218 lines
7.3 KiB
Markdown
218 lines
7.3 KiB
Markdown
# Delivery
|
|
|
|
## Introduction of Delivery
|
|
|
|
`Delivery` is a mechanism used in **knowledge distillation**, which is to **align the intermediate results** between the teacher model and the student model by delivering and rewriting these intermediate results between them. As shown in the figure below, deliveries can be used to:
|
|
|
|
- **Deliver the output of a layer of the teacher model directly to a layer of the student model.** In some knowledge distillation algorithms, we may need to deliver the output of a layer of the teacher model to the student model directly. For example, in [LAD](https://arxiv.org/abs/2108.10520) algorithm, the student model needs to obtain the label assignment of the teacher model directly.
|
|
- **Align the inputs of the teacher model and the student model.** For example, in the MMClassification framework, some widely used data augmentations such as [mixup](https://arxiv.org/abs/1710.09412) and [CutMix](https://arxiv.org/abs/1905.04899) are not implemented in Data Pipelines but in `forward_train`, and due to the randomness of these data augmentation methods, it may lead to a gap between the input of the teacher model and the student model.
|
|
|
|
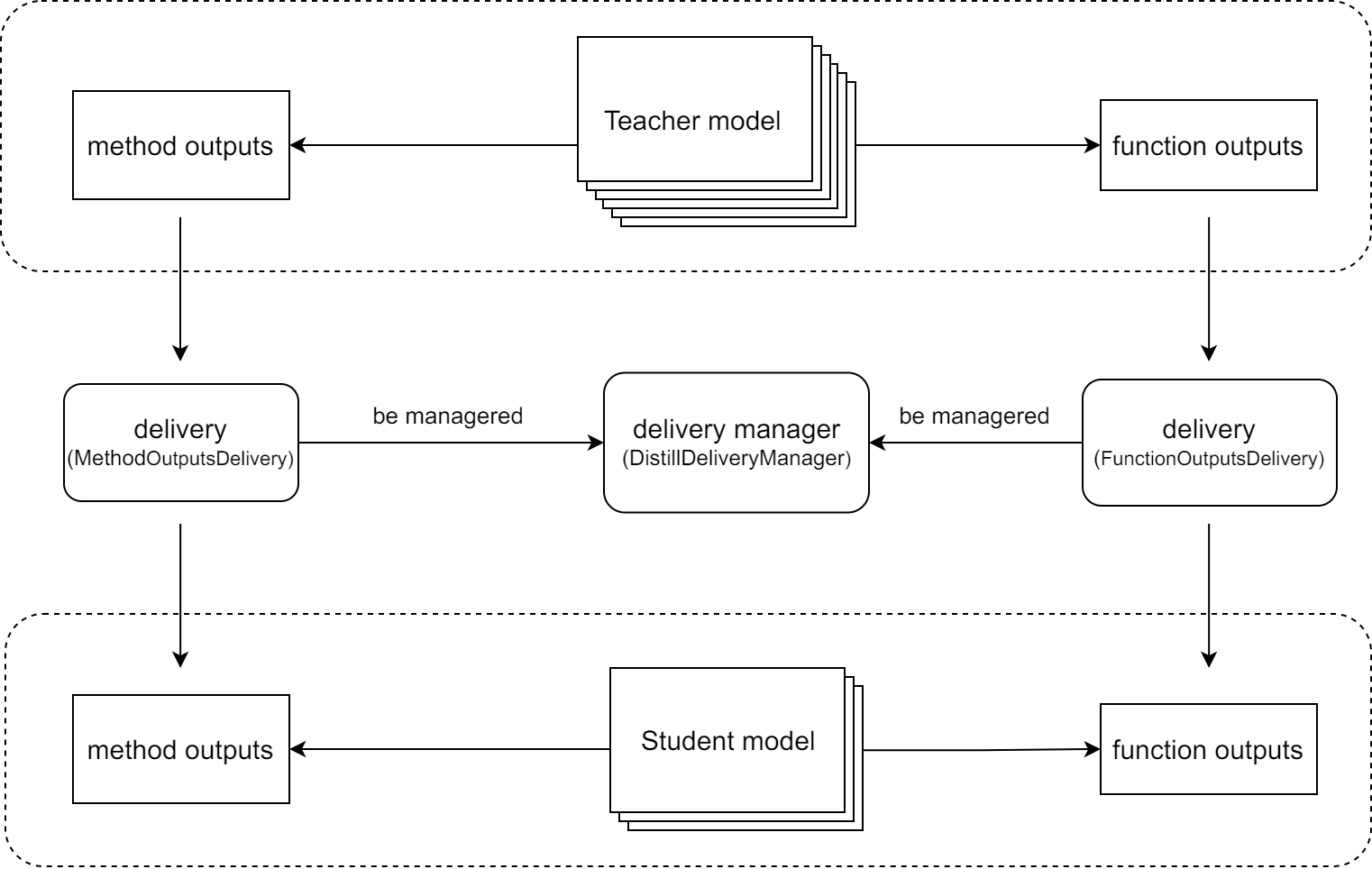
|
|
|
|
In general, the delivery mechanism allows us to deliver intermediate results between the teacher model and the student model **without adding additional code**, which reduces the hard coding in the source code.
