreceipt.py) for more details.
|
||||
|
|
|
@ -30,9 +30,9 @@ You can switch between English and Chinese in the lower-left corner of the layou
|
|||
basic_concepts/structures.md
|
||||
basic_concepts/transforms.md
|
||||
basic_concepts/evaluation.md
|
||||
basic_concepts/datasets.md
|
||||
basic_concepts/overview.md
|
||||
basic_concepts/data_flow.md
|
||||
basic_concepts/datasets.md
|
||||
basic_concepts/models.md
|
||||
basic_concepts/visualizers.md
|
||||
basic_concepts/convention.md
|
||||
|
|
|
@ -4,64 +4,14 @@
|
|||
|
||||
After decades of development, the OCR community has produced a series of related datasets that often provide annotations of text in a variety of styles, making it necessary for users to convert these datasets to the required format when using them. MMOCR supports dozens of commonly used text-related datasets and provides a [data preparation script](./data_prepare/dataset_preparer.md) to help users prepare the datasets with only one command.
|
||||
|
||||
In the following, we provide a brief overview of the data formats defined in MMOCR for each task.
|
||||
In this section, we will introduce a typical process of preparing a dataset for MMOCR:
|
||||
|
||||
- As shown in the following code block, the text detection task uses the data format `TextDetDataset`, which holds the bounding box annotations, file names, and other information required for the text detection task. We provide a sample annotation file in the `tests/data/det_toy_dataset/instances_test.json` path.
|
||||
1. [Download datasets and convert its format to the suggested one](#downloading-datasets-and-converting-format)
|
||||
2. [Modify the config file](#dataset-configuration)
|
||||
|
||||
```json
|
||||
{
|
||||
"metainfo":
|
||||
{
|
||||
"dataset_type": "TextDetDataset",
|
||||
"task_name": "textdet",
|
||||
"category": [{"id": 0, "name": "text"}]
|
||||
},
|
||||
"data_list":
|
||||
[
|
||||
{
|
||||
"img_path": "test_img.jpg",
|
||||
"height": 640,
|
||||
"width": 640,
|
||||
"instances":
|
||||
[
|
||||
{
|
||||
"polygon": [0, 0, 0, 10, 10, 20, 20, 0],
|
||||
"bbox": [0, 0, 10, 20],
|
||||
"bbox_label": 0,
|
||||
"ignore": false,
|
||||
},
|
||||
],
|
||||
//...
|
||||
}
|
||||
]
|
||||
}
|
||||
```
|
||||
However, the first step is not necessary if you already have a dataset in the format that MMOCR supports. You can read [Dataset Classes](../basic_concepts/datasets.md#dataset-classes-and-annotation-formats) for more details.
|
||||
|
||||
- As shown in the following code block, the text recognition task uses the data format `TextRecogDataset`, which holds information such as text instances and image paths required by the text recognition task. An example annotation file is provided in the `tests/data/rec_toy_dataset/labels.json` path.
|
||||
|
||||
```json
|
||||
{
|
||||
"metainfo":
|
||||
{
|
||||
"dataset_type": "TextRecogDataset",
|
||||
"task_name": "textrecog",
|
||||
},
|
||||
"data_list":
|
||||
[
|
||||
{
|
||||
"img_path": "test_img.jpg",
|
||||
"instances":
|
||||
[
|
||||
{
|
||||
"text": "GRAND"
|
||||
}
|
||||
]
|
||||
}
|
||||
]
|
||||
}
|
||||
```
|
||||
|
||||
## Downloading Datasets and Format Conversion
|
||||
## Downloading Datasets and Converting Format
|
||||
|
||||
As an example of the data preparation steps, you can use the following command to prepare the ICDAR 2015 dataset for text detection task.
