From 3167c469693b5812cb6e49aa456bdd83dadb24e0 Mon Sep 17 00:00:00 2001
From: Nioolek <40284075+Nioolek@users.noreply.github.com>
Date: Sun, 18 Sep 2022 12:17:29 +0800
Subject: [PATCH] Add yolov5 head and doc (#14)
* add yolov5_description.md
* add yolov5_head.py
---
.../yolov5_description.md | 672 ++++++++++++++++++
mmyolo/models/dense_heads/__init__.py | 9 +
mmyolo/models/dense_heads/yolov5_head.py | 643 +++++++++++++++++
3 files changed, 1324 insertions(+)
create mode 100644 docs/zh_cn/algorithm_descriptions/yolov5_description.md
create mode 100644 mmyolo/models/dense_heads/__init__.py
create mode 100644 mmyolo/models/dense_heads/yolov5_head.py
diff --git a/docs/zh_cn/algorithm_descriptions/yolov5_description.md b/docs/zh_cn/algorithm_descriptions/yolov5_description.md
new file mode 100644
index 00000000..4cc629ef
--- /dev/null
+++ b/docs/zh_cn/algorithm_descriptions/yolov5_description.md
@@ -0,0 +1,672 @@
+# YOLOv5 原理和实现全解析
+
+## 0 简介
+
+
+
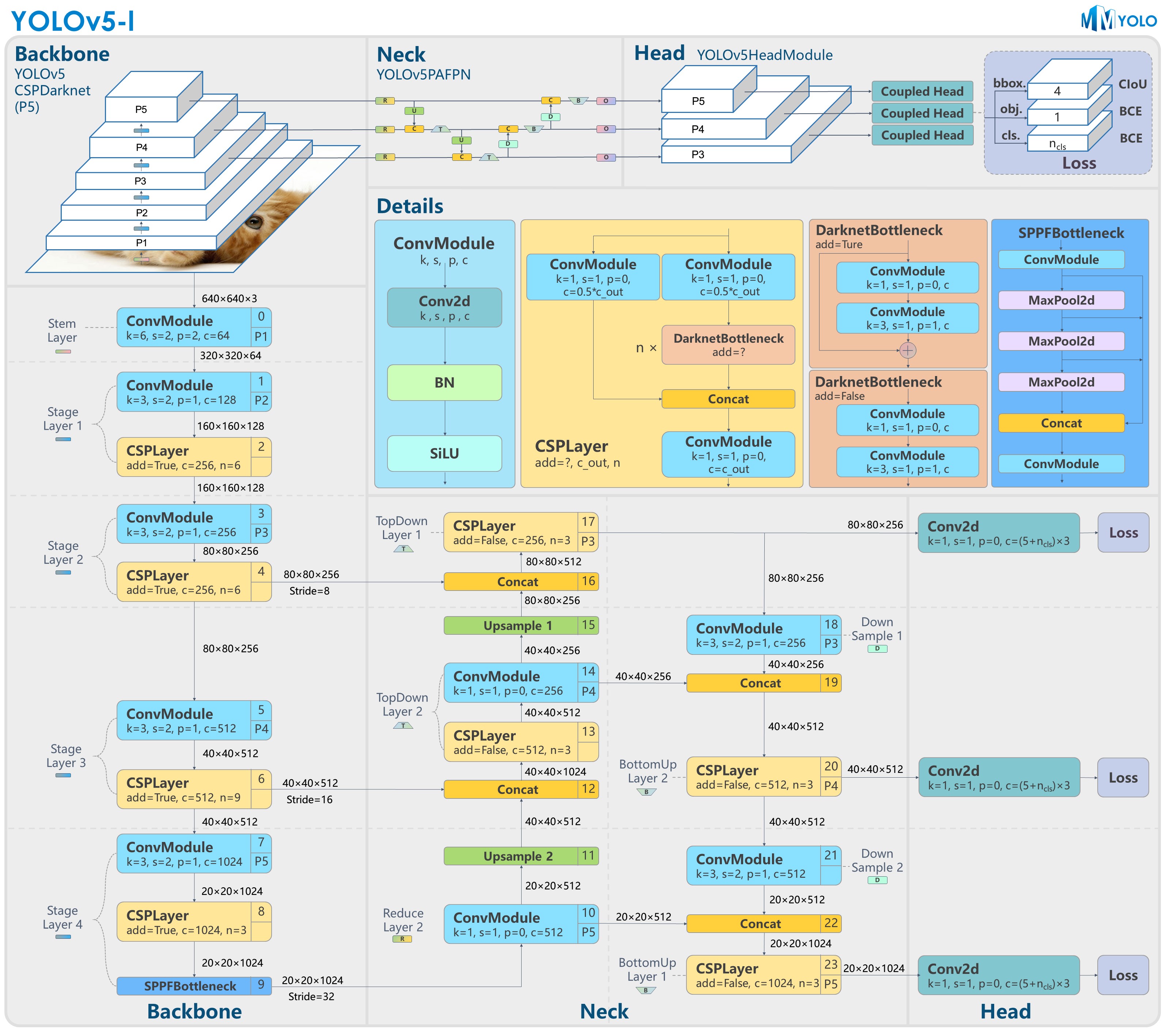
+
+
+以上结构图由 RangeKing@github 绘制。
+
+YOLOv5 是一个面向实时工业应用而开源的目标检测算法,受到了广泛关注。我们认为让 YOLOv5 爆火的原因不单纯在于 YOLOv5 算法本身的优异性,
+更多的在于开源库的实用和鲁棒性。简单来说 YOLOv5 开源库的主要特点为:
+
+1. **友好和完善的部署支持**
+2. **算法训练速度极快**,在 300 epoch 情况下训练时长和大部分 one-stage 算法如 RetinaNet、ATSS 和 two-stage 算法如 Faster R-CNN
+ 12 epoch 时间接近
+3. 框架进行了**非常多的 corner case 优化**,功能和文档也比较丰富
+
+本文将从 YOLOv5 算法本身原理讲起,然后重点分析 MMYOLO 中的实现。关于 YOLOv5 的使用指南和速度等对比请阅读后续文档。
+
+希望本文能够成为你入门和掌握 YOLOv5 的核心文档。由于 YOLOv5 本身也在不断迭代更新,因此我们也会不断的更新本文档。请注意阅读最新版本。
+
+MMYOLO 实现配置:`configs/yolov5/yolov5_s-v61_syncbn_fast_8xb16-300e_coco.py`
