[](https://pypi.org/project/mmpretrain)
[](https://mmpretrain.readthedocs.io/zh_CN/latest/)
[](https://github.com/open-mmlab/mmpretrain/actions)
[](https://codecov.io/gh/open-mmlab/mmpretrain)
[](https://github.com/open-mmlab/mmpretrain/blob/main/LICENSE)
[](https://github.com/open-mmlab/mmpretrain/issues)
[](https://github.com/open-mmlab/mmpretrain/issues)
[📘 中文文档](https://mmpretrain.readthedocs.io/zh_CN/latest/) |
[🛠️ 安装教程](https://mmpretrain.readthedocs.io/zh_CN/latest/get_started.html) |
[👀 模型库](https://mmpretrain.readthedocs.io/zh_CN/latest/modelzoo_statistics.html) |
[🆕 更新日志](https://mmpretrain.readthedocs.io/zh_CN/latest/notes/changelog.html) |
[🤔 报告问题](https://github.com/open-mmlab/mmpretrain/issues/new/choose)
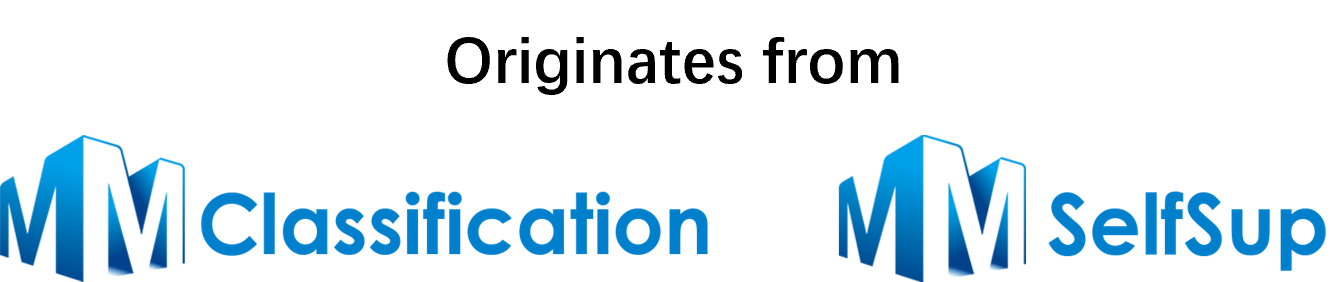
[English](/README.md) | 简体中文