|
||||
|
||||
|
|
|
@ -1,3 +1,489 @@
|
|||
# 数据集\[待更新\]
|
||||
# 数据集类
|
||||
|
||||
待更新
|
||||
## 概览
|
||||
|
||||
在 MMOCR 中,所有的数据集都通过不同的基于 [mmengine.BaseDataset](mmengine.dataset.BaseDataset) 的 Dataset 类进行处理。 Dataset 类负责加载数据并进行初始解析,然后将其馈送到 [数据流水线](./transforms.md) 进行数据预处理、增强、格式化等操作。
|
||||
|
||||
<div align="center">
|
||||
|
||||
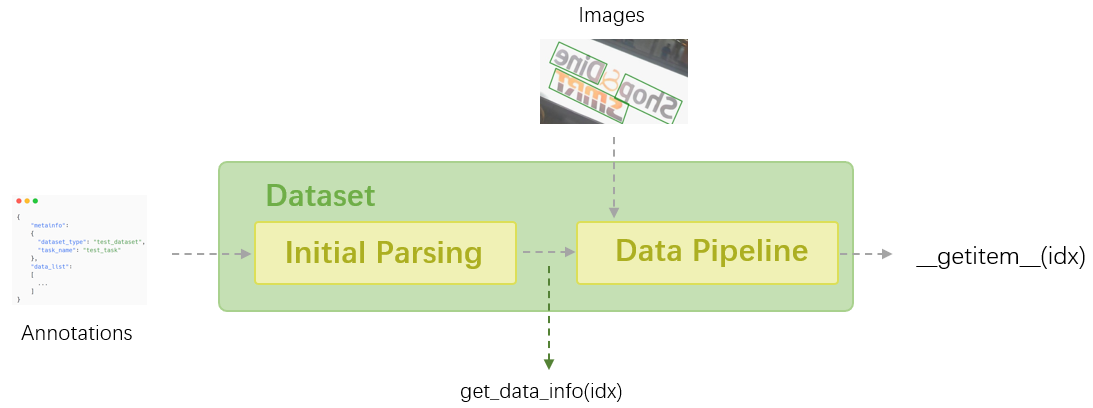
|
||||
|
||||
</div>
|
||||
|
||||
在本教程中,我们将介绍 Dataset 类的一些常见接口,以及 MMOCR 中 Dataset 实现的使用以及它们支持的注释类型。
|
||||
|
||||
```{tip}
|
||||
Dataset 类支持一些高级功能,例如懒加载、数据序列化、利用各种数据集包装器执行数据连接、重复和类别平衡。这些内容将不在本教程中介绍,但您可以阅读 {external+mmengine:doc}`MMEngine: BaseDataset <advanced_tutorials/basedataset>` 了解更多详细信息。
|
||||
```
|
||||
|
||||
## 常见接口
|
||||
|
||||
现在,让我们看一个具体的示例并学习 Dataset 类的一些典型接口。`OCRDataset` 是 MMOCR 中默认使用的 Dataset 实现,因为它的标注格式足够灵活,支持 *所有* OCR 任务(详见 [OCRDataset](#ocrdataset))。现在我们将实例化一个 `OCRDataset` 对象,其中将加载 `tests/data/det_toy_dataset` 中的玩具数据集。
|
||||
|
||||
```python
|
||||
from mmocr.datasets import OCRDataset
|
||||
from mmengine.registry import init_default_scope
|
||||
init_default_scope('mmocr')
|
||||
|
||||
train_pipeline = [
|
||||
dict(
|
||||
type='LoadImageFromFile'),
|
||||
dict(
|
||||
type='LoadOCRAnnotations',
|
||||
with_polygon=True,
|
||||
with_bbox=True,
|
||||
with_label=True,
|
||||
),
|
||||
dict(type='RandomCrop', min_side_ratio=0.1),
|
||||
dict(type='Resize', scale=(640, 640), keep_ratio=True),
|
||||
dict(type='Pad', size=(640, 640)),
|
||||
dict(
|
||||
type='PackTextDetInputs',
|
||||
meta_keys=('img_path', 'ori_shape', 'img_shape'))
|
||||
]
|
||||
dataset = OCRDataset(
|
||||
data_root='tests/data/det_toy_dataset',
|
||||
ann_file='textdet_test.json',
|
||||
test_mode=False,
|
||||
pipeline=train_pipeline)
|
||||
|
||||
```
|
||||
|
||||
让我们查看一下这个数据集的大小:
|
||||
|
||||
```python
|
||||
>>> print(len(dataset))
|
||||
|
||||
10
|
||||
```
|
||||
|
||||
通常,Dataset 类加载并存储两种类型的信息:(1)**元信息**:储存数据集的属性,例如此数据集中可用的对象类别。 (2)**标注**:图像的路径及其标签。我们可以通过 `dataset.metainfo` 访问元信息:
|
||||
|
||||
```python
|
||||
>>> from pprint import pprint
|
||||
>>> pprint(dataset.metainfo)
|
||||
|
||||
{'category': [{'id': 0, 'name': 'text'}],
|
||||
'dataset_type': 'TextDetDataset',
|
||||
'task_name': 'textdet'}
|
||||
```
|
||||
|