+
+YOLOv5 官方开源库地址:https://github.com/ultralytics/yolov5
+
+## 1 v6.1 算法原理和 MMYOLO 实现解析
+
+YOLOv5 官方 release 地址:https://github.com/ultralytics/yolov5/releases/tag/v6.1
+
+
+
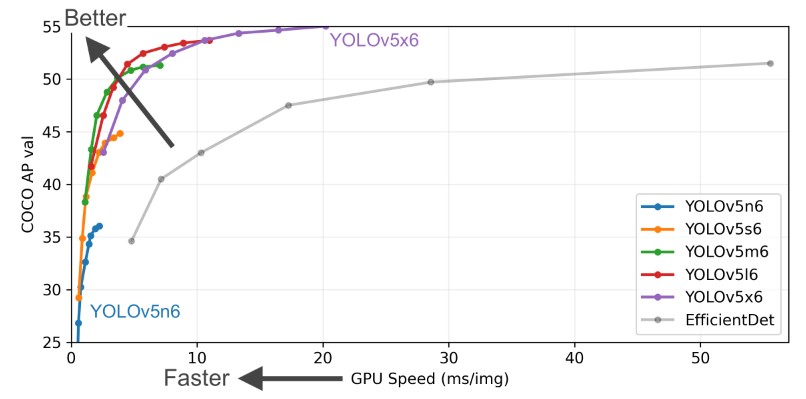
+
+
+
+
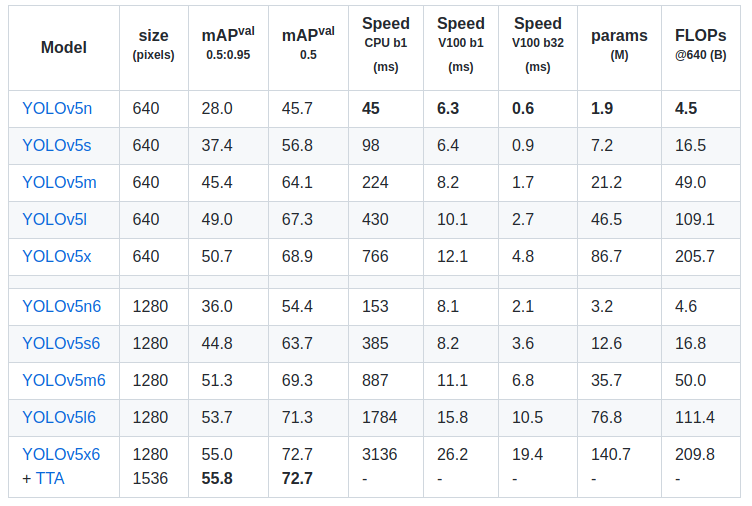
+
+
+性能如上表所示。YOLOv5 有 P5 和 P6 两个不同训练输入尺度的模型,P6 即为 1280x1280 输入的大模型,通常用的是 P5 常规模型,
+输入尺寸是 640x640 。本文解读的也是 P5 模型结构。
+
+通常来说,目标检测算法都可以分成如下数据增强、模型结构、loss 计算等组件,YOLOv5 也一样,如下所示:
+
+
+
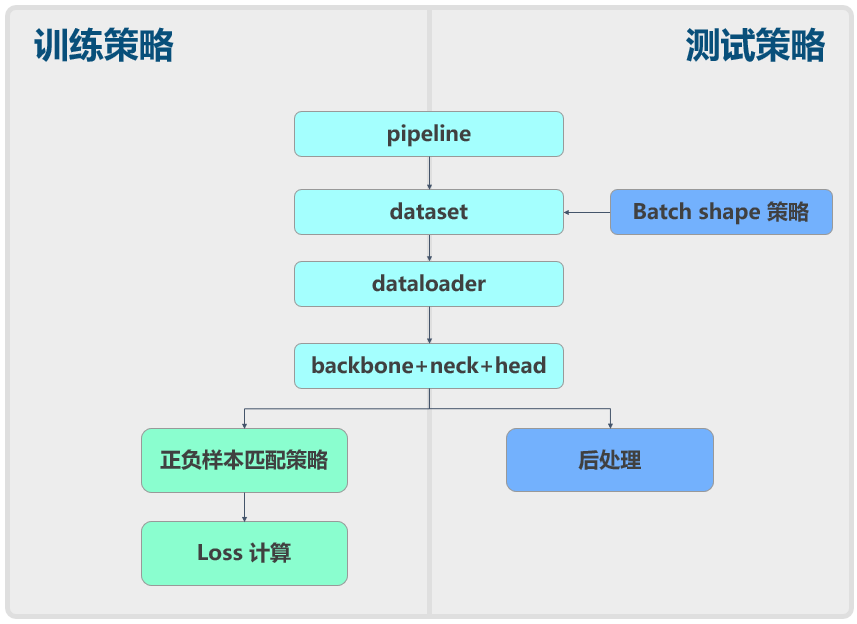
+
+
+下面将从原理和结合 MMYOLO 的具体实现方面进行简要分析。
+
+### 1.1 数据增强模块
+
+YOLOv5 目标检测算法中使用的数据增强比较多,包括:
+
+- **Mosaic 马赛克**
+- **RandomAffine 随机仿射变换**
+- **MixUp**
+- **图像模糊等采用 Albu 库实现的变换**
+- **HSV 颜色空间增强**
+- **随机水平翻转**
+
+其中 Mosaic 数据增强概率为 1,表示一定会触发,而对于 small 和 nano 两个版本的模型不使用 MixUp,其他的 l/m/x 系列模型则采用了 0.1 的概率
+触发 MixUp。小模型能力有限,一般不会采用 MixUp 等强数据增强策略。
+
+其核心的 Mosaic + RandomAffine+ MixUp 过程简要绘制如下:
+
+
+
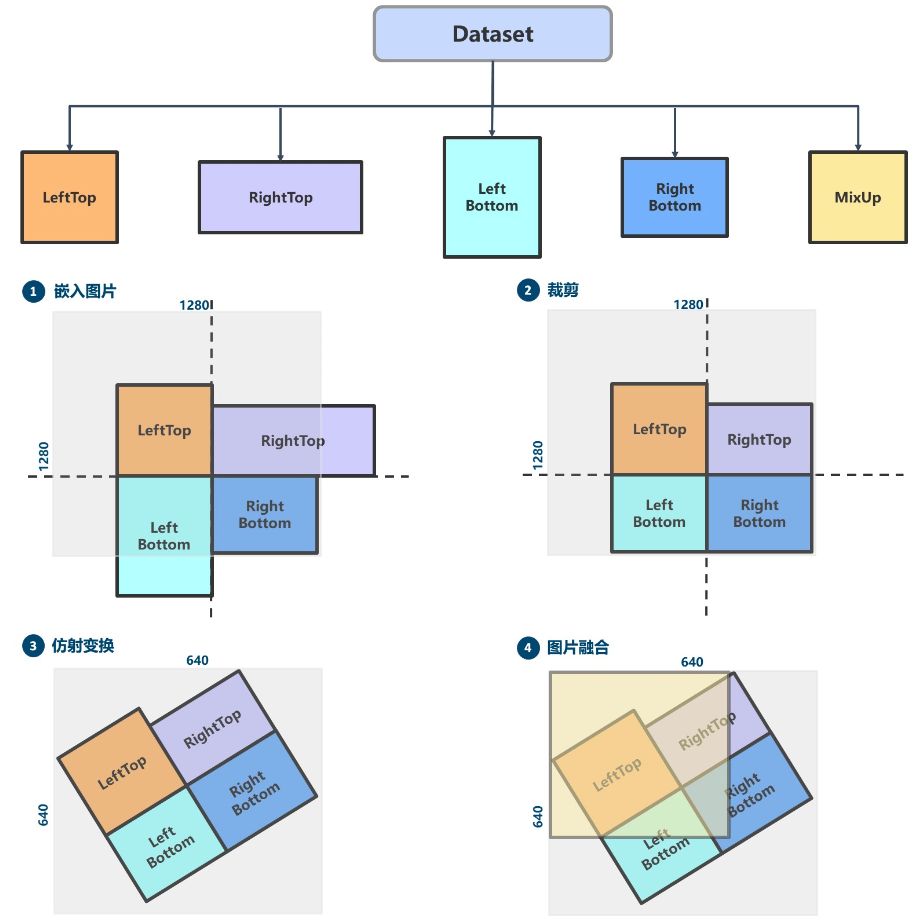
+
+
+下面对其进行简要分析。
+
+#### 1.1.1 Mosaic 马赛克
+
+
+
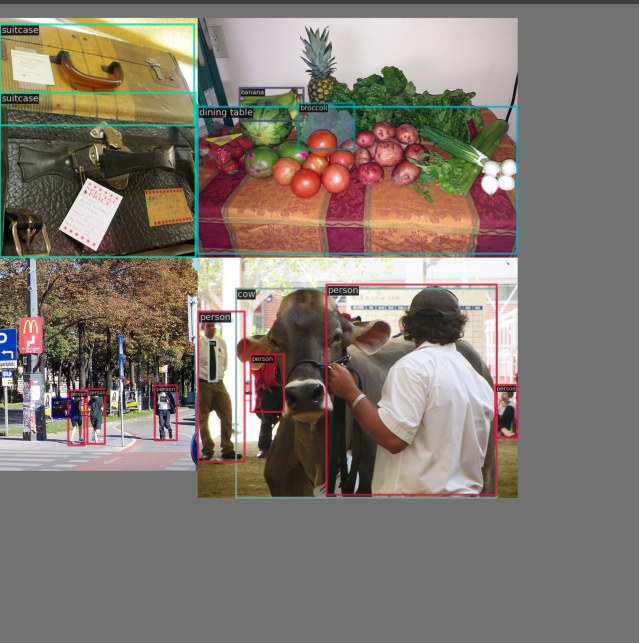
+
+
+Mosaic 属于混合类数据增强,因为它在运行时候需要 4 张图片拼接,变相的相当于增加了训练的 batch size。其运行过程简要概况为:
+
+1. 随机生成拼接后 4 张图的交接中心点坐标,此时就相当于确定了 4 张拼接图片的交接点
+2. 随机出另外 3 张图片的索引以及读取对应的标注
+3. 对每张图片采用保持宽高比的 resize 操作缩放到指定大小
+4. 按照上下左右规则,计算每张图片在待输出图片中应该放置的位置,因为图片可能出界故还需要计算裁剪坐标
+5. 利用裁剪坐标将缩放后的图片裁剪,然后贴到前面计算出的位置,其余位置全部补 114 像素值
+6. 对每张图片的标注也进行相应处理
+
+注意:由于拼接了 4 张图,所以输出图片面积会扩大 4 倍,从 640x640 变成 1280x1280,因此要想恢复为 640x640,
+必须要再接一个 **RandomAffine 随机仿射变换,否则图片面积就一直是扩大 4 倍的**。
+
+#### 1.1.2 RandomAffine 随机仿射变换
+
+
+
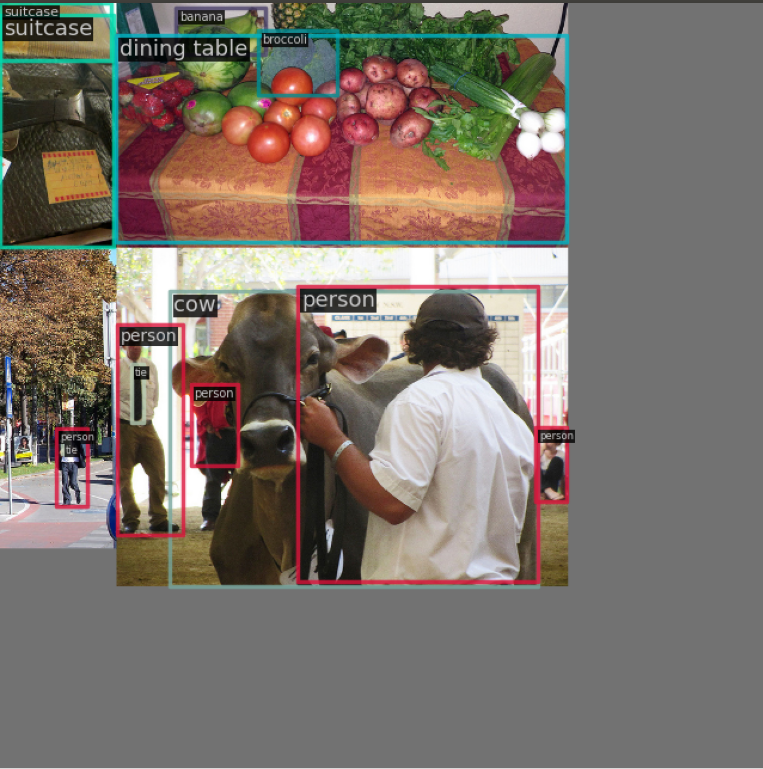
+
+
