mirror of https://github.com/open-mmlab/mmocr.git
Compare commits
15 Commits
Author | SHA1 | Date |
---|---|---|
|
966296f26a | |
|
2caab0a4e7 | |
|
b18a09b2f0 | |
|
9551af6e5a | |
|
1dcd6fa695 | |
|
6b3f6f5285 | |
|
0cd2878b04 | |
|
bbe8964f00 | |
|
a344280bcb | |
|
4eb3cc7de5 | |
|
e9a31ddd70 | |
|
1e696887b9 | |
|
231cff5da2 | |
|
8afc79f370 | |
|
9e713c63fe |
.circleci
.github/ISSUE_TEMPLATE
configs/textrecog/satrn
dataset_zoo/iiit5k
docs
en
zh_cn
mmocr
apis/inferencers
datasets/preparers
dumpers
obtainers
models/textdet/detectors
requirements
tools
dataset_converters/textrecog
visualizations
|
@ -129,6 +129,7 @@ workflows:
|
|||
ignore:
|
||||
- dev-1.x
|
||||
- 1.x
|
||||
- main
|
||||
pr_stage_test:
|
||||
when:
|
||||
not:
|
||||
|
@ -141,6 +142,7 @@ workflows:
|
|||
ignore:
|
||||
- dev-1.x
|
||||
- test-1.x
|
||||
- main
|
||||
- build_cpu:
|
||||
name: minimum_version_cpu
|
||||
torch: 1.6.0
|
||||
|
@ -191,3 +193,4 @@ workflows:
|
|||
branches:
|
||||
only:
|
||||
- dev-1.x
|
||||
- main
|
||||
|
|
|
@ -1,6 +1,6 @@
|
|||
name: "🐞 Bug report"
|
||||
description: "Create a report to help us reproduce and fix the bug"
|
||||
labels: bug
|
||||
labels: kind/bug
|
||||
title: "[Bug] "
|
||||
|
||||
body:
|
||||
|
|
|
@ -196,11 +196,11 @@ We hope the toolbox and benchmark could serve the growing research community by
|
|||
If you find this project useful in your research, please consider cite:
|
||||
|
||||
```bibtex
|
||||
@article{mmocr2021,
|
||||
@article{mmocr2022,
|
||||
title={MMOCR: A Comprehensive Toolbox for Text Detection, Recognition and Understanding},
|
||||
author={Kuang, Zhanghui and Sun, Hongbin and Li, Zhizhong and Yue, Xiaoyu and Lin, Tsui Hin and Chen, Jianyong and Wei, Huaqiang and Zhu, Yiqin and Gao, Tong and Zhang, Wenwei and Chen, Kai and Zhang, Wayne and Lin, Dahua},
|
||||
journal= {arXiv preprint arXiv:2108.06543},
|
||||
year={2021}
|
||||
author={MMOCR Developer Team},
|
||||
howpublished = {\url{https://github.com/open-mmlab/mmocr}},
|
||||
year={2022}
|
||||
}
|
||||
```
|
||||
|
||||
|
|
|
@ -232,10 +232,10 @@ MMOCR 是一款由来自不同高校和企业的研发人员共同参与贡献
|
|||
|
||||
## 欢迎加入 OpenMMLab 社区
|
||||
|
||||
扫描下方的二维码可关注 OpenMMLab 团队的 [知乎官方账号](https://www.zhihu.com/people/openmmlab),加入 OpenMMLab 团队的 [官方交流 QQ 群](https://r.vansin.top/?r=join-qq),或通过添加微信“Open小喵Lab”加入官方交流微信群。
|
||||
扫描下方的二维码可关注 OpenMMLab 团队的 知乎官方账号,扫描下方微信二维码添加喵喵好友,进入 MMOCR 微信交流社群。【加好友申请格式:研究方向+地区+学校/公司+姓名】
|
||||
|
||||
<div align="center">
|
||||
<img src="https://raw.githubusercontent.com/open-mmlab/mmcv/master/docs/en/_static/zhihu_qrcode.jpg" height="400" /> <img src="https://cdn.vansin.top/OpenMMLab/q3.png" height="400" /> <img src="https://raw.githubusercontent.com/open-mmlab/mmcv/master/docs/en/_static/wechat_qrcode.jpg" height="400" />
|
||||
<img src="https://raw.githubusercontent.com/open-mmlab/mmcv/master/docs/en/_static/zhihu_qrcode.jpg" height="400" /> <img src="https://github.com/open-mmlab/mmocr/assets/62195058/bf1e53fe-df4f-4296-9e1b-61db8971985e" height="400" />
|
||||
</div>
|
||||
|
||||
我们会在 OpenMMLab 社区为大家
|
||||
|
|
|
@ -12,6 +12,8 @@ _base_ = [
|
|||
'_base_satrn_shallow.py',
|
||||
]
|
||||
|
||||
train_cfg = dict(type='EpochBasedTrainLoop', max_epochs=20, val_interval=1)
|
||||
|
||||
# dataset settings
|
||||
train_list = [_base_.mjsynth_textrecog_train, _base_.synthtext_textrecog_train]
|
||||
test_list = [
|
||||
|
|
|
@ -17,7 +17,7 @@ train_preparer = dict(
|
|||
url='https://download.openmmlab.com/mmocr/data/mixture/IIIT5K/'
|
||||
'train_label.txt',
|
||||
save_name='iiit5k_train.txt',
|
||||
md5='f4731ce1eadc259532c2834266e5126d',
|
||||
md5='beee914aaf3ec5794622b843d743c5a6',
|
||||
content=['annotation'],
|
||||
mapping=[['iiit5k_train.txt', 'annotations/train.txt']])
|
||||
]),
|
||||
|
@ -47,7 +47,7 @@ test_preparer = dict(
|
|||
url='https://download.openmmlab.com/mmocr/data/mixture/IIIT5K/'
|
||||
'test_label.txt',
|
||||
save_name='iiit5k_test.txt',
|
||||
md5='82ecfa34a28d59284d1914dc906f5380',
|
||||
md5='117bcd9b4245f61fa57bfb37361674b3',
|
||||
content=['annotation'],
|
||||
mapping=[['iiit5k_test.txt', 'annotations/test.txt']])
|
||||
]),
|
||||
|
|
File diff suppressed because it is too large
Load Diff
|
@ -127,24 +127,6 @@ html_theme_options = {
|
|||
},
|
||||
]
|
||||
},
|
||||
{
|
||||
'name':
|
||||
'Version',
|
||||
'children': [
|
||||
{
|
||||
'name': 'MMOCR 0.x',
|
||||
'url': 'https://mmocr.readthedocs.io/en/latest/',
|
||||
'description': 'Main branch'
|
||||
},
|
||||
{
|
||||
'name': 'MMOCR 1.x',
|
||||
'url': 'https://mmocr.readthedocs.io/en/dev-1.x/',
|
||||
'description': '1.x branch'
|
||||
},
|
||||
],
|
||||
'active':
|
||||
True,
|
||||
},
|
||||
],
|
||||
# Specify the language of shared menu
|
||||
'menu_lang':
|
||||
|
|
|
@ -237,7 +237,8 @@ MMOCR has different version requirements on MMEngine, MMCV and MMDetection at ea
|
|||
|
||||
| MMOCR | MMEngine | MMCV | MMDetection |
|
||||
| -------------- | --------------------------- | -------------------------- | --------------------------- |
|
||||
| dev-1.x | 0.7.1 \<= mmengine \< 1.0.0 | 2.0.0rc4 \<= mmcv \< 2.1.0 | 3.0.0rc5 \<= mmdet \< 3.1.0 |
|
||||
| dev-1.x | 0.7.1 \<= mmengine \< 1.1.0 | 2.0.0rc4 \<= mmcv \< 2.1.0 | 3.0.0rc5 \<= mmdet \< 3.2.0 |
|
||||
| 1.0.1 | 0.7.1 \<= mmengine \< 1.1.0 | 2.0.0rc4 \<= mmcv \< 2.1.0 | 3.0.0rc5 \<= mmdet \< 3.2.0 |
|
||||
| 1.0.0 | 0.7.1 \<= mmengine \< 1.0.0 | 2.0.0rc4 \<= mmcv \< 2.1.0 | 3.0.0rc5 \<= mmdet \< 3.1.0 |
|
||||
| 1.0.0rc6 | 0.6.0 \<= mmengine \< 1.0.0 | 2.0.0rc4 \<= mmcv \< 2.1.0 | 3.0.0rc5 \<= mmdet \< 3.1.0 |
|
||||
| 1.0.0rc\[4-5\] | 0.1.0 \<= mmengine \< 1.0.0 | 2.0.0rc1 \<= mmcv \< 2.1.0 | 3.0.0rc0 \<= mmdet \< 3.1.0 |
|
||||
|
|
|
@ -1,18 +1,17 @@
|
|||
# Dataset Preparer (Beta)
|
||||
|
||||
```{note}
|
||||
Dataset Preparer is still in beta version and might not be stable enough. You
|
||||
are welcome to try it out and report any issues to us.
|
||||
Dataset Preparer is still in beta version and might not be stable enough. You are welcome to try it out and report any issues to us.
|
||||
```
|
||||
|
||||
## One-click data preparation script
|
||||
|
||||
MMOCR provides a unified one-stop data preparation script `prepare_dataset.py`.
|
||||
|
||||
Only one line of command is needed to complete the data download, decompression, and format conversion.
|
||||
Only one line of command is needed to complete the data download, decompression, format conversion, and basic configure generation.
|
||||
|
||||
```bash
|
||||
python tools/dataset_converters/prepare_dataset.py [$DATASET_NAME] --task [$TASK] --nproc [$NPROC]
|
||||
python tools/dataset_converters/prepare_dataset.py [-h] [--nproc NPROC] [--task {textdet,textrecog,textspotting,kie}] [--splits SPLITS [SPLITS ...]] [--lmdb] [--overwrite-cfg] [--dataset-zoo-path DATASET_ZOO_PATH] datasets [datasets ...]
|
||||
```
|
||||
|
||||
| ARGS | Type | Description |
|
||||
|
@ -28,13 +27,13 @@ python tools/dataset_converters/prepare_dataset.py [$DATASET_NAME] --task [$TASK
|
|||
For example, the following command shows how to use the script to prepare the ICDAR2015 dataset for text detection task.
|
||||
|
||||
```bash
|
||||
python tools/dataset_converters/prepare_dataset.py icdar2015 --task textdet
|
||||
python tools/dataset_converters/prepare_dataset.py icdar2015 --task textdet --overwrite-cfg
|
||||
```
|
||||
|
||||
Also, the script supports preparing multiple datasets at the same time. For example, the following command shows how to prepare the ICDAR2015 and TotalText datasets for text recognition task.
|
||||
|
||||
```bash
|
||||
python tools/dataset_converters/prepare_dataset.py icdar2015 totaltext --task textrecog
|
||||
python tools/dataset_converters/prepare_dataset.py icdar2015 totaltext --task textrecog --overwrite-cfg
|
||||
```
|
||||
|
||||
To check the supported datasets of Dataset Preparer, please refer to [Dataset Zoo](./datasetzoo.md). Some of other datasets that need to be prepared manually are listed in [Text Detection](./det.md) and [Text Recognition](./recog.md).
|
||||
|
@ -81,30 +80,30 @@ For example, if we want to change the training set of `configs/textrecog/crnn/cr
|
|||
]
|
||||
```
|
||||
|
||||
### Configuration of Dataset Preparer
|
||||
## Design
|
||||
|
||||
Dataset preparer uses a modular design to enhance extensibility, which allows users to extend it to other public or private datasets easily. The configuration files of the dataset preparers are stored in the `dataset_zoo/`, where all the configs of currently supported datasets can be found here. The directory structure is as follows:
|
||||
There are many OCR datasets with different languages, annotation formats, and scenarios. There are generally two ways to use these datasets: to quickly understand the relevant information about the dataset, or to use it to train models. To meet these two usage scenarios, MMOCR provides dataset automatic preparation scripts. The dataset automatic preparation script uses modular design, which greatly enhances scalability, and allows users to easily configure other public or private datasets. The configuration files for the dataset automatic preparation script are uniformly stored in the `dataset_zoo/` directory. Users can find all the configuration files for the dataset preparation scripts officially supported by MMOCR in this directory. The directory structure of this folder is as follows:
|
||||
|
||||
```text
|
||||
dataset_zoo/
|
||||
├── icdar2015
|
||||
│ ├── metafile.yml
|
||||
│ ├── sample_anno.md
|
||||
│ ├── textdet.py
|
||||
│ ├── textrecog.py
|
||||
│ └── textspotting.py
|
||||
└── wildreceipt
|
||||
├── metafile.yml
|
||||
├── sample_anno.md
|
||||
├── kie.py
|
||||
├── textdet.py
|
||||
├── textrecog.py
|
||||
└── textspotting.py
|
||||
```
|
||||
|
||||
`metafile.yml` is the metafile of the dataset, which contains the basic information of the dataset, including the year of publication, the author of the paper, and other information such as license. The other files named by the task are the configuration files of the dataset preparer, which are used to configure the download, decompression, format conversion, etc. of the dataset. These configs are in Python format, and their usage is completely consistent with the configuration files in MMOCR repo. See [Configuration File Documentation](../config.md) for detailed usage.
|
||||
### Dataset-related Information
|
||||
|
||||
#### Metafile
|
||||
|
||||
Take the ICDAR2015 dataset as an example, the `metafile.yml` stores the basic information of the dataset:
|
||||
The relevant information of a dataset includes the annotation format, annotation examples, and basic statistical information of the dataset. Although this information can be found on the official website of each dataset, it is scattered across various websites, and users need to spend a lot of time to discover the basic information of the dataset. Therefore, MMOCR has designed some paradigms to help users quickly understand the basic information of the dataset. MMOCR divides the relevant information of the dataset into two parts. One part is the basic information of the dataset, including the year of publication, the authors of the paper, and copyright information, etc. The other part is the annotation information of the dataset, including the annotation format and annotation examples. MMOCR provides a paradigm for each part, and contributors can fill in the basic information of the dataset according to the paradigm. This way, users can quickly understand the basic information of the dataset. Based on the basic information of the dataset, MMOCR provides a `metafile.yml` file, which contains the basic information of the corresponding dataset, including the year of publication, the authors of the paper, and copyright information, etc. In this way, users can quickly understand the basic information of the dataset. This file is not mandatory during the dataset preparation process (so users can ignore it when adding their own private datasets), but to better understand the information of various public datasets, MMOCR recommends that users read the corresponding metafile information before using the dataset preparation script to understand whether the characteristics of the dataset meet the user's needs. MMOCR uses ICDAR2015 as an example, and its sample content is shown below:
|
||||
|
||||
```yaml
|
||||
Name: 'Incidental Scene Text IC15'
|
||||
|
@ -137,104 +136,640 @@ Data:
|
|||
Link: https://creativecommons.org/licenses/by/4.0/
|
||||
```
|
||||
|
||||
It is not mandatory to use the metafile in the dataset preparation process (so users can ignore this file when preparing private datasets), but in order to better understand the information of each public dataset, we recommend that users read the metafile before preparing the dataset, which will help to understand whether the datasets meet their needs.
|
||||
Specifically, MMOCR lists the meaning of each field in the following table:
|
||||
|
||||
```{warning}
|
||||
The following section is outdated as of MMOCR 1.0.0rc6.
|
||||
```
|
||||
| Field Name | Meaning |
|
||||
| :--------------- | :------------------------------------------------------------------------------------------------------- |
|
||||
| Name | The name of the dataset |
|
||||
| Paper.Title | The title of the paper for the dataset |
|
||||
| Paper.URL | The URL of the paper for the dataset |
|
||||
| Paper.Venue | The venue of the paper for the dataset |
|
||||
| Paper.Year | The year of publication for the paper |
|
||||
| Paper.BibTeX | The BibTeX citation of the paper for the dataset |
|
||||
| Data.Website | The official website of the dataset |
|
||||
| Data.Language | The supported languages of the dataset |
|
||||
| Data.Scene | The supported scenes of the dataset, such as `Natural Scene`, `Document`, `Handwritten`, etc. |
|
||||
| Data.Granularity | The supported granularities of the dataset, such as `Character`, `Word`, `Line`, etc. |
|
||||
| Data.Tasks | The supported tasks of the dataset, such as `textdet`, `textrecog`, `textspotting`, `kie`, etc. |
|
||||
| Data.License | License information for the dataset. Use `N/A` if no license exists. |
|
||||
| Data.Format | File format of the annotation files, such as `.txt`, `.xml`, `.json`, etc. |
|
||||
| Data.Keywords | Keywords describing the characteristics of the dataset, such as `Horizontal`, `Vertical`, `Curved`, etc. |
|
||||
|
||||
#### Config of Dataset Preparer
|
||||
For the annotation information of the dataset, MMOCR provides a `sample_anno.md` file, which users can use as a template to fill in the annotation information of the dataset, so that users can quickly understand the annotation information of the dataset. MMOCR uses ICDAR2015 as an example, and the sample content is as follows:
|
||||
|
||||
Next, we will introduce the conventional fields and usage of the dataset preparer configuration files.
|
||||
````markdown
|
||||
**Text Detection**
|
||||
|
||||
In the configuration files, there are two fields `data_root` and `cache_path`, which are used to store the converted dataset and the temporary files such as the archived files downloaded during the data preparation process.
|
||||
```text
|
||||
# x1,y1,x2,y2,x3,y3,x4,y4,trans
|
||||
|
||||
377,117,463,117,465,130,378,130,Genaxis Theatre
|
||||
493,115,519,115,519,131,493,131,[06]
|
||||
374,155,409,155,409,170,374,170,###
|
||||
````
|
||||
|
||||
`sample_anno.md` provides annotation information for different tasks of the dataset, including the format of the annotation files (text corresponds to `txt` files, and the format of the annotation files can also be found in `meta.yml`), and examples of the annotations.
|
||||
|
||||
With the information in these two files, users can quickly understand the basic information of the dataset. Additionally, MMOCR has summarized the basic information of all datasets, and users can view the basic information of all datasets in the [Overview](.overview.md).
|
||||
|
||||
### Dataset Usage
|
||||
|
||||
After decades of development, the OCR field has seen a series of related datasets emerge, often providing text annotation files in various styles, making it necessary for users to perform format conversion when using these datasets. Therefore, to facilitate dataset preparation for users, we have designed the Dataset Preparer to help users quickly prepare datasets in the format supported by MMOCR. For details, please refer to the [Dataset Format](../../basic_concepts/datasets.md) document. The following figure shows a typical workflow for running the Dataset Preparer.
|
||||
|
||||
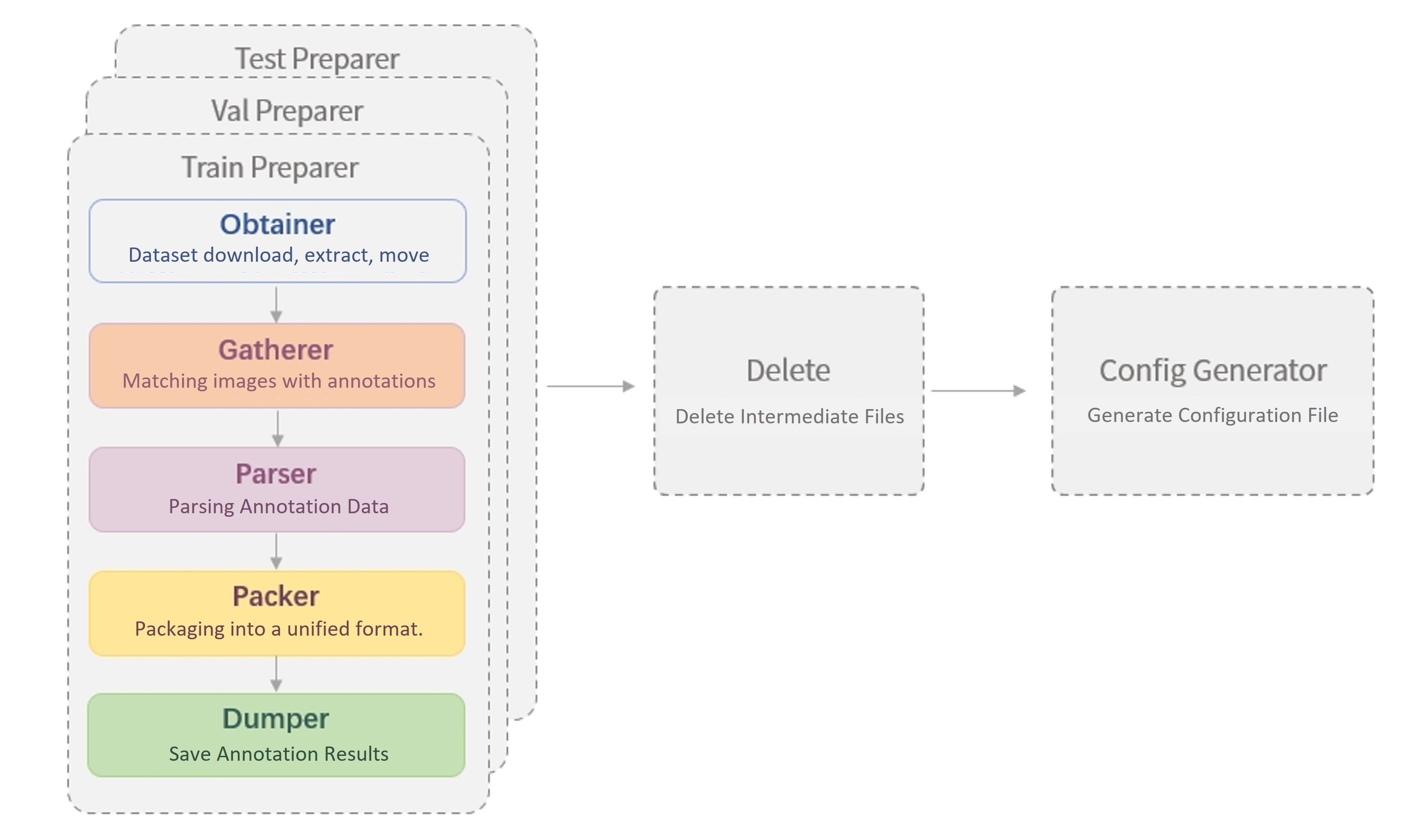
|
||||
|
||||
The figure shows that when running the Dataset Preparer, the following operations will be performed in sequence:
|
||||
|
||||
1. For the training set, validation set, and test set, the preparers will perform:
|
||||
1. [Dataset download, extraction, and movement (Obtainer)](#Dataset-download-extraction-and-movement-obtainer)
|
||||
2. [Matching annotations with images (Gatherer)](#dataset-collection-gatherer)
|
||||
3. [Parsing original annotations (Parser)](#dataset-parsing-parser)
|
||||
4. [Packing annotations into a unified format (Packer)](#dataset-conversion-packer)
|
||||
5. [Saving annotations (Dumper)](#annotation-saving-dumper)
|
||||
2. Delete files (Delete)
|
||||
3. Generate the configuration file for the data set (Config Generator).
|
||||
|
||||
To handle various types of datasets, MMOCR has designed each component as a plug-and-play module, and allows users to configure the dataset preparation process through configuration files located in `dataset_zoo/`. These configuration files are in Python format and can be used in the same way as other configuration files in MMOCR, as described in the [Configuration File documentation](../config.md).