||||
对于标注,我们可以通过 `dataset.get_data_info(idx)` 访问它。该方法返回一个字典,其中包含数据集中第 `idx` 个样本的信息。该样本已经经过初步解析,但尚未由 [数据流水线](./transforms.md) 处理。
|
||||
|
||||
```python
|
||||
>>> from pprint import pprint
|
||||
>>> pprint(dataset.get_data_info(0))
|
||||
|
||||
{'height': 720,
|
||||
'img_path': 'tests/data/det_toy_dataset/test/img_10.jpg',
|
||||
'instances': [{'bbox': [260.0, 138.0, 284.0, 158.0],
|
||||
'bbox_label': 0,
|
||||
'ignore': True,
|
||||
'polygon': [261, 138, 284, 140, 279, 158, 260, 158]},
|
||||
...,
|
||||
{'bbox': [1011.0, 157.0, 1079.0, 173.0],
|
||||
'bbox_label': 0,
|
||||
'ignore': True,
|
||||
'polygon': [1011, 157, 1079, 160, 1076, 173, 1011, 170]}],
|
||||
'sample_idx': 0,
|
||||
'seg_map': 'test/gt_img_10.txt',
|
||||
'width': 1280}
|
||||
```
|
||||
|
||||
另一方面,我们可以通过 `dataset[idx]` 或 `dataset.__getitem__(idx)` 获取由数据流水线完整处理过后的样本,该样本可以直接馈入模型并执行完整的训练/测试循环。它有两个字段:
|
||||
|
||||
- `inputs`:经过数据增强后的图像;
|
||||
- `data_samples`:包含经过数据增强后的标注和元信息的 [DataSample](./structures.md),这些元信息可能由一些数据变换产生,并用以记录该样本的某些关键属性。
|
||||
|
||||
```python
|
||||
>>> pprint(dataset[0])
|
||||
|
||||
{'data_samples': <TextDetDataSample(
|
||||
|
||||
META INFORMATION
|
||||
ori_shape: (720, 1280)
|
||||
img_path: 'tests/data/det_toy_dataset/imgs/test/img_10.jpg'
|
||||
img_shape: (640, 640)
|
||||
|
||||
DATA FIELDS
|
||||
gt_instances: <InstanceData(
|
||||
|
||||
META INFORMATION
|
||||
|
||||
DATA FIELDS
|
||||
labels: tensor([0, 0, 0])
|
||||
polygons: [array([207.33984 , 104.65409 , 208.34634 , 84.528305, 231.49594 ,
|
||||
86.54088 , 226.46341 , 104.65409 , 207.33984 , 104.65409 ],
|
||||
dtype=float32), array([237.53496 , 103.6478 , 235.52196 , 84.528305, 365.36096 ,
|
||||
86.54088 , 364.35446 , 107.67296 , 237.53496 , 103.6478 ],
|
||||
dtype=float32), array([105.68293, 166.03773, 105.68293, 151.94969, 177.14471, 150.94339,
|
||||
178.15121, 165.03145, 105.68293, 166.03773], dtype=float32)]
|
||||
ignored: tensor([ True, False, True])
|
||||
bboxes: tensor([[207.3398, 84.5283, 231.4959, 104.6541],
|
||||
[235.5220, 84.5283, 365.3610, 107.6730],
|
||||
[105.6829, 150.9434, 178.1512, 166.0377]])
|
||||
) at 0x7f7359f04fa0>
|
||||
) at 0x7f735a0508e0>,
|
||||
'inputs': tensor([[[129, 111, 131, ..., 0, 0, 0], ...