+随机仿射变换有两个目的:
+
+1. 对图片进行随机几何仿射变换
+2. 将 Mosaic 输出的扩大 4 倍的图片还原为 640x640 尺寸
+
+随机仿射变换包括平移、旋转、缩放、错切等几何增强操作,同时由于 Mosaic 和 RandomAffine 属于比较强的增强操作,会引入较大噪声,
+因此需要对增强后的标注进行处理,过滤规则为
+
+1. 增强后的 gt bbox 宽高要大于 wh_thr
+2. 增强后的 gt bbox 面积和增强前的 gt bbox 面积要大于 ar_thr,防止增强太严重
+3. 最大宽高比要小于 area_thr,防止宽高比改变太多
+
+由于旋转后标注框会变大导致不准确,因此目标检测里面很少会使用旋转数据增强。
+
+#### 1.1.3 MixUp
+
+
+
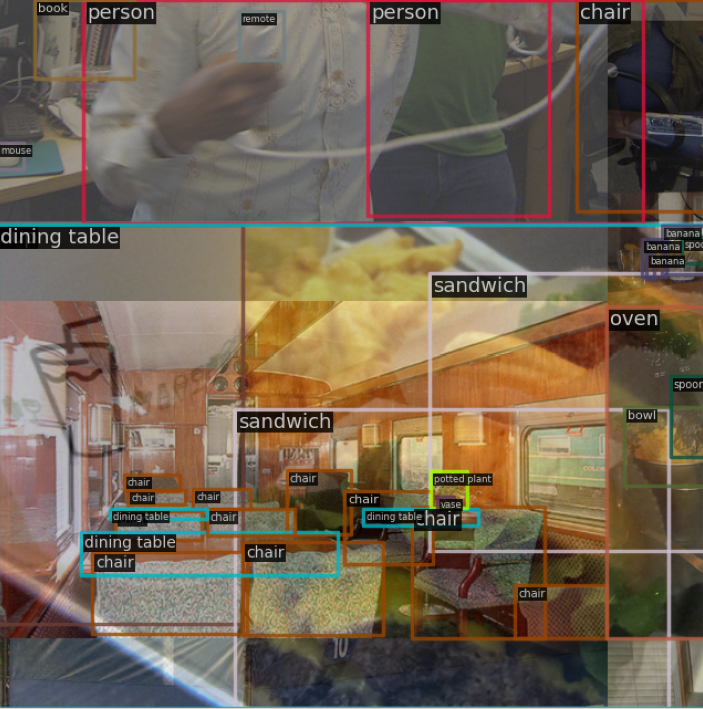
+
+
+MixUp 和 Mosaic 类似,也是属于混合图片类增强,其是随机从另外一张图,然后两种图随机混合而成。其实现方法有多种,常见的做法是:
+要么 label 直接拼接起来,要么 label 也采用 alpha 混合,作者的做法非常简单,对 label 直接拼接即可,而图片通过分布采样混合。
+
+需要特别注意的是:
+**YOLOv5 实现的 MixUp 中,随机出来的另一张图也需要经过 Mosaic 马赛克 + RandomAffine 随机仿射变换 增强后才能混合。这个和其他开源库实现可能不太一样**。
+
+### 1.1.4 图像模糊和其他数据增强
+
+
+
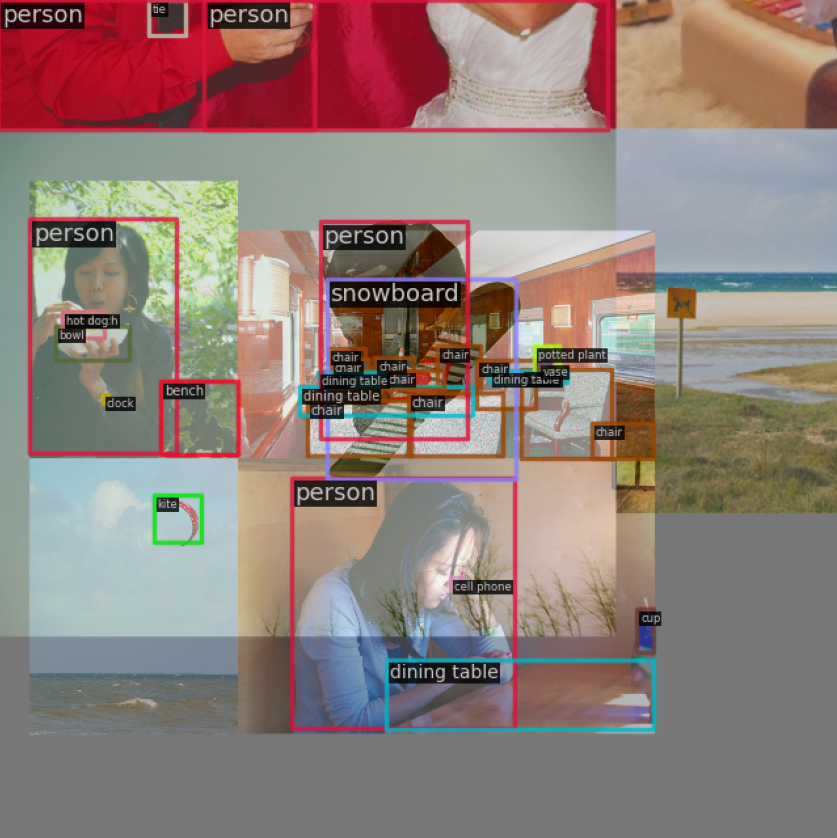
+
+
+剩下的数据增强包括
+
+- **图像模糊等采用 Albu 库实现的变换**
+- **HSV 颜色空间增强**
+- **随机水平翻转**
+
+MMDet 开源库中已经对 Albu 第三方数据增强库进行了封装,使得用户可以简单的通过配置即可使用 Albu 库中提供的任何数据增强功能。
+而 HSV 颜色空间增强和随机水平翻转都是属于比较常规的数据增强,不需要特殊介绍。
+
+#### 1.1.5 MMYOLO 实现解析
+
+常规的单图数据增强例如随机翻转等比较容易实现,而 Mosiac 类的混合数据增强则不太容易。在 MMDet 复现的 YOLOX 算法中
+提出了 MultiImageMixDataset 数据集包装器的概念,其实现过程如下:
+
+
+
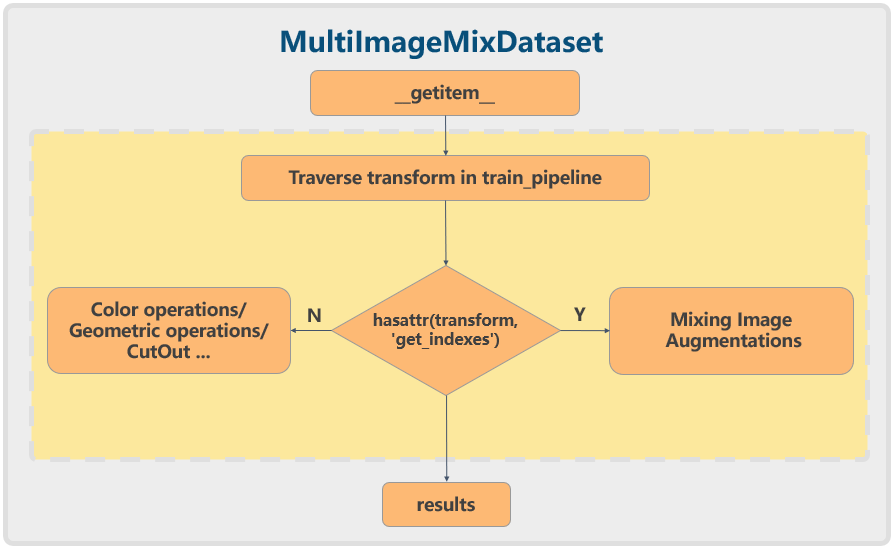
+
+
+对于 Mosiac 等混合类数据增强,会额外实现一个 `get_indexes` 方法用来获取其他图片索引,然后得到 4 张图片信息后就可以进行 Mosiac 增强了。
+以 MMDet 中实现的 YOLOX 为例,其配置文件写法如下所示:
+
+```python
+train_pipeline = [
+ dict(type='Mosaic', img_scale=img_scale, pad_val=114.0),
+ dict(
+ type='RandomAffine',
+ scaling_ratio_range=(0.1, 2),
+ border=(-img_scale[0] // 2, -img_scale[1] // 2)),
+ dict(
+ type='MixUp',
+ img_scale=img_scale,
+ ratio_range=(0.8, 1.6),
+ pad_val=114.0),
+ ...