|
||||
|
||||
In `dataset_zoo/`, each dataset has its own folder, and the configuration files are named after the task to distinguish different configurations under different tasks. Taking the text detection part of ICDAR2015 as an example, the sample configuration file `dataset_zoo/icdar2015/textdet.py` is shown below:
|
||||
|
||||
```python
|
||||
data_root = './data/icdar2015'
|
||||
cache_path = './data/cache'
|
||||
data_root = 'data/icdar2015'
|
||||
cache_path = 'data/cache'
|
||||
train_preparer = dict(
|
||||
obtainer=dict(
|
||||
type='NaiveDataObtainer',
|
||||
cache_path=cache_path,
|
||||
files=[
|
||||
dict(
|
||||
url='https://rrc.cvc.uab.es/downloads/ch4_training_images.zip',
|
||||
save_name='ic15_textdet_train_img.zip',
|
||||
md5='c51cbace155dcc4d98c8dd19d378f30d',
|
||||
content=['image'],
|
||||
mapping=[['ic15_textdet_train_img', 'textdet_imgs/train']]),
|
||||
dict(
|
||||
url='https://rrc.cvc.uab.es/downloads/'
|
||||
'ch4_training_localization_transcription_gt.zip',
|
||||
save_name='ic15_textdet_train_gt.zip',
|
||||
md5='3bfaf1988960909014f7987d2343060b',
|
||||
content=['annotation'],
|
||||
mapping=[['ic15_textdet_train_gt', 'annotations/train']]),
|
||||
]),
|
||||
gatherer=dict(
|
||||
type='PairGatherer',
|
||||
img_suffixes=['.jpg', '.JPG'],
|
||||
rule=[r'img_(\d+)\.([jJ][pP][gG])', r'gt_img_\1.txt']),
|
||||
parser=dict(type='ICDARTxtTextDetAnnParser', encoding='utf-8-sig'),
|
||||
packer=dict(type='TextDetPacker'),
|
||||
dumper=dict(type='JsonDumper'),
|
||||
)
|
||||
|
||||
test_preparer = dict(
|
||||
obtainer=dict(
|
||||
type='NaiveDataObtainer',
|
||||
cache_path=cache_path,
|
||||
files=[
|
||||
dict(
|
||||
url='https://rrc.cvc.uab.es/downloads/ch4_test_images.zip',
|
||||
save_name='ic15_textdet_test_img.zip',
|
||||
md5='97e4c1ddcf074ffcc75feff2b63c35dd',
|
||||
content=['image'],
|
||||
mapping=[['ic15_textdet_test_img', 'textdet_imgs/test']]),
|
||||
dict(
|
||||
url='https://rrc.cvc.uab.es/downloads/'
|
||||
'Challenge4_Test_Task4_GT.zip',
|
||||
save_name='ic15_textdet_test_gt.zip',
|
||||
md5='8bce173b06d164b98c357b0eb96ef430',
|
||||
content=['annotation'],
|
||||
mapping=[['ic15_textdet_test_gt', 'annotations/test']]),
|
||||
]),
|
||||
gatherer=dict(
|
||||
type='PairGatherer',
|
||||
img_suffixes=['.jpg', '.JPG'],
|
||||
rule=[r'img_(\d+)\.([jJ][pP][gG])', r'gt_img_\1.txt']),
|
||||
parser=dict(type='ICDARTxtTextDetAnnParser', encoding='utf-8-sig'),
|
||||
packer=dict(type='TextDetPacker'),
|
||||
dumper=dict(type='JsonDumper'),
|
||||
)
|
||||
|
||||
delete = ['annotations', 'ic15_textdet_test_img', 'ic15_textdet_train_img']
|
||||
config_generator = dict(type='TextDetConfigGenerator')
|
||||
```
|
||||
|
||||
Data preparation usually contains two steps: "raw data preparation" and "format conversion and saving". Therefore, we use the `data_obtainer` and `data_converter` to configure the behavior of these two steps. In some cases, users can also ignore `data_converter` to only download and decompress the raw data, without performing format conversion and saving. Or, for the local stored dataset, use ignore `data_obtainer` to only perform format conversion and saving.
|
||||
#### Dataset download extraction and movement (Obtainer)
|
||||
|
||||
Take the text detection task of the ICDAR2015 dataset (`dataset_zoo/icdar2015/textdet.py`) as an example:
|
||||
The `obtainer` module in Dataset Preparer is responsible for downloading, extracting, and moving the dataset. Currently, MMOCR only provides the `NaiveDataObtainer`. Generally speaking, the built-in `NaiveDataObtainer` is sufficient for downloading most datasets that can be accessed through direct links, and supports operations such as extraction, moving files, and renaming. However, MMOCR currently does not support automatically downloading datasets stored in resources that require login, such as Baidu or Google Drive. Here is a brief introduction to the `NaiveDataObtainer`.
|
||||
|
||||
| Field Name | Meaning |
|
||||
| ---------- | -------------------------------------------------------------------------------------------- |
|
||||
| cache_path | Dataset cache path, used to store the compressed files downloaded during dataset preparation |
|
||||
| data_root | Root directory where the dataset is stored |
|
||||
| files | Dataset file list, used to describe the download information of the dataset |
|
||||
|
||||
The `files` field is a list, and each element in the list is a dictionary used to describe the download information of a dataset file. The table below shows the meaning of each field:
|
||||
|
||||
| Field Name | Meaning |
|
||||
| ------------------ | ------------------------------------------------------------------------------------------------------------------------------------------ |
|
||||
| url | Download link for the dataset file |
|
||||
| save_name | Name used to save the dataset file |
|
||||
| md5 (optional) | MD5 hash of the dataset file, used to check if the downloaded file is complete |
|
||||
| split (optional) | Dataset split the file belongs to, such as `train`, `test`, etc., this field can be omitted |
|
||||
| content (optional) | Content of the dataset file, such as `image`, `annotation`, etc., this field can be omitted |
|
||||
| mapping (optional) | Decompression mapping of the dataset file, used to specify the storage location of the file after decompression, this field can be omitted |
|
||||
|
||||
The Dataset Preparer follows the following conventions:
|
||||
|
||||
- Images of different types of datasets are moved to the corresponding category `{taskname}_imgs/{split}/` folder, such as `textdet_imgs/train/`.
|
||||
- For a annotation file containing annotation information for all images, the annotations are moved to `annotations/{split}.*` file, such as `annotations/train.json`.
|
||||
- For a annotation file containing annotation information for one image, all annotation files are moved to `annotations/{split}/` folder, such as `annotations/train/`.
|
||||
- For some other special cases, such as all training, testing, and validation images are in one folder, the images can be moved to a self-set folder, such as `{taskname}_imgs/imgs/`, and the image storage location should be specified in the subsequent `gatherer` module.
|
||||
|
||||
An example configuration is as follows:
|
||||
|
||||
```python
|
||||
data_obtainer = dict(
|
||||
type='NaiveDataObtainer',
|
||||
cache_path=cache_path,
|
||||
data_root=data_root,
|
||||
files=[
|
||||
obtainer=dict(
|
||||
type='NaiveDataObtainer',
|
||||
cache_path=cache_path,
|
||||
files=[
|
||||
dict(
|
||||
url='https://rrc.cvc.uab.es/downloads/ch4_training_images.zip',
|
||||
save_name='ic15_textdet_train_img.zip',
|
||||
md5='c51cbace155dcc4d98c8dd19d378f30d',
|
||||
content=['image'],
|
||||
mapping=[['ic15_textdet_train_img', 'textdet_imgs/train']]),
|
||||
dict(
|
||||
url='https://rrc.cvc.uab.es/downloads/'
|
||||
'ch4_training_localization_transcription_gt.zip',
|
||||
save_name='ic15_textdet_train_gt.zip',
|
||||
md5='3bfaf1988960909014f7987d2343060b',
|
||||
content=['annotation'],
|
||||
mapping=[['ic15_textdet_train_gt', 'annotations/train']]),
|
||||
]),
|
||||
```
|
||||
|
||||
#### Dataset collection (Gatherer)
|
||||
|
||||
The `gatherer` module traverses the files in the dataset directory, matches image files with their corresponding annotation files, and organizes a file list for the `parser` module to read. Therefore, it is necessary to know the matching rules between image files and annotation files in the current dataset. There are two commonly used annotation storage formats for OCR datasets: one is multiple annotation files corresponding to multiple images, and the other is a single annotation file corresponding to multiple images, for example:
|
||||
|
||||
```text
|
||||
Many-to-Many
|
||||
├── {taskname}_imgs/{split}/img_img_1.jpg
|
||||
├── annotations/{split}/gt_img_1.txt
|
||||
├── {taskname}_imgs/{split}/img_2.jpg
|
||||
├── annotations/{split}/gt_img_2.txt
|
||||
├── {taskname}_imgs/{split}/img_3.JPG
|
||||
├── annotations/{split}/gt_img_3.txt
|
||||
|
||||
One-to-Many
|
||||
├── {taskname}/{split}/img_1.jpg
|
||||
├── {taskname}/{split}/img_2.jpg
|
||||
├── {taskname}/{split}/img_3.JPG
|
||||
├── annotations/gt.txt
|
||||
```
|
||||
|
||||
Specific design is as follows:
|
||||
|
||||
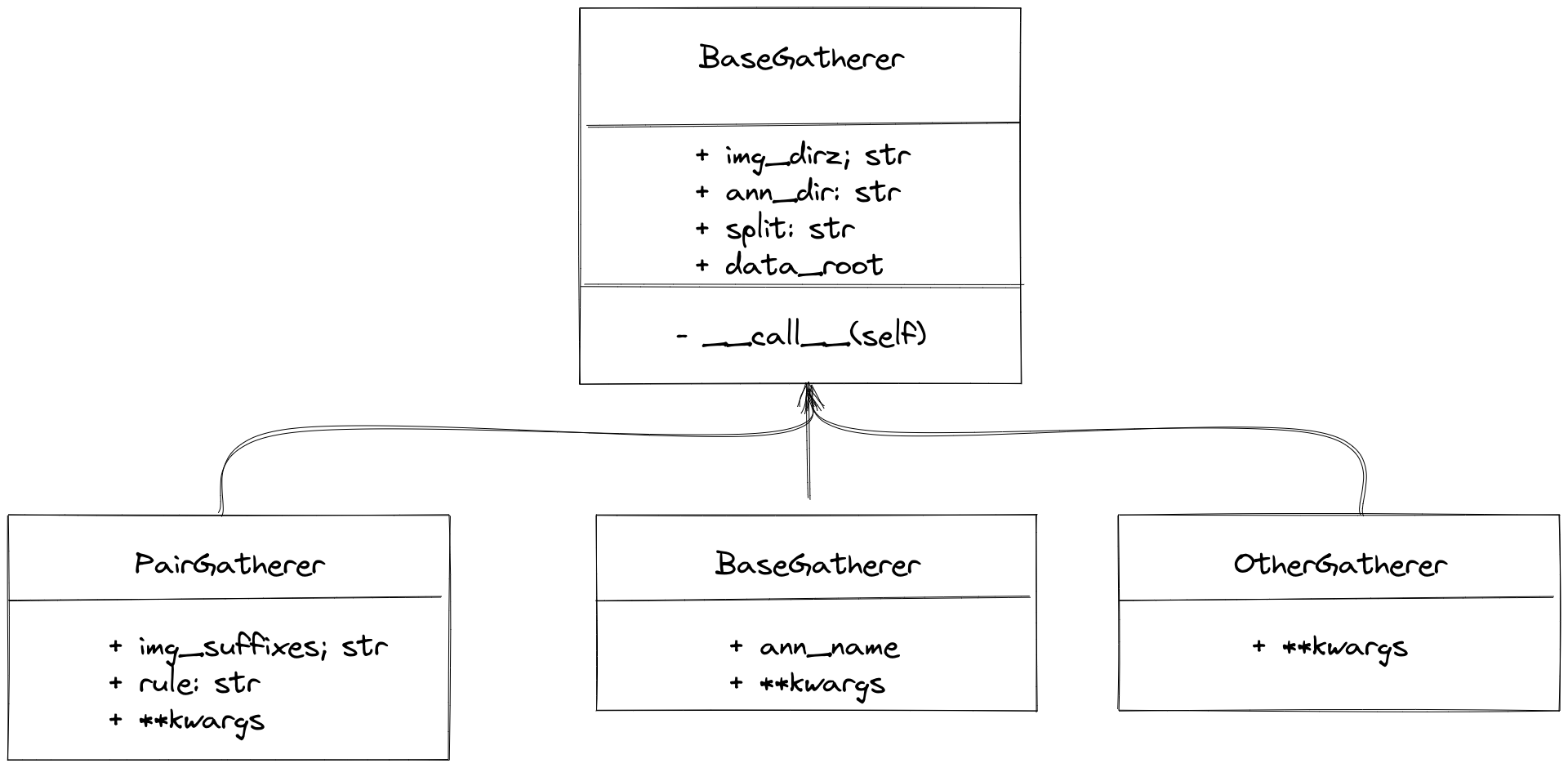
|
||||
|
||||
MMOCR has built-in `PairGatherer` and `MonoGatherer` to handle the two common cases mentioned above. `PairGatherer` is used for many-to-many situations, while `MonoGatherer` is used for one-to-many situations.
|
||||
|
||||
```{note}
|
||||
To simplify processing, the gatherer assumes that the dataset's images and annotations are stored separately in `{taskname}_imgs/{split}/` and `annotations/`, respectively. In particular, for many-to-many situations, the annotation file needs to be placed in `annotations/{split}`.
|
||||
```
|
||||
|
||||
- In the many-to-many case, `PairGatherer` needs to find the image files and corresponding annotation files according to a certain naming convention. First, the suffix of the image needs to be specified by the `img_suffixes` parameter, as in the example above `img_suffixes=[.jpg,.JPG]`. In addition, a pair of [regular expressions](https://docs.python.org/3/library/re.html) `rule` is used to specify the correspondence between the image and annotation files. For example, `rule=[r'img_(\d+)\.([jJ][pP][gG])',r'gt_img_\1.txt']`. The first regular expression is used to match the image file name, `\d+` is used to match the image sequence number, and `([jJ][pP][gG])` is used to match the image suffix. The second regular expression is used to match the annotation file name, where `\1` associates the matched image sequence number with the annotation file sequence number. An example configuration is:
|
||||
|
||||
```python
|
||||
gatherer=dict(
|
||||
type='PairGatherer',
|
||||
img_suffixes=['.jpg', '.JPG'],
|
||||
rule=[r'img_(\d+)\.([jJ][pP][gG])', r'gt_img_\1.txt']),
|
||||
```
|
||||
|
||||
For the case of one-to-many, it is usually simple, and the user only needs to specify the annotation file name. For example, for the training set configuration:
|
||||
|
||||
```python
|
||||
gatherer=dict(type='MonoGatherer', ann_name='train.txt'),
|
||||
```
|
||||
|
||||
MMOCR has also made conventions on the return value of `Gatherer`. `Gatherer` returns a tuple with two elements. The first element is a list of image paths (including all image paths) or the folder containing all images. The second element is a list of annotation file paths (including all annotation file paths) or the path of the annotation file (the annotation file contains all image annotation information). Specifically, the return value of `PairGatherer` is (list of image paths, list of annotation file paths), as shown below:
|
||||
|
||||
```python
|
||||
(['{taskname}_imgs/{split}/img_1.jpg', '{taskname}_imgs/{split}/img_2.jpg', '{taskname}_imgs/{split}/img_3.JPG'],
|
||||
['annotations/{split}/gt_img_1.txt', 'annotations/{split}/gt_img_2.txt', 'annotations/{split}/gt_img_3.txt'])
|
||||
```
|
||||
|
||||
`MonoGatherer` returns a tuple containing the path to the image directory and the path to the annotation file, as follows:
|
||||
|
||||
```python
|
||||
('{taskname}/{split}', 'annotations/gt.txt')
|
||||
```
|
||||
|
||||
#### Dataset parsing (Parser)
|
||||
|
||||
`Parser` is mainly used to parse the original annotation files. Since the original annotation formats vary greatly, MMOCR provides `BaseParser` as a base class, which users can inherit to implement their own `Parser`. In `BaseParser`, MMOCR has designed two interfaces: `parse_files` and `parse_file`, where the annotation parsing is conventionally carried out. For the two different input situations of `Gatherer` (many-to-many, one-to-many), the implementations of these two interfaces should be different.
|
||||
|
||||
- `BaseParser` by default handles the many-to-many situation. Among them, `parse_files` distributes the data in parallel to multiple `parse_file` processes, and each `parse_file` parses the annotation of a single image separately.
|
||||
- For the one-to-many situation, the user needs to override `parse_files` to implement loading the annotation and returning standardized results.
|
||||
|
||||
The interface of `BaseParser` is defined as follows:
|
||||
|
||||
```python
|
||||
class BaseParser:
|
||||
def __call__(self, img_paths, ann_paths):
|
||||
return self.parse_files(img_paths, ann_paths)
|
||||
|
||||
def parse_files(self, img_paths: Union[List[str], str],
|
||||
ann_paths: Union[List[str], str]) -> List[Tuple]:
|
||||
samples = track_parallel_progress_multi_args(
|
||||
self.parse_file, (img_paths, ann_paths), nproc=self.nproc)
|
||||
return samples
|
||||
|
||||
@abstractmethod
|
||||
def parse_file(self, img_path: str, ann_path: str) -> Tuple:
|
||||
|
||||
raise NotImplementedError
|
||||
```
|
||||
|
||||
In order to ensure the uniformity of subsequent modules, MMOCR has made conventions for the return values of `parse_files` and `parse_file`. The return value of `parse_file` is a tuple, the first element of which is the image path, and the second element is the annotation information. The annotation information is a list, each element of which is a dictionary with the fields `poly`, `text`, and `ignore`, as shown below:
|
||||
|
||||
```python
|
||||
# An example of returned values:
|
||||
(
|
||||
'imgs/train/xxx.jpg',
|
||||
[
|
||||
dict(
|
||||
url='https://rrc.cvc.uab.es/downloads/ch4_training_images.zip',
|
||||
save_name='ic15_textdet_train_img.zip',
|
||||
md5='c51cbace155dcc4d98c8dd19d378f30d',
|
||||
split=['train'],
|
||||
content=['image'],
|
||||
mapping=[['ic15_textdet_train_img', 'imgs/train']]),
|
||||
dict(
|
||||
url='https://rrc.cvc.uab.es/downloads/ch4_test_images.zip',
|
||||
save_name='ic15_textdet_test_img.zip',
|
||||
md5='97e4c1ddcf074ffcc75feff2b63c35dd',
|
||||
split=['test'],
|
||||
content=['image'],
|
||||
mapping=[['ic15_textdet_test_img', 'imgs/test']]),
|
||||
poly=[0, 1, 1, 1, 1, 0, 0, 0],
|
||||
text='hello',
|
||||
ignore=False),
|
||||
...
|
||||
]
|
||||
)
|
||||
```
|
||||
|
||||
The output of `parse_files` is a list, and each element in the list is the return value of `parse_file`. An example is:
|
||||
|
||||
```python
|
||||
[
|
||||
(
|
||||
'imgs/train/xxx.jpg',
|
||||
[
|
||||
dict(
|
||||
poly=[0, 1, 1, 1, 1, 0, 0, 0],
|
||||
text='hello',
|
||||
ignore=False),
|
||||
...
|
||||
]
|
||||
),
|
||||
...
|
||||
]
|
||||
```
|
||||
|
||||
#### Dataset Conversion (Packer)
|
||||
|
||||
`Packer` is mainly used to convert data into a unified annotation format, because the input data is the output of parsers and the format has been fixed. Therefore, the packer only needs to convert the input format into a unified annotation format for each task. Currently, MMOCR supports tasks such as text detection, text recognition, end-to-end OCR, and key information extraction, and MMOCR has a corresponding packer for each task, as shown below:
|
||||
|
||||
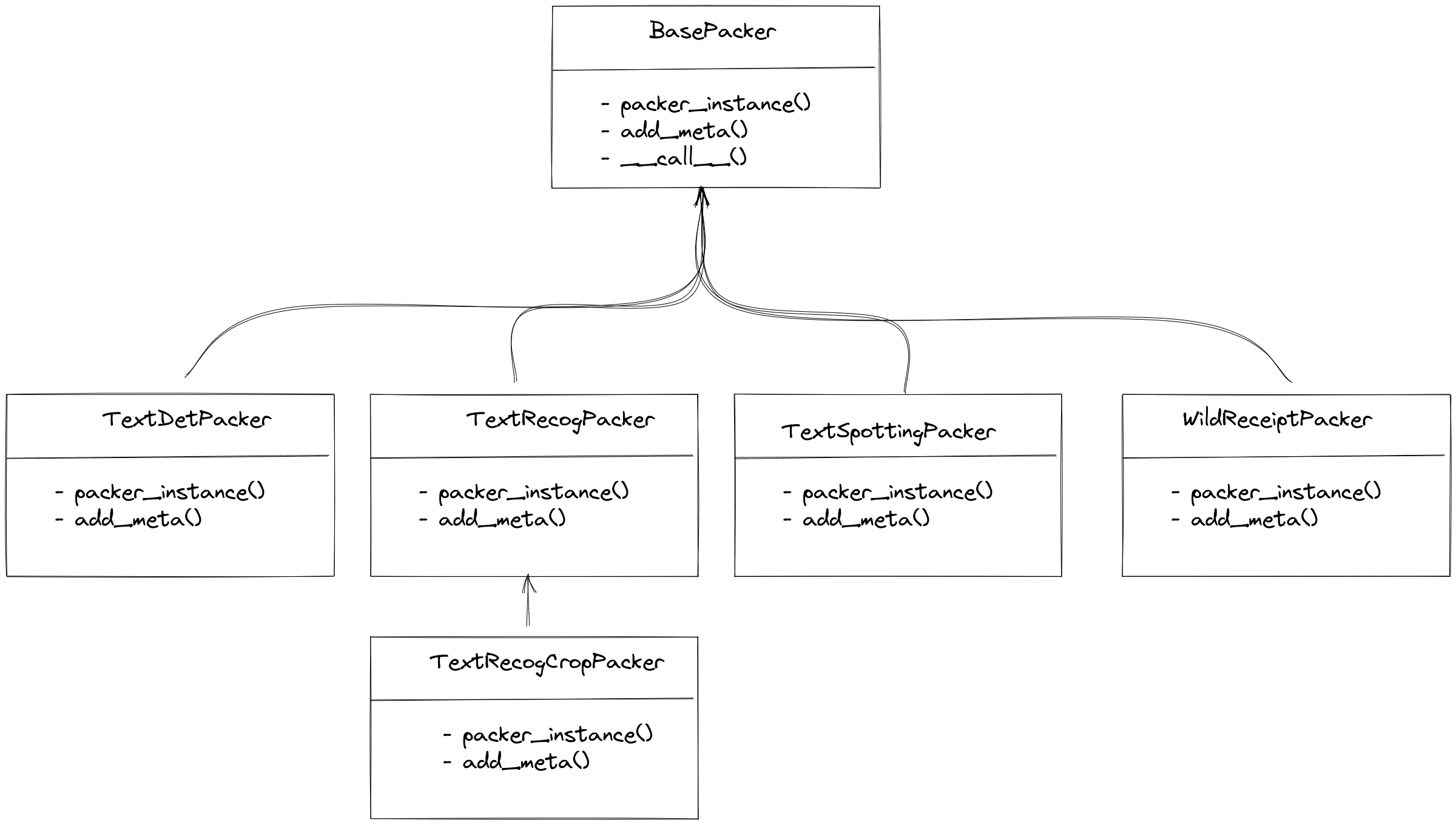
|
||||
|
||||
For text detection, end-to-end OCR, and key information extraction, MMOCR has a unique corresponding `Packer`. However, for text recognition, MMOCR provides two `Packer` options: `TextRecogPacker` and `TextRecogCropPacker`, due to the existence of two types of datasets:
|
||||
|
||||
- Each image is a recognition sample, and the annotation information returned by the `parser` is only a `dict(text='xxx')`. In this case, `TextRecogPacker` can be used.
|
||||
- The dataset does not crop text from the image, and it essentially contains end-to-end OCR annotations that include the position information of the text and the corresponding text information. `TextRecogCropPacker` will crop the text from the image and then convert it into the unified format for text recognition.
|
||||
|
||||
#### Annotation Saving (Dumper)
|
||||
|
||||
The `dumper` module is used to determine what format the data should be saved in. Currently, MMOCR supports `JsonDumper`, `WildreceiptOpensetDumper`, and `TextRecogLMDBDumper`. They are used to save data in the standard MMOCR JSON format, the Wildreceipt format, and the LMDB format commonly used in the academic community for text recognition, respectively.
