* [Feat] Migrate blip caption to mmpretrain. (#50) * Migrate blip caption to mmpretrain * minor fix * support train * [Feature] Support OFA caption task. (#51) * [Feature] Support OFA caption task. * Remove duplicated files. * [Feature] Support OFA vqa task. (#58) * [Feature] Support OFA vqa task. * Fix lint. * [Feat] Add BLIP retrieval to mmpretrain. (#55) * init * minor fix for train * fix according to comments * refactor * Update Blip retrieval. (#62) * [Feature] Support OFA visual grounding task. (#59) * [Feature] Support OFA visual grounding task. * minor add TODO --------- Co-authored-by: yingfhu <yingfhu@gmail.com> * [Feat] Add flamingos coco caption and vqa. (#60) * first init * init flamingo coco * add vqa * minor fix * remove unnecessary modules * Update config * Use `ApplyToList`. --------- Co-authored-by: mzr1996 <mzr1996@163.com> * [Feature]: BLIP2 coco retrieval (#53) * [Feature]: Add blip2 retriever * [Feature]: Add blip2 all modules * [Feature]: Refine model * [Feature]: x1 * [Feature]: Runnable coco ret * [Feature]: Runnable version * [Feature]: Fix lint * [Fix]: Fix lint * [Feature]: Use 364 img size * [Feature]: Refactor blip2 * [Fix]: Fix lint * refactor files * minor fix * minor fix --------- Co-authored-by: yingfhu <yingfhu@gmail.com> * Remove * fix blip caption inputs (#68) * [Feat] Add BLIP NLVR support. (#67) * first init * init flamingo coco * add vqa * add nlvr * refactor nlvr * minor fix * minor fix * Update dataset --------- Co-authored-by: mzr1996 <mzr1996@163.com> * [Feature]: BLIP2 Caption (#70) * [Feature]: Add language model * [Feature]: blip2 caption forward * [Feature]: Reproduce the results * [Feature]: Refactor caption * refine config --------- Co-authored-by: yingfhu <yingfhu@gmail.com> * [Feat] Migrate BLIP VQA to mmpretrain (#69) * reformat * change * change * change * change * change * change * change * change * change * change * change * change * change * change * change * change * change * change * change * refactor code --------- Co-authored-by: yingfhu <yingfhu@gmail.com> * Update RefCOCO dataset * [Fix] fix lint * [Feature] Implement inference APIs for multi-modal tasks. (#65) * [Feature] Implement inference APIs for multi-modal tasks. * [Project] Add gradio demo. * [Improve] Update requirements * Update flamingo * Update blip * Add NLVR inferencer * Update flamingo * Update hugging face model register * Update ofa vqa * Update BLIP-vqa (#71) * Update blip-vqa docstring (#72) * Refine flamingo docstring (#73) * [Feature]: BLIP2 VQA (#61) * [Feature]: VQA forward * [Feature]: Reproduce accuracy * [Fix]: Fix lint * [Fix]: Add blank line * minor fix --------- Co-authored-by: yingfhu <yingfhu@gmail.com> * [Feature]: BLIP2 docstring (#74) * [Feature]: Add caption docstring * [Feature]: Add docstring to blip2 vqa * [Feature]: Add docstring to retrieval * Update BLIP-2 metafile and README (#75) * [Feature]: Add readme and docstring * Update blip2 results --------- Co-authored-by: mzr1996 <mzr1996@163.com> * [Feature] BLIP Visual Grounding on MMPretrain Branch (#66) * blip grounding merge with mmpretrain * remove commit * blip grounding test and inference api * refcoco dataset * refcoco dataset refine config * rebasing * gitignore * rebasing * minor edit * minor edit * Update blip-vqa docstring (#72) * rebasing * Revert "minor edit" This reverts commit 639cec757c215e654625ed0979319e60f0be9044. * blip grounding final * precommit * refine config * refine config * Update blip visual grounding --------- Co-authored-by: Yiqin Wang 王逸钦 <wyq1217@outlook.com> Co-authored-by: mzr1996 <mzr1996@163.com> * Update visual grounding metric * Update OFA docstring, README and metafiles. (#76) * [Docs] Update installation docs and gradio demo docs. (#77) * Update OFA name * Update Visual Grounding Visualizer * Integrate accelerate support * Fix imports. * Fix timm backbone * Update imports * Update README * Update circle ci * Update flamingo config * Add gradio demo README * [Feature]: Add scienceqa (#1571) * [Feature]: Add scienceqa * [Feature]: Change param name * Update docs * Update video --------- Co-authored-by: Hubert <42952108+yingfhu@users.noreply.github.com> Co-authored-by: yingfhu <yingfhu@gmail.com> Co-authored-by: Yuan Liu <30762564+YuanLiuuuuuu@users.noreply.github.com> Co-authored-by: Yiqin Wang 王逸钦 <wyq1217@outlook.com> Co-authored-by: Rongjie Li <limo97@163.com> |
||
---|---|---|
.. | ||
README.md | ||
metafile.yml | ||
ofa-base_finetuned_caption.py | ||
ofa-base_finetuned_refcoco.py | ||
ofa-base_finetuned_vqa.py | ||
ofa-base_zeroshot_vqa.py | ||
ofa-large_zeroshot_vqa.py |
README.md
OFA
Abstract
In this work, we pursue a unified paradigm for multimodal pretraining to break the scaffolds of complex task/modality-specific customization. We propose OFA, a Task-Agnostic and Modality-Agnostic framework that supports Task Comprehensiveness. OFA unifies a diverse set of cross-modal and unimodal tasks, including image generation, visual grounding, image captioning, image classification, language modeling, etc., in a simple sequence-to-sequence learning framework. OFA follows the instruction-based learning in both pretraining and finetuning stages, requiring no extra task-specific layers for downstream tasks. In comparison with the recent state-of-the-art vision & language models that rely on extremely large cross-modal datasets, OFA is pretrained on only 20M publicly available image-text pairs. Despite its simplicity and relatively small-scale training data, OFA achieves new SOTAs in a series of cross-modal tasks while attaining highly competitive performances on uni-modal tasks. Our further analysis indicates that OFA can also effectively transfer to unseen tasks and unseen domains.