+]
+
+train_dataset = dict(
+ # use MultiImageMixDataset wrapper to support mosaic and mixup
+ type='MultiImageMixDataset',
+ dataset=dict(
+ type='CocoDataset',
+ pipeline=[
+ dict(type='LoadImageFromFile'),
+ dict(type='LoadAnnotations', with_bbox=True)
+ ]),
+ pipeline=train_pipeline)
+```
+
+MultiImageMixDataset 数据集包装其传入一个包括 Mosaic 和 RandAffine 等数据增强,而 CocoDataset 中也需要传入一个包括图片和
+标注加载的 pipeline。通过这种方式就可以快速的实现混合类数据增强。
+
+但是上述实现有一个缺点:
+**对于不熟悉 MMDet 的用户来说,其经常会忘记 Mosaic 必须要和 MultiImageMixDataset 配合使用,否则会报错,而且这样会加大复杂度和理解难度**。
+
+为了解决这个问题,在 MMYOLO 中进一步进行了简化。直接让 pipeline 能够获取到 dataset 对象,此时就可以将 Mosaic 等混合类数据增强的实现
+和使用变成和随机翻转一样。此时在 MMYOLO 中 YOLOX 的配置写法变成如下所示:
+
+```python
+pre_transform = [
+ dict(type='LoadImageFromFile'),
+ dict(type='LoadAnnotations', with_bbox=True)
+]
+
+train_pipeline = [
+ *pre_transform,
+ dict(
+ type='Mosaic',
+ img_scale=img_scale,
+ pad_val=114.0,
+ pre_transform=pre_transform),
+ dict(
+ type='mmdet.RandomAffine',
+ scaling_ratio_range=(0.1, 2),
+ border=(-img_scale[0] // 2, -img_scale[1] // 2)),
+ dict(
+ type='YOLOXMixUp',
+ img_scale=img_scale,
+ ratio_range=(0.8, 1.6),
+ pad_val=114.0,
+ pre_transform=pre_transform),
+ ...
+]
+```
+
+此时就不再需要 MultiImageMixDataset 了,使用和理解上会更加简单。
+
+回到 YOLOv5 配置上,因为 YOLOv5 实现的 MixUp 中,随机出来的另一张图也需要经过 Mosaic 马赛克+RandomAffine 随机仿射变换 增强后才能混合,
+故YOLOv5-m 数据增强配置如下所示:
+
+```python
+pre_transform = [
+ dict(type='LoadImageFromFile'),
+ dict(type='LoadAnnotations', with_bbox=True)
+]
+
+mosaic_transform= [
+ dict(
+ type='Mosaic',
+ img_scale=img_scale,
+ pad_val=114.0,
+ pre_transform=pre_transform),
+ dict(
+ type='YOLOv5RandomAffine',
+ max_rotate_degree=0.0,
+ max_shear_degree=0.0,
+ scaling_ratio_range=(0.1, 1.9), # scale = 0.9
+ border=(-img_scale[0] // 2, -img_scale[1] // 2),
+ border_val=(114, 114, 114))
+]
+
+train_pipeline = [
+ *pre_transform,
+ *mosaic_transform,
+ dict(
+ type='YOLOv5MixUp',
+ prob=0.1,
+ pre_transform=[
+ *pre_transform,
+ *mosaic_transform
+ ]),
+ ...
+]
+```
+
+### 1.2 网络结构
+
+本小结由 RangeKing@github 撰写,非常感谢!!!
+
+YOLOv5 网络结构是标准的 `CSPDarknet` + `PAFPN` + `非解耦 Head`。
+
+YOLOv5 网络结构大小由 `deepen_factor` 和 `widen_factor` 两个参数决定,其中 `deepen_factor` 控制网络结构深度
+即 `CSPLayer` 中 `DarknetBottleneck` 模块堆叠的数量,`widen_factor` 控制网络结构宽度即模块输出特征图的通道数。以 YOLOv5-l 为例,
+其 `deepen_factor = widen_factor = 1.0` ,整体结构图如上所示。
+
+图的上半部分为模型总览;下半部分为具体网络结构,其中的模块均标有序号,方便用户与 YOLOv5 官方仓库的配置文件对应;中间部分为各子模块的具体构成。
+
+如果想使用 netron 可视化网络结构图细节,可以直接将 MMDeploy 导出的 ONNX 文件格式使用 netron 打开。
+
+#### 1.2.1 Backbone
+
+在 MMYOLO 中 `CSPDarknet` 继承自 `BaseBackbone`,整体结构和 `ResNet` 类似,共 5 层结构,
+包含 1 个 `Stem Layer` 和 4 个 `Stage Layer`
+
+- `Stem Layer` 是 1 个 6x6 kernel 的 `ConvModule`,相较于 v6.1 版本之前的 `Focus` 模块更加高效
+- 前 3 个 `Stage Layer` 由 1 个 `ConvModule` 和 1 个 `CSPLayer` 组成。如上图 Details 部分,
+ 其中 `ConvModule` 为 3x3 `Conv2d` + `BatchNorm` + `SiLU 激活函数`。`CSPLayer` 即 YOLOv5 官方仓库中的 C3 模块,
+ 由 3 个 `ConvModule` + n 个 `DarknetBottleneck`(带残差连接) 组成
+- 第 4 个 `Stage Layer` 在最后增加了 `SPPF` 模块。`SPPF` 模块是将输入串行通过多个 5x5 大小的 `MaxPool2d` 层,
+ 与 `SPP` 模块效果相同,但速度更快
+- P5 模型结构会在 `Stage Layer` 2-4 之后分别输出,进入 `Neck` 结构,共抽取三个输出特征图,以 640x640 输入图片为例,
+ 其输出特征为 (B,256,80,80)、 (B,512,40,40) 和 (B,1024,20,20),stride 为 8/16/32
+
+#### 1.2.2 Neck
+
+YOLOv5 官方仓库的配置文件中并没有 Neck 部分,为方便用户与其他目标检测网络结构相对应,
+我们将官方仓库的 `Head` 拆分成 `PAFPN` 和 `Head` 两部分。
+
+基于 `BaseYOLONeck` 结构,YOLOv5 `Neck` 也是遵循同一套构建流程,对于不存在的模块,我们采用 `nn.Identity` 代替。
+
+Neck 模块输出特征图和 Backbone 完全一致即为 (B,256,80,80)、 (B,512,40,40) 和 (B,1024,20,20)。
+
+#### 1.2.3 Head
+
+YOLOv5 Head 结构和 YOLOv3 完全一样 `为非解耦 Head`。Head 模块只包括 3 个不共享权重的卷积,用于将输入特征图进行变换而已。
+