|
||||
|
||||
#### Delete files (Delete)
|
||||
|
||||
When processing a dataset, temporary files that are not needed may be generated. Here, a list of such files or folders can be passed in, which will be deleted when the conversion is finished.
|
||||
|
||||
#### Generate the configuration file for the dataset (ConfigGenerator)
|
||||
|
||||
In order to automatically generate basic configuration files after preparing the dataset, MMOCR has implemented `TextDetConfigGenerator`, `TextRecogConfigGenerator`, and `TextSpottingConfigGenerator` for each task. The main parameters supported by these generators are as follows:
|
||||
|
||||
| Field Name | Meaning |
|
||||
| ----------- | --------------------------------------------------------------------------------------------------------------------------------------------------------------------------------------------- |
|
||||
| data_root | Root directory where the dataset is stored. |
|
||||
| train_anns | Path to the training set annotations in the configuration file. If not specified, it defaults to `[dict(ann_file='{taskname}_train.json', dataset_postfix='']`. |
|
||||
| val_anns | Path to the validation set annotations in the configuration file. If not specified, it defaults to an empty string. |
|
||||
| test_anns | Path to the test set annotations in the configuration file. If not specified, it defaults to `[dict(ann_file='{taskname}_test.json', dataset_postfix='']`. |
|
||||
| config_path | Path to the directory where the configuration files for the algorithm are stored. The configuration generator will write the default configuration to `{config_path}/{taskname}/_base_/datasets/{dataset_name}.py`. If not specified, it defaults to `configs/`. |
|
||||
|
||||
After preparing all the files for the dataset, the configuration generator will automatically generate the basic configuration files required to call the dataset. Below is a minimal example of a `TextDetConfigGenerator` configuration:
|
||||
|
||||
```python
|
||||
config_generator = dict(type='TextDetConfigGenerator')
|
||||
```
|
||||
|
||||
The generated file will be placed by default under `configs/{task}/_base_/datasets/`. In this example, the basic configuration file for the ICDAR 2015 dataset will be generated at `configs/textdet/_base_/datasets/icdar2015.py`.
|
||||
|
||||
```python
|
||||
icdar2015_textdet_data_root = 'data/icdar2015'
|
||||
|
||||
icdar2015_textdet_train = dict(
|
||||
type='OCRDataset',
|
||||
data_root=icdar2015_textdet_data_root,
|
||||
ann_file='textdet_train.json',
|
||||
filter_cfg=dict(filter_empty_gt=True, min_size=32),
|
||||
pipeline=None)
|
||||
|
||||
icdar2015_textdet_test = dict(
|
||||
type='OCRDataset',
|
||||
data_root=icdar2015_textdet_data_root,
|
||||
ann_file='textdet_test.json',
|
||||
test_mode=True,
|
||||
pipeline=None)
|
||||
```
|
||||
|
||||
If the dataset is special and there are several variants of the annotations, the configuration generator also supports generating variables pointing to each variant in the base configuration. However, this requires users to differentiate them by using different `dataset_postfix` when setting up. For example, the ICDAR 2015 text recognition dataset has two annotation versions for the test set, the original version and the 1811 version, which can be specified in `test_anns` as follows:
|
||||
|
||||
```python
|
||||
config_generator = dict(
|
||||
type='TextRecogConfigGenerator',
|
||||
test_anns=[
|
||||
dict(ann_file='textrecog_test.json'),
|
||||
dict(dataset_postfix='857', ann_file='textrecog_test_857.json')
|
||||
])
|
||||
```
|
||||
|
||||
The default type of `data_obtainer` is `NaiveDataObtainer`, which mainly downloads and decompresses the original files to the specified directory. Here, we configure the URL, save name, MD5 value, etc. of the original dataset files through the `files` parameter. The `mapping` parameter is used to specify the path where the data is decompressed or moved. In addition, the two optional parameters `split` and `content` respectively indicate the content type stored in the compressed file and the corresponding dataset.
|
||||
The configuration generator will generate the following configurations:
|
||||
|
||||
```python
|
||||
data_converter = dict(
|
||||
type='TextDetDataConverter',
|
||||
splits=['train', 'test'],
|
||||
data_root=data_root,
|
||||
gatherer=dict(
|
||||
type='pair_gather',
|
||||
suffixes=['.jpg', '.JPG'],
|
||||
rule=[r'img_(\d+)\.([jJ][pP][gG])', r'gt_img_\1.txt']),
|
||||
parser=dict(type='ICDARTxtTextDetAnnParser'),
|
||||
icdar2015_textrecog_data_root = 'data/icdar2015'
|
||||
|
||||
icdar2015_textrecog_train = dict(
|
||||
type='OCRDataset',
|
||||
data_root=icdar2015_textrecog_data_root,
|
||||
ann_file='textrecog_train.json',
|
||||
pipeline=None)
|
||||
|
||||
icdar2015_textrecog_test = dict(
|
||||
type='OCRDataset',
|
||||
data_root=icdar2015_textrecog_data_root,
|
||||
ann_file='textrecog_test.json',
|
||||
test_mode=True,
|
||||
pipeline=None)
|
||||
|
||||
icdar2015_1811_textrecog_test = dict(
|
||||
type='OCRDataset',
|
||||
data_root=icdar2015_textrecog_data_root,
|
||||
ann_file='textrecog_test_1811.json',
|
||||
test_mode=True,
|
||||
pipeline=None)
|
||||
```
|
||||
|
||||
With this file, MMOCR can directly import this dataset into the `dataloader` from the model configuration file (the following sample is excerpted from [`configs/textdet/dbnet/dbnet_resnet18_fpnc_1200e_icdar2015.py`](/configs/textdet/dbnet/dbnet_resnet18_fpnc_1200e_icdar2015.py)):
|
||||
|
||||
```python
|
||||
_base_ = [
|
||||
'../_base_/datasets/icdar2015.py',
|
||||
# ...
|
||||
]
|
||||
|
||||
# dataset settings
|
||||
icdar2015_textdet_train = _base_.icdar2015_textdet_train
|
||||
icdar2015_textdet_test = _base_.icdar2015_textdet_test
|
||||
# ...
|
||||
|
||||
train_dataloader = dict(
|
||||
dataset=icdar2015_textdet_train)
|
||||
|
||||
val_dataloader = dict(
|
||||
dataset=icdar2015_textdet_test)
|
||||
|
||||
test_dataloader = val_dataloader
|
||||
```
|
||||
|
||||
```{note}
|
||||
By default, the configuration generator does not overwrite existing base configuration files unless the user manually specifies `overwrite-cfg` when running the script.
|
||||
```
|
||||
|
||||
## Adding a new dataset to Dataset Preparer
|
||||
|
||||
### Adding Public Datasets
|
||||
|
||||
MMOCR has already supported many [commonly used public datasets](./datasetzoo.md). If the dataset you want to use has not been supported yet and you are willing to [contribute to the MMOCR](../../notes/contribution_guide.md) open-source community, you can follow the steps below to add a new dataset.
|
||||
|
||||
In the following example, we will show you how to add the **ICDAR2013** dataset step by step.
|
||||
|
||||
#### Adding `metafile.yml`
|
||||
|
||||
First, make sure that the dataset you want to add does not already exist in `dataset_zoo/`. Then, create a new folder named after the dataset you want to add, such as `icdar2013/` (usually, use lowercase alphanumeric characters without symbols to name the dataset). In the `icdar2013/` folder, create a `metafile.yml` file and fill in the basic information of the dataset according to the following template:
|
||||
|
||||
```yaml
|
||||
Name: 'Incidental Scene Text IC13'
|
||||
Paper:
|
||||
Title: ICDAR 2013 Robust Reading Competition
|
||||
URL: https://www.imlab.jp/publication_data/1352/icdar_competition_report.pdf
|
||||
Venue: ICDAR
|
||||
Year: '2013'
|
||||
BibTeX: '@inproceedings{karatzas2013icdar,
|
||||
title={ICDAR 2013 robust reading competition},
|
||||
author={Karatzas, Dimosthenis and Shafait, Faisal and Uchida, Seiichi and Iwamura, Masakazu and i Bigorda, Lluis Gomez and Mestre, Sergi Robles and Mas, Joan and Mota, David Fernandez and Almazan, Jon Almazan and De Las Heras, Lluis Pere},
|
||||
booktitle={2013 12th international conference on document analysis and recognition},
|
||||
pages={1484--1493},
|
||||
year={2013},
|
||||
organization={IEEE}}'
|
||||
Data:
|
||||
Website: https://rrc.cvc.uab.es/?ch=2
|
||||
Language:
|
||||
- English
|
||||
Scene:
|
||||
- Natural Scene
|
||||
Granularity:
|
||||
- Word
|
||||
Tasks:
|
||||
- textdet
|
||||
- textrecog
|
||||
- textspotting
|
||||
License:
|
||||
Type: N/A
|
||||
Link: N/A
|
||||
Format: .txt
|
||||
Keywords:
|
||||
- Horizontal
|
||||
```
|
||||
|
||||
#### Add Annotation Examples
|
||||
|
||||
Finally, you can add an annotation example file `sample_anno.md` under the `dataset_zoo/icdar2013/` directory to help the documentation script add annotation examples when generating documentation. The annotation example file is a Markdown file that typically contains the raw data format of a single sample. For example, the following code block shows a sample data file for the ICDAR2013 dataset:
|
||||
|
||||
````markdown
|
||||
**Text Detection**
|
||||
|
||||
```text
|
||||
# train split
|
||||
# x1 y1 x2 y2 "transcript"
|
||||
|
||||
158 128 411 181 "Footpath"
|
||||
443 128 501 169 "To"
|
||||
64 200 363 243 "Colchester"
|
||||
|
||||
# test split
|
||||
# x1, y1, x2, y2, "transcript"
|
||||
|
||||
38, 43, 920, 215, "Tiredness"
|
||||
275, 264, 665, 450, "kills"
|
||||
0, 699, 77, 830, "A"
|
||||
````
|
||||
|
||||
#### Add configuration files for corresponding tasks
|
||||
|
||||
In the `dataset_zoo/icdar2013` directory, add a `.py` configuration file named after the task. For example, `textdet.py`, `textrecog.py`, `textspotting.py`, `kie.py`, etc. The configuration template is shown below:
|
||||
|
||||
```python
|
||||
data_root = ''
|
||||
data_cache = 'data/cache'
|
||||
train_prepare = dict(
|
||||
obtainer=dict(
|
||||
type='NaiveObtainer',
|
||||
data_cache=data_cache,
|
||||
files=[
|
||||
dict(
|
||||
url='xx',
|
||||
md5='',
|
||||
save_name='xxx',
|
||||
mapping=list())
|
||||
]),
|
||||
gatherer=dict(type='xxxGatherer', **kwargs),
|
||||
parser=dict(type='xxxParser', **kwargs),
|
||||
packer=dict(type='TextxxxPacker'), # Packer for the task
|
||||
dumper=dict(type='JsonDumper'),
|
||||
delete=['annotations', 'ic15_textdet_test_img', 'ic15_textdet_train_img'])
|
||||
)
|
||||
test_prepare = dict(
|
||||
obtainer=dict(
|
||||
type='NaiveObtainer',
|
||||
data_cache=data_cache,
|
||||
files=[
|
||||
dict(
|
||||
url='xx',
|
||||
md5='',
|
||||
save_name='xxx',
|
||||
mapping=list())
|
||||
]),
|
||||
gatherer=dict(type='xxxGatherer', **kwargs),
|
||||
parser=dict(type='xxxParser', **kwargs),
|
||||
packer=dict(type='TextxxxPacker'), # Packer for the task
|
||||
dumper=dict(type='JsonDumper'),
|
||||
)
|
||||
```
|
||||
|
||||
```{warning}
|
||||
This section is outdated and not yet synchronized with its Chinese version, please switch the language for the latest information.
|
||||
```
|
||||
Taking the file detection task as an example, let's introduce the specific content of the configuration file. In general, users do not need to implement new `obtainer`, `gatherer`, `packer`, or `dumper`, but usually need to implement a new `parser` according to the annotation format of the dataset.
|
||||
|
||||
`data_converter` is responsible for loading and converting the original to the format supported by MMOCR. We provide a number of built-in data converters for different tasks, such as `TextDetDataConverter`, `TextRecogDataConverter`, `TextSpottingDataConverter`, and `WildReceiptConverter` (Since we only support WildReceipt dataset for KIE task at present, we only provide this converter for now).
|
||||
Regarding the configuration of `obtainer`, we will not go into detail here, and you can refer to [Data set download, extraction, and movement (Obtainer)](#Dataset-download-extraction-and-movement-obtainer).
|
||||
|
||||
Take the text detection task as an example, `TextDetDataConverter` mainly completes the following work:
|
||||
|
||||
- Collect and match the images and original annotation files, such as the image `img_1.jpg` and the annotation `gt_img_1.txt`
|
||||
- Load and parse the original annotations to obtain necessary information such as the bounding box and text
|
||||
- Convert the parsed data to the format supported by MMOCR
|
||||
- Dump the converted data to the specified path and format
|
||||
|
||||
The above steps can be configured separately through `gatherer`, `parser`, `dumper`.
|
||||
|
||||
Specifically, the `gatherer` is used to collect and match the images and annotations in the original dataset. Typically, there are two relations between images and annotations, one is many-to-many, the other is many-to-one.
|
||||
For the `gatherer`, by observing the obtained ICDAR2013 dataset files, we found that each image has a corresponding `.txt` format annotation file:
|
||||
|
||||
```text
|
||||
many-to-many
|
||||
├── img_1.jpg
|
||||
├── gt_img_1.txt
|
||||
├── img_2.jpg
|
||||
├── gt_img_2.txt
|
||||
├── img_3.JPG
|
||||
├── gt_img_3.txt
|
||||
|
||||
one-to-many
|
||||
├── img_1.jpg
|
||||
├── img_2.jpg
|
||||
├── img_3.JPG
|
||||
├── gt.txt
|
||||
data_root
|
||||
├── textdet_imgs/train/
|
||||
│ ├── img_1.jpg
|
||||
│ ├── img_2.jpg
|
||||
│ └── ...
|
||||
├── annotations/train/
|
||||
│ ├── gt_img_1.txt
|
||||
│ ├── gt_img_2.txt
|
||||
│ └── ...
|
||||
```
|
||||
|
||||
Therefore, we provide two built-in gatherers, `pair_gather` and `mono_gather`, to handle the two cases. `pair_gather` is used for the case of many-to-many, and `mono_gather` is used for the case of one-to-many. `pair_gather` needs to specify the `suffixes` parameter to indicate the suffix of the image, such as `suffixes=[.jpg,.JPG]` in the above example. In addition, we need to specify the corresponding relationship between the image and the annotation file through the regular expression, such as `rule=[r'img_(\d+)\.([jJ][pP][gG])',r'gt_img_\1.txt']` in the above example. Where `\d+` is used to match the serial number of the image, `([jJ][pP][gG])` is used to match the suffix of the image, and `\_1` matches the serial number of the image and the serial number of the annotation file.
|
||||
Moreover, the name of each annotation file corresponds to the image: `gt_img_1.txt` corresponds to `img_1.jpg`, and so on. Therefore, `PairGatherer` can be used to match them.
|
||||
|
||||
When the image and annotation file are matched, the original annotations will be parsed. Since the annotation format is usually varied from dataset to dataset, the parsers are usually dataset related. Then, the parser will pack the required data into the MMOCR format.
|
||||
```python
|
||||
gatherer=dict(
|
||||
type='PairGatherer',
|
||||
img_suffixes=['.jpg'],
|
||||
rule=[r'(\w+)\.jpg', r'gt_\1.txt'])
|
||||
```
|
||||
|
||||
Finally, we can specify the dumpers to decide the data format. Currently, we support `JsonDumper`, `WildreceiptOpensetDumper`, and `TextRecogLMDBDumper`. They are used to save the data in the standard MMOCR Json format, Wildreceipt format, and the LMDB format commonly used in academia in the field of text recognition, respectively.
|
||||
The first regular expression in the rule is used to match the image file name, and the second regular expression is used to match the annotation file name. Here, `(\w+)` is used to match the image file name, and `gt_\1.txt` is used to match the annotation file name, where `\1` represents the content matched by the first regular expression. That is, it replaces `img_xx.jpg` with `gt_img_xx.txt`.
|
||||
|
||||
Next, you need to implement a `parser` to parse the original annotation files into a standard format. Usually, before adding a new dataset, users can browse the [details page](./datasetzoo.md) of the supported datasets and check if there is a dataset with the same format. If there is, you can use the parser of that dataset directly. Otherwise, you need to implement a new format parser.
|
||||
|
||||
Data format parsers are stored in the `mmocr/datasets/preparers/parsers` directory. All parsers need to inherit from `BaseParser` and implement the `parse_file` or `parse_files` method. For more information, please refer to [Parsing original annotations (Parser)](#dataset-parsing-parser).
|
||||
|
||||
By observing the annotation files of the ICDAR2013 dataset:
|
||||
|
||||
```text
|
||||
158 128 411 181 "Footpath"
|
||||
443 128 501 169 "To"
|
||||
64 200 363 243 "Colchester"
|
||||
542, 710, 938, 841, "break"
|
||||
87, 884, 457, 1021, "could"
|
||||
517, 919, 831, 1024, "save"
|
||||
```
|
||||
|
||||
We found that the built-in `ICDARTxtTextDetAnnParser` already meets the requirements, so we can directly use this parser and configure it in the `preparer`.
|
||||
|
||||
```python
|
||||
parser=dict(
|
||||
type='ICDARTxtTextDetAnnParser',
|
||||
remove_strs=[',', '"'],
|
||||
encoding='utf-8',
|
||||
format='x1 y1 x2 y2 trans',
|
||||
separator=' ',
|
||||
mode='xyxy')
|
||||
```
|
||||
|
||||
In the configuration for the `ICDARTxtTextDetAnnParser`, `remove_strs=[',', '"']` is specified to remove extra quotes and commas in the annotation files. In the `format` section, `x1 y1 x2 y2 trans` indicates that each line in the annotation file contains four coordinates and a text content separated by spaces (`separator`=' '). Also, `mode` is set to `xyxy`, which means that the coordinates in the annotation file are the coordinates of the top-left and bottom-right corners, so that `ICDARTxtTextDetAnnParser` can parse the annotations into a unified format.
|
||||
|
||||
For the `packer`, taking the file detection task as an example, its `packer` is `TextDetPacker`, and its configuration is as follows:
|
||||
|
||||
```python
|
||||
packer=dict(type='TextDetPacker')
|
||||
```
|
||||
|
||||
Finally, specify the `dumper`, which is generally saved in json format. Its configuration is as follows:
|
||||
|
||||
```python
|
||||
dumper=dict(type='JsonDumper')
|
||||
```
|
||||
|
||||
After the above configuration, the configuration file for the ICDAR2013 training set is as follows:
|
||||
|
||||
```python
|
||||
train_preparer = dict(
|
||||
obtainer=dict(
|
||||
type='NaiveDataObtainer',
|
||||
cache_path=cache_path,
|
||||
files=[
|
||||
dict(
|
||||
url='https://rrc.cvc.uab.es/downloads/'
|
||||
'Challenge2_Training_Task12_Images.zip',
|
||||
save_name='ic13_textdet_train_img.zip',
|
||||
md5='a443b9649fda4229c9bc52751bad08fb',
|
||||
content=['image'],
|
||||
mapping=[['ic13_textdet_train_img', 'textdet_imgs/train']]),
|
||||
dict(
|
||||
url='https://rrc.cvc.uab.es/downloads/'
|
||||
'Challenge2_Training_Task1_GT.zip',
|
||||
save_name='ic13_textdet_train_gt.zip',
|
||||
md5='f3a425284a66cd67f455d389c972cce4',
|
||||
content=['annotation'],
|
||||
mapping=[['ic13_textdet_train_gt', 'annotations/train']]),
|
||||
]),
|
||||
gatherer=dict(
|
||||
type='PairGatherer',
|
||||
img_suffixes=['.jpg'],
|
||||
rule=[r'(\w+)\.jpg', r'gt_\1.txt']),
|
||||
parser=dict(
|
||||
type='ICDARTxtTextDetAnnParser',
|
||||
remove_strs=[',', '"'],
|
||||
format='x1 y1 x2 y2 trans',
|
||||
separator=' ',
|
||||
mode='xyxy'),
|
||||
packer=dict(type='TextDetPacker'),
|
||||
dumper=dict(type='JsonDumper'),
|
||||
)
|
||||
```
|
||||
|
||||
To automatically generate the basic configuration after the dataset is prepared, you also need to configure the corresponding task's `config_generator`.
|
||||
|
||||
In this example, since it is a text detection task, you only need to set the generator to `TextDetConfigGenerator`.
|
||||
|
||||
```python
|
||||
config_generator = dict(type='TextDetConfigGenerator')
|
||||
```
|
||||
|
||||
### Use DataPreparer to prepare customized dataset
|
||||
|
||||
|
|
|
@ -1,10 +1,10 @@
|
|||
# Useful Tools
|
||||
|
||||
## Analysis Tools
|
||||
## Visualization Tools
|
||||
|
||||
### Dataset Visualization Tool
|
||||
|
||||
MMOCR provides a dataset visualization tool `tools/analysis_tools/browse_datasets.py` to help users troubleshoot possible dataset-related problems. You just need to specify the path to the training config (usually stored in `configs/textdet/dbnet/xxx.py`) or the dataset config (usually stored in `configs/textdet/_base_/datasets/xxx.py`), and the tool will automatically plots the transformed (or original) images and labels.
|
||||
MMOCR provides a dataset visualization tool `tools/visualizations/browse_datasets.py` to help users troubleshoot possible dataset-related problems. You just need to specify the path to the training config (usually stored in `configs/textdet/dbnet/xxx.py`) or the dataset config (usually stored in `configs/textdet/_base_/datasets/xxx.py`), and the tool will automatically plots the transformed (or original) images and labels.