|
||||
[ 19, 18, 15, ..., 0, 0, 0]]], dtype=torch.uint8)}
|
||||
```
|
||||
|
||||
## 数据集类及标注格式
|
||||
|
||||
每个数据集实现只能加载特定格式的数据集。这里列出了所有支持的数据集类及其兼容的格式,以及一个示例配置,以演示如何在实践中使用它们。
|
||||
|
||||
```{note}
|
||||
如果您不熟悉配置系统,可以阅读 [数据集配置文件](../user_guides/dataset_prepare.md#数据集配置文件)。
|
||||
```
|
||||
|
||||
### OCRDataset
|
||||
|
||||
通常,OCR 数据集中有许多不同类型的标注,在不同的子任务(如文本检测和文本识别)中,格式也经常会有所不同。这些差异可能会导致在使用不同数据集时需要不同的数据加载代码,增加了用户的学习和维护成本。
|
||||
|
||||
在 MMOCR 中,我们提出了一种统一的数据集格式,可以适应 OCR 的所有三个子任务:文本检测、文本识别和端到端 OCR。这种设计最大程度地提高了数据集的一致性,允许在不同任务之间重复使用数据标注,也使得数据集管理更加方便。考虑到流行的数据集格式并不一致,MMOCR 提供了 [Dataset Preparer](../user_guides/data_prepare/dataset_preparer.md) 来帮助用户将其数据集转换为 MMOCR 格式。我们也十分鼓励研究人员基于此数据格式开发自己的数据集。
|
||||
|
||||
#### 标注格式
|
||||
|
||||
此标注文件是一个 `.json` 文件,存储一个包含 `metainfo` 和 `data_list` 的 `dict`,前者包括有关数据集的基本信息,后者由每个图片的标注组成。这里呈现了标注文件中的所有字段的列表,但其中某些字段仅会在特定任务中被用到。
|
||||
|
||||
```python
|
||||
{
|
||||
"metainfo":
|
||||
{
|
||||
"dataset_type": "TextDetDataset", # 可选项: TextDetDataset/TextRecogDataset/TextSpotterDataset
|
||||
"task_name": "textdet", # 可选项: textdet/textspotter/textrecog
|
||||
"category": [{"id": 0, "name": "text"}] # 在 textdet/textspotter 里用到
|
||||
},
|
||||
"data_list":
|
||||
[
|
||||
{
|
||||
"img_path": "test_img.jpg",
|
||||
"height": 604,
|
||||
"width": 640,
|
||||
"instances": # 一图内的多个实例
|
||||
[
|
||||
{
|
||||
"bbox": [0, 0, 10, 20], # textdet/textspotter 内用到, [x1, y1, x2, y2]。
|
||||
"bbox_label": 0, # 对象类别, 在 MMOCR 中恒为 0 (文本)
|
||||
"polygon": [0, 0, 0, 10, 10, 20, 20, 0], # textdet/textspotter 内用到。 [x1, y1, x2, y2, ....]
|
||||
"text": "mmocr", # textspotter/textrecog 内用到
|
||||
"ignore": False # textspotter/textdet 内用到,决定是否在训练时忽略该实例
|
||||
},
|
||||
#...