|
|
|
|
## Usage of Delivery
|
|
|
|
Currently, we support two deliveries: `FunctionOutputsDelivery` and `MethodOutputsDelivery`, both of which inherit from `DistillDiliver`. And these deliveries can be managed by `DistillDeliveryManager` or just be used on their own.
|
|
|
|
Their relationship is shown below.
|
|
|
|
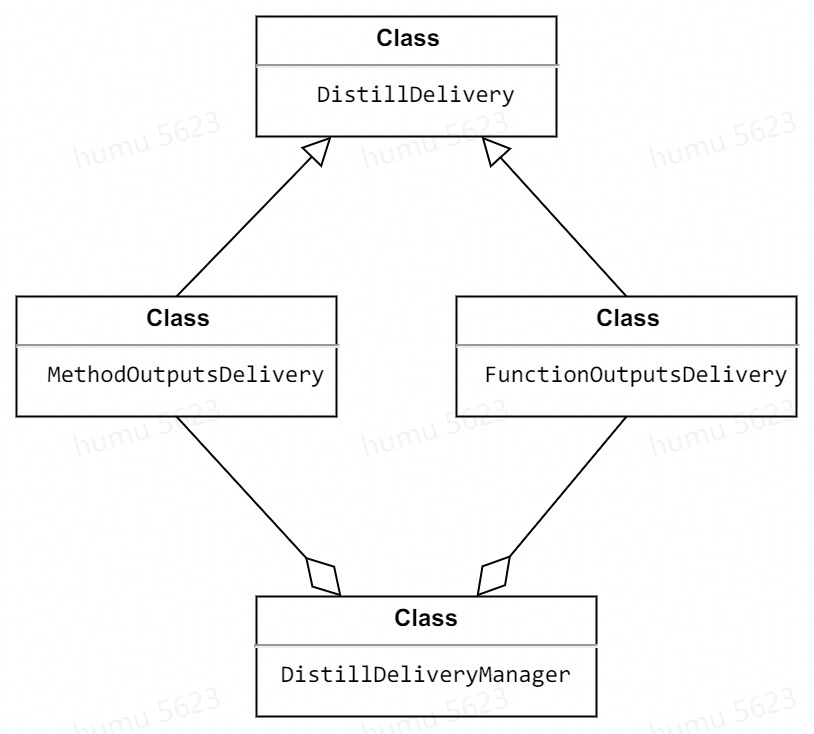
|
|
|
|
### FunctionOutputsDelivery
|
|
|
|
`FunctionOutputsDelivery` is used to align the **function's** intermediate results between the teacher model and the student model.
|
|
|
|
```{note}
|
|
When initializing `FunctionOutputsDelivery`, you need to pass `func_path` argument, which requires extra attention. For example,
|
|
`anchor_inside_flags` is a function in mmdetection to check whether the
|
|
anchors are inside the border. This function is in
|
|
`mmdet/core/anchor/utils.py` and used in
|
|
`mmdet/models/dense_heads/anchor_head`. Then the `func_path` should be
|
|
`mmdet.models.dense_heads.anchor_head.anchor_inside_flags` but not
|
|
`mmdet.core.anchor.utils.anchor_inside_flags`.
|
|
```
|
|
|
|
#### Case 1: Delivery single function's output from the teacher to the student.
|
|
|
|
```Python
|
|
import random
|
|
from mmrazor.core import FunctionOutputsDelivery
|
|
|
|
def toy_func() -> int:
|
|
return random.randint(0, 1000000)
|
|
|
|
delivery = FunctionOutputsDelivery(max_keep_data=1, func_path='toy_module.toy_func')
|
|
|
|
# override_data is False, which means that not override the data with
|
|
# the recorded data. So it will get the original output of toy_func
|
|
# in teacher model, and it is also recorded to be deliveried to the student.
|
|
delivery.override_data = False
|
|
with delivery:
|
|
output_teacher = toy_module.toy_func()
|
|
|
|
# override_data is True, which means that override the data with
|
|
# the recorded data, so it will get the output of toy_func
|
|
# in teacher model rather than the student's.
|
|
delivery.override_data = True
|
|
with delivery:
|
|
output_student = toy_module.toy_func()
|
|
|
|
print(output_teacher == output_student)
|
|
```
|
|
|
|
Out:
|
|
|
|
```Python
|
|
True
|
|
```
|
|
|
|
#### Case 2: Delivery multi function's outputs from the teacher to the student.
|
|
|
|
If a function is executed more than once during the forward of the teacher model, all the outputs of this function will be used to override function outputs from the student model
|
|
|
|
```{note}
|
|
Delivery order is first-in first-out.