|
||||
|
||||
#### Usage
|
||||
|
||||
|
@ -25,11 +25,11 @@ python tools/visualizations/browse_dataset.py \
|
|||
| config | str | (required) Path to the config. |
|
||||
| -o, --output-dir | str | If GUI is not available, specifying an output path to save the visualization results. |
|
||||
| -p, --phase | str | Phase of dataset to visualize. Use "train", "test" or "val" if you just want to visualize the default split. It's also possible to be a dataset variable name, which might be useful when a dataset split has multiple variants in the config. |
|
||||
| -m, --mode | `original`, `transformed`, `pipeline` | Display mode: display original pictures or transformed pictures or comparison pictures. `original` only visualizes the original dataset & annotations; `transformed` shows the resulting images processed through all the transforms; `pipeline` shows all the intermediate images. Defaults to "transformed". |
|
||||
| -m, --mode | `original`, `transformed`, `pipeline` | Display mode: display original pictures or transformed pictures or comparison pictures.`original` only visualizes the original dataset & annotations; `transformed` shows the resulting images processed through all the transforms; `pipeline` shows all the intermediate images. Defaults to "transformed". |
|
||||
| -t, --task | `auto`, `textdet`, `textrecog` | Specify the task type of the dataset. If `auto`, the task type will be inferred from the config. If the script is unable to infer the task type, you need to specify it manually. Defaults to `auto`. |
|
||||
| -n, --show-number | int | The number of samples to visualized. If not specified, display all images in the dataset. |
|
||||
| -i, --show-interval | float | Interval of visualization (s), defaults to 2. |
|
||||
| --cfg-options | float | Override configs. [Example](./config.md#command-line-modification) |
|
||||
| --cfg-options | float | Override configs.[Example](./config.md#command-line-modification) |
|
||||
|
||||
#### Examples
|
||||
|
||||
|
@ -37,7 +37,7 @@ The following example demonstrates how to use the tool to visualize the training
|
|||
|
||||
```Bash
|
||||
# Example: Visualizing the training data used by dbnet_r50dcn_v2_fpnc_1200e_icadr2015 model
|
||||
python tools/analysis_tools/browse_dataset.py configs/textdet/dbnet/dbnet_resnet50-dcnv2_fpnc_1200e_icdar2015.py
|
||||
python tools/visualizations/browse_dataset.py configs/textdet/dbnet/dbnet_resnet50-dcnv2_fpnc_1200e_icdar2015.py
|
||||
```
|
||||
|
||||
By default, the visualization mode is "transformed", and you will see the images & annotations being transformed by the pipeline:
|
||||
|
@ -49,7 +49,7 @@ By default, the visualization mode is "transformed", and you will see the images
|
|||
If you just want to visualize the original dataset, simply set the mode to "original":
|
||||
|
||||
```Bash
|
||||
python tools/analysis_tools/browse_dataset.py configs/textdet/dbnet/dbnet_resnet50-dcnv2_fpnc_1200e_icdar2015.py -m original
|
||||
python tools/visualizations/browse_dataset.py configs/textdet/dbnet/dbnet_resnet50-dcnv2_fpnc_1200e_icdar2015.py -m original
|
||||
```
|
||||
|
||||
<div align=center><img src="https://user-images.githubusercontent.com/22607038/206646570-382d0f26-908a-4ab4-b1a7-5cc31fa70c5f.jpg" style=" width: auto; height: 40%; "></div>
|
||||
|
@ -57,7 +57,7 @@ python tools/analysis_tools/browse_dataset.py configs/textdet/dbnet/dbnet_resnet
|
|||
Or, to visualize the entire pipeline:
|
||||
|
||||
```Bash
|
||||
python tools/analysis_tools/browse_dataset.py configs/textdet/dbnet/dbnet_resnet50-dcnv2_fpnc_1200e_icdar2015.py -m pipeline
|
||||
python tools/visualizations/browse_dataset.py configs/textdet/dbnet/dbnet_resnet50-dcnv2_fpnc_1200e_icdar2015.py -m pipeline
|
||||
```
|
||||
|
||||
<div align=center><img src="https://user-images.githubusercontent.com/22607038/206637571-287640c0-1f55-453f-a2fc-9f9734b9593f.jpg" style=" width: auto; height: 40%; "></div>
|
||||
|
@ -65,7 +65,7 @@ python tools/analysis_tools/browse_dataset.py configs/textdet/dbnet/dbnet_resnet
|
|||
In addition, users can also visualize the original images and their corresponding labels of the dataset by specifying the path to the dataset config file, for example:
|
||||
|
||||
```Bash
|
||||
python tools/analysis_tools/browse_dataset.py configs/textrecog/_base_/datasets/icdar2015.py
|
||||
python tools/visualizations/browse_dataset.py configs/textrecog/_base_/datasets/icdar2015.py
|
||||
```
|
||||
|
||||
Some datasets might have multiple variants. For example, the test split of `icdar2015` textrecog dataset has two variants, which the [base dataset config](/configs/textrecog/_base_/datasets/icdar2015.py) defines as follows:
|
||||
|
@ -85,11 +85,58 @@ icdar2015_1811_textrecog_test = dict(
|
|||
In this case, you can specify the variant name to visualize the corresponding dataset:
|
||||
|
||||
```Bash
|
||||
python tools/analysis_tools/browse_dataset.py configs/textrecog/_base_/datasets/icdar2015.py -p icdar2015_1811_textrecog_test
|
||||
python tools/visualizations/browse_dataset.py configs/textrecog/_base_/datasets/icdar2015.py -p icdar2015_1811_textrecog_test
|
||||
```
|
||||
|
||||
Based on this tool, users can easily verify if the annotation of a custom dataset is correct.
|
||||
|
||||
### Hyper-parameter Scheduler Visualization
|
||||
|
||||
This tool aims to help the user to check the hyper-parameter scheduler of the optimizer (without training), which support the "learning rate" or "momentum"
|
||||
|
||||
#### Introduce the scheduler visualization tool
|
||||
|
||||
```bash
|
||||
python tools/visualizations/vis_scheduler.py \
|
||||
${CONFIG_FILE} \
|
||||
[-p, --parameter ${PARAMETER_NAME}] \
|
||||
[-d, --dataset-size ${DATASET_SIZE}] \
|
||||
[-n, --ngpus ${NUM_GPUs}] \
|
||||
[-s, --save-path ${SAVE_PATH}] \
|
||||
[--title ${TITLE}] \
|
||||
[--style ${STYLE}] \
|
||||
[--window-size ${WINDOW_SIZE}] \
|
||||
[--cfg-options]
|
||||
```
|
||||
|
||||
**Description of all arguments**:
|
||||
|
||||
- `config`: The path of a model config file.
|
||||
- **`-p, --parameter`**: The param to visualize its change curve, choose from "lr" and "momentum". Default to use "lr".
|
||||
- **`-d, --dataset-size`**: The size of the datasets. If set,`build_dataset` will be skipped and `${DATASET_SIZE}` will be used as the size. Default to use the function `build_dataset`.
|
||||
- **`-n, --ngpus`**: The number of GPUs used in training, default to be 1.
|
||||
- **`-s, --save-path`**: The learning rate curve plot save path, default not to save.
|
||||
- `--title`: Title of figure. If not set, default to be config file name.
|
||||
- `--style`: Style of plt. If not set, default to be `whitegrid`.
|
||||
- `--window-size`: The shape of the display window. If not specified, it will be set to `12*7`. If used, it must be in the format `'W*H'`.
|
||||
- `--cfg-options`: Modifications to the configuration file, refer to [Learn about Configs](../user_guides/config.md).
|
||||
|
||||
```{note}
|
||||
Loading annotations maybe consume much time, you can directly specify the size of the dataset with `-d, dataset-size` to save time.
|
||||
```
|
||||
|
||||
#### How to plot the learning rate curve without training
|
||||
|
||||
You can use the following command to plot the step learning rate schedule used in the config `configs/textdet/dbnet/dbnet_resnet50-dcnv2_fpnc_1200e_icdar2015.py`:
|
||||
|
||||
```bash
|
||||
python tools/visualizations/vis_scheduler.py configs/textdet/dbnet/dbnet_resnet50-dcnv2_fpnc_1200e_icdar2015.py -d 100
|
||||
```
|
||||
|
||||
<div align=center><img src="https://user-images.githubusercontent.com/43344034/232757392-b29b8e3a-77af-451c-8786-d3b4259ab388.png" style=" width: auto; height: 40%; "></div>
|
||||
|
||||
## Analysis Tools
|
||||
|
||||
### Offline Evaluation Tool
|
||||
|
||||
For saved prediction results, we provide an offline evaluation script `tools/analysis_tools/offline_eval.py`. The following example demonstrates how to use this tool to evaluate the output of the "PSENet" model offline.
|
||||
|
@ -110,11 +157,11 @@ python tools/analysis_tools/offline_eval.py configs/textdet/psenet/psenet_r50_fp
|
|||
|
||||
In addition, based on this tool, users can also convert predictions obtained from other libraries into MMOCR-supported formats, then use MMOCR's built-in metrics to evaluate them.
|
||||
|
||||
| ARGS | Type | Description |
|
||||
| ------------- | ----- | ------------------------------------------------------------------ |
|
||||
| config | str | (required) Path to the config. |
|
||||
| pkl_results | str | (required) The saved predictions. |
|
||||
| --cfg-options | float | Override configs. [Example](./config.md#command-line-modification) |
|
||||
| ARGS | Type | Description |
|
||||
| ------------- | ----- | ----------------------------------------------------------------- |
|
||||
| config | str | (required) Path to the config. |
|
||||
| pkl_results | str | (required) The saved predictions. |
|
||||
| --cfg-options | float | Override configs.[Example](./config.md#command-line-modification) |
|
||||
|
||||
### Calculate FLOPs and the Number of Parameters
|
||||
|
||||
|
|
|
@ -123,24 +123,6 @@ html_theme_options = {
|
|||
},
|
||||
]
|
||||
},
|
||||
{
|
||||
'name':
|
||||
'版本',
|
||||
'children': [
|
||||
{
|
||||
'name': 'MMOCR 0.x',
|
||||
'url': 'https://mmocr.readthedocs.io/zh_CN/latest/',
|
||||
'description': 'main 分支文档'
|
||||
},
|
||||
{
|
||||
'name': 'MMOCR 1.x',
|
||||
'url': 'https://mmocr.readthedocs.io/zh_CN/dev-1.x/',
|
||||
'description': '1.x 分支文档'
|
||||
},
|
||||
],
|
||||
'active':
|
||||
True,
|
||||
},
|
||||
],
|
||||
# Specify the language of shared menu
|
||||
'menu_lang':
|
||||
|
|
|
@ -236,7 +236,8 @@ docker run --gpus all --shm-size=8g -it -v {实际数据目录}:/mmocr/data mmoc
|
|||
|
||||
| MMOCR | MMEngine | MMCV | MMDetection |
|
||||
| -------------- | --------------------------- | -------------------------- | --------------------------- |
|
||||
| dev-1.x | 0.7.1 \<= mmengine \< 1.0.0 | 2.0.0rc4 \<= mmcv \< 2.1.0 | 3.0.0rc5 \<= mmdet \< 3.1.0 |
|
||||
| dev-1.x | 0.7.1 \<= mmengine \< 1.1.0 | 2.0.0rc4 \<= mmcv \< 2.1.0 | 3.0.0rc5 \<= mmdet \< 3.2.0 |
|
||||
| 1.0.1 | 0.7.1 \<= mmengine \< 1.1.0 | 2.0.0rc4 \<= mmcv \< 2.1.0 | 3.0.0rc5 \<= mmdet \< 3.2.0 |
|
||||
| 1.0.0 | 0.7.1 \<= mmengine \< 1.0.0 | 2.0.0rc4 \<= mmcv \< 2.1.0 | 3.0.0rc5 \<= mmdet \< 3.1.0 |
|
||||
| 1.0.0rc6 | 0.6.0 \<= mmengine \< 1.0.0 | 2.0.0rc4 \<= mmcv \< 2.1.0 | 3.0.0rc5 \<= mmdet \< 3.1.0 |
|
||||
| 1.0.0rc\[4-5\] | 0.1.0 \<= mmengine \< 1.0.0 | 2.0.0rc1 \<= mmcv \< 2.1.0 | 3.0.0rc0 \<= mmdet \< 3.1.0 |
|
||||
|
|
|
@ -44,7 +44,7 @@ python tools/dataset_converters/prepare_dataset.py icdar2015 totaltext --task te
|
|||
|
||||
### LMDB 格式
|
||||
|
||||
在文本识别任务中,我们通常使用 LMDB 格式来存储数据,以加快数据的读取速度。在使用 `prepare_dataset.py` 脚本准备数据时,可以通过 `--lmdb` 参数来指定将数据转换为 LMDB 格式。例如:
|
||||
在文本识别任务中,通常使用 LMDB 格式来存储数据,以加快数据的读取速度。在使用 `prepare_dataset.py` 脚本准备数据时,可以通过 `--lmdb` 参数来指定将数据转换为 LMDB 格式。例如:
|
||||
|
||||
```bash
|
||||
python tools/dataset_converters/prepare_dataset.py icdar2015 --task textrecog --lmdb
|
||||
|
@ -52,7 +52,7 @@ python tools/dataset_converters/prepare_dataset.py icdar2015 --task textrecog --
|
|||
|
||||
数据集准备完成后,Dataset Preparer 会在 `configs/textrecog/_base_/datasets/` 中生成 `icdar2015_lmdb.py` 配置。你可以继承该配置,并将 `dataloader` 指向 LMDB 数据集。然而,LMDB 数据集的读取需要配合 [`LoadImageFromNDArray`](mmocr.datasets.transforms.LoadImageFromNDArray),因此你也同样需要修改 `pipeline`。
|
||||
|
||||
例如,我们想要将 `configs/textrecog/crnn/crnn_mini-vgg_5e_mj.py` 的训练集改为刚刚生成的 icdar2015,则需要作如下修改:
|
||||
例如,想要将 `configs/textrecog/crnn/crnn_mini-vgg_5e_mj.py` 的训练集改为刚刚生成的 icdar2015,则需要作如下修改:
|
||||
|
||||
1. 修改 `configs/textrecog/crnn/crnn_mini-vgg_5e_mj.py`:
|
||||
|
||||
|
@ -80,30 +80,30 @@ python tools/dataset_converters/prepare_dataset.py icdar2015 --task textrecog --
|
|||
]
|
||||
```
|
||||
|
||||
### 数据集配置
|
||||
## 设计
|
||||
|
||||
数据集自动化准备脚本使用了模块化的设计,极大地增强了扩展性,用户能够很方便地配置其他公开数据集或私有数据集。数据集自动化准备脚本的配置文件被统一存储在 `dataset_zoo/` 目录下,用户可以在该目录下找到所有已由 MMOCR 官方支持的数据集准备脚本配置文件。该文件夹的目录结构如下:
|
||||
OCR 数据集数量众多,不同的数据集有着不同的语言,不同的标注格式,不同的场景等。 数据集的使用情况一般有两种,一种是快速的了解数据集的相关信息,另一种是在使用数据集训练模型。为了满足这两种使用场景MMOCR 提供数据集自动化准备脚本,数据集自动化准备脚本使用了模块化的设计,极大地增强了扩展性,用户能够很方便地配置其他公开数据集或私有数据集。数据集自动化准备脚本的配置文件被统一存储在 `dataset_zoo/` 目录下,用户可以在该目录下找到所有已由 MMOCR 官方支持的数据集准备脚本配置文件。该文件夹的目录结构如下:
|
||||
|
||||
```text
|
||||
dataset_zoo/
|
||||
├── icdar2015
|
||||
│ ├── metafile.yml
|
||||
│ ├── sample_anno.md
|
||||
│ ├── textdet.py
|
||||
│ ├── textrecog.py
|
||||
│ └── textspotting.py
|
||||
└── wildreceipt
|
||||
├── metafile.yml
|
||||
├── sample_anno.md
|
||||
├── kie.py
|
||||
├── textdet.py
|
||||
├── textrecog.py
|
||||
└── textspotting.py
|
||||
```
|
||||
|
||||
其中,`metafile.yml` 是数据集的元信息文件,其中存放了对应数据集的基本信息,包括发布年份,论文作者,以及版权等其他信息。其它以任务名命名的则是数据集准备脚本的配置文件,用于配置数据集的下载、解压、格式转换等操作。这些配置文件采用了 Python 格式,其使用方法与 MMOCR 算法库的其他配置文件完全一致,详见[配置文件文档](../config.md)。
|
||||
### 数据集相关信息
|
||||
|
||||
#### 数据集元文件
|
||||
|
||||
以数据集 ICDAR2015 为例,`metafile.yml` 中存储了基础的数据集信息:
|
||||
数据集的相关信息包括数据集的标注格式、数据集的标注示例、数据集的基本统计信息等。虽然在每个数据集的官网中都有这些信息,但是这些信息分散在各个数据集的官网中,用户需要花费大量的时间来挖掘数据集的基本信息。因此,MMOCR 设计了一些范式,它可以帮助用户快速了解数据集的基本信息。 MMOCR 将数据集的相关信息分为两个部分,一部分是数据集的基本信息包括包括发布年份,论文作者,以及版权等其他信息,另一部分是数据集的标注信息,包括数据集的标注格式、数据集的标注示例。每一部分 MMOCR 都会提供一个范式,贡献者可以根据范式来填写数据集的基本信息,使用用户就可以快速了解数据集的基本信息。 根据数据集的基本信息 MMOCR 提供了一个 `metafile.yml` 文件,其中存放了对应数据集的基本信息,包括发布年份,论文作者,以及版权等其他信息,这样用户就可以快速了解数据集的基本信息。该文件在数据集准备过程中并不是强制要求的(因此用户在使用添加自己的私有数据集时可以忽略该文件),但为了用户更好地了解各个公开数据集的信息,MMOCR 建议用户在使用数据集准备脚本前阅读对应的元文件信息,以了解该数据集的特征是否符合用户需求。MMOCR 以 ICDAR2015 作为示例, 其示例内容如下所示:
|
||||
|
||||
```yaml
|
||||
Name: 'Incidental Scene Text IC15'
|
||||
|
@ -136,118 +136,184 @@ Data:
|
|||
Link: https://creativecommons.org/licenses/by/4.0/
|
||||
```
|
||||
|
||||
该文件在数据集准备过程中并不是强制要求的(因此用户在使用添加自己的私有数据集时可以忽略该文件),但为了用户更好地了解各个公开数据集的信息,我们建议用户在使用数据集准备脚本前阅读对应的元文件信息,以了解该数据集的特征是否符合用户需求。
|
||||
具体地,MMOCR 在下表中列出每个字段对应的含义:
|
||||
|
||||
```{warning}
|
||||
自 MMOCR 1.0.0rc6 起,接下来的章节可能会与实际实现有所出入。
|
||||
```
|
||||
| 字段名 | 含义 |
|
||||
| :--------------- | :-------------------------------------------------------------------- |
|
||||
| Name | 数据集的名称 |
|
||||
| Paper.Title | 数据集论文的标题 |
|
||||
| Paper.URL | 数据集论文的链接 |
|
||||
| Paper.Venue | 数据集论文发表的会议/期刊名称 |
|
||||
| Paper.Year | 数据集论文发表的年份 |
|
||||
| Paper.BibTeX | 数据集论文的引用的 BibTex |
|
||||
| Data.Website | 数据集的官方网站 |
|
||||
| Data.Language | 数据集支持的语言 |
|
||||
| Data.Scene | 数据集支持的场景,如 `Natural Scene`, `Document`, `Handwritten` 等 |
|
||||
| Data.Granularity | 数据集支持的粒度,如 `Character`, `Word`, `Line` 等 |
|
||||
| Data.Tasks | 数据集支持的任务,如 `textdet`, `textrecog`, `textspotting`, `kie` 等 |
|
||||
| Data.License | 数据集的许可证信息,如果不存在许可证,则使用 `N/A` 填充 |
|
||||
| Data.Format | 数据集标注文件的格式,如 `.txt`, `.xml`, `.json` 等 |
|
||||
| Data.Keywords | 数据集的特性关键词,如 `Horizontal`, `Vertical`, `Curved` 等 |
|
||||
|
||||
#### 数据集准备脚本配置文件
|
||||
对于数据集的标注信息,MMOCR 提供了一个 `sample_anno.md` 文件,用户可以根据范式来填写数据集的标注信息,这样用户就可以快速了解数据集的标注信息。MMOCR 以 ICDAR2015 作为示例, 其示例内容如下所示:
|
||||
|
||||
下面,我们将介绍数据集准备脚本配置文件 `textXXX.py` 的默认字段与使用方法。
|
||||
````markdown
|
||||
**Text Detection**
|
||||
|
||||
我们在配置文件中提供了 `data_root` 与 `cache_path` 两个默认字段,分别用于存放转换后的 MMOCR 格式的数据集文件,以及在数据准备过程中下载的压缩包等临时文件。
|
||||
```text
|
||||
# x1,y1,x2,y2,x3,y3,x4,y4,trans
|
||||
|
||||
377,117,463,117,465,130,378,130,Genaxis Theatre
|
||||
493,115,519,115,519,131,493,131,[06]
|
||||
374,155,409,155,409,170,374,170,###
|
||||
```
|
||||
````
|
||||
|
||||
`sample_anno.md` 中包含数据集针对不同任务的标注信息,包含标注文件的格式(text 对应的是 txt 文件,标注文件的格式也可以在 meta.yml 中找到),标注的示例。
|
||||
|
||||
通过上述两个文件的信息,用户就可以快速了解数据集的基本信息,同时 MMOCR 汇总了所有数据集的基本信息,用户可以在 [Overview](.overview.md) 中查看所有数据集的基本信息。
|
||||
|
||||
### 数据集使用
|
||||
|
||||
经过数十年的发展,OCR 领域涌现出了一系列的相关数据集,这些数据集往往采用风格各异的格式来提供文本的标注文件,使得用户在使用这些数据集时不得不进行格式转换。因此,为了方便用户进行数据集准备,我们设计了 Dataset Preaprer,帮助用户快速将数据集准备为 MMOCR 支持的格式, 详见[数据格式文档](../../basic_concepts/datasets.md)。下图展示了 Dataset Preparer 的典型运行流程。
|
||||
|
||||
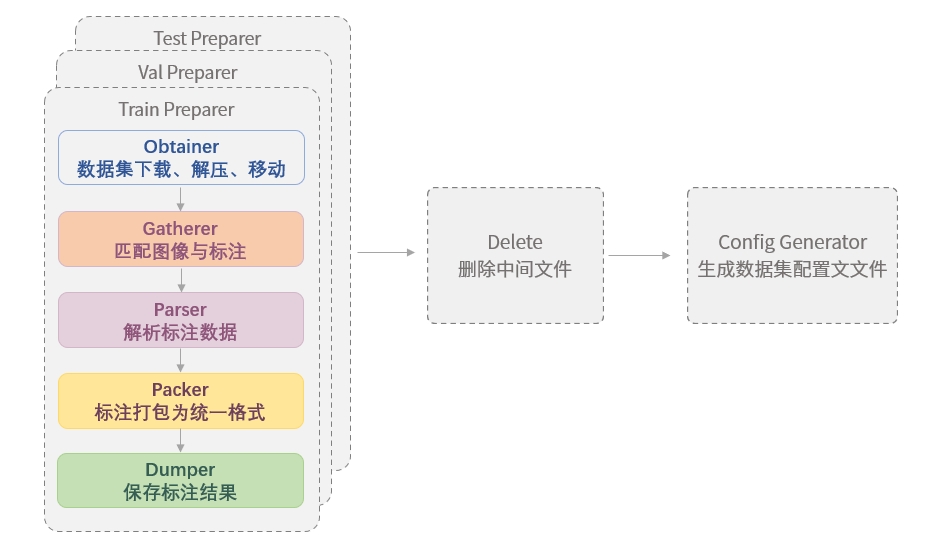
|
||||
|
||||
由图可见,Dataset Preparer 在运行时,会依次执行以下操作:
|
||||
|
||||
1. 对训练集、验证集和测试集,由各 preparer 进行:
|
||||
|
||||
1. [数据集的下载、解压、移动(Obtainer)](#数据集下载解压移动-obtainer)
|
||||
2. [匹配标注与图像(Gatherer)](#数据集收集-gatherer)
|
||||
3. [解析原标注(Parser)](#数据集解析-parser)
|
||||
4. [打包标注为统一格式(Packer)](#数据集转换-packer)
|
||||
5. [保存标注(Dumper)](#标注保存-dumper)
|
||||
|
||||
2. 删除文件(Delete)
|
||||
|
||||
3. 生成数据集的配置文件(Config Generator)
|
||||
|
||||
为了便于应对各种数据集的情况,MMOCR 将每个部分均设计为可插拔的模块,并允许用户通过 dataset_zoo/ 下的配置文件对数据集准备流程进行配置。这些配置文件采用了 Python 格式,其使用方法与 MMOCR 算法库的其他配置文件完全一致,详见[配置文件文档](../config.md)。
|
||||
|
||||
在 `dataset_zoo/` 下,每个数据集均占有一个文件夹,文件夹下会以任务名命名配置文件,以区分不同任务下的配置。以 ICDAR2015 文字检测部分为例,示例配置 `dataset_zoo/icdar2015/textdet.py` 如下所示:
|
||||
|
||||
```python
|
||||
data_root = './data/icdar2015'
|
||||
cache_path = './data/cache'
|
||||
```
|
||||
|
||||
其次,数据集的准备通常包含了“原始数据准备”、“格式转换和保存”及“生成基础配置”这三个主要步骤。因此,我们约定通过 `data_obtainer`、 `data_converter` 和 `config_generator` 参数来配置这三个步骤的行为。
|
||||
|
||||
```{note}
|
||||
如果用户需要跳过某一步骤,则可以省略配置相应参数。例如,如果数据集本身已经遵循 MMOCR 的格式,用户就可以省略掉 `data_converter` 的配置来跳过数据集格式的转换。或者,如果用户不需要自动生成基础配置,可以忽略掉 `config_generator` 的配置。
|
||||
```
|
||||
|
||||
接下来,我们将以 ICDAR2015 数据集的文本检测任务准备配置文件(`dataset_zoo/icdar2015/textdet.py`)为例,逐个模块解析 Dataset Preparer 的运行流程。
|
||||
|
||||
##### 原始数据准备 - `data_obtainer`
|
||||
|
||||
```python
|
||||
data_obtainer = dict(
|
||||
type='NaiveDataObtainer',
|
||||
cache_path=cache_path,
|
||||
data_root=data_root,
|
||||
files=[
|
||||
dict(
|
||||
url='https://rrc.cvc.uab.es/downloads/ch4_training_images.zip',
|
||||
save_name='ic15_textdet_train_img.zip',
|
||||
md5='c51cbace155dcc4d98c8dd19d378f30d',
|
||||
split=['train'],
|
||||
content=['image'],
|
||||
mapping=[['ic15_textdet_train_img', 'textdet_imgs/train']]),
|
||||
dict(
|
||||
url='https://rrc.cvc.uab.es/downloads/'