|
||||
],
|
||||
}
|
||||
#... 多图片
|
||||
]
|
||||
}
|
||||
```
|
||||
|
||||
#### 示例配置
|
||||
|
||||
以下是配置的一部分,我们在 `train_dataloader` 中使用 `OCRDataset` 加载用于文本检测模型的 ICDAR2015 数据集。请注意,`OCRDataset` 可以加载由 Dataset Preparer 准备的任何 OCR 数据集。也就是说,您可以将其用于文本识别和文本检测,但您仍然需要根据不同任务的需求修改 `pipeline` 中的数据变换。
|
||||
|
||||
```python
|
||||
pipeline = [
|
||||
dict(
|
||||
type='LoadImageFromFile'),
|
||||
dict(
|
||||
type='LoadOCRAnnotations',
|
||||
with_polygon=True,
|
||||
with_bbox=True,
|
||||
with_label=True,
|
||||
),
|
||||
dict(
|
||||
type='PackTextDetInputs',
|
||||
meta_keys=('img_path', 'ori_shape', 'img_shape'))
|
||||
]
|
||||
|
||||
icdar2015_textdet_train = dict(
|
||||
type='OCRDataset',
|
||||
data_root='data/icdar2015',
|
||||
ann_file='textdet_train.json',
|
||||
filter_cfg=dict(filter_empty_gt=True, min_size=32),
|
||||
pipeline=pipeline)
|
||||
|
||||
train_dataloader = dict(
|
||||
batch_size=16,
|
||||
num_workers=8,
|
||||
persistent_workers=True,
|
||||
sampler=dict(type='DefaultSampler', shuffle=True),
|
||||
dataset=icdar2015_textdet_train)
|
||||
```
|
||||
|
||||
### RecogLMDBDataset
|
||||
|
||||
当数据量非常大时,从文件中读取图像或标签可能会很慢。此外,在学术界,大多数场景文本识别数据集的图像和标签都以 lmdb 格式存储。([示例](https://github.com/clovaai/deep-text-recognition-benchmark))
|
||||
|
||||
为了更接近主流实践并提高数据存储效率,MMOCR支持通过 `RecogLMDBDataset` 从 lmdb 数据集加载图像和标签。
|
||||
|
||||
#### 标注格式
|
||||
|
||||
MMOCR 会读取 lmdb 数据集中的以下键:
|
||||
|
||||
- `num_samples`:描述数据集的数据量的参数。
|
||||
- 图像和标签的键分别以 `image-000000001` 和 `label-000000001` 的格式命名,索引从1开始。
|
||||
|
||||
MMOCR 在 `tests/data/rec_toy_dataset/imgs.lmdb` 中提供了一个 toy lmdb 数据集。您可以使用以下代码片段了解其格式。
|
||||
|
||||
```python
|
||||
>>> import lmdb
|
||||
>>>
|
||||
>>> env = lmdb.open('tests/data/rec_toy_dataset/imgs.lmdb')
|
||||
>>> txn = env.begin()
|
||||
>>> for k, v in txn.cursor():
|
||||
>>> print(k, v)
|
||||
|
||||
b'image-000000001' b'\xff...'
|
||||
b'image-000000002' b'\xff...'
|
||||
b'image-000000003' b'\xff...'
|
||||
b'image-000000004' b'\xff...'
|
||||
b'image-000000005' b'\xff...'
|
||||
b'image-000000006' b'\xff...'
|
||||
b'image-000000007' b'\xff...'
|
||||
b'image-000000008' b'\xff...'
|
||||
b'image-000000009' b'\xff...'
|
||||
b'image-000000010' b'\xff...'
|
||||
b'label-000000001' b'GRAND'
|
||||
b'label-000000002' b'HOTEL'
|
||||
b'label-000000003' b'HOTEL'
|
||||
b'label-000000004' b'PACIFIC'
|
||||
b'label-000000005' b'03/09/2009'
|
||||
b'label-000000006' b'ANING'
|
||||
b'label-000000007' b'Virgin'
|
||||
b'label-000000008' b'america'
|
||||
b'label-000000009' b'ATTACK'
|
||||
b'label-000000010' b'DAVIDSON'
|
||||
b'num-samples' b'10'
|
||||
|
||||