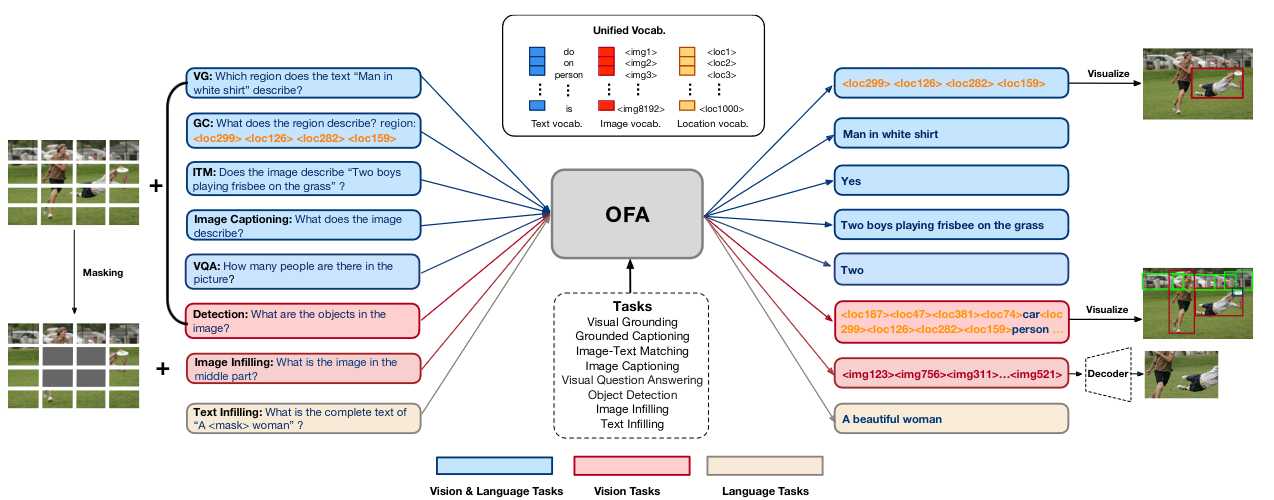
How to use it?
Use the model
from mmpretrain import inference_model
result = inference_model('ofa-base_3rdparty-finetuned_caption', 'demo/cat-dog.png')
print(result)
# {'pred_caption': 'a dog and a kitten sitting next to each other'}
Test Command
Prepare your dataset according to the docs.
Test:
python tools/test.py configs/ofa/ofa-base_finetuned_refcoco.py https://download.openmmlab.com/mmclassification/v1/ofa/ofa-base_3rdparty_refcoco_20230418-2797d3ab.pth
Models and results
Image Caption on COCO
Model | Params (M) | BLEU-4 | CIDER | Config | Download |
---|---|---|---|---|---|
ofa-base_3rdparty-finetuned_caption * |
182.24 | 42.64 | 144.50 | config | model |
Models with * are converted from the official repo. The config files of these models are only for inference. We haven't reprodcue the training results.
Visual Grounding on RefCOCO
Model | Params (M) | Accuracy (testA) | Accuracy (testB) | Config | Download |
---|---|---|---|---|---|
ofa-base_3rdparty-finetuned_refcoco * |
182.24 | 90.49 | 83.63 | config | model |
Models with * are converted from the official repo. The config files of these models are only for inference. We haven't reprodcue the training results.
Visual Question Answering on VQAv2
Model | Params (M) | Accuracy | Config | Download |
---|---|---|---|---|
ofa-base_3rdparty-finetuned_vqa * |
182.24 | 78.00 | config | model |
ofa-base_3rdparty-zeroshot_vqa * |
182.24 | 58.32 | config | model |
Models with * are converted from the official repo. The config files of these models are only for inference. We haven't reprodcue the training results.
Citation
@article{wang2022ofa,
author = {Peng Wang and
An Yang and
Rui Men and
Junyang Lin and
Shuai Bai and
Zhikang Li and
Jianxin Ma and
Chang Zhou and
Jingren Zhou and
Hongxia Yang},
title = {OFA: Unifying Architectures, Tasks, and Modalities Through a Simple Sequence-to-Sequence
Learning Framework},
journal = {CoRR},
volume = {abs/2202.03052},
year = {2022}
}