## Motivation The DETR-related modules have been refactored in open-mmlab/mmdetection#8763, which causes breakings of MaskFormer and Mask2Former in both MMDetection (has been fixed in open-mmlab/mmdetection#9515) and MMSegmentation. This pr fix the bugs in MMSegmentation. ### TO-DO List - [x] update configs - [x] check and modify data flow - [x] fix unit test - [x] aligning inference - [x] write a ckpt converter - [x] write ckpt update script - [x] update model zoo - [x] update model link in readme - [x] update [faq.md](https://github.com/open-mmlab/mmsegmentation/blob/dev-1.x/docs/en/notes/faq.md#installation) ## Tips of Fixing other implementations based on MaskXFormer of mmseg 1. The Transformer modules should be built directly. The original building with register manner has been refactored. 2. The config requires to be modified. Delete `type` and modify several keys, according to the modifications in this pr. 3. The `batch_first` is set `True` uniformly in the new implementations. Hence the data flow requires to be transposed and config of `batch_first` needs to be modified. 4. The checkpoint trained on the old implementation should be converted to be used in the new one. ### Convert script ```Python import argparse from copy import deepcopy from collections import OrderedDict import torch from mmengine.config import Config from mmseg.models import build_segmentor from mmseg.utils import register_all_modules register_all_modules(init_default_scope=True) def parse_args(): parser = argparse.ArgumentParser( description='MMSeg convert MaskXFormer model, by Li-Qingyun') parser.add_argument('Mask_what_former', type=int, help='Mask what former, can be a `1` or `2`', choices=[1, 2]) parser.add_argument('CFG_FILE', help='config file path') parser.add_argument('OLD_CKPT_FILEPATH', help='old ckpt file path') parser.add_argument('NEW_CKPT_FILEPATH', help='new ckpt file path') args = parser.parse_args() return args args = parse_args() def get_new_name(old_name: str): new_name = old_name if 'encoder.layers' in new_name: new_name = new_name.replace('attentions.0', 'self_attn') new_name = new_name.replace('ffns.0', 'ffn') if 'decoder.layers' in new_name: if args.Mask_what_former == 2: # for Mask2Former new_name = new_name.replace('attentions.0', 'cross_attn') new_name = new_name.replace('attentions.1', 'self_attn') else: # for Mask2Former new_name = new_name.replace('attentions.0', 'self_attn') new_name = new_name.replace('attentions.1', 'cross_attn') return new_name def cvt_sd(old_sd: OrderedDict): new_sd = OrderedDict() for name, param in old_sd.items(): new_name = get_new_name(name) assert new_name not in new_sd new_sd[new_name] = param assert len(new_sd) == len(old_sd) return new_sd if __name__ == '__main__': cfg = Config.fromfile(args.CFG_FILE) model_cfg = cfg.model segmentor = build_segmentor(model_cfg) refer_sd = segmentor.state_dict() old_ckpt = torch.load(args.OLD_CKPT_FILEPATH) old_sd = old_ckpt['state_dict'] new_sd = cvt_sd(old_sd) print(segmentor.load_state_dict(new_sd)) new_ckpt = deepcopy(old_ckpt) new_ckpt['state_dict'] = new_sd torch.save(new_ckpt, args.NEW_CKPT_FILEPATH) print(f'{args.NEW_CKPT_FILEPATH} has been saved!') ``` Usage: ```bash # for example python ckpt4pr2532.py 1 configs/maskformer/maskformer_r50-d32_8xb2-160k_ade20k-512x512.py original_ckpts/maskformer_r50-d32_8xb2-160k_ade20k-512x512_20221030_182724-cbd39cc1.pth cvt_outputs/maskformer_r50-d32_8xb2-160k_ade20k-512x512_20221030_182724.pth python ckpt4pr2532.py 2 configs/mask2former/mask2former_r50_8xb2-160k_ade20k-512x512.py original_ckpts/mask2former_r50_8xb2-160k_ade20k-512x512_20221204_000055-4c62652d.pth cvt_outputs/mask2former_r50_8xb2-160k_ade20k-512x512_20221204_000055.pth ``` --------- Co-authored-by: MeowZheng <meowzheng@outlook.com> |
||
---|---|---|
.. | ||
README.md | ||
maskformer.yml | ||
maskformer_r50-d32_8xb2-160k_ade20k-512x512.py | ||
maskformer_r101-d32_8xb2-160k_ade20k-512x512.py | ||
maskformer_swin-s_upernet_8xb2-160k_ade20k-512x512.py | ||
maskformer_swin-t_upernet_8xb2-160k_ade20k-512x512.py |
README.md
MaskFormer
MaskFormer: Per-Pixel Classification is Not All You Need for Semantic Segmentation
Introduction
Abstract
Modern approaches typically formulate semantic segmentation as a per-pixel classification task, while instance-level segmentation is handled with an alternative mask classification. Our key insight: mask classification is sufficiently general to solve both semantic- and instance-level segmentation tasks in a unified manner using the exact same model, loss, and training procedure. Following this observation, we propose MaskFormer, a simple mask classification model which predicts a set of binary masks, each associated with a single global class label prediction. Overall, the proposed mask classification-based method simplifies the landscape of effective approaches to semantic and panoptic segmentation tasks and shows excellent empirical results. In particular, we observe that MaskFormer outperforms per-pixel classification baselines when the number of classes is large. Our mask classification-based method outperforms both current state-of-the-art semantic (55.6 mIoU on ADE20K) and panoptic segmentation (52.7 PQ on COCO) models.