```
|
||||
|
||||
#### 示例配置
|
||||
|
||||
以下是示例配置的一部分,我们在其中使用 `RecogLMDBDataset` 加载 toy 数据集。由于 `RecogLMDBDataset` 会将图像加载为 numpy 数组,因此如果要在数据管道中成功加载图像,应该记得把`LoadImageFromFile` 替换成 `LoadImageFromNDArray` 。
|
||||
|
||||
```python
|
||||
pipeline = [
|
||||
dict(
|
||||
type='LoadImageFromNDArray'),
|
||||
dict(
|
||||
type='LoadOCRAnnotations',
|
||||
with_text=True,
|
||||
),
|
||||
dict(
|
||||
type='PackTextRecogInputs',
|
||||
meta_keys=('img_path', 'ori_shape', 'img_shape'))
|
||||
]
|
||||
|
||||
toy_textrecog_train = dict(
|
||||
type='RecogLMDBDataset',
|
||||
data_root='tests/data/rec_toy_dataset/',
|
||||
ann_file='imgs.lmdb',
|
||||
pipeline=pipeline)
|
||||
|
||||
train_dataloader = dict(
|
||||
batch_size=16,
|
||||
num_workers=8,
|
||||
persistent_workers=True,
|
||||
sampler=dict(type='DefaultSampler', shuffle=True),
|
||||
dataset=toy_textrecog_train)
|
||||
```
|
||||
|
||||
### RecogTextDataset
|
||||
|
||||
在 MMOCR 1.0 之前,MMOCR 0.x 的文本识别任务的输入是文本文件。这些格式已在 MMOCR 1.0 中弃用,这个类随时可能被删除。[更多信息](../migration/dataset.md)
|
||||
|
||||
#### 标注格式
|
||||
|
||||
文本文件可以是 `txt` 格式或 `jsonl` 格式。简单的 `.txt` 标注通过空格将图像名称和词语标注分隔开,因此这种格式并无法处理文本实例中包含空格的情况。
|
||||
|
||||
```text
|
||||
img1.jpg OpenMMLab
|
||||
img2.jpg MMOCR
|
||||
```
|
||||
|
||||
`jsonl` 格式使用类似字典的结构来表示标注,其中键 `filename` 和 `text` 存储图像名称和单词标签。
|
||||
|
||||
```json
|
||||
{"filename": "img1.jpg", "text": "OpenMMLab"}
|
||||
{"filename": "img2.jpg", "text": "MMOCR"}
|
||||
```
|
||||
|
||||
#### 示例配置
|
||||
|
||||
以下是一个示例配置,我们在训练中使用 `RecogTextDataset` 加载 txt 标签,而在测试中使用 jsonl 标签。
|
||||
|
||||
```python
|
||||
pipeline = [
|
||||
dict(
|
||||
type='LoadImageFromFile'),
|
||||
dict(
|
||||
type='LoadOCRAnnotations',
|
||||
with_polygon=True,
|
||||
with_bbox=True,
|
||||
with_label=True,
|
||||
),
|
||||
dict(
|
||||
type='PackTextDetInputs',
|
||||
meta_keys=('img_path', 'ori_shape', 'img_shape'))
|
||||
]
|
||||
|
||||
# loading 0.x txt format annos
|
||||
txt_dataset = dict(
|
||||
type='RecogTextDataset',
|
||||
data_root=data_root,
|
||||
ann_file='old_label.txt',
|
||||
data_prefix=dict(img_path='imgs'),
|
||||
parser_cfg=dict(
|
||||
type='LineStrParser',
|
||||
keys=['filename', 'text'],
|
||||
keys_idx=[0, 1]),
|
||||
pipeline=pipeline)
|
||||
|
||||
|
||||
train_dataloader = dict(
|
||||
batch_size=16,
|
||||
num_workers=8,
|
||||
persistent_workers=True,
|
||||
sampler=dict(type='DefaultSampler', shuffle=True),
|
||||
dataset=txt_dataset)
|
||||
|
||||
# loading 0.x json line format annos
|
||||
jsonl_dataset = dict(
|
||||
type='RecogTextDataset',
|
||||
data_root=data_root,
|
||||
ann_file='old_label.jsonl',