|
||||
'ch4_training_localization_transcription_gt.zip',
|
||||
save_name='ic15_textdet_train_gt.zip',
|
||||
md5='3bfaf1988960909014f7987d2343060b',
|
||||
split=['train'],
|
||||
content=['annotation'],
|
||||
mapping=[['ic15_textdet_train_gt', 'annotations/train']]),
|
||||
# ...
|
||||
])
|
||||
```
|
||||
|
||||
数据准备器 `data_obtainer` 的类型默认为 `NaiveDataObtainer`,其主要功能是依次下载压缩包并解压到指定目录。在这里,我们通过 `files` 参数来配置下载的压缩包的 URL、保存名称、MD5 值等信息。其中,`mapping` 参数用于指定该压缩包中的数据解压后的存放路径。另外 `split` 和 `content` 这两个可选参数则分别标明了该压缩包中存储的内容类型与其对应的数据集合。
|
||||
|
||||
##### 格式转换和保存 - `data_converter`
|
||||
|
||||
```python
|
||||
data_converter = dict(
|
||||
type='TextDetDataConverter',
|
||||
splits=['train', 'test'],
|
||||
data_root=data_root,
|
||||
data_root = 'data/icdar2015'
|
||||
cache_path = 'data/cache'
|
||||
train_preparer = dict(
|
||||
obtainer=dict(
|
||||
type='NaiveDataObtainer',
|
||||
cache_path=cache_path,
|
||||
files=[
|
||||
dict(
|
||||
url='https://rrc.cvc.uab.es/downloads/ch4_training_images.zip',
|
||||
save_name='ic15_textdet_train_img.zip',
|
||||
md5='c51cbace155dcc4d98c8dd19d378f30d',
|
||||
content=['image'],
|
||||
mapping=[['ic15_textdet_train_img', 'textdet_imgs/train']]),
|
||||
dict(
|
||||
url='https://rrc.cvc.uab.es/downloads/'
|
||||
'ch4_training_localization_transcription_gt.zip',
|
||||
save_name='ic15_textdet_train_gt.zip',
|
||||
md5='3bfaf1988960909014f7987d2343060b',
|
||||
content=['annotation'],
|
||||
mapping=[['ic15_textdet_train_gt', 'annotations/train']]),
|
||||
]),
|
||||
gatherer=dict(
|
||||
type='pair_gather',
|
||||
suffixes=['.jpg', '.JPG'],
|
||||
type='PairGatherer',
|
||||
img_suffixes=['.jpg', '.JPG'],
|
||||
rule=[r'img_(\d+)\.([jJ][pP][gG])', r'gt_img_\1.txt']),
|
||||
parser=dict(type='ICDARTxtTextDetAnnParser'),
|
||||
parser=dict(type='ICDARTxtTextDetAnnParser', encoding='utf-8-sig'),
|
||||
packer=dict(type='TextDetPacker'),
|
||||
dumper=dict(type='JsonDumper'),
|
||||
delete=['annotations', 'ic15_textdet_test_img', 'ic15_textdet_train_img'])
|
||||
)
|
||||
|
||||
test_preparer = dict(
|
||||
obtainer=dict(
|
||||
type='NaiveDataObtainer',
|
||||
cache_path=cache_path,
|
||||
files=[
|
||||
dict(
|
||||
url='https://rrc.cvc.uab.es/downloads/ch4_test_images.zip',
|
||||
save_name='ic15_textdet_test_img.zip',
|
||||
md5='97e4c1ddcf074ffcc75feff2b63c35dd',
|
||||
content=['image'],
|
||||
mapping=[['ic15_textdet_test_img', 'textdet_imgs/test']]),
|
||||
dict(
|
||||
url='https://rrc.cvc.uab.es/downloads/'
|
||||
'Challenge4_Test_Task4_GT.zip',
|
||||
save_name='ic15_textdet_test_gt.zip',
|
||||
md5='8bce173b06d164b98c357b0eb96ef430',
|
||||
content=['annotation'],
|
||||
mapping=[['ic15_textdet_test_gt', 'annotations/test']]),
|
||||
]),
|
||||
gatherer=dict(
|
||||
type='PairGatherer',
|
||||
img_suffixes=['.jpg', '.JPG'],
|
||||
rule=[r'img_(\d+)\.([jJ][pP][gG])', r'gt_img_\1.txt']),
|
||||
parser=dict(type='ICDARTxtTextDetAnnParser', encoding='utf-8-sig'),
|
||||
packer=dict(type='TextDetPacker'),
|
||||
dumper=dict(type='JsonDumper'),
|
||||
)
|
||||
|
||||
delete = ['annotations', 'ic15_textdet_test_img', 'ic15_textdet_train_img']
|
||||
config_generator = dict(type='TextDetConfigGenerator')
|
||||
```
|
||||
|
||||
数据转换器 `data_converter` 主要由转换器及子模块 `gatherer`、`parser` 及 `dumper` 组成,负责完成原始数据的读取与格式转换,并保存为 MMOCR 支持的格式。
|
||||
#### 数据集下载、解压、移动 (Obtainer)
|
||||
|
||||
目前,MMOCR 中支持的数据集转换器类别如下:
|
||||
Dataset Preparer 中,`obtainer` 模块负责了数据集的下载、解压和移动。如今,MMOCR 暂时只提供了 `NaiveDataObtainer`。通常来说,内置的 `NaiveDataObtainer` 即可完成绝大部分可以通过直链访问的数据集的下载,并支持解压、移动文件和重命名等操作。然而,MMOCR 暂时不支持自动下载存储在百度或谷歌网盘等需要登陆才能访问资源的数据集。 这里简要介绍一下 `NaiveDataObtainer`.
|
||||
|
||||
- 文本检测任务数据转换器 `TextDetDataConverter`
|
||||
- 文本识别任务数据转换器
|
||||
- `TextRecogDataConverter`
|
||||
- `TextRecogCropConverter`
|
||||
- 端到端文本检测识别任务转换器 `TextSpottingDataConverter`
|
||||
- 关键信息抽取任务数据转换器 `WildReceiptConverter`
|
||||
| 字段名 | 含义 |
|
||||
| ---------- | ---------------------------------------------------------- |
|
||||
| cache_path | 数据集缓存路径,用于存储数据集准备过程中下载的压缩包等文件 |
|
||||
| data_root | 数据集存储的根目录 |
|
||||
| files | 数据集文件列表,用于描述数据集的下载信息 |
|
||||
|
||||
MMOCR 中目前支持的转换器主要以任务为边界,这是因为不同任务所需的数据格式有细微的差异。
|
||||
比较特别的是,文本识别任务有两个数据转换器,这是因为不同的文本识别数据集提供文字图片的方式有所差别。有的数据集提供了仅包含文字的小图,它们天然适用于文本识别任务,可以直接使用 `TextRecogDataConverter` 处理。而有的数据集提供的是包含了周围场景的大图,因此在准备数据集时,我们需要预先根据标注信息把文字区域裁剪出来,这种情况下则要用到 `TextRecogCropConverter`。
|
||||
`files` 字段是一个列表,列表中的每个元素都是一个字典,用于描述一个数据集文件的下载信息。如下表所示:
|
||||
|
||||
简单介绍下 `TextRecogCropConverter` 数据转换器的使用方法:
|
||||
| 字段名 | 含义 |
|
||||
| ----------------- | -------------------------------------------------------------------- |
|
||||
| url | 数据集文件的下载链接 |
|
||||
| save_name | 数据集文件的保存名称 |
|
||||
| md5 (可选) | 数据集文件的 md5 值,用于校验下载的文件是否完整 |
|
||||
| split (可选) | 数据集文件所属的数据集划分,如 `train`,`test` 等,该字段可以空缺 |
|
||||
| content (可选) | 数据集文件的内容,如 `image`,`annotation` 等,该字段可以空缺 |
|
||||
| mapping (可选) | 数据集文件的解压映射,用于指定解压后的文件存储的位置,该字段可以空缺 |
|
||||
|
||||
- 由于标注文件的解析方式与 TextDet 环节一致,所以仅需继承 `dataset_zoo/xxx/textdet.py` 的 data_converter,并修改type值为 'TextRecogCropConverter',`TextRecogCropConverter` 会在执行 `pack_instance()` 方法时根据解析获得的标注信息完成文字区域的裁剪。
|
||||
- 同时,根据是否存在旋转文字区域标注内置了两种裁剪方式,默认按照水平文本框裁剪。如果存在旋转的文字区域,可以设置 `crop_with_warp=True` 切换为使用 OpenCV warpPerspective 方法进行裁剪。
|
||||
同时,Dataset Preparer 存在以下约定:
|
||||
|
||||
- 不同类型的数据集的图片统一移动到对应类别 `{taskname}_imgs/{split}/`文件夹下,如 `textdet_imgs/train/`。
|
||||
- 对于一个标注文件包含所有图像的标注信息的情况,标注移到到`annotations/{split}.*`文件中。 如 `annotations/train.json`。
|
||||
- 对于一个标注文件包含一个图像的标注信息的情况,所有的标注文件移动到`annotations/{split}/`文件中。 如 `annotations/train/`。
|
||||
- 对于一些其他的特殊情况,比如所有训练、测试、验证的图像都在一个文件夹下,可以将图像移动到自己设定的文件夹下,比如 `{taskname}_imgs/imgs/`,同时要在后续的 `gatherer` 模块中指定图像的存储位置。
|
||||
|
||||
示例配置如下:
|
||||
|
||||
```python
|
||||
_base_ = ['textdet.py']
|
||||
|
||||
data_converter = dict(
|
||||
type='TextRecogCropConverter',
|
||||
crop_with_warp=True)
|
||||
obtainer=dict(
|
||||
type='NaiveDataObtainer',
|
||||
cache_path=cache_path,
|
||||
files=[
|
||||
dict(
|
||||
url='https://rrc.cvc.uab.es/downloads/ch4_training_images.zip',
|
||||
save_name='ic15_textdet_train_img.zip',
|
||||
md5='c51cbace155dcc4d98c8dd19d378f30d',
|
||||
content=['image'],
|
||||
mapping=[['ic15_textdet_train_img', 'textdet_imgs/train']]),
|
||||
dict(
|
||||
url='https://rrc.cvc.uab.es/downloads/'
|
||||
'ch4_training_localization_transcription_gt.zip',
|
||||
save_name='ic15_textdet_train_gt.zip',
|
||||
md5='3bfaf1988960909014f7987d2343060b',
|
||||
content=['annotation'],
|
||||
mapping=[['ic15_textdet_train_gt', 'annotations/train']]),
|
||||
]),
|
||||
```
|
||||
|
||||
接下来,我们将具体解析 `data_converter` 的功能。以文本检测任务为例,`TextDetDataConverter` 与各子模块配合,主要完成以下工作:
|
||||
#### 数据集收集 (Gatherer)
|
||||
|
||||
- `gatherer` 负责收集并匹配原始数据集中的图片与标注文件,如图像 `img_1.jpg` 与标注 `gt_img_1.txt`
|
||||
- `parser` 负责读取原始标注文件,解析出文本框坐标与文本内容等必要信息
|
||||
- 转换器将解析后的数据统一转换至 MMOCR 中当前任务的格式
|
||||
- `dumper` 将转换后的数据保存为指定路径和文件格式
|
||||
- 转换器删除 `delete` 参数指定的临时文件
|
||||
|
||||
以上个步骤我们分别可以通过 `gatherer`,`parser`,`dumper`, `delete` 来进行配置。
|
||||
|
||||
###### `gatherer`
|
||||
|
||||
作为数据转换的第一步,`data_converter` 会通过 `gatherer` 遍历数据集目录下的文件,将图像与标注文件一一对应,并整理出一份文件列表供 `parser` 读取。因此,我们首先需要知道当前数据集下,图片文件与标注文件匹配的规则。
|
||||
|
||||
OCR 数据集通常有两种标注保存形式,一种为多个标注文件对应多张图片,一种则为单个标注文件对应多张图片,如:
|
||||
`gatherer` 遍历数据集目录下的文件,将图像与标注文件一一对应,并整理出一份文件列表供 `parser` 读取。因此,首先需要知道当前数据集下,图片文件与标注文件匹配的规则。OCR 数据集有两种常用标注保存形式,一种为多个标注文件对应多张图片,一种则为单个标注文件对应多张图片,如:
|
||||
|
||||
```text
|
||||
多对多
|
||||
|
@ -265,35 +331,142 @@ OCR 数据集通常有两种标注保存形式,一种为多个标注文件对
|
|||
├── annotations/gt.txt
|
||||
```
|
||||
|
||||
具体设计如下所示
|
||||
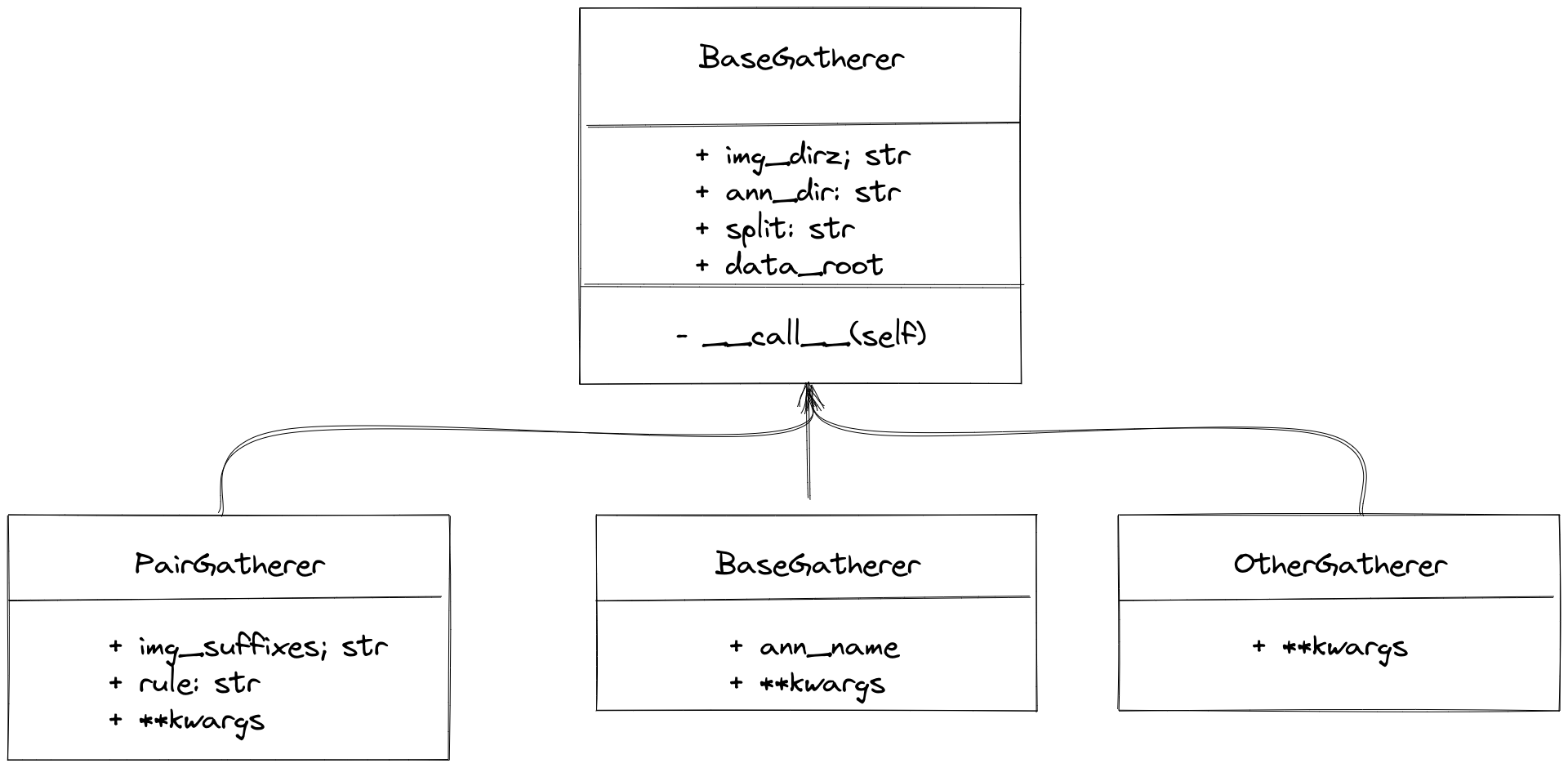
|
||||
|
||||
MMOCR 内置了 `PairGatherer` 与 `MonoGatherer` 来处理以上这两种常用情况。其中 `PairGatherer` 用于多对多的情况,`MonoGatherer` 用于单对多的情况。
|
||||
|
||||
```{note}
|
||||
为了简化处理,gatherer 约定数据集的图片和标注需要分别储存在 `{taskname}_imgs/{split}/` 和 `annotations/` 下。特别地,对于多对多的情况,标注文件需要放置于 `annotations/{split}` 下。如本例中,icdar 2015 训练集的图片就被储存在 `textdet_imgs/train/` 下,而训练的标注则被存在 `annotations/train/` 下。
|
||||
为了简化处理,gatherer 约定数据集的图片和标注需要分别储存在 `{taskname}_imgs/{split}/` 和 `annotations/` 下。特别地,对于多对多的情况,标注文件需要放置于 `annotations/{split}`。
|
||||
```
|
||||
|
||||
因此,我们内置了 `pair_gather` 与 `mono_gather` 来处理以上这两种情况。其中 `pair_gather` 用于多对多的情况,`mono_gather` 用于单对多的情况。
|
||||
- 在多对多的情况下,`PairGatherer` 需要按照一定的命名规则找到图片文件和对应的标注文件。首先,需要通过 `img_suffixes` 参数指定图片的后缀名,如上述例子中的 `img_suffixes=[.jpg,.JPG]`。此外,还需要通过[正则表达式](https://docs.python.org/3/library/re.html) `rule`, 来指定图片与标注文件的对应关系,其中,规则 `rule` 是一个**正则表达式对**,例如 `rule=[r'img_(\d+)\.([jJ][pP][gG])',r'gt_img_\1.txt']`。 第一个正则表达式用于匹配图片文件名,`\d+` 用于匹配图片的序号,`([jJ][pP][gG])` 用于匹配图片的后缀名。 第二个正则表达式用于匹配标注文件名,其中 `\1` 则将匹配到的图片序号与标注文件序号对应起来。示例配置为
|
||||
|
||||
在多对多的情况下,`pair_gather` 需要按照一定的命名规则找到图片文件和对应的标注文件。首先,我们需要通过 `suffixes` 参数指定图片的后缀名,如上述例子中的 `suffixes=[.jpg,.JPG]`。此外,还需要通过正则表达式来指定图片与标注文件的对应关系,如上述例子中的 `rule=[r'img_(\d+)\.([jJ][pP][gG])',r'gt_img_\1.txt']`。其中 `\d+` 用于匹配图片的序号,`([jJ][pP][gG])` 用于匹配图片的后缀名,`\1` 则将匹配到的图片序号与标注文件序号对应起来。
|
||||
```python
|
||||
gatherer=dict(
|
||||
type='PairGatherer',
|
||||
img_suffixes=['.jpg', '.JPG'],
|
||||
rule=[r'img_(\d+)\.([jJ][pP][gG])', r'gt_img_\1.txt']),
|
||||
```
|
||||
|
||||
单对多的情况通常比较简单,用户只需要指定每个 split 对应的标注文件即可。因此 `mono_gather` 预设了 `train_ann`、`val_ann` 和 `test_ann` 参数,供用户直接指定标注文件。
|
||||
- 单对多的情况通常比较简单,用户只需要指定标注文件名即可。对于训练集示例配置为
|
||||
|
||||
###### `parser`
|
||||
```python
|
||||
gatherer=dict(type='MonoGatherer', ann_name='train.txt'),
|
||||
```
|
||||
|
||||
当获取了图像与标注文件的对应关系后,data preparer 将解析原始标注文件。由于不同数据集的标注格式通常有很大的区别,当我们需要支持新的数据集时,**通常需要实现一个新的 `parser` 来解析原始标注文件**。parser 将任务相关的数据解析后打包成 MMOCR 的统一格式。
|
||||
MMOCR 同样对 `Gatherer` 的返回值做了约定,`Gatherer` 会返回两个元素的元组,第一个元素为图像路径列表(包含所有图像路径) 或者所有图像所在的文件夹, 第二个元素为标注文件路径列表(包含所有标注文件路径)或者标注文件的路径(该标注文件包含所有图像标注信息)。
|
||||
具体而言,`PairGatherer` 的返回值为(图像路径列表, 标注文件路径列表),示例如下:
|
||||
|
||||
###### `dumper`
|
||||
```python
|
||||
(['{taskname}_imgs/{split}/img_1.jpg', '{taskname}_imgs/{split}/img_2.jpg', '{taskname}_imgs/{split}/img_3.JPG'],
|
||||
['annotations/{split}/gt_img_1.txt', 'annotations/{split}/gt_img_2.txt', 'annotations/{split}/gt_img_3.txt'])
|
||||
```
|
||||
|
||||
之后,我们可以通过指定不同的 dumper 来决定要将数据保存为何种格式。目前,我们支持 `JsonDumper`, `WildreceiptOpensetDumper`,及 `TextRecogLMDBDumper`。他们分别用于将数据保存为标准的 MMOCR Json 格式、Wildreceipt 格式,及文本识别领域学术界常用的 LMDB 格式。
|
||||
`MonoGatherer` 的返回值为(图像文件夹路径, 标注文件路径), 示例为:
|
||||
|
||||
###### `delete`
|
||||
```python
|
||||
('{taskname}/{split}', 'annotations/gt.txt')
|
||||
```
|
||||
|
||||
#### 数据集解析 (Parser)
|
||||
|
||||
`Parser` 主要用于解析原始的标注文件,因为原始标注情况多种多样,因此 MMOCR 提供了 `BaseParser` 作为基类,用户可以继承该类来实现自己的 `Parser`。在 `BaseParser` 中,MMOCR 设计了两个接口:`parse_files` 和 `parse_file`,约定在其中进行标注的解析。而对于 `Gatherer` 的两种不同输入情况(多对多、单对多),这两个接口的实现则应有所不同。
|
||||
|
||||
- `BaseParser` 默认处理**多对多**的情况。其中,由 `parer_files` 将数据并行分发至多个 `parse_file` 进程,并由每个 `parse_file` 分别进行单个图像标注的解析。
|
||||
- 对于**单对多**的情况,用户则需要重写 `parse_files`,以实现加载标注,并返回规范的结果。
|
||||
|
||||
`BaseParser` 的接口定义如下所示:
|
||||
|
||||
```python
|
||||
class BaseParser:
|
||||
|
||||
def __call__(self, img_paths, ann_paths):
|
||||
return self.parse_files(img_paths, ann_paths)
|
||||
|
||||
def parse_files(self, img_paths: Union[List[str], str],
|
||||
ann_paths: Union[List[str], str]) -> List[Tuple]:
|
||||
samples = track_parallel_progress_multi_args(
|
||||
self.parse_file, (img_paths, ann_paths), nproc=self.nproc)
|
||||
return samples
|
||||
|
||||
@abstractmethod
|
||||
def parse_file(self, img_path: str, ann_path: str) -> Tuple:
|
||||
|
||||
raise NotImplementedError
|
||||
```
|
||||
|
||||
为了保证后续模块的统一性,MMOCR 对 `parse_files` 与 `parse_file` 的返回值做了约定。 `parse_file` 的返回值为一个元组,元组中的第一个元素为图像路径,第二个元素为标注信息。标注信息为一个列表,列表中的每个元素为一个字典,字典中的字段为`poly`, `text`, `ignore`,如下所示:
|
||||
|
||||
```python
|
||||
# An example of returned values:
|
||||
(
|
||||
'imgs/train/xxx.jpg',
|
||||
[
|
||||
dict(
|
||||
poly=[0, 1, 1, 1, 1, 0, 0, 0],
|
||||
text='hello',
|
||||
ignore=False),
|
||||
...