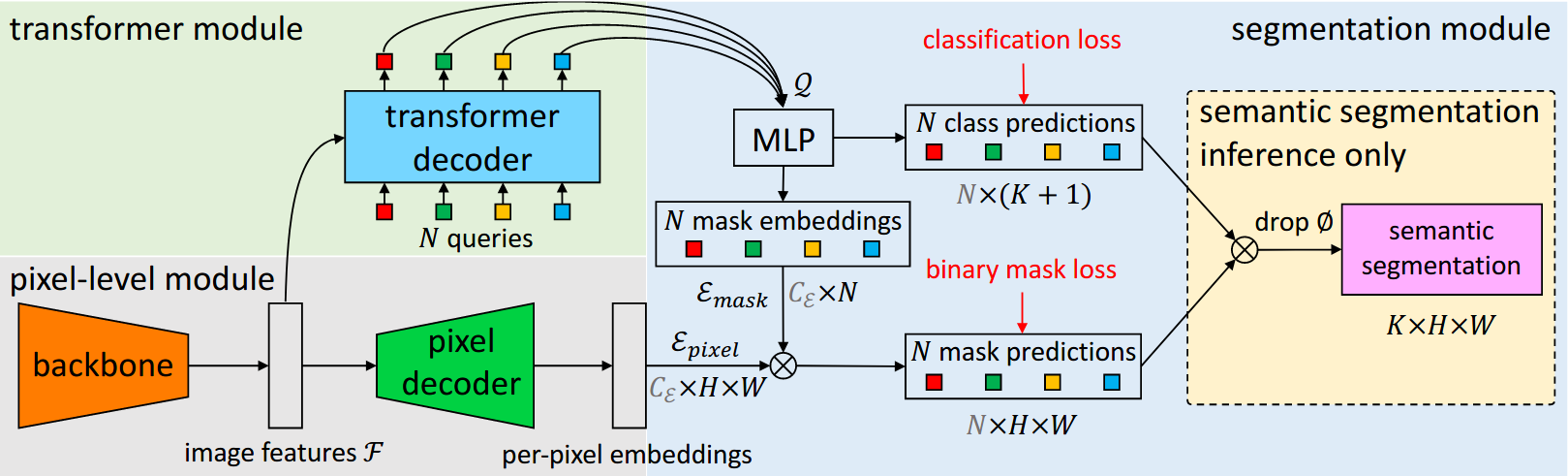
@article{cheng2021per,
title={Per-pixel classification is not all you need for semantic segmentation},
author={Cheng, Bowen and Schwing, Alex and Kirillov, Alexander},
journal={Advances in Neural Information Processing Systems},
volume={34},
pages={17864--17875},
year={2021}
}
Usage
- MaskFormer model needs to install MMDetection first.
pip install "mmdet>=3.0.0rc4"
Results and models
ADE20K
Method | Backbone | Crop Size | Lr schd | Mem (GB) | Inf time (fps) | mIoU | mIoU(ms+flip) | config | download |
---|---|---|---|---|---|---|---|---|---|
MaskFormer | R-50-D32 | 512x512 | 160000 | 3.29 | 42.20 | 44.29 | - | config | model | log |
MaskFormer | R-101-D32 | 512x512 | 160000 | 4.12 | 34.90 | 45.11 | - | config | model | log |
MaskFormer | Swin-T | 512x512 | 160000 | 3.73 | 40.53 | 46.69 | - | config | model | log |
MaskFormer | Swin-S | 512x512 | 160000 | 5.33 | 26.98 | 49.36 | - | config | model | log |
Note:
- All experiments of MaskFormer are implemented with 8 V100 (32G) GPUs with 2 samplers per GPU.
- The results of MaskFormer are relatively not stable. The accuracy (mIoU) of model with
R-101-D32
is from 44.7 to 46.0, and withSwin-S
is from 49.0 to 49.8. - The ResNet backbones utilized in MaskFormer models are standard
ResNet
rather thanResNetV1c
. - Test time augmentation is not supported in MMSegmentation 1.x version yet, we would add "ms+flip" results as soon as possible.