|
||||
data_prefix=dict(img_path='imgs'),
|
||||
parser_cfg=dict(
|
||||
type='LineJsonParser',
|
||||
keys=['filename', 'text'],
|
||||
pipeline=pipeline))
|
||||
|
||||
test_dataloader = dict(
|
||||
batch_size=16,
|
||||
num_workers=8,
|
||||
persistent_workers=True,
|
||||
sampler=dict(type='DefaultSampler', shuffle=False),
|
||||
dataset=jsonl_dataset)
|
||||
```
|
||||
|
||||
### IcdarDataset
|
||||
|
||||
在 MMOCR 1.0 之前,MMOCR 0.x 的文本检测输入采用了类似 COCO 格式的注释。这些格式已在 MMOCR 1.0 中弃用,这个类在将来的任何时候都可能被删除。[更多信息](../migration/dataset.md)
|
||||
|
||||
#### 标注格式
|
||||
|
||||
```json
|
||||
{
|
||||
"images": [
|
||||
{
|
||||
"id": 1,
|
||||
"width": 800,
|
||||
"height": 600,
|
||||
"file_name": "test.jpg"
|
||||
}
|
||||
],
|
||||
"annotations": [
|
||||
{
|
||||
"id": 1,
|
||||
"image_id": 1,
|
||||
"category_id": 1,
|
||||
"bbox": [0,0,10,10],
|
||||
"segmentation": [
|
||||
[0,0,10,0,10,10,0,10]
|
||||
],
|
||||
"area": 100,
|
||||
"iscrowd": 0
|
||||
}
|
||||
]
|
||||
}
|
||||
|
||||
```
|
||||
|
||||
#### 配置示例
|
||||
|
||||
这是配置示例的一部分,其中我们令 `train_dataloader` 使用 `IcdarDataset` 来加载旧标签。
|
||||
|
||||
```python
|
||||
pipeline = [
|
||||
dict(
|
||||
type='LoadImageFromFile'),
|
||||
dict(
|
||||
type='LoadOCRAnnotations',
|
||||
with_polygon=True,
|
||||
with_bbox=True,
|
||||
with_label=True,
|
||||
),
|
||||
dict(
|
||||
type='PackTextDetInputs',
|
||||
meta_keys=('img_path', 'ori_shape', 'img_shape'))
|
||||
]
|
||||
|
||||
icdar2015_textdet_train = dict(
|
||||
type='IcdarDatasetDataset',
|
||||
data_root='data/det/icdar2015',
|
||||
ann_file='instances_training.json',
|
||||
filter_cfg=dict(filter_empty_gt=True, min_size=32),
|
||||
pipeline=pipeline)
|
||||
|
||||
train_dataloader = dict(
|
||||
batch_size=16,
|
||||
num_workers=8,
|
||||
persistent_workers=True,
|
||||
sampler=dict(type='DefaultSampler', shuffle=True),
|
||||
dataset=icdar2015_textdet_train)
|
||||
```
|
||||
|
||||
### WildReceiptDataset
|
||||
|
||||
该类为 [WildReceipt](https://mmocr.readthedocs.io/en/dev-1.x/user_guides/data_prepare/datasetzoo.html#wildreceipt) 数据集定制。
|
||||
|
||||
#### 标注格式
|
||||
|
||||
```json
|
||||
// Close Set
|
||||
{
|
||||
"file_name": "image_files/Image_16/11/d5de7f2a20751e50b84c747c17a24cd98bed3554.jpeg",
|
||||
"height": 1200,
|
||||
"width": 1600,
|
||||
"annotations":
|
||||
[
|
||||
{
|
||||
"box": [550.0, 190.0, 937.0, 190.0, 937.0, 104.0, 550.0, 104.0],
|
||||
"text": "SAFEWAY",
|
||||
"label": 1
|
||||
},
|
||||
{
|
||||
"box": [1048.0, 211.0, 1074.0, 211.0, 1074.0, 196.0, 1048.0, 196.0],
|
||||
"text": "TM",
|
||||
"label": 25
|
||||
}
|
||||
], //...