+前面的 PAFPN 依然是输出 3 个不同尺度的特征图,shape 为(B,256,80,80)、 (B,512,40,40) 和 (B,1024,20,20)。
+由于 YOLOv5 是非解耦输出即分类和 bbox 检测等都是在同一个卷积的不同通道中完成,以 COCO 80 类为例,在输入为 640x640 分辨率情况下,
+其 Head 模块输出的 shape 分别为 (B, 3x(4+1+80),80,80), (B, 3x(4+1+80),40,40) 和 (B, 3x(4+1+80),20,20。
+其中 3 表示 3 个 anchor,4 表示 bbox 预测分支,1 表示 obj 预测分支,80 表示类别预测分支。
+
+### 1.3 正负样本匹配策略
+
+正负样本匹配策略的核心是确定预测特征图的所有位置中哪些位置应该是正样本,哪些是负样本,甚至有些是忽略样本。
+匹配策略是目标检测算法的核心,一个好的匹配策略明显可以提升算法性能。
+
+YOLOV5 的匹配策略简单总结为:**采用了 anchor 和 gt_bbox 的 shape 匹配度作为划分规则,同时引入跨邻域网格策略来增加正样本**。
+其主要包括如下两个核心步骤:
+
+1. 对于任何一个输出层,抛弃了常用的基于 Max IoU 匹配的规则,而是直接采用 shape 规则匹配,
+ 也就是该 GT Bbox 和当前层的 Anchor 计算宽高比,如果宽高比例大于设定阈值,则说明该 GT Bbox 和 Anchor 匹配度不够,
+ 将该 GT Bbox 过滤暂时丢掉,在该层预测中该 GT Bbox 对应的网格内的预测位置认为是负样本
+2. 对于剩下的 GT Bbox(也就是匹配上的 GT Bbox),计算其落在哪个网格内,同时利用四舍五入规则,
+ 找出最近的两个网格,将这三个网格都认为是负责预测该 GT Bbox 的,可以发现粗略估计正样本数相比前 YOLO 系列,至少增加了三倍
+
+下面对每个部分进行详细说明。部分描述和图示直接或间接参考自官方 [Repo](https://github.com/ultralytics/YOLOv5/issues/6998#44)。
+
+#### 1.3.1 Anchor 设置
+
+YOLOv5 是 Anchor-based 的目标检测算法,Anchor size 的获取方式与 YOLOv3 相同,是使用 kmeans算法进行聚类获得。
+
+在用户更换了数据集后,可以使用 MMDet 里带有的 Anchor 分析工具,对自己的数据集进行分析,确定合适的 Anchor size。
+
+若你的 MMDet 通过 mim 安装,可使用以下命令分析 Anchor:
+
+```shell
+mim run mmdet optimize_anchors ${CONFIG} --algorithm k-means
+--input-shape ${INPUT_SHAPE [WIDTH HEIGHT]} --output-dir ${OUTPUT_DIR}
+```
+
+若 MMDet 为其他方式安装,可进入 MMDet 所在目录,使用以下命令分析 Anchor:
+
+```shell
+python tools/analysis_tools/optimize_anchors.py ${CONFIG} --algorithm k-means
+ --input-shape ${INPUT_SHAPE [WIDTH HEIGHT]} --output-dir ${OUTPUT_DIR}
+```
+
+然后在 [config 文件](../../../configs/yolov5/yolov5_s-v61_syncbn_fast_8xb16-300e_coco.py)里修改默认 Anchor size:
+
+```python
+anchors = [[(10, 13), (16, 30), (33, 23)], [(30, 61), (62, 45), (59, 119)],
+ [(116, 90), (156, 198), (373, 326)]]
+```
+
+#### 1.3.2 Bbox 编解码过程
+
+在 Anchor-based 算法中,预测框通常会基于 Anchor 进行变换,然后预测变换量,这对应 GT Bbox 编码过程,而在预测后需要进行 Pred Bbox 解码,
+还原为真实尺度的 Bbox,这对应 Pred Bbox 解码过程。
+
+在 YOLOv3 中,回归公式为:
+
+```math
+b_x=\sigma(t_x)+c_x \\
+b_y=\sigma(t_y)+c_y \\
+b_w=a_w\cdot e^{t_w} \\
+b_h=a_h\cdot e^{t_h} \\
+```
+
+公式中,
+
+```math
+a_w 代表 Anchor 的宽度 \\
+c_x 代表 Grid 所处的坐标 \\
+\sigma 代表 Sigmoid 公式。
+```
+
+而在 YOLOv5 中,回归公式为:
+
+```math
+b_x=(2\cdot\sigma(t_x)-0.5)+c_x \\
+b_y=(2\cdot\sigma(t_y)-0.5)+c_y \\
+b_w=a_w\cdot(2\cdot\sigma(t_w))^2 \\
+b_h=a_h\cdot(2\cdot\sigma(t_h))^2
+```
+
+改进之处主要有以下几点:
+
+- 中心点坐标范围从 (0, 1) 调整至 (-0.5, 1.5)
+- 宽高范围从
+
+```math
+(0,+\infty)
+```
+
+调整至
+
+```math
+(0,4a_{wh})
+```
+
+这个改进具有以下好处:
+
+- **中心点能更好的预测到 0 和 1**。有助于更精准回归出 box 坐标。
+
+
+
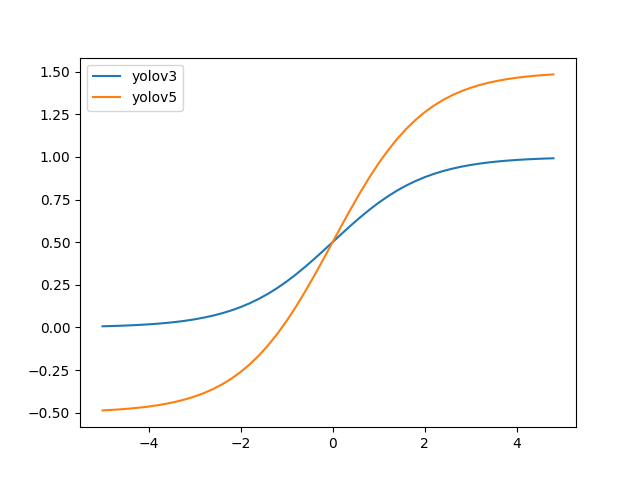
+
+
+- 宽高回归公式 exp(x) 是无界的,这会导致**梯度失去控制**,造成训练不稳定。YOLOv5 中改进后的宽高回归公式优化了此问题。
+
+
+
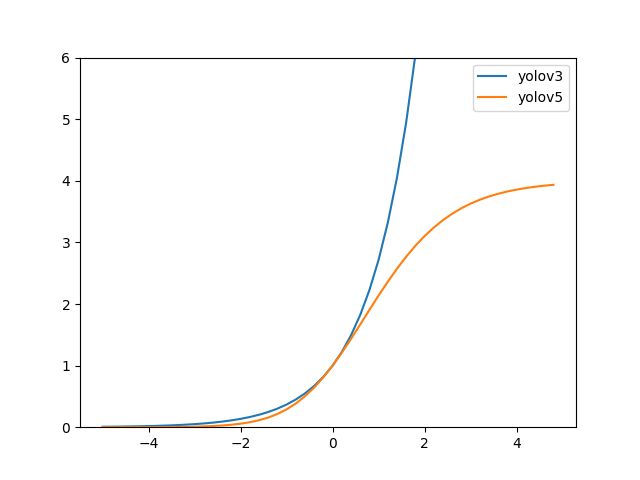
+
+
+#### 1.3.3 匹配策略
+
+在 MMYOLO 设计中,无论网络是 Anchor-based 还是 Anchor-free,**我们统一使用 prior 称呼 Anchor**。
+
+正样本匹配包含以下两步:
+
+**(1) “比例”比较**
+
+将 GT Bbox 的 WH 与 Prior 的 WH 进行“比例”比较。
+
+比较流程:
+
+```math
+r_w = w\_{gt} / w\_{pt} \\
+r_h = h\_{gt} / h\_{pt} \\
+r_w^{max}=max(r_w, 1/r_w) \\
+r_h^{max}=max(r_h, 1/r_h) \\
+r^{max}=max(r_w^{max}, r_h^{max}) \\
+if\ \ r_{max} < prior\_match\_thr: match!
+```
+
+此处我们用一个 GT Bbox 与 P3 特征图的 Prior 进行匹配的案例进行讲解+图示:
+
+
+
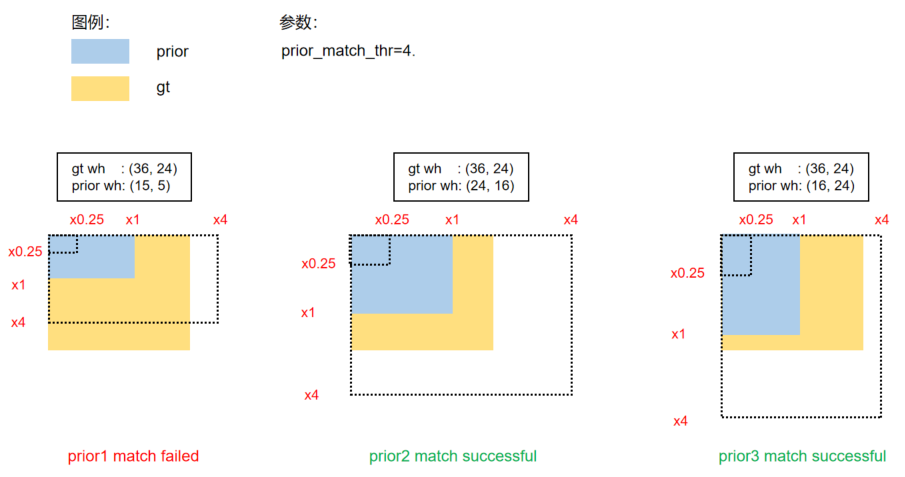
+
+
+prior1 匹配失败的原因是
+
+```math
+h\_{gt}\ /\ h\_{prior}\ =\ 4.8\ >\ prior\_match\_thr
+```
+
+**(2) 将步骤 1 中 match 的 GT 分配对应的正样本**
+
+依然沿用上面的例子:
+
+GT Bbox (cx, cy, w, h) 值为 (26, 37, 36, 24),
+
+Prior WH 值为 \[(15, 5), (24, 16), (16, 24)\],其中在 P3 特征图上,stride为 8,通过计算 prior2,prior3 能够 match。
+
+计算过程如下:
+
+**(2.1) 将 GT Bbox 的中心点坐标对应到 P3 的 grid 上**
+
+```math
+GT_x^{center_grid}=26/8=3.25 \\