|
|
```
|
|
|
|
```Python
|
|
delivery = FunctionOutputsDelivery(
|
|
max_keep_data=2, func_path='toy_module.toy_func')
|
|
|
|
delivery.override_data = False
|
|
with delivery:
|
|
output1_teacher = toy_module.toy_func()
|
|
output2_teacher = toy_module.toy_func()
|
|
|
|
delivery.override_data = True
|
|
with delivery:
|
|
output1_student = toy_module.toy_func()
|
|
output2_student = toy_module.toy_func()
|
|
|
|
print(output1_teacher == output1_student and output2_teacher == output2_student)
|
|
```
|
|
|
|
Out:
|
|
|
|
```Python
|
|
True
|
|
```
|
|
|
|
### MethodOutputsDelivery
|
|
|
|
`MethodOutputsDelivery` is used to align the **method's** intermediate results between the teacher model and the student model.
|
|
|
|
#### Case: Align the inputs of the teacher model and the student model
|
|
|
|
Here we use mixup as an example to show how to align the inputs of the teacher model and the student model.
|
|
|
|
- Without Delivery
|
|
|
|
```Python
|
|
# main.py
|
|
from mmcls.models.utils import Augments
|
|
from mmrazor.core import MethodOutputsDelivery
|
|
|
|
augments_cfg = dict(type='BatchMixup', alpha=1., num_classes=10, prob=1.0)
|
|
augments = Augments(augments_cfg)
|
|
|
|
imgs = torch.randn(2, 3, 32, 32)
|
|
label = torch.randint(0, 10, (2,))
|
|
|
|
imgs_teacher, label_teacher = augments(imgs, label)
|
|
imgs_student, label_student = augments(imgs, label)
|
|
|
|
print(torch.equal(label_teacher, label_student))
|
|
print(torch.equal(imgs_teacher, imgs_student))
|
|
```
|
|
|
|
Out:
|
|
|
|
```Python
|
|
False
|
|
False
|
|
from mmcls.models.utils import Augments
|
|
from mmrazor.core import DistillDeliveryManager
|
|
```
|
|
|
|
The results are different due to the randomness of mixup.
|
|
|
|
- With Delivery
|
|
|
|
```Python
|
|
delivery = MethodOutputsDelivery(
|
|
max_keep_data=1, method_path='mmcls.models.utils.Augments.__call__')
|
|
|
|
delivery.override_data = False
|
|
with delivery:
|
|
imgs_teacher, label_teacher = augments(imgs, label)
|
|
|
|
delivery.override_data = True
|
|
with delivery:
|
|
imgs_student, label_student = augments(imgs, label)
|
|
|
|
print(torch.equal(label_teacher, label_student))
|
|
print(torch.equal(imgs_teacher, imgs_student))
|
|
```
|
|
|
|
Out:
|
|
|
|
```Python
|
|
True
|
|
True
|
|
```
|
|
|
|
The randomness is eliminated by using `MethodOutputsDelivery`.
|
|
|
|
### 2.3 DistillDeliveryManager
|
|
|
|
`DistillDeliveryManager` is actually a context manager, used to manage delivers. When entering the ` DistillDeliveryManager`, all delivers managed will be started.
|
|
|
|
With the help of `DistillDeliveryManager`, we are able to manage several different DistillDeliveries with as little code as possible, thereby reducing the possibility of errors.
|
|
|
|
#### Case: Manager deliveries with DistillDeliveryManager
|
|
|
|
```Python
|
|
from mmcls.models.utils import Augments
|
|
from mmrazor.core import DistillDeliveryManager
|
|
|
|
augments_cfg = dict(type='BatchMixup', alpha=1., num_classes=10, prob=1.0)
|
|
augments = Augments(augments_cfg)
|
|
|
|
distill_deliveries = [
|
|
ConfigDict(type='MethodOutputs', max_keep_data=1,
|
|
method_path='mmcls.models.utils.Augments.__call__')]
|
|
|
|
# instantiate DistillDeliveryManager
|
|
manager = DistillDeliveryManager(distill_deliveries)
|
|
|
|
imgs = torch.randn(2, 3, 32, 32)
|
|
label = torch.randint(0, 10, (2,))
|
|
|
|
manager.override_data = False
|
|
with manager:
|
|
imgs_teacher, label_teacher = augments(imgs, label)
|
|
|
|
manager.override_data = True
|
|
with manager:
|
|
imgs_student, label_student = augments(imgs, label)
|
|
|
|
print(torch.equal(label_teacher, label_student))
|
|
print(torch.equal(imgs_teacher, imgs_student))
|
|
```
|
|
|
|
Out:
|
|
|
|
```Python
|
|
True
|
|
True
|
|
```
|
|
|
|
## Reference
|
|
|
|
\[1\] Zhang, Hongyi, et al. "mixup: Beyond empirical risk minimization." *arXiv* abs/1710.09412 (2017).
|
|
|
|
\[2\] Yun, Sangdoo, et al. "Cutmix: Regularization strategy to train strong classifiers with localizable features." *ICCV* (2019).
|
|
|
|
\[3\] Nguyen, Chuong H., et al. "Improving object detection by label assignment distillation." *WACV* (2022).
|