|
||||
]
|
||||
)
|
||||
```
|
||||
|
||||
`parse_files` 的输出为一个列表,列表中的每个元素为 `parse_file` 的返回值。 示例为:
|
||||
|
||||
```python
|
||||
[
|
||||
(
|
||||
'imgs/train/xxx.jpg',
|
||||
[
|
||||
dict(
|
||||
poly=[0, 1, 1, 1, 1, 0, 0, 0],
|
||||
text='hello',
|
||||
ignore=False),
|
||||
...
|
||||
]
|
||||
),
|
||||
...
|
||||
]
|
||||
```
|
||||
|
||||
#### 数据集转换 (Packer)
|
||||
|
||||
`packer` 主要是将数据转化到统一的标注格式, 因为输入的数据为 Parsers 的输出,格式已经固定, 因此 Packer 只需要将输入的格式转化为每种任务统一的标注格式即可。如今 MMOCR 支持的任务有文本检测、文本识别、端对端OCR 以及关键信息提取,MMOCR 针对每个任务均有对应的 Packer,如下所示:
|
||||
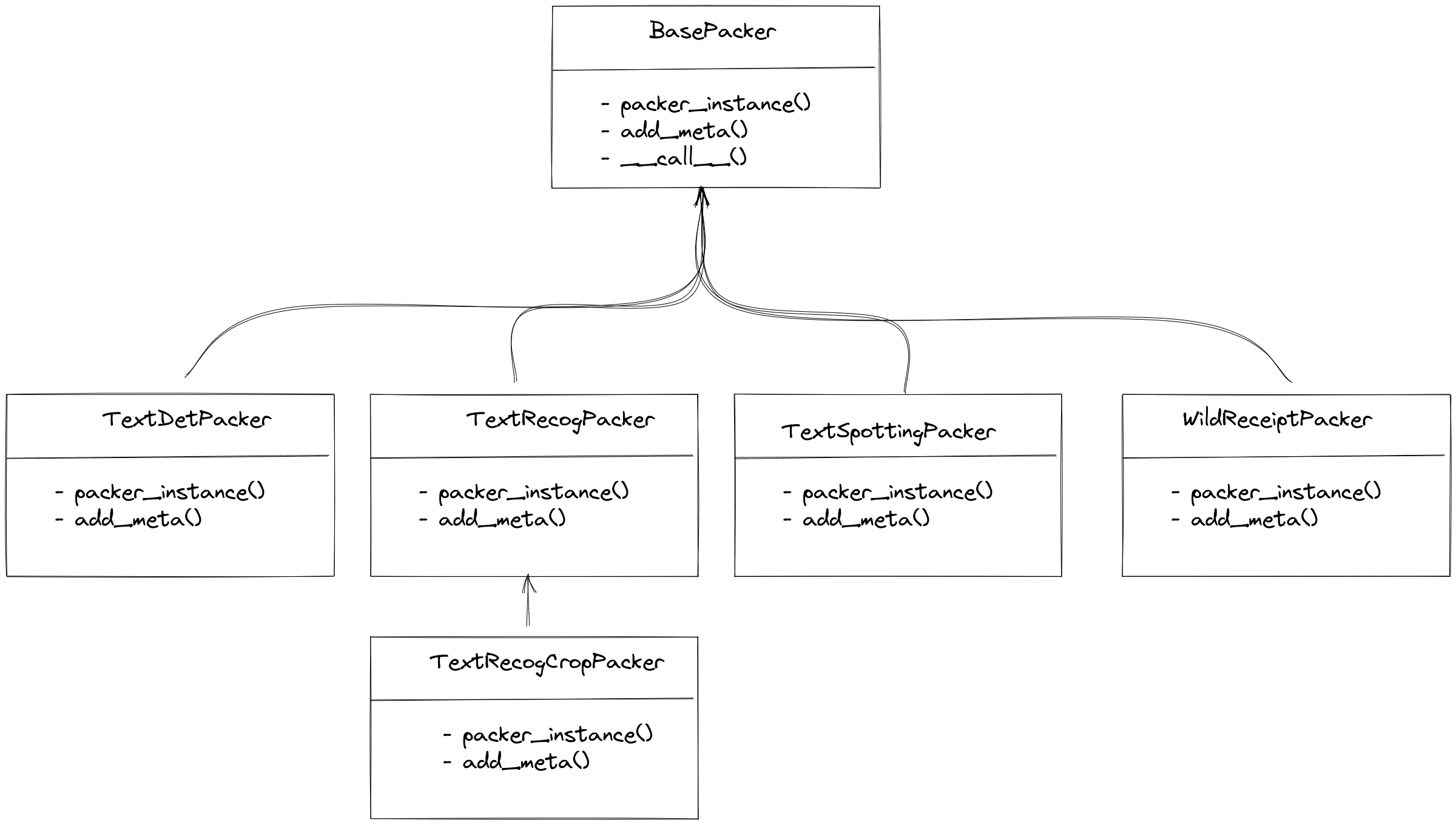
|
||||
|
||||
对于文字检测、端对端OCR及关键信息提取,MMOCR 均有唯一对应的 `Packer`。而在文字识别领域, MMOCR 则提供了两种 `Packer`,分别为 `TextRecogPacker` 和 `TextRecogCropPacker`,其原因在与文字识别的数据集存在两种情况:
|
||||
|
||||
- 每个图像均为一个识别样本,`parser` 返回的标注信息仅为一个`dict(text='xxx')`,此时使用 `TextRecogPacker` 即可。
|
||||
- 数据集没有将文字从图像中裁剪出来,本质是一个端对端OCR的标注,包含了文字的位置信息以及对应的文本信息,`TextRecogCropPacker` 会将文字从图像中裁剪出来,然后再转化成文字识别的统一格式。
|
||||
|
||||
#### 标注保存 (Dumper)
|
||||
|
||||
`dumper` 来决定要将数据保存为何种格式。目前,MMOCR 支持 `JsonDumper`, `WildreceiptOpensetDumper`,及 `TextRecogLMDBDumper`。他们分别用于将数据保存为标准的 MMOCR Json 格式、Wildreceipt 格式,及文本识别领域学术界常用的 LMDB 格式。
|
||||
|
||||
#### 临时文件清理 (Delete)
|
||||
|
||||
在处理数据集时,往往会产生一些不需要的临时文件。这里可以以列表的形式传入这些文件或文件夹,在结束转换时即会删除。
|
||||
|
||||
##### 生成基础配置 - `config_generator`
|
||||
#### 生成基础配置 (ConfigGenerator)
|
||||
|
||||
为了在数据集准备完毕后可以自动生成基础配置,目前,MMOCR 按任务实现了 `TextDetConfigGenerator`、`TextRecogConfigGenerator` 和 `TextSpottingConfigGenerator`。它们支持的主要参数如下:
|
||||
|
||||
| 字段名 | 含义 |
|
||||
| ----------- | --------------------------------------------------------------------------------------------------------------------------------------------------- |
|
||||
| data_root | 数据集存储的根目录 |
|
||||
| train_anns | 配置文件内训练集标注的路径。若不指定,则默认为 `[dict(ann_file='{taskname}_train.json', dataset_postfix='']`。 |
|
||||
| val_anns | 配置文件内验证集标注的路径。若不指定,则默认为空。 |
|
||||
| test_anns | 配置文件内测试集标注的路径。若不指定,则默认指向 `[dict(ann_file='{taskname}_test.json', dataset_postfix='']`。 |
|
||||
| config_path | 算法库存放配置文件的路径,配置生成器会将默认配置写入 `{config_path}/{taskname}/_base_/datasets/{dataset_name}.py` 下。若不指定,则默认为 `configs/` |
|
||||
|
||||
在准备好数据集的所有文件后,配置生成器就会自动生成调用该数据集所需要的基础配置文件。下面给出了一个最小化的 `TextDetConfigGenerator` 配置示例:
|
||||
|
||||
```python
|
||||
config_generator = dict(type='TextDetConfigGenerator', data_root=data_root)
|
||||
config_generator = dict(type='TextDetConfigGenerator')
|
||||
```
|
||||
|
||||
在准备好数据集的所有文件后,配置生成器 `TextDetConfigGenerator` 就会自动为 MMOCR 生成调用该数据集所需要的基础配置文件。生成后的文件默认会被置于 `configs/{task}/_base_/datasets/` 下。例如,本例中,icdar 2015 的基础配置文件就会被生成在 `configs/textdet/_base_/datasets/icdar2015.py` 下:
|
||||
生成后的文件默认会被置于 `configs/{task}/_base_/datasets/` 下。例如,本例中,icdar 2015 的基础配置文件就会被生成在 `configs/textdet/_base_/datasets/icdar2015.py` 下:
|
||||
|
||||
```python
|
||||
icdar2015_textdet_data_root = 'data/icdar2015'
|
||||
|
@ -313,7 +486,44 @@ icdar2015_textdet_test = dict(
|
|||
pipeline=None)
|
||||
```
|
||||
|
||||
有了该文件后,我们就能从模型的配置文件中直接导入该数据集到 `dataloader` 中使用(以下样例节选自 [`configs/textdet/dbnet/dbnet_resnet18_fpnc_1200e_icdar2015.py`](/configs/textdet/dbnet/dbnet_resnet18_fpnc_1200e_icdar2015.py)):
|
||||
假如数据集比较特殊,标注存在着几个变体,配置生成器也支持在基础配置中生成指向各自变体的变量,但这需要用户在设置时用不同的 `dataset_postfix` 区分。例如,ICDAR 2015 文字识别数据的测试集就存在着原版和 1811 两种标注版本,可以在 `test_anns` 中指定它们,如下所示:
|
||||
|
||||
```python
|
||||
config_generator = dict(
|
||||
type='TextRecogConfigGenerator',
|
||||
test_anns=[
|
||||
dict(ann_file='textrecog_test.json'),
|
||||
dict(dataset_postfix='857', ann_file='textrecog_test_857.json')
|
||||
])
|
||||
```
|
||||
|
||||
配置生成器会生成以下配置:
|
||||
|
||||
```python
|
||||
icdar2015_textrecog_data_root = 'data/icdar2015'
|
||||
|
||||
icdar2015_textrecog_train = dict(
|
||||
type='OCRDataset',
|
||||
data_root=icdar2015_textrecog_data_root,
|
||||
ann_file='textrecog_train.json',
|
||||
pipeline=None)
|
||||
|
||||
icdar2015_textrecog_test = dict(
|
||||
type='OCRDataset',
|
||||
data_root=icdar2015_textrecog_data_root,
|
||||
ann_file='textrecog_test.json',
|
||||
test_mode=True,
|
||||
pipeline=None)
|
||||
|
||||
icdar2015_1811_textrecog_test = dict(
|
||||
type='OCRDataset',
|
||||
data_root=icdar2015_textrecog_data_root,
|
||||
ann_file='textrecog_test_1811.json',
|
||||
test_mode=True,
|
||||
pipeline=None)
|
||||
```
|
||||
|
||||
有了该文件后,MMOCR 就能从模型的配置文件中直接导入该数据集到 `dataloader` 中使用(以下样例节选自 [`configs/textdet/dbnet/dbnet_resnet18_fpnc_1200e_icdar2015.py`](/configs/textdet/dbnet/dbnet_resnet18_fpnc_1200e_icdar2015.py)):
|
||||
|
||||
```python
|
||||
_base_ = [
|
||||
|
@ -339,19 +549,17 @@ test_dataloader = val_dataloader
|
|||
除非用户在运行脚本的时候手动指定了 `overwrite-cfg`,配置生成器默认不会自动覆盖已经存在的基础配置文件。
|
||||
```
|
||||
|
||||
由于每个任务所需的基本数据集配置格式不一,我们也针对各个任务推出了 `TextRecogConfigGenerator` 及 `TextSpottingConfigGenerator` 等生成器。
|
||||
|
||||
## 向 Dataset Preparer 添加新的数据集
|
||||
|
||||
### 添加公开数据集
|
||||
|
||||
MMOCR 已经支持了许多[常用的公开数据集](./datasetzoo.md)。如果你想用的数据集还没有被支持,并且你也愿意为 MMOCR 开源社区[贡献代码](../../notes/contribution_guide.md),你可以按照以下步骤来添加一个新的数据集。
|
||||
|
||||
接下来我们以添加 **ICDAR2013** 数据集为例,展示如何一步一步地添加一个新的公开数据集。
|
||||
接下来以添加 **ICDAR2013** 数据集为例,展示如何一步一步地添加一个新的公开数据集。
|
||||
|
||||
#### 添加 `metafile.yml`
|
||||
|
||||
首先,我们确认 `dataset_zoo/` 中不存在我们准备添加的数据集。然后我们先新建以待添加数据集命名的文件夹,如 `icdar2013/`(通常,我们使用不包含符号的小写英文字母及数字来命名数据集)。在 `icdar2013/` 文件夹中,我们新建 `metafile.yml` 文件,并按照以下模板来填充数据集的基本信息:
|
||||
首先,确认 `dataset_zoo/` 中不存在准备添加的数据集。然后我们先新建以待添加数据集命名的文件夹,如 `icdar2013/`(通常,使用不包含符号的小写英文字母及数字来命名数据集)。在 `icdar2013/` 文件夹中,新建 `metafile.yml` 文件,并按照以下模板来填充数据集的基本信息:
|
||||
|
||||
```yaml
|
||||
Name: 'Incidental Scene Text IC13'
|
||||
|
@ -387,281 +595,9 @@ Data:
|
|||
- Horizontal
|
||||
```
|
||||
|
||||
`metafile.yml` 存储了数据集的基本信息,这些信息一方面可以帮助用户了解数据集的基本情况,另一方面也会被用于自动化脚本来生成相应的数据集文档。因此,当你向 MMOCR Dataset Preparer 添加新的数据集支持时,请按照以上模板来填充 `metafile.yml` 文件。具体地,我们在下表中列出每个字段对应的含义:
|
||||
|
||||
| 字段名 | 含义 |
|
||||
| :--------------- | :-------------------------------------------------------------------- |
|
||||
| Name | 数据集的名称 |
|
||||
| Paper.Title | 数据集论文的标题 |
|
||||
| Paper.URL | 数据集论文的链接 |
|
||||
| Paper.Venue | 数据集论文发表的会议/期刊名称 |
|
||||
| Paper.Year | 数据集论文发表的年份 |
|
||||
| Paper.BibTeX | 数据集论文的引用的 BibTex |
|
||||
| Data.Website | 数据集的官方网站 |
|
||||
| Data.Language | 数据集支持的语言 |
|
||||
| Data.Scene | 数据集支持的场景,如 `Natural Scene`, `Document`, `Handwritten` 等 |
|
||||
| Data.Granularity | 数据集支持的粒度,如 `Character`, `Word`, `Line` 等 |
|
||||
| Data.Tasks | 数据集支持的任务,如 `textdet`, `textrecog`, `textspotting`, `kie` 等 |
|
||||
| Data.License | 数据集的许可证信息,如果不存在许可证,则使用 `N/A` 填充 |
|
||||
| Data.Format | 数据集标注文件的格式,如 `.txt`, `.xml`, `.json` 等 |
|
||||
| Data.Keywords | 数据集的特性关键词,如 `Horizontal`, `Vertical`, `Curved` 等 |
|
||||
|
||||
#### 添加对应任务的配置文件
|
||||
|
||||
在 `dataset_zoo/icdar2013` 中,我们接着添加以任务名称命名的 `.py` 配置文件。如 `textdet.py`,`textrecog.py`,`textspotting.py`,`kie.py` 等。在该文件中,我们需要对数据获取方式和转换方式进行配置。
|
||||
|
||||
##### 配置数据集获取方法 `data_obtainer`
|
||||
|
||||
以文本检测任务为例:
|
||||
|
||||
```python
|
||||
data_obtainer = dict(
|
||||
type='NaiveDataObtainer',
|
||||
cache_path=cache_path,
|
||||
data_root=data_root,
|
||||
files=[
|
||||
dict(
|
||||
url='https://rrc.cvc.uab.es/downloads/'
|
||||
'Challenge2_Training_Task12_Images.zip',
|
||||
save_name='ic13_textdet_train_img.zip',
|
||||
md5='a443b9649fda4229c9bc52751bad08fb',
|
||||
split=['train'],
|
||||
content=['image'],
|
||||
mapping=[['ic13_textdet_train_img', 'textdet_imgs/train']]),
|
||||
dict(
|
||||
url='https://rrc.cvc.uab.es/downloads/'
|
||||
'Challenge2_Training_Task1_GT.zip',
|
||||
save_name='ic13_textdet_test_img.zip',
|
||||
md5='af2e9f070c4c6a1c7bdb7b36bacf23e3',
|
||||
split=['test'],
|
||||
content=['image'],
|
||||
mapping=[['ic13_textdet_test_img', 'textdet_imgs/test']]),
|
||||
dict(
|
||||
url='https://rrc.cvc.uab.es/downloads/'
|
||||
'Challenge2_Test_Task12_Images.zip',
|
||||
save_name='ic13_textdet_train_gt.zip',
|
||||
md5='f3a425284a66cd67f455d389c972cce4',
|
||||
split=['train'],
|
||||
content=['annotation'],
|
||||
mapping=[['ic13_textdet_train_gt', 'annotations/train']]),
|
||||
dict(
|
||||
url='https://rrc.cvc.uab.es/downloads/'
|
||||
'Challenge2_Test_Task1_GT.zip',
|
||||
save_name='ic13_textdet_test_gt.zip',
|
||||
md5='3191c34cd6ac28b60f5a7db7030190fb',
|
||||
split=['test'],
|
||||
content=['annotation'],
|
||||
mapping=[['ic13_textdet_test_gt', 'annotations/test']]),
|
||||
])
|
||||
```
|
||||
|
||||
我们首先需要配置数据集获取方法,即 `data_obtainer`,通常来说,内置的 `NaiveDataObtainer` 即可完成绝大部分可以通过直链访问的数据集的下载。`NaiveDataObtainer` 将完成下载、解压、移动文件和重命名等操作。目前,我们暂时不支持自动下载存储在百度或谷歌网盘等需要登陆才能访问资源的数据集。
|
||||
|
||||
如下表所示,`data_obtainer` 主要由四个字段构成:
|
||||
|
||||
| 字段名 | 含义 |
|
||||
| ---------- | ---------------------------------------------------------- |
|
||||
| type | 数据集获取方法,目前仅支持 `NaiveDataObtainer` |
|
||||
| cache_path | 数据集缓存路径,用于存储数据集准备过程中下载的压缩包等文件 |
|
||||
| data_root | 数据集存储的根目录 |
|
||||
| files | 数据集文件列表,用于描述数据集的下载信息 |
|
||||
|
||||
`files` 字段是一个列表,列表中的每个元素都是一个字典,用于描述一个数据集文件的下载信息。如下表所示:
|
||||
|
||||
| 字段名 | 含义 |
|
||||
| ----------------- | -------------------------------------------------------------------- |
|
||||
| url | 数据集文件的下载链接 |
|
||||
| save_name | 数据集文件的保存名称 |
|
||||
| md5 (可选) | 数据集文件的 md5 值,用于校验下载的文件是否完整 |
|
||||
| split (可选) | 数据集文件所属的数据集划分,如 `train`,`test` 等,该字段可以空缺 |
|
||||
| content (可选) | 数据集文件的内容,如 `image`,`annotation` 等,该字段可以空缺 |
|
||||
| mapping (可选) | 数据集文件的解压映射,用于指定解压后的文件存储的位置,该字段可以空缺 |
|
||||
|
||||
```{note}
|
||||
为了让 `data_converter.gatherer` 正常运行,我们约定数据集的图片和标注分别储存在 `{taskname}_imgs/{split}/` 和 `annotations/` 下。特别地,对于[多对多的情况](#gatherer),标注文件需要放置于 `annotations/{split}` 下。
|
||||
```
|
||||
|
||||
##### 配置数据集转换器 `data_converter`
|
||||
|
||||
数据集转换器 `data_converter` 主要由标注文件获取器 `gatherer`,原始标注解析器 `parser`,以及数据存储器 `dumper` 组成。其中 `gatherer` 负责将图片与标注文件进行匹配,`parser` 负责将原始标注文件解析为标准格式,`dumper` 负责将标准格式的标注文件存储为 MMOCR 支持的格式。
|
||||
|
||||
一般来说,用户无需重新实现新的 `gatherer` 或 `dumper`,但是通常需要根据数据集的标注格式实现新的 `parser`。
|
||||
|
||||
我们通过观察获取的 ICDAR2013 数据集文件发现,其每一张图片都有一个对应的 `.txt` 格式的标注文件:
|
||||
|
||||
```text
|
||||
data_root
|
||||
├── textdet_imgs/train/
|
||||
│ ├── img_1.jpg
|
||||
│ ├── img_2.jpg
|
||||
│ └── ...
|
||||
├── annotations/train/
|
||||
│ ├── gt_img_1.txt
|
||||
│ ├── gt_img_2.txt
|
||||
│ └── ...
|
||||
```
|
||||
|
||||
且每个标注文件名与图片的对应关系为:`gt_img_1.txt` 对应 `img_1.jpg`,以此类推。因此,我们可以使用 `pair_gather` 来进行匹配。
|
||||
|
||||
```python
|
||||
gatherer=dict(
|
||||
type='pair_gather',
|
||||
suffixes=['.jpg'],
|
||||
rule=[r'(\w+)\.jpg', r'gt_\1.txt'])
|
||||
```
|
||||
|
||||
其中,规则 `rule` 是一个[正则表达式对](https://docs.python.org/3/library/re.html),第一个正则表达式用于匹配图片文件名,第二个正则表达式用于匹配标注文件名。在这里,我们使用 `(\w+)` 来匹配图片文件名,使用 `gt_\1.txt` 来匹配标注文件名,其中 `\1` 表示第一个正则表达式匹配到的内容。即,实现了将 `img_xx.jpg` 替换为 `gt_img_xx.txt` 的功能。
|
||||
|
||||
接下来,我们需要实现 `parser`,即将原始标注文件解析为标准格式。通常来说,用户在添加新的数据集前,可以浏览已支持数据集的[详情页](./datasetzoo.md),并查看是否已有相同格式的数据集。如果已有相同格式的数据集,则可以直接使用该数据集的 `parser`。否则,则需要实现新的格式解析器。
|
||||
|
||||
数据格式解析器被统一存储在 `mmocr/datasets/preparers/parsers` 目录下。所有的 `parser` 都需要继承 `BaseParser`,并实现 `parse_file` 或 `parse_files` 方法。
|
||||
|
||||
其中,`parse_file()` 方法用于解析单个标注文件,如下代码块所示,`parse_file()` 接受两个参数,`file` 是一个 `Tuple` 类型的变量,包含了由 `gatherer` 获取的图片文件路径和标注文件路径,而 `split` 则会传入当前处理的数据集划分,如 `train` 或 `test`。
|
||||
|
||||
```python
|
||||
def parse_file(self, file: Tuple, split: str) -> Tuple:
|
||||
"""Convert annotation for a single image.
|
||||
|
||||
Args:
|
||||
file (Tuple): A tuple of path to image and annotation
|
||||
split (str): Current split.