+GT_y^{center_grid}=37/8=4.625
+```
+
+
+
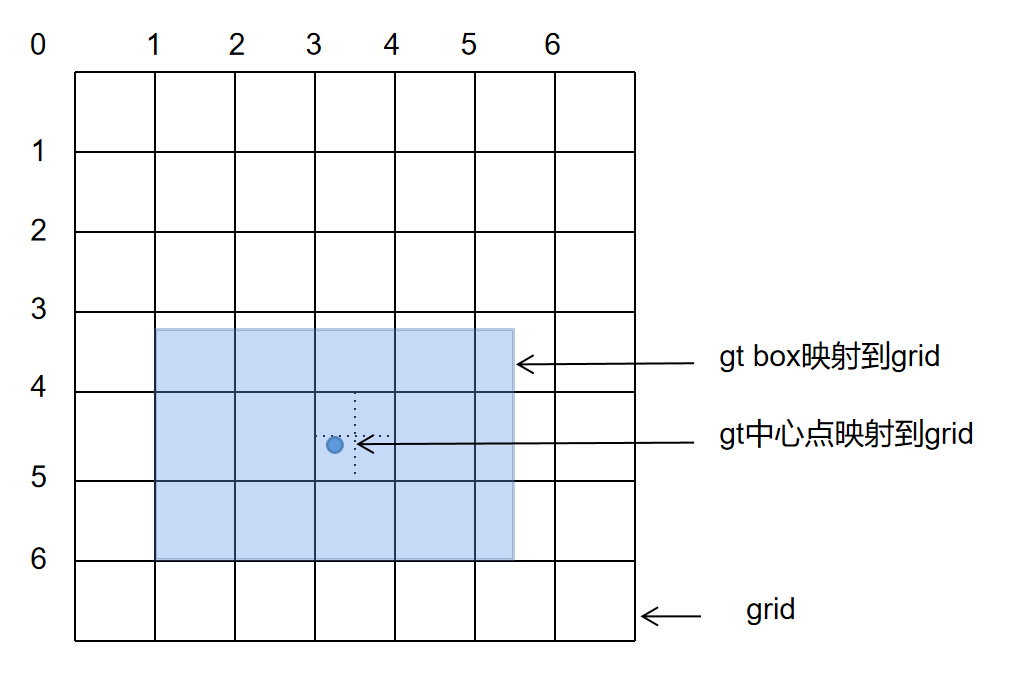
+
+
+(2.2) 将 GT Bbox 中心点所在的 grid 分成四个象限,**由于中心点落在了左下角的象限当中,那么会将物体的左、下两个 grid 也认为是正样本**
+
+
+
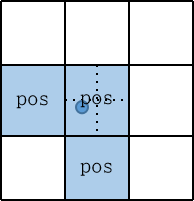
+
+
+下图展示中心点落到不同位置时的正样本分配情况:
+
+
+
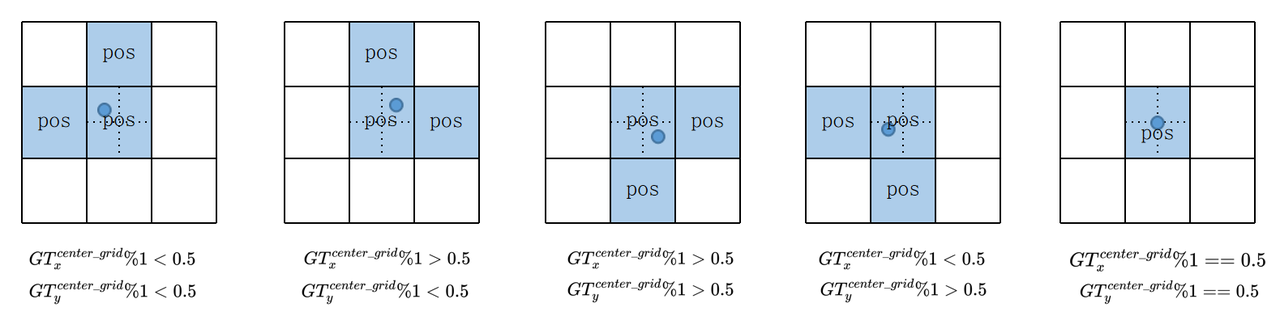
+
+
+那么 YOLOv5 的 Assign 方式具体带来了哪些改进?
+
+- 一个 GT Bbox 能够匹配多个 Prior
+
+- 一个 GT Bbox 和一个Prior 匹配时,能分配 1-3 个正样本
+
+- 以上策略能**适度缓解目标检测中常见的正负样本不均衡问题**。
+
+而 YOLOv5 中的回归方式,和 Assign 方式是相互呼应的:
+
+1. 中心点回归方式:
+
+
+
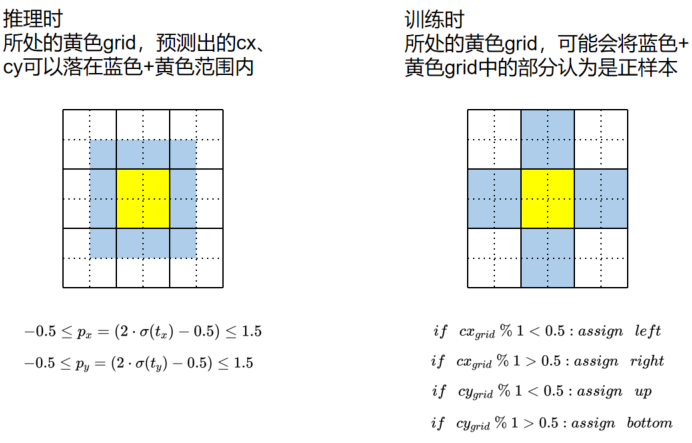
+
+
+2. WH 回归方式:
+
+
+
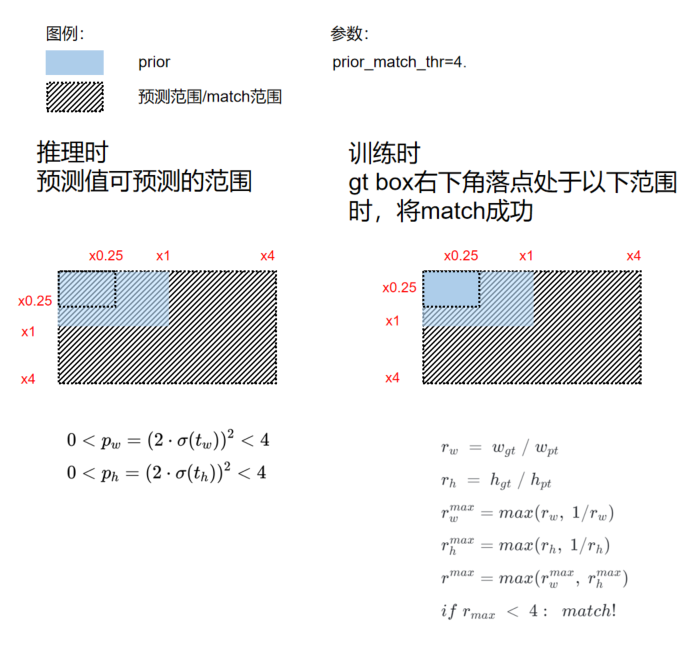
+
+
+### 1.4 Loss设计
+
+YOLOv5 中总共包含 3 个 Loss,分别为:
+
+- Classes loss:使用的是 BCE loss
+- Objectness loss:使用的是 BCE loss
+- Location loss:使用的是 CIoU loss
+
+三个 loss 按照一定比例汇总。
+
+```math
+Loss=\lambda_1L_{cls}+\lambda_2L_{obj}+\lambda_3L_{loc}
+```
+
+在 Objectness loss 中,P3,P4,P5 层的 Objectness loss 按照不同权重进行相加,默认的设置是
+
+```python
+obj_level_weights=[4., 1., 0.4]
+```
+
+```math
+L_{obj}=4.0\cdot L_{obj}^{small}+1.0\cdot L_{obj}^{medium}+0.4\cdot L_{obj}^{large}
+```
+
+在复现中我们发现 YOLOv5 中使用的 CIoU 与目前最新官方 CIoU 存在一定的差距,差距体现在 alpha 参数的计算。
+
+官方版本:
+
+参考资料:https://github.com/Zzh-tju/CIoU/blob/master/layers/modules/multibox_loss.py#L53-L55
+
+```python
+alpha = (ious > 0.5).float() * v / (1 - ious + v)
+```
+
+YOLOv5 版本:
+
+```python
+alpha = v / (v - ious + (1 + eps))
+```
+
+这是一个有趣的细节,后续需要测试不同 alpha 计算方式情况下带来的精度差距。
+
+### 1.5 优化策略和训练过程
+
+YOLOv5 对每个优化器参数组进行非常精细的控制,简单来说包括如下部分。
+
+#### 1.5.1 优化器分组
+
+将优化参数分成 Conv/Bias/BN 三组,在 WarmUp 阶段,不同组采用不同的 lr 以及 momentum 更新曲线。
+同时在 WarmUp 阶段采用的是 iter-based 更新策略,而非 WarmUp 阶段则变成epoch-based 更新策略,可谓是 trick 十足。
+
+MMYOLO 中是采用 YOLOv5OptimizerConstructor 优化器构造器实现优化器参数分组。
+优化器构造器的作用就是对一些特殊的参数组初始化过程进行精细化控制,因此可以很好的满足需求。
+
+而不同的参数组采用不同的调度曲线功能则是通过 YOLOv5ParamSchedulerHook 实现。
+
+#### 1.5.2 weight decay 参数自适应
+
+作者针对不同的 batch size 采用了不同的 weight decay 策略,具体来说为:
+
+1. 当训练batch size \<= 64 时,weight decay 不变
+2. 当训练batch size > 64 时,weight decay 会根据总 batch size 进行线性缩放
+
+MMYOLO 也是通过 YOLOv5OptimizerConstructor 实现。
+
+#### 1.5.3 梯度累加
+
+为了最大化不同 batch size 情况下的性能,作者设置总 batch size 小于 64 时候会自动开启梯度累加功能。
+
+训练过程和大部分 YOLO 类似,包括如下策略:
+
+1. 没有使用预训练权重
+2. 没有采用多尺度训练策略,同时可以开启 cudnn.benchmark 进一步加速训练
+3. 使用了 EMA 策略平滑模型
+4. 默认采用 AMP 自动混合精度训练
+
+需要特意说明的是:YOLOv5 官方对于 small 模型是采用单卡 v100 训练,bs 为 128,而 m/l/x 等是采用不同数目的多卡实现的,
+这种训练策略不太规范,**为此在 MMYOLO 中全部采用了 8 卡,每卡 16 bs 的设置,同时为了避免性能差异,训练时候开启了 SyncBN**。
+
+### 1.6 推理和后处理过程
+
+#### 1.6.1 推理过程
+
+YOLOv5 后处理过程和 YOLOv3 非常类似,实际上 YOLO 系列的后处理逻辑都是类似的。其核心控制参数为:
+
+1. **multi_label**
+
+对于多类别预测来说是否考虑多标签,也就是同一个预测位置中预测的多个类别概率,是否当做单类处理。因为 YOLOv5 采用 sigmoid 预测模式,
+在考虑多标签情况下可能会出现一个物体检测出两个不同类别的框,这有助于评估指标 mAP,但是不利于实际应用。
+因此在需要算评估指标时候 multi_label 是 True,而推理或者实际应用时候是 False
+
+2. **score_thr 和 nms_thr**
+
+score_thr 阈值用于过滤类别分值,低于分值的检测框当做背景处理,nms_thr 是 nms 时阈值。同样的,在计算评估指标 mAP 阶段可以将 score_thr 设置的非常低,这通常能够提高召回率,从而提升 mAP,但是对于实际应用来说没有意义,且会导致推理过程极慢。为此在测试和推理阶段会设置不同的阈值
+
+3. **nms_pre 和 max_per_img**
+
+nms_pre 表示 nms 前的最大保留检测框数目,这通常是为了防止 nms 运行时候输入框过多导致速度过慢问题,默认值是 30000。
+max_per_img 表示最终保留的最大检测框数目,通常设置为 300。
+
+以 COCO 80 类为例,假设输入图片大小为 640x640
+
+
+
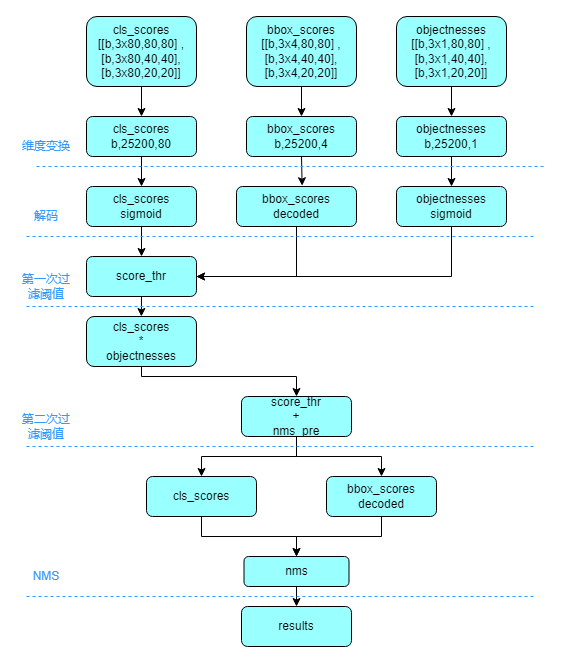
+
+
+其推理和后处理过程为:
+
+**(1) 维度变换**
+
+YOLOv5 输出特征图尺度为 80x80、40x40 和 20x20 的三个特征图,每个位置共 3 个 anchor,因此输出特征图通道为 3x(5+80)=255。
+YOLOv5 是非解耦输出头,而其他大部分算法都是解耦输出头,为了统一后处理逻辑,我们提前将其进行解耦,
+分成了类别预测分支、bbox 预测分支和 obj 预测分支。
+
+将三个不同尺度的类别预测分支、bbox 预测分支和 obj 预测分支进行拼接,并进行维度变换。为了后续方便处理,会将原先的通道维度置换到最后,
+类别预测分支、bbox 预测分支和 obj 预测分支的 shape
+分别为 (b, 3x80x80+3x40x40+3x20x20, 80)=(b,25200,80),(b,25200,4),(b,25200,1)。
+
+**(2) 解码还原到原图尺度**