|
||||
}
|
||||
|
||||
// Open Set
|
||||
{
|
||||
"file_name": "image_files/Image_12/10/845be0dd6f5b04866a2042abd28d558032ef2576.jpeg",
|
||||
"height": 348,
|
||||
"width": 348,
|
||||
"annotations":
|
||||
[
|
||||
{
|
||||
"box": [114.0, 19.0, 230.0, 19.0, 230.0, 1.0, 114.0, 1.0],
|
||||
"text": "CHOEUN",
|
||||
"label": 2,
|
||||
"edge": 1
|
||||
},
|
||||
{
|
||||
"box": [97.0, 35.0, 236.0, 35.0, 236.0, 19.0, 97.0, 19.0],
|
||||
"text": "KOREANRESTAURANT",
|
||||
"label": 2,
|
||||
"edge": 1
|
||||
}
|
||||
]
|
||||
}
|
||||
```
|
||||
|
||||
#### 配置示例
|
||||
|
||||
请参考 [SDMGR 的配置](https://github.com/open-mmlab/mmocr/blob/f30c16ce96bd2393570c04eeb9cf48a7916315cc/configs/kie/sdmgr/sdmgr_novisual_60e_wildreceipt.py)。
|
||||
|
|
|
@ -30,9 +30,9 @@
|
|||
basic_concepts/structures.md
|
||||
basic_concepts/transforms.md
|
||||
basic_concepts/evaluation.md
|
||||
basic_concepts/datasets.md
|
||||
basic_concepts/overview.md
|
||||
basic_concepts/data_flow.md
|
||||
basic_concepts/datasets.md
|
||||
basic_concepts/models.md
|
||||
basic_concepts/visualizers.md
|
||||
basic_concepts/convention.md
|
||||
|
|
|
@ -4,62 +4,12 @@
|
|||
|
||||
经过数十年的发展,OCR 领域涌现出了一系列的相关数据集,这些数据集往往采用风格各异的格式来提供文本的标注文件,使得用户在使用这些数据集时不得不进行格式转换。因此,为了方便用户进行数据集准备,我们提供了[一键式的数据准备脚本](./data_prepare/dataset_preparer.md),使得用户仅需使用一行命令即可完成数据集准备的全部步骤。
|
||||
|
||||
下面,我们对 MMOCR 内支持的各任务的数据格式进行简要的介绍。
|
||||
在这一节,我们将介绍一个典型的数据集准备流程:
|
||||
|
||||
- 如以下代码块所示,文本检测任务采用数据格式 `TextDetDataset`,其中存放了文本检测任务所需的边界盒标注、文件名等信息。我们在 `tests/data/det_toy_dataset/instances_test.json` 路径中提供了一个示例标注文件。
|
||||
1. [下载数据集并将其格式转换为 MMOCR 支持的格式](#数据集下载及格式转换)
|
||||
2. [修改配置文件](#修改配置文件)
|
||||
|
||||
```json
|
||||
{
|
||||
"metainfo":
|
||||
{
|
||||
"dataset_type": "TextDetDataset",
|
||||
"task_name": "textdet",
|
||||
"category": [{"id": 0, "name": "text"}]
|
||||
},
|
||||
"data_list":
|
||||
[
|
||||
{
|
||||
"img_path": "test_img.jpg",
|
||||
"height": 640,
|
||||
"width": 640,
|
||||
"instances":
|
||||
[
|
||||
{
|
||||
"polygon": [0, 0, 0, 10, 10, 20, 20, 0],
|
||||
"bbox": [0, 0, 10, 20],
|
||||
"bbox_label": 0,
|
||||
"ignore": false
|
||||
}
|
||||
],
|
||||
//...
|
||||
}
|
||||
]
|
||||
}
|
||||
```
|
||||
|
||||
- 如以下代码块所示,文本识别任务采用数据格式 `TextRecogDataset`,其中存放了文本识别任务所需的文本内容及图片路径等信息。我们在 `tests/data/rec_toy_dataset/labels.json` 路径中提供了一个示例标注文件。
|
||||
|
||||
```json
|
||||
{
|
||||
"metainfo":
|
||||
{
|
||||
"dataset_type": "TextRecogDataset",
|
||||
"task_name": "textrecog",
|
||||
},
|
||||
"data_list":
|
||||
[
|
||||
{
|
||||
"img_path": "test_img.jpg",
|
||||
"instances":
|
||||
[
|
||||
{
|
||||
"text": "GRAND"
|
||||
}
|
||||
]
|
||||
}
|
||||
]
|
||||
}
|
||||
```
|
||||
然而,如果你已经有了 MMOCR 支持的格式的数据集,那么第一步就不是必须的。你可以阅读[数据集类及标注格式](../basic_concepts/datasets.md#数据集类及标注格式)来了解更多细节。
|
||||
|
||||
## 数据集下载及格式转换
|
||||
|
||||
|
@ -86,7 +36,7 @@ data/icdar2015
|
|||
python tools/analysis_tools/browse_dataset.py configs/textdet/_base_/datasets/icdar2015.py
|
||||
```
|
||||
|
||||
## 数据集配置文件
|
||||
## 修改配置文件
|
||||
|
||||
### 单数据集训练
|
||||
|
||||
|
|
Loading…
Reference in New Issue