|
||||
|
||||
Returns:
|
||||
Tuple: A tuple of (img_path, instance). Instance is a dict
|
||||
containing parsed annotations, which should contain the
|
||||
following keys:
|
||||
- 'poly' or 'box' (textdet or textspotting)
|
||||
- 'text' (textspotting or textrecog)
|
||||
- 'ignore' (all task)
|
||||
"""
|
||||
```
|
||||
|
||||
`parse_file()` 方法的输出是一个 `Tuple` 类型的变量,包含了图片文件路径和标注信息 `instance`。其中,`instance` 是一个字典列表,包含了解析后的标注信息,依据不同的任务类型,该列表中的每一个字典必须包含以下键:
|
||||
|
||||
| 键名 | 任务类型 | 说明 |
|
||||
| :------- | :--------------------- | :------------------------------------- |
|
||||
| box/poly | textdet/textspotting | 矩形框坐标 `box` 或多边形框坐标 `poly` |
|
||||
| text | textrecog/textspotting | 文本内容 `text` |
|
||||
| ignore | all task | 是否忽略该样本 |
|
||||
|
||||
以下代码块反映了一个 `parse_file()` 方法返回的数据示例:
|
||||
|
||||
```python
|
||||
('imgs/train/xxx.jpg',
|
||||
dict(
|
||||
poly=[[[0, 1], [1, 1], [1, 0], [0, 0]]],
|
||||
text='hello',
|
||||
ignore=False)
|
||||
)
|
||||
```
|
||||
|
||||
需要注意的是,`parse_file()` 方法解析单个标注文件,并返回单张图片的标注信息,**仅能在标注图像与标注文件满足“多对多”关系时使用**。当仅存在单个标注文件时,可以通过重写 `parse_files()` 方法的方式,直接返回所有样本的数据信息。用户可以参见 `mmocr/datasets/preparers/parsers/totaltext_parser.py` 中的实现。
|
||||
|
||||
通过观察 ICDAR2013 数据集的标注文件:
|
||||
|
||||
```text
|
||||
158 128 411 181 "Footpath"
|
||||
443 128 501 169 "To"
|
||||
64 200 363 243 "Colchester"
|
||||
542, 710, 938, 841, "break"
|
||||
87, 884, 457, 1021, "could"
|
||||
517, 919, 831, 1024, "save"
|
||||
```
|
||||
|
||||
我们发现内置的 `ICDARTxtTextDetAnnParser` 已经可以满足我们的需求,因此我们可以直接使用该 `parser`,并将其配置到 `preparer` 中。
|
||||
|
||||
```python
|
||||
parser=dict(
|
||||
type='ICDARTxtTextDetAnnParser',
|
||||
remove_strs=[',', '"'],
|
||||
encoding='utf-8',
|
||||
format='x1 y1 x2 y2 trans',
|
||||
separator=' ',
|
||||
mode='xyxy')
|
||||
```
|
||||
|
||||
其中,由于标注文件中混杂了多余的引号 `“”` 和逗号 `,`,我们通过指定 `remove_strs=[',', '"']` 来进行移除。另外,我们在 `format` 中指定了标注文件的格式,其中 `x1 y1 x2 y2 trans` 表示标注文件中的每一行包含了四个坐标和一个文本内容,且坐标和文本内容之间使用空格分隔(`separator`=' ')。另外,我们需要指定 `mode` 为 `xyxy`,表示标注文件中的坐标是左上角和右下角的坐标,这样以来,`ICDARTxtTextDetAnnParser` 即可将该格式的标注解析为 MMOCR 统一格式。
|
||||
|
||||
最后,数据格式转换器 `data_converter` 的完整配置如下:
|
||||
|
||||
```python
|
||||
data_converter = dict(
|
||||
type='TextDetDataConverter',
|
||||
splits=['train', 'test'],
|
||||
data_root=data_root,
|
||||
gatherer=dict(
|
||||
type='pair_gather',
|
||||
suffixes=['.jpg'],
|
||||
rule=[r'(\w+)\.jpg', r'gt_\1.txt']),
|
||||
parser=dict(
|
||||
type='ICDARTxtTextDetAnnParser',
|
||||
remove_strs=[',', '"'],
|
||||
encoding='utf-8',
|
||||
format='x1 y1 x2 y2 trans',
|
||||
separator=' ',
|
||||
mode='xyxy'),
|
||||
dumper=dict(type='JsonDumper'))
|
||||
```
|
||||
|
||||
##### 配置基础配置生成器 `config_generator`
|
||||
|
||||
为了在数据集准备完毕后可以自动生成基础配置,我们还需要配置一下对应任务的 `config_generator`。目前,MMOCR 按任务实现了 `TextDetConfigGenerator`、`TextRecogConfigGenerator` 和 `TextSpottingConfigGenerator`。它们支持的主要参数如下:
|
||||
|
||||
| 字段名 | 含义 |
|
||||
| ----------- | --------------------------------------------------------------------------------------------------------------------------------------------------- |
|
||||
| data_root | 数据集存储的根目录 |
|
||||
| train_anns | 配置文件内训练集标注的路径。若不指定,则默认为 `[dict(ann_file='{taskname}_train.json', dataset_postfix='']`。 |
|
||||
| val_anns | 配置文件内验证集标注的路径。若不指定,则默认为空。 |
|
||||
| test_anns | 配置文件内测试集标注的路径。若不指定,则默认指向 `[dict(ann_file='{taskname}_test.json', dataset_postfix='']`。 |
|
||||
| config_path | 算法库存放配置文件的路径,配置生成器会将默认配置写入 `{config_path}/{taskname}/_base_/datasets/{dataset_name}.py` 下。若不指定,则默认为 `configs/` |
|
||||
|
||||
在本例中,我们只需要设置一下 `TextDetConfigGenerator` 的 `data_root` 字段,其它字段保持默认即可。
|
||||
|
||||
```python
|
||||
config_generator = dict(type='TextDetConfigGenerator', data_root=data_root)
|
||||
```
|
||||
|
||||
假如数据集比较特殊,标注存在着几个变体,配置生成器也支持在基础配置中生成指向各自变体的变量,但这需要用户在设置时用不同的 `dataset_postfix` 区分。例如,ICDAR 2015 文字识别数据的测试集就存在着原版和 1811 两种标注版本,我们可以在 `test_anns` 中指定它们,如下所示:
|
||||
|
||||
```python
|
||||
config_generator = dict(
|
||||
type='TextRecogConfigGenerator',
|
||||
data_root=data_root,
|
||||
test_anns=[
|
||||
dict(ann_file='textrecog_test.json'),
|
||||
dict(dataset_postfix='857', ann_file='textrecog_test_857.json')
|
||||
])
|
||||
```
|
||||
|
||||
配置生成器会生成以下配置:
|
||||
|
||||
```python
|
||||
icdar2015_textrecog_data_root = 'data/icdar2015'
|
||||
|
||||
icdar2015_textrecog_train = dict(
|
||||
type='OCRDataset',
|
||||
data_root=icdar2015_textrecog_data_root,
|
||||
ann_file='textrecog_train.json',
|
||||
pipeline=None)
|
||||
|
||||
icdar2015_textrecog_test = dict(
|
||||
type='OCRDataset',
|
||||
data_root=icdar2015_textrecog_data_root,
|
||||
ann_file='textrecog_test.json',
|
||||
test_mode=True,
|
||||
pipeline=None)
|
||||
|
||||
icdar2015_1811_textrecog_test = dict(
|
||||
type='OCRDataset',
|
||||
data_root=icdar2015_textrecog_data_root,
|
||||
ann_file='textrecog_test_1811.json',
|
||||
test_mode=True,
|
||||
pipeline=None)
|
||||
```
|
||||
|
||||
#### 添加标注示例
|
||||
|
||||
最后,我们可以在 `dataset_zoo/icdar2013/` 目录下添加标注示例文件 `sample_anno.md` 以帮助文档脚本在生成文档时添加标注示例,标注示例文件是一个 Markdown 文件,其内容通常包含了单个样本的原始数据格式。例如,以下代码块展示了 ICDAR2013 数据集的数据样例文件:
|
||||
最后,可以在 `dataset_zoo/icdar2013/` 目录下添加标注示例文件 `sample_anno.md` 以帮助文档脚本在生成文档时添加标注示例,标注示例文件是一个 Markdown 文件,其内容通常包含了单个样本的原始数据格式。例如,以下代码块展示了 ICDAR2013 数据集的数据样例文件:
|
||||
|
||||
````markdown
|
||||
**Text Detection**
|
||||
|
@ -683,6 +619,162 @@ icdar2015_1811_textrecog_test = dict(
|
|||
```
|
||||
````
|
||||
|
||||
#### 添加对应任务的配置文件
|
||||
|
||||
在 `dataset_zoo/icdar2013` 中,接着添加以任务名称命名的 `.py` 配置文件。如 `textdet.py`,`textrecog.py`,`textspotting.py`,`kie.py` 等。配置模板如下所示:
|
||||
|
||||
```python
|
||||
data_root = ''
|
||||
data_cache = 'data/cache'
|
||||
train_prepare = dict(
|
||||
obtainer=dict(
|
||||
type='NaiveObtainer',
|
||||
data_cache=data_cache,
|
||||
files=[
|
||||
dict(
|
||||
url='xx',
|
||||
md5='',
|
||||
save_name='xxx',
|
||||
mapping=list())
|
||||
]),
|
||||
gatherer=dict(type='xxxGatherer', **kwargs),
|
||||
parser=dict(type='xxxParser', **kwargs),
|
||||
packer=dict(type='TextxxxPacker'), # 对应任务的 Packer
|
||||
dumper=dict(type='JsonDumper'),
|
||||
)
|
||||
test_prepare = dict(
|
||||
obtainer=dict(
|
||||
type='NaiveObtainer',
|
||||
data_cache=data_cache,
|
||||
files=[
|
||||
dict(
|
||||
url='xx',
|
||||
md5='',
|
||||
save_name='xxx',
|
||||
mapping=list())
|
||||
]),
|
||||
gatherer=dict(type='xxxGatherer', **kwargs),
|
||||
parser=dict(type='xxxParser', **kwargs),
|
||||
packer=dict(type='TextxxxPacker'), # 对应任务的 Packer
|
||||
dumper=dict(type='JsonDumper'),
|
||||
)
|
||||
```
|
||||
|
||||
以文件检测任务为例,来介绍配置文件的具体内容。
|
||||
一般情况下用户无需重新实现新的 `obtainer`, `gatherer`, `packer` 或 `dumper`,但是通常需要根据数据集的标注格式实现新的 `parser`。
|
||||
对于 `obtainer` 的配置这里不在做过的介绍,可以参考 [数据集下载、解压、移动](#数据集下载解压移动-obtainer)。
|
||||
针对 `gatherer`,通过观察获取的 ICDAR2013 数据集文件发现,其每一张图片都有一个对应的 `.txt` 格式的标注文件:
|
||||
|
||||
```text
|
||||
data_root
|
||||
├── textdet_imgs/train/
|
||||
│ ├── img_1.jpg
|
||||
│ ├── img_2.jpg
|
||||
│ └── ...
|
||||
├── annotations/train/
|
||||
│ ├── gt_img_1.txt
|
||||
│ ├── gt_img_2.txt
|
||||
│ └── ...
|
||||
```
|
||||
|
||||
且每个标注文件名与图片的对应关系为:`gt_img_1.txt` 对应 `img_1.jpg`,以此类推。因此可以使用 `PairGatherer` 来进行匹配。
|
||||
|
||||
```python
|
||||
gatherer=dict(
|
||||
type='PairGatherer',
|
||||
img_suffixes=['.jpg'],
|
||||
rule=[r'(\w+)\.jpg', r'gt_\1.txt'])
|
||||
```
|
||||
|
||||
规则 `rule` 第一个正则表达式用于匹配图片文件名,第二个正则表达式用于匹配标注文件名。在这里,使用 `(\w+)` 来匹配图片文件名,使用 `gt_\1.txt` 来匹配标注文件名,其中 `\1` 表示第一个正则表达式匹配到的内容。即,实现了将 `img_xx.jpg` 替换为 `gt_img_xx.txt` 的功能。
|
||||
|
||||
接下来,需要实现 `parser`,即将原始标注文件解析为标准格式。通常来说,用户在添加新的数据集前,可以浏览已支持数据集的[详情页](./datasetzoo.md),并查看是否已有相同格式的数据集。如果已有相同格式的数据集,则可以直接使用该数据集的 `parser`。否则,则需要实现新的格式解析器。
|
||||
|
||||
数据格式解析器被统一存储在 `mmocr/datasets/preparers/parsers` 目录下。所有的 `parser` 都需要继承 `BaseParser`,并实现 `parse_file` 或 `parse_files` 方法。具体可以参考[数据集解析](#数据集解析)
|
||||
|
||||
通过观察 ICDAR2013 数据集的标注文件:
|
||||
|
||||
```text
|
||||
158 128 411 181 "Footpath"
|
||||
443 128 501 169 "To"
|
||||
64 200 363 243 "Colchester"
|
||||
542, 710, 938, 841, "break"
|
||||
87, 884, 457, 1021, "could"
|
||||
517, 919, 831, 1024, "save"
|
||||
```
|
||||
|
||||
我们发现内置的 `ICDARTxtTextDetAnnParser` 已经可以满足需求,因此可以直接使用该 `parser`,并将其配置到 `preparer` 中。
|
||||
|
||||
```python
|
||||
parser=dict(
|
||||
type='ICDARTxtTextDetAnnParser',
|
||||
remove_strs=[',', '"'],
|
||||
encoding='utf-8',
|
||||
format='x1 y1 x2 y2 trans',
|
||||
separator=' ',
|
||||
mode='xyxy')
|
||||
```
|
||||
|
||||
其中,由于标注文件中混杂了多余的引号 `“”` 和逗号 `,`,可以通过指定 `remove_strs=[',', '"']` 来进行移除。另外在 `format` 中指定了标注文件的格式,其中 `x1 y1 x2 y2 trans` 表示标注文件中的每一行包含了四个坐标和一个文本内容,且坐标和文本内容之间使用空格分隔(`separator`=' ')。另外,需要指定 `mode` 为 `xyxy`,表示标注文件中的坐标是左上角和右下角的坐标,这样以来,`ICDARTxtTextDetAnnParser` 即可将该格式的标注解析为统一格式。
|
||||
|
||||
对于 `packer`,以文件检测任务为例,其 `packer` 为 `TextDetPacker`,其配置如下:
|
||||
|
||||
```python
|
||||
packer=dict(type='TextDetPacker')
|
||||
```
|
||||
|
||||
最后,指定 `dumper`,这里一般情况下保存为json格式,其配置如下:
|
||||
|
||||
```python
|
||||
dumper=dict(type='JsonDumper')
|
||||
```
|
||||
|
||||
经过上述配置后,针对 ICDAR2013 训练集的配置文件如下:
|
||||
|
||||
```python
|
||||
train_preparer = dict(
|
||||
obtainer=dict(
|
||||
type='NaiveDataObtainer',
|
||||
cache_path=cache_path,
|
||||
files=[
|
||||
dict(
|
||||
url='https://rrc.cvc.uab.es/downloads/'
|
||||
'Challenge2_Training_Task12_Images.zip',
|
||||
save_name='ic13_textdet_train_img.zip',
|
||||
md5='a443b9649fda4229c9bc52751bad08fb',
|
||||
content=['image'],
|
||||
mapping=[['ic13_textdet_train_img', 'textdet_imgs/train']]),
|
||||
dict(
|
||||
url='https://rrc.cvc.uab.es/downloads/'
|
||||
'Challenge2_Training_Task1_GT.zip',
|
||||
save_name='ic13_textdet_train_gt.zip',
|
||||
md5='f3a425284a66cd67f455d389c972cce4',
|
||||
content=['annotation'],
|
||||
mapping=[['ic13_textdet_train_gt', 'annotations/train']]),
|
||||
]),
|
||||
gatherer=dict(
|
||||
type='PairGatherer',
|
||||
img_suffixes=['.jpg'],
|
||||
rule=[r'(\w+)\.jpg', r'gt_\1.txt']),
|
||||
parser=dict(
|
||||
type='ICDARTxtTextDetAnnParser',
|
||||
remove_strs=[',', '"'],
|
||||
format='x1 y1 x2 y2 trans',
|
||||
separator=' ',
|
||||
mode='xyxy'),
|
||||
packer=dict(type='TextDetPacker'),
|
||||
dumper=dict(type='JsonDumper'),
|
||||
)
|
||||
```
|
||||
|
||||
为了在数据集准备完毕后可以自动生成基础配置, 还需要配置一下对应任务的 `config_generator`。
|
||||
|
||||
在本例中,因为为文字检测任务,仅需要设置 Generator 为 `TextDetConfigGenerator`即可
|
||||
|
||||
```python
|
||||
config_generator = dict(type='TextDetConfigGenerator', )
|
||||
```
|
||||
|
||||
### 添加私有数据集
|
||||
|
||||
待更新...
|
||||
|
|
|
@ -1,10 +1,10 @@
|
|||
# 常用工具
|
||||
|
||||
## 分析工具
|
||||
## 可视化工具
|
||||
|
||||
### 数据集可视化工具
|
||||
|
||||
MMOCR 提供了数据集可视化工具 `tools/analysis_tools/browse_datasets.py` 以辅助用户排查可能遇到的数据集相关的问题。用户只需要指定所使用的训练配置文件(通常存放在如 `configs/textdet/dbnet/xxx.py` 文件中)或数据集配置(通常存放在 `configs/textdet/_base_/datasets/xxx.py` 文件中)路径。该工具将依据输入的配置文件类型自动将经过数据流水线(data pipeline)处理过的图像及其对应的标签,或原始图片及其对应的标签绘制出来。
|
||||
MMOCR 提供了数据集可视化工具 `tools/visualizations/browse_datasets.py` 以辅助用户排查可能遇到的数据集相关的问题。用户只需要指定所使用的训练配置文件(通常存放在如 `configs/textdet/dbnet/xxx.py` 文件中)或数据集配置(通常存放在 `configs/textdet/_base_/datasets/xxx.py` 文件中)路径。该工具将依据输入的配置文件类型自动将经过数据流水线(data pipeline)处理过的图像及其对应的标签,或原始图片及其对应的标签绘制出来。
|
||||
|
||||
#### 支持参数
|
||||
|
||||
|
@ -37,7 +37,7 @@ python tools/visualizations/browse_dataset.py \
|
|||
|
||||
```Bash
|
||||
# 使用默认参数可视化 "dbnet_r50dcn_v2_fpnc_1200e_icadr2015" 模型的训练数据
|
||||
python tools/analysis_tools/browse_dataset.py configs/textdet/dbnet/dbnet_resnet50-dcnv2_fpnc_1200e_icdar2015.py
|
||||
python tools/visualizations/browse_dataset.py configs/textdet/dbnet/dbnet_resnet50-dcnv2_fpnc_1200e_icdar2015.py
|
||||
```
|
||||
|
||||
默认情况下,可视化模式为 "transformed",您将看到经由数据流水线变换过后的图像和标注:
|
||||
|
@ -49,7 +49,7 @@ python tools/analysis_tools/browse_dataset.py configs/textdet/dbnet/dbnet_resnet
|
|||
如果您只想可视化原始数据集,只需将模式设置为 "original":
|
||||
|
||||
```Bash
|
||||
python tools/analysis_tools/browse_dataset.py configs/textdet/dbnet/dbnet_resnet50-dcnv2_fpnc_1200e_icdar2015.py -m original
|
||||
python tools/visualizations/browse_dataset.py configs/textdet/dbnet/dbnet_resnet50-dcnv2_fpnc_1200e_icdar2015.py -m original
|
||||
```
|
||||
|
||||
<div align=center><img src="https://user-images.githubusercontent.com/22607038/206646570-382d0f26-908a-4ab4-b1a7-5cc31fa70c5f.jpg" style=" width: auto; height: 40%; "></div>
|
||||
|
@ -57,7 +57,7 @@ python tools/analysis_tools/browse_dataset.py configs/textdet/dbnet/dbnet_resnet
|
|||
或者,您也可以使用 "pipeline" 模式来可视化整个数据流水线的中间结果:
|
||||
|
||||
```Bash
|
||||
python tools/analysis_tools/browse_dataset.py configs/textdet/dbnet/dbnet_resnet50-dcnv2_fpnc_1200e_icdar2015.py -m pipeline
|
||||
python tools/visualizations/browse_dataset.py configs/textdet/dbnet/dbnet_resnet50-dcnv2_fpnc_1200e_icdar2015.py -m pipeline
|
||||
```
|
||||
|
||||
<div align=center><img src="https://user-images.githubusercontent.com/22607038/206637571-287640c0-1f55-453f-a2fc-9f9734b9593f.jpg" style=" width: auto; height: 40%; "></div>
|
||||
|
@ -65,7 +65,7 @@ python tools/analysis_tools/browse_dataset.py configs/textdet/dbnet/dbnet_resnet
|
|||
另外,用户还可以通过指定数据集配置文件的路径来可视化数据集的原始图像及其对应的标注,例如:
|
||||
|
||||
```Bash
|
||||
python tools/analysis_tools/browse_dataset.py configs/textrecog/_base_/datasets/icdar2015.py
|
||||
python tools/visualizations/browse_dataset.py configs/textrecog/_base_/datasets/icdar2015.py
|
||||
```
|
||||
|
||||
部分数据集可能有多个变体。例如,`icdar2015` 文本识别数据集的[配置文件](/configs/textrecog/_base_/datasets/icdar2015.py)中包含两个测试集变体,分别为 `icdar2015_textrecog_test` 和 `icdar2015_1811_textrecog_test`,如下所示:
|
||||
|
@ -85,11 +85,58 @@ icdar2015_1811_textrecog_test = dict(
|
|||
在这种情况下,用户可以通过指定 `-p` 参数来可视化不同的变体,例如,使用以下命令可视化 `icdar2015_1811_textrecog_test` 变体:
|
||||
|
||||
```Bash
|
||||
python tools/analysis_tools/browse_dataset.py configs/textrecog/_base_/datasets/icdar2015.py -p icdar2015_1811_textrecog_test
|
||||
python tools/visualizations/browse_dataset.py configs/textrecog/_base_/datasets/icdar2015.py -p icdar2015_1811_textrecog_test
|
||||
```
|
||||
|
||||
基于该工具,用户可以轻松地查看数据集的原始图像及其对应的标注,以便于检查数据集的标注是否正确。
|
||||
|
||||
### 优化器参数策略可视化工具
|
||||
|
||||
MMOCR提供了优化器参数可视化工具 `tools/visualizations/vis_scheduler.py` 以辅助用户排查优化器的超参数调度器(无需训练),支持学习率(learning rate)和动量(momentum)。
|
||||
|
||||
#### 工具简介
|
||||
|
||||
```bash
|
||||
python tools/visualizations/vis_scheduler.py \
|
||||
${CONFIG_FILE} \
|
||||
[-p, --parameter ${PARAMETER_NAME}] \
|
||||
[-d, --dataset-size ${DATASET_SIZE}] \
|
||||
[-n, --ngpus ${NUM_GPUs}] \
|
||||
[-s, --save-path ${SAVE_PATH}] \
|
||||
[--title ${TITLE}] \
|
||||
[--style ${STYLE}] \
|
||||
[--window-size ${WINDOW_SIZE}] \
|
||||
[--cfg-options]
|
||||
```
|
||||
|
||||
**所有参数的说明**:
|
||||
|
||||
- `config` : 模型配置文件的路径。
|
||||
- **`-p, parameter`**: 可视化参数名,只能为 `["lr", "momentum"]` 之一, 默认为 `"lr"`.