+
+分类预测分支和 obj 分支需要进行 sigmoid 计算,而 bbox 预测分支需要进行解码,还原为真实的原图解码后 xyxy 格式
+
+**(3) 第一次阈值过滤**
+
+遍历 batch 中的每张图,然后用 score_thr 对类别预测分值进行阈值过滤,去掉低于 score_thr 的预测结果
+
+**(4) 第二次阈值过滤**
+
+将 obj 预测分值和过滤后的类别预测分值相乘,然后依然采用 score_thr 进行阈值过滤。
+在这过程中还需要考虑 **multi_label 和 nms_pre,确保过滤后的检测框数目不会多于 nms_pre**。
+
+**(5) 还原到原图尺度和 nms**
+
+基于前处理过程,将剩下的检测框还原到网络输出前的原图尺度,然后进行 nms 即可。最终输出的检测框不能多于 **max_per_img**。
+
+#### 1.6.2 batch shape 策略
+
+为了加速验证集的推理过程,作者提出了 batch shape 策略,其核心原则是:**确保在 batch 推理过程中同一个 batch 内的图片 pad 像素最少,
+不要求整个验证过程中所有 batch 的图片尺度一样**。
+
+其大概流程是:将整个测试或者验证数据的宽高比进行排序,然后依据 batch 设置将排序后的图片组成一个 batch,
+同时计算这个 batch 内最佳的 batch shape,防止 pad 像素过多,最佳 batch shape 计算原则为在保持宽高比的情况下进行 pad,不追求正方形图片输出。
+
+```python
+ image_shapes = []
+ for data_info in data_list:
+ image_shapes.append((data_info['width'], data_info['height']))
+
+ image_shapes = np.array(image_shapes, dtype=np.float64)
+
+ n = len(image_shapes) # number of images
+ batch_index = np.floor(np.arange(n) / self.batch_size).astype(
+ np.int) # batch index
+ number_of_batches = batch_index[-1] + 1 # number of batches
+
+ aspect_ratio = image_shapes[:, 1] / image_shapes[:, 0] # aspect ratio
+ irect = aspect_ratio.argsort()
+
+ data_list = [data_list[i] for i in irect]
+
+ aspect_ratio = aspect_ratio[irect]
+ # Set training image shapes
+ shapes = [[1, 1]] * number_of_batches
+ for i in range(number_of_batches):
+ aspect_ratio_index = aspect_ratio[batch_index == i]
+ min_index, max_index = aspect_ratio_index.min(
+ ), aspect_ratio_index.max()
+ if max_index < 1:
+ shapes[i] = [max_index, 1]
+ elif min_index > 1:
+ shapes[i] = [1, 1 / min_index]
+
+ batch_shapes = np.ceil(
+ np.array(shapes) * self.img_size / self.size_divisor +
+ self.pad).astype(np.int) * self.size_divisor
+
+ for i, data_info in enumerate(data_list):
+ data_info['batch_shape'] = batch_shapes[batch_index[i]]
+```
+
+## 2 总结
+
+本文对 YOLOv5 原理和在 MMYOLO 实现进行了详细解析,希望能帮助用户理解算法实现过程。同时请注意:由于 YOLOv5 本身也在不断更新,
+本开源库也会不断迭代,请及时阅读和同步最新版本。
diff --git a/mmyolo/models/dense_heads/__init__.py b/mmyolo/models/dense_heads/__init__.py
new file mode 100644
index 00000000..bb793f68
--- /dev/null
+++ b/mmyolo/models/dense_heads/__init__.py
@@ -0,0 +1,9 @@
+# Copyright (c) OpenMMLab. All rights reserved.
+from .yolov5_head import YOLOv5Head, YOLOv5HeadModule
+from .yolov6_head import YOLOv6Head, YOLOv6HeadModule
+from .yolox_head import YOLOXHead, YOLOXHeadModule
+
+__all__ = [
+ 'YOLOv5Head', 'YOLOv6Head', 'YOLOXHead', 'YOLOv5HeadModule',
+ 'YOLOv6HeadModule', 'YOLOXHeadModule'
+]
diff --git a/mmyolo/models/dense_heads/yolov5_head.py b/mmyolo/models/dense_heads/yolov5_head.py
new file mode 100644
index 00000000..6fda0f71
--- /dev/null
+++ b/mmyolo/models/dense_heads/yolov5_head.py
@@ -0,0 +1,643 @@
+# Copyright (c) OpenMMLab. All rights reserved.
+import copy
+import math
+from typing import List, Optional, Sequence, Tuple, Union
+
+import torch
+import torch.nn as nn
+from mmengine.config import ConfigDict
+from mmengine.dist import get_dist_info
+from mmengine.logging import print_log
+from mmengine.model import BaseModule
+from mmengine.structures import InstanceData
+from torch import Tensor
+
+from mmdet.models.dense_heads.base_dense_head import BaseDenseHead
+from mmdet.models.utils import filter_scores_and_topk, multi_apply
+from mmdet.utils import (ConfigType, OptConfigType, OptInstanceList,
+ OptMultiConfig)
+from mmyolo.registry import MODELS, TASK_UTILS
+from ..utils import make_divisible
+
+
+@MODELS.register_module()
+class YOLOv5HeadModule(BaseModule):
+ """YOLOv5Head head module used in `YOLOv5`.
+
+ Args:
+ num_classes (int): Number of categories excluding the background
+ category.
+ in_channels (int): Number of channels in the input feature map.
+ widen_factor (float): Width multiplier, multiply number of
+ channels in each layer by this amount. Default: 1.0.
+ num_base_priors:int: The number of priors (points) at a point
+ on the feature grid.
+ featmap_strides (Sequence[int]): Downsample factor of each feature map.
+ Defaults to (8, 16, 32).
+ init_cfg (:obj:`ConfigDict` or list[:obj:`ConfigDict`] or dict or
+ list[dict], optional): Initialization config dict.
+ Defaults to None.
+ """
+
+ def __init__(self,
+ num_classes: int,
+ in_channels: Union[int, Sequence],
+ widen_factor: float = 1.0,
+ num_base_priors: int = 3,
+ featmap_strides: Sequence[int] = (8, 16, 32),
+ init_cfg: OptMultiConfig = None):
+ super().__init__(init_cfg=init_cfg)
+ self.num_classes = num_classes
+ self.in_channels = in_channels
+ self.widen_factor = widen_factor
+
+ self.featmap_strides = featmap_strides
+ self.num_out_attrib = 5 + self.num_classes
+ self.num_levels = len(self.featmap_strides)
+ self.num_base_priors = num_base_priors
+
+ in_channels = []
+ for channel in self.in_channels:
+ channel = make_divisible(channel, self.widen_factor)
+ in_channels.append(channel)
+ self.in_channels = in_channels
+
+ self._init_layers()
+
+ def _init_layers(self):
+ """initialize conv layers in YOLOv5 head."""
+ self.convs_pred = nn.ModuleList()
+ for i in range(self.num_levels):
+ conv_pred = nn.Conv2d(self.in_channels[i],
+ self.num_base_priors * self.num_out_attrib,
+ 1)
+
+ self.convs_pred.append(conv_pred)
+
+ def init_weights(self):
+ """Initialize the bias of YOLOv5 head."""
+ super().init_weights()
+ for mi, s in zip(self.convs_pred, self.featmap_strides): # from
+ b = mi.bias.data.view(3, -1)
+ # obj (8 objects per 640 image)
+ b.data[:, 4] += math.log(8 / (640 / s)**2)
+ b.data[:, 5:] += math.log(0.6 / (self.num_classes - 0.999999))
+
+ mi.bias.data = b.view(-1)
+
+ def forward(self, x: Tuple[Tensor]) -> Tuple[List]:
+ """Forward features from the upstream network.
+
+ Args:
+ x (Tuple[Tensor]): Features from the upstream network, each is
+ a 4D-tensor.
+ Returns:
+ Tuple[List]: A tuple of multi-level classification scores, bbox
+ predictions, and objectnesses.
+ """
+ assert len(x) == self.num_levels
+ return multi_apply(self.forward_single, x, self.convs_pred)
+
+ def forward_single(self, x: Tensor,
+ convs: nn.Module) -> Tuple[Tensor, Tensor, Tensor]:
+ """Forward feature of a single scale level."""
+
+ pred_map = convs(x)
+ bs, _, ny, nx = pred_map.shape
+ pred_map = pred_map.view(bs, self.num_base_priors, self.num_out_attrib,
+ ny, nx)
+
+ cls_score = pred_map[:, :, 5:, ...].reshape(bs, -1, ny, nx)
+ bbox_pred = pred_map[:, :, :4, ...].reshape(bs, -1, ny, nx)
+ objectness = pred_map[:, :, 4:5, ...].reshape(bs, -1, ny, nx)
+
+ return cls_score, bbox_pred, objectness
+
+
+@MODELS.register_module()
+class YOLOv5Head(BaseDenseHead):
+ """YOLOv5Head head used in `YOLOv5`.
+
+ Args:
+ head_module(nn.Module): Base module used for YOLOv5Head
+ prior_generator(dict): Points generator feature maps in
+ 2D points-based detectors.
+ bbox_coder (:obj:`ConfigDict` or dict): Config of bbox coder.
+ loss_cls (:obj:`ConfigDict` or dict): Config of classification loss.
+ loss_bbox (:obj:`ConfigDict` or dict): Config of localization loss.
+ loss_obj (:obj:`ConfigDict` or dict): Config of objectness loss.
+ prior_match_thr (float): Defaults to 4.0.
+ obj_level_weights (List[float]): Defaults to [4.0, 1.0, 0.4].
+ train_cfg (:obj:`ConfigDict` or dict, optional): Training config of
+ anchor head. Defaults to None.
+ test_cfg (:obj:`ConfigDict` or dict, optional): Testing config of
+ anchor head. Defaults to None.
+ init_cfg (:obj:`ConfigDict` or list[:obj:`ConfigDict`] or dict or
+ list[dict], optional): Initialization config dict.
+ Defaults to None.
+ """
+
+ def __init__(self,
+ head_module: nn.Module,
+ prior_generator: ConfigType = dict(
+ type='mmdet.YOLOAnchorGenerator',
+ base_sizes=[[(10, 13), (16, 30), (33, 23)],
+ [(30, 61), (62, 45), (59, 119)],
+ [(116, 90), (156, 198), (373, 326)]],
+ strides=[8, 16, 32]),
+ bbox_coder: ConfigType = dict(type='YOLOv5BBoxCoder'),
+ loss_cls: ConfigType = dict(
+ type='mmdet.CrossEntropyLoss',
+ use_sigmoid=True,
+ reduction='mean',
+ loss_weight=0.5),
+ loss_bbox: ConfigType = dict(
+ type='IoULoss',
+ iou_mode='ciou',
+ bbox_format='xywh',
+ eps=1e-7,
+ reduction='mean',
+ loss_weight=0.05,
+ return_iou=True),
+ loss_obj: ConfigType = dict(
+ type='mmdet.CrossEntropyLoss',
+ use_sigmoid=True,
+ reduction='mean',
+ loss_weight=1.0),
+ prior_match_thr: float = 4.0,
+ obj_level_weights: List[float] = [4.0, 1.0, 0.4],
+ train_cfg: OptConfigType = None,
+ test_cfg: OptConfigType = None,
+ init_cfg: OptMultiConfig = None):
+ super().__init__(init_cfg=init_cfg)
+
+ self.head_module = MODELS.build(head_module)
+ self.num_classes = self.head_module.num_classes
+ self.featmap_strides = self.head_module.featmap_strides
+ self.num_levels = len(self.featmap_strides)
+
+ self.train_cfg = train_cfg
+ self.test_cfg = test_cfg
+
+ self.loss_cls: nn.Module = MODELS.build(loss_cls)
+ self.loss_bbox: nn.Module = MODELS.build(loss_bbox)
+ self.loss_obj: nn.Module = MODELS.build(loss_obj)
+
+ self.prior_generator = TASK_UTILS.build(prior_generator)
+ self.bbox_coder = TASK_UTILS.build(bbox_coder)
+ self.num_base_priors = self.prior_generator.num_base_priors[0]
+
+ self.featmap_sizes = [torch.empty(1)] * self.num_levels
+
+ self.prior_match_thr = prior_match_thr
+ self.obj_level_weights = obj_level_weights
+
+ self.special_init()
+
+ def special_init(self):
+ """Since YOLO series algorithms will inherit from YOLOv5Head, but
+ different algorithms have special initialization process.
+
+ The special_init function is designed to deal with this situation.
+ """
+ assert len(self.obj_level_weights) == len(
+ self.featmap_strides) == self.num_levels
+ if self.prior_match_thr != 4.0:
+ print_log("""!!!Now, you've changed the prior_match_thr
+ parameter to something other than 4.0. Please make sure
+ that you have modified both the regression formula in
+ bbox_coder and before loss_box computation,
+ otherwise the accuracy may be degraded!!!""")
+
+ priors_base_sizes = torch.tensor(
+ self.prior_generator.base_sizes, dtype=torch.float)
+ featmap_strides = torch.tensor(
+ self.featmap_strides, dtype=torch.float)[:, None, None]
+ self.register_buffer(
+ 'priors_base_sizes',
+ priors_base_sizes / featmap_strides,
+ persistent=False)
+
+ grid_offset = torch.tensor([
+ [0, 0], # center
+ [1, 0], # left
+ [0, 1], # up
+ [-1, 0], # right
+ [0, -1], # bottom
+ ]).float() * 0.5
+ self.register_buffer(
+ 'grid_offset', grid_offset[:, None], persistent=False)
+
+ prior_inds = torch.arange(self.num_base_priors).float().view(
+ self.num_base_priors, 1)
+ self.register_buffer('prior_inds', prior_inds, persistent=False)
+
+ def forward(self, x: Tuple[Tensor]) -> Tuple[List]:
+ """Forward features from the upstream network.
+
+ Args:
+ x (Tuple[Tensor]): Features from the upstream network, each is
+ a 4D-tensor.
+ Returns:
+ Tuple[List]: A tuple of multi-level classification scores, bbox
+ predictions, and objectnesses.
+ """
+ return self.head_module(x)
+
+ def predict_by_feat(self,
+