|
||||
- **`-d, --dataset-size`**: 数据集的大小。如果指定,`build_dataset` 将被跳过并使用这个大小作为数据集大小,默认使用 `build_dataset` 所得数据集的大小。
|
||||
- **`-n, --ngpus`**: 使用 GPU 的数量, 默认为1。
|
||||
- **`-s, --save-path`**: 保存的可视化图片的路径,默认不保存。
|
||||
- `--title`: 可视化图片的标题,默认为配置文件名。
|
||||
- `--style`: 可视化图片的风格,默认为 `whitegrid`。
|
||||
- `--window-size`: 可视化窗口大小,如果没有指定,默认为 `12*7`。如果需要指定,按照格式 \`W\*H'。
|
||||
- `--cfg-options`: 对配置文件的修改,参考[学习配置文件](../user_guides/config.md)。
|
||||
|
||||
```{note}
|
||||
部分数据集在解析标注阶段比较耗时,可直接将 `-d, dataset-size` 指定数据集的大小,以节约时间。
|
||||
```
|
||||
|
||||
#### 如何在开始训练前可视化学习率曲线
|
||||
|
||||
你可以使用如下命令来绘制配置文件 `configs/textdet/dbnet/dbnet_resnet50-dcnv2_fpnc_1200e_icdar2015.py` 将会使用的变化率曲线:
|
||||
|
||||
```bash
|
||||
python tools/visualizations/vis_scheduler.py configs/textdet/dbnet/dbnet_resnet50-dcnv2_fpnc_1200e_icdar2015.py -d 100
|
||||
```
|
||||
|
||||
<div align=center><img src="https://user-images.githubusercontent.com/43344034/232755081-cad8fe62-349d-400a-bc38-7f5d17824011.png" style=" width: auto; height: 40%; "></div>
|
||||
|
||||
## 分析工具
|
||||
|
||||
### 离线评测工具
|
||||
|
||||
对于已保存的预测结果,我们提供了离线评测脚本 `tools/analysis_tools/offline_eval.py`。例如,以下代码演示了如何使用该工具对 "PSENet" 模型的输出结果进行离线评估:
|
||||
|
|
|
@ -13,11 +13,11 @@ except ImportError:
|
|||
from .version import __version__, short_version
|
||||
|
||||
mmcv_minimum_version = '2.0.0rc4'
|
||||
mmcv_maximum_version = '2.1.0'
|
||||
mmcv_maximum_version = '2.2.0'
|
||||
mmcv_version = digit_version(mmcv.__version__)
|
||||
if mmengine is not None:
|
||||
mmengine_minimum_version = '0.7.1'
|
||||
mmengine_maximum_version = '1.0.0'
|
||||
mmengine_maximum_version = '1.1.0'
|
||||
mmengine_version = digit_version(mmengine.__version__)
|
||||
|
||||
if not mmengine or mmcv_version < digit_version('2.0.0rc0') or digit_version(
|
||||
|
@ -43,7 +43,7 @@ assert (mmengine_version >= digit_version(mmengine_minimum_version)
|
|||
f'<{mmengine_maximum_version}.'
|
||||
|
||||
mmdet_minimum_version = '3.0.0rc5'
|
||||
mmdet_maximum_version = '3.1.0'
|
||||
mmdet_maximum_version = '3.4.0'
|
||||
mmdet_version = digit_version(mmdet.__version__)
|
||||
|
||||
assert (mmdet_version >= digit_version(mmdet_minimum_version)
|
||||
|
|
|
@ -80,7 +80,9 @@ class MMOCRInferencer(BaseMMOCRInferencer):
|
|||
type='TextSpottingLocalVisualizer',
|
||||
name=f'inferencer{ts}',
|
||||
font_families=self.textrec_inferencer.visualizer.
|
||||
font_families))
|
||||
font_families,
|
||||
font_properties=self.textrec_inferencer.visualizer.
|
||||
font_properties))
|
||||
else:
|
||||
self.mode = 'rec'
|
||||
if kie is not None:
|
||||
|
@ -398,7 +400,7 @@ class MMOCRInferencer(BaseMMOCRInferencer):
|
|||
pred_name = osp.splitext(osp.basename(img_path))[0]
|
||||
pred_name = f'{pred_name}.json'
|
||||
pred_out_file = osp.join(pred_out_dir, pred_name)
|
||||
mmengine.dump(pred_result, pred_out_file)
|
||||
mmengine.dump(pred_result, pred_out_file, ensure_ascii=False)
|
||||
|
||||
result_dict['predictions'] = pred_results
|
||||
if print_result:
|
||||
|
|
|
@ -21,4 +21,4 @@ class JsonDumper(BaseDumper):
|
|||
|
||||
filename = f'{self.task}_{self.split}.json'
|
||||
dst_file = osp.join(self.data_root, filename)
|
||||
mmengine.dump(data, dst_file)
|
||||
mmengine.dump(data, dst_file, ensure_ascii=False)
|
||||
|
|
|
@ -1,4 +1,5 @@
|
|||
# Copyright (c) OpenMMLab. All rights reserved.
|
||||
from .aws_s3_obtainer import AWSS3Obtainer
|
||||
from .naive_data_obtainer import NaiveDataObtainer
|
||||
|
||||
__all__ = ['NaiveDataObtainer']
|
||||
__all__ = ['NaiveDataObtainer', 'AWSS3Obtainer']
|
||||
|
|
|
@ -0,0 +1,122 @@
|
|||
# Copyright (c) OpenMMLab. All rights reserved.
|
||||
import os.path as osp
|
||||
import ssl
|
||||
from typing import Dict, List, Optional
|
||||
|
||||
from mmengine import mkdir_or_exist
|
||||
|
||||
from mmocr.registry import DATA_OBTAINERS
|
||||
from .naive_data_obtainer import NaiveDataObtainer
|
||||
|
||||
ssl._create_default_https_context = ssl._create_unverified_context
|
||||
|
||||
|
||||
@DATA_OBTAINERS.register_module()
|
||||
class AWSS3Obtainer(NaiveDataObtainer):
|
||||
"""A AWS S3 obtainer.
|
||||
|
||||
download -> extract -> move
|
||||
|
||||
Args:
|
||||
files (list[dict]): A list of file information.
|
||||
cache_path (str): The path to cache the downloaded files.
|
||||
data_root (str): The root path of the dataset. It is usually set auto-
|
||||
matically and users do not need to set it manually in config file
|
||||
in most cases.
|
||||
task (str): The task of the dataset. It is usually set automatically
|
||||
and users do not need to set it manually in config file
|
||||
in most cases.
|
||||
"""
|
||||
|
||||
def __init__(self, files: List[Dict], cache_path: str, data_root: str,
|
||||
task: str) -> None:
|
||||
try:
|
||||
import boto3
|
||||
from botocore import UNSIGNED
|
||||
from botocore.config import Config
|
||||
except ImportError:
|
||||
raise ImportError(
|
||||
'Please install boto3 to download hiertext dataset.')
|
||||
self.files = files
|
||||
self.cache_path = cache_path
|
||||
self.data_root = data_root
|
||||
self.task = task
|
||||
self.s3_client = boto3.client(
|
||||
's3', config=Config(signature_version=UNSIGNED))
|
||||
self.total_length = 0
|
||||
mkdir_or_exist(self.data_root)
|
||||
mkdir_or_exist(osp.join(self.data_root, f'{task}_imgs'))
|
||||
mkdir_or_exist(osp.join(self.data_root, 'annotations'))
|
||||
mkdir_or_exist(self.cache_path)
|
||||
|
||||
def find_bucket_key(self, s3_path: str):
|
||||
"""This is a helper function that given an s3 path such that the path
|
||||
is of the form: bucket/key It will return the bucket and the key
|
||||
represented by the s3 path.
|
||||
|
||||
Args:
|
||||
s3_path (str): The AWS s3 path.
|
||||
"""
|
||||
s3_components = s3_path.split('/', 1)
|
||||
bucket = s3_components[0]
|
||||
s3_key = ''
|
||||
if len(s3_components) > 1:
|
||||
s3_key = s3_components[1]
|
||||
return bucket, s3_key
|
||||
|
||||
def s3_download(self, s3_bucket: str, s3_object_key: str, dst_path: str):
|
||||
"""Download file from given s3 url with progress bar.
|
||||
|
||||
Args:
|
||||
s3_bucket (str): The s3 bucket to download the file.
|
||||
s3_object_key (str): The s3 object key to download the file.
|
||||
dst_path (str): The destination path to save the file.
|
||||
"""
|
||||
meta_data = self.s3_client.head_object(
|
||||
Bucket=s3_bucket, Key=s3_object_key)
|
||||
total_length = int(meta_data.get('ContentLength', 0))
|
||||
downloaded = 0
|
||||
|
||||
def progress(chunk):
|
||||
nonlocal downloaded
|
||||
downloaded += chunk
|
||||
percent = min(100. * downloaded / total_length, 100)
|
||||
file_name = osp.basename(dst_path)
|
||||
print(f'\rDownloading {file_name}: {percent:.2f}%', end='')
|
||||
|
||||
print(f'Downloading {dst_path}')
|
||||
self.s3_client.download_file(
|
||||
s3_bucket, s3_object_key, dst_path, Callback=progress)
|
||||
|
||||
def download(self, url: Optional[str], dst_path: str) -> None:
|
||||
"""Download file from given url with progress bar.
|
||||
|
||||
Args:
|
||||
url (str): The url to download the file.
|
||||
dst_path (str): The destination path to save the file.
|
||||
"""
|
||||
if url is None and not osp.exists(dst_path):
|
||||
raise FileNotFoundError(
|
||||
'Direct url is not available for this dataset.'
|
||||
' Please manually download the required files'
|
||||
' following the guides.')
|
||||
|
||||
if url.startswith('magnet'):
|
||||
raise NotImplementedError('Please use any BitTorrent client to '
|
||||
'download the following magnet link to '
|
||||
f'{osp.abspath(dst_path)} and '
|
||||
f'try again.\nLink: {url}')
|
||||
|
||||
print('Downloading...')
|
||||
print(f'URL: {url}')
|
||||
print(f'Destination: {osp.abspath(dst_path)}')
|
||||
print('If you stuck here for a long time, please check your network, '
|
||||
'or manually download the file to the destination path and '
|
||||
'run the script again.')
|
||||
if url.startswith('s3://'):
|
||||
url = url[5:]
|
||||
bucket, key = self.find_bucket_key(url)
|
||||
self.s3_download(bucket, key, osp.abspath(dst_path))
|
||||
elif url.startswith('https://') or url.startswith('http://'):
|
||||
super().download(url, dst_path)
|
||||
print('')
|
|
@ -138,6 +138,7 @@ class MMDetWrapper(BaseModel):
|
|||
# convert by text_repr_type
|
||||
if self.text_repr_type == 'quad':
|
||||
for j, poly in enumerate(filterd_polygons):
|
||||
poly = poly.reshape(-1, 2)
|
||||
rect = cv2.minAreaRect(poly)
|
||||
vertices = cv2.boxPoints(rect)
|
||||
poly = vertices.flatten()
|
||||
|
|
|
@ -1,4 +1,4 @@
|
|||
# Copyright (c) Open-MMLab. All rights reserved.
|
||||
|
||||
__version__ = '1.0.0'
|
||||
__version__ = '1.0.1'
|
||||
short_version = __version__
|
||||
|
|
|
@ -1,3 +1,3 @@
|
|||
mmcv>=2.0.0rc4,<2.1.0
|
||||
mmdet>=3.0.0rc5,<3.1.0
|
||||
mmengine>=0.7.0, <1.0.0
|
||||
mmcv>=2.0.0rc4,<2.2.0
|
||||
mmdet>=3.0.0rc5,<3.4.0
|
||||
mmengine>=0.7.0, <1.1.0
|
||||
|
|
|
@ -0,0 +1 @@
|
|||
boto3
|
|
@ -154,9 +154,9 @@ def generate_ann(root_path, split, image_infos, preserve_vertical):
|
|||
dst_image_root = osp.join(root_path, 'crops', split)
|
||||
ignore_image_root = osp.join(root_path, 'ignores', split)
|
||||
if split == 'training':
|
||||
dst_label_file = osp.join(root_path, f'train_label.{format}')
|
||||
dst_label_file = osp.join(root_path, 'train_label.json')
|
||||
elif split == 'val':
|
||||
dst_label_file = osp.join(root_path, f'val_label.{format}')
|
||||
dst_label_file = osp.join(root_path, 'val_label.json')
|
||||
mmengine.mkdir_or_exist(dst_image_root)
|
||||
mmengine.mkdir_or_exist(ignore_image_root)
|
||||
|
||||
|
|
|
@ -0,0 +1,286 @@
|
|||
# Copyright (c) OpenMMLab. All rights reserved.
|
||||
import argparse
|
||||
import json
|
||||
import os.path as osp
|
||||
import re
|
||||
from pathlib import Path
|
||||
from unittest.mock import MagicMock
|
||||
|
||||
import matplotlib.pyplot as plt
|
||||
import rich
|
||||
import torch.nn as nn
|
||||
from mmengine.config import Config, DictAction
|
||||
from mmengine.hooks import Hook
|
||||
from mmengine.model import BaseModel
|
||||
from mmengine.registry import init_default_scope
|
||||
from mmengine.runner import Runner
|
||||
from mmengine.visualization import Visualizer
|
||||
from rich.progress import BarColumn, MofNCompleteColumn, Progress, TextColumn
|
||||
|
||||
from mmocr.registry import DATASETS
|
||||
|
||||
|
||||
class SimpleModel(BaseModel):
|
||||
"""simple model that do nothing in train_step."""
|
||||
|
||||
def __init__(self):
|
||||
super(SimpleModel, self).__init__()
|
||||
self.data_preprocessor = nn.Identity()
|
||||
self.conv = nn.Conv2d(1, 1, 1)
|
||||
|
||||
def forward(self, inputs, data_samples, mode='tensor'):
|
||||
pass
|
||||
|
||||
def train_step(self, data, optim_wrapper):
|
||||
pass
|
||||
|
||||
|
||||
class ParamRecordHook(Hook):
|
||||
|
||||
def __init__(self, by_epoch):
|
||||
super().__init__()
|
||||
self.by_epoch = by_epoch
|
||||
self.lr_list = []
|
||||
self.momentum_list = []
|
||||
self.wd_list = []
|
||||
self.task_id = 0
|
||||
self.progress = Progress(BarColumn(), MofNCompleteColumn(),
|
||||
TextColumn('{task.description}'))
|
||||
|
||||
def before_train(self, runner):
|
||||
if self.by_epoch:
|
||||
total = runner.train_loop.max_epochs
|
||||
self.task_id = self.progress.add_task(
|
||||
'epochs', start=True, total=total)
|
||||
else:
|
||||
total = runner.train_loop.max_iters
|
||||
self.task_id = self.progress.add_task(
|
||||
'iters', start=True, total=total)
|
||||
self.progress.start()
|
||||
|
||||
def after_train_epoch(self, runner):
|
||||
if self.by_epoch:
|
||||
self.progress.update(self.task_id, advance=1)
|
||||
|
||||
def after_train_iter(self, runner, batch_idx, data_batch, outputs):
|
||||
if not self.by_epoch:
|
||||
self.progress.update(self.task_id, advance=1)
|
||||
self.lr_list.append(runner.optim_wrapper.get_lr()['lr'][0])
|
||||
self.momentum_list.append(
|
||||
runner.optim_wrapper.get_momentum()['momentum'][0])
|
||||
self.wd_list.append(
|
||||
runner.optim_wrapper.param_groups[0]['weight_decay'])
|
||||
|
||||
def after_train(self, runner):
|
||||
self.progress.stop()
|
||||
|
||||
|
||||
def parse_args():
|
||||
parser = argparse.ArgumentParser(
|
||||
description='Visualize a Dataset Pipeline')
|
||||
parser.add_argument('config', help='config file path')
|
||||
parser.add_argument(
|
||||
'-p',
|
||||
'--parameter',
|
||||
type=str,
|
||||
default='lr',
|
||||
choices=['lr', 'momentum', 'wd'],
|
||||
help='The parameter to visualize its change curve, choose from'
|
||||
'"lr", "wd" and "momentum". Defaults to "lr".')
|
||||
parser.add_argument(
|
||||
'-d',
|
||||
'--dataset-size',
|
||||
type=int,
|
||||
help='The size of the dataset. If specify, `build_dataset` will '
|
||||
'be skipped and use this size as the dataset size.')
|
||||
parser.add_argument(
|
||||
'-n',
|
||||
'--ngpus',
|
||||
type=int,
|
||||
default=1,
|
||||
help='The number of GPUs used in training.')
|
||||
parser.add_argument(
|
||||
'-s',
|
||||
'--save-path',
|
||||
type=Path,
|
||||
help='The learning rate curve plot save path')
|
||||
parser.add_argument(
|
||||
'--log-level',
|
||||
default='WARNING',
|
||||
help='The log level of the handler and logger. Defaults to '
|
||||
'WARNING.')
|
||||
parser.add_argument('--title', type=str, help='title of figure')
|
||||
parser.add_argument(
|
||||
'--style', type=str, default='whitegrid', help='style of plt')
|
||||
parser.add_argument('--not-show', default=False, action='store_true')
|
||||
parser.add_argument(
|
||||
'--window-size',
|
||||
default='12*7',
|
||||
help='Size of the window to display images, in format of "$W*$H".')
|
||||
parser.add_argument(
|
||||
'--cfg-options',
|
||||
nargs='+',
|
||||
action=DictAction,
|
||||
help='override some settings in the used config, the key-value pair '
|
||||
'in xxx=yyy format will be merged into config file. If the value to '
|
||||
'be overwritten is a list, it should be like key="[a,b]" or key=a,b '
|
||||
'It also allows nested list/tuple values, e.g. key="[(a,b),(c,d)]" '
|
||||
'Note that the quotation marks are necessary and that no white space '
|
||||
'is allowed.')
|
||||
args = parser.parse_args()
|
||||
if args.window_size != '':
|
||||
assert re.match(r'\d+\*\d+', args.window_size), \
|
||||
"'window-size' must be in format 'W*H'."
|
||||
|
||||
return args
|
||||
|
||||
|
||||
def plot_curve(lr_list, args, param_name, iters_per_epoch, by_epoch=True):
|
||||
"""Plot learning rate vs iter graph."""
|
||||
try:
|
||||
import seaborn as sns
|
||||
sns.set_style(args.style)
|
||||
except ImportError:
|
||||
pass
|
||||
|
||||
wind_w, wind_h = args.window_size.split('*')
|
||||
wind_w, wind_h = int(wind_w), int(wind_h)
|
||||
plt.figure(figsize=(wind_w, wind_h))
|
||||
|
||||
ax: plt.Axes = plt.subplot()
|
||||
ax.plot(lr_list, linewidth=1)
|
||||
|
||||
if by_epoch:
|
||||
ax.xaxis.tick_top()
|
||||
ax.set_xlabel('Iters')
|
||||
ax.xaxis.set_label_position('top')
|
||||
sec_ax = ax.secondary_xaxis(
|
||||
'bottom',
|
||||
functions=(lambda x: x / iters_per_epoch,
|
||||
lambda y: y * iters_per_epoch))
|
||||
sec_ax.set_xlabel('Epochs')
|
||||
else:
|
||||
plt.xlabel('Iters')
|
||||
plt.ylabel(param_name)
|
||||
|
||||
if args.title is None:
|
||||
plt.title(f'{osp.basename(args.config)} {param_name} curve')
|
||||
else:
|
||||
plt.title(args.title)
|
||||
|
||||
|
||||
def simulate_train(data_loader, cfg, by_epoch):
|
||||
model = SimpleModel()
|
||||
param_record_hook = ParamRecordHook(by_epoch=by_epoch)
|
||||
default_hooks = dict(
|
||||
param_scheduler=cfg.default_hooks['param_scheduler'],
|
||||
runtime_info=None,
|
||||
timer=None,
|
||||
logger=None,
|
||||
checkpoint=None,
|
||||
sampler_seed=None,
|
||||
param_record=param_record_hook)
|
||||
|
||||
runner = Runner(
|
||||
model=model,
|
||||
work_dir=cfg.work_dir,
|
||||
train_dataloader=data_loader,
|
||||
train_cfg=cfg.train_cfg,
|
||||
log_level=cfg.log_level,
|
||||
optim_wrapper=cfg.optim_wrapper,
|
||||
param_scheduler=cfg.param_scheduler,
|
||||
default_scope=cfg.default_scope,
|
||||
default_hooks=default_hooks,
|
||||
visualizer=MagicMock(spec=Visualizer),
|
||||
custom_hooks=cfg.get('custom_hooks', None))
|
||||
|
||||
runner.train()
|
||||
|
||||
param_dict = dict(
|
||||
lr=param_record_hook.lr_list,
|
||||
momentum=param_record_hook.momentum_list,
|
||||
wd=param_record_hook.wd_list)
|
||||
|
||||
return param_dict
|
||||
|
||||
|
||||
def build_dataset(cfg):
|
||||
return DATASETS.build(cfg)
|
||||
|
||||
|
||||
def main():
|
||||
args = parse_args()
|
||||
cfg = Config.fromfile(args.config)
|
||||
|
||||
init_default_scope(cfg.get('default_scope', 'mmocr'))
|
||||
|
||||
if args.cfg_options is not None:
|
||||
cfg.merge_from_dict(args.cfg_options)
|
||||
if cfg.get('work_dir', None) is None:
|
||||
# use config filename as default work_dir if cfg.work_dir is None
|
||||
cfg.work_dir = osp.join('./work_dirs',
|
||||
osp.splitext(osp.basename(args.config))[0])
|
||||
|
||||
cfg.log_level = args.log_level
|
||||
|
||||
# make sure save_root exists
|
||||
if args.save_path and not args.save_path.parent.exists():
|
||||
raise FileNotFoundError(
|
||||
f'The save path is {args.save_path}, and directory '
|
||||
f"'{args.save_path.parent}' do not exist.")
|
||||
|
||||
# init logger
|
||||
print('Param_scheduler :')
|
||||
rich.print_json(json.dumps(cfg.param_scheduler))
|
||||
|
||||
# prepare data loader
|
||||
batch_size = cfg.train_dataloader.batch_size * args.ngpus
|
||||
|
||||
if 'by_epoch' in cfg.train_cfg:
|
||||
by_epoch = cfg.train_cfg.get('by_epoch')
|
||||
elif 'type' in cfg.train_cfg:
|
||||
by_epoch = cfg.train_cfg.get('type') == 'EpochBasedTrainLoop'
|
||||
else:
|
||||
raise ValueError('please set `train_cfg`.')
|
||||
|
||||
if args.dataset_size is None and by_epoch:
|
||||
dataset_size = len(build_dataset(cfg.train_dataloader.dataset))
|
||||
else:
|
||||
dataset_size = args.dataset_size or batch_size
|
||||
|
||||
class FakeDataloader(list):
|
||||
dataset = MagicMock(metainfo=None)
|
||||
|
||||
data_loader = FakeDataloader(range(dataset_size // batch_size))
|
||||
dataset_info = (
|
||||
f'\nDataset infos:'
|
||||
f'\n - Dataset size: {dataset_size}'
|
||||
f'\n - Batch size per GPU: {cfg.train_dataloader.batch_size}'
|
||||
f'\n - Number of GPUs: {args.ngpus}'
|
||||
f'\n - Total batch size: {batch_size}')
|
||||
if by_epoch:
|
||||
dataset_info += f'\n - Iterations per epoch: {len(data_loader)}'
|
||||
rich.print(dataset_info + '\n')
|
||||
|
||||
# simulation training process
|
||||
param_dict = simulate_train(data_loader, cfg, by_epoch)
|
||||
param_list = param_dict[args.parameter]
|
||||
|
||||
if args.parameter == 'lr':
|
||||
param_name = 'Learning Rate'
|
||||
elif args.parameter == 'momentum':
|
||||
param_name = 'Momentum'
|
||||
else:
|
||||
param_name = 'Weight Decay'
|
||||
plot_curve(param_list, args, param_name, len(data_loader), by_epoch)
|
||||
|
||||
if args.save_path:
|
||||
plt.savefig(args.save_path)
|
||||
print(f'\nThe {param_name} graph is saved at {args.save_path}')
|
||||
|
||||
if not args.not_show:
|
||||
plt.show()
|
||||
|
||||
|
||||
if __name__ == '__main__':
|
||||
main()
|
Loading…
Reference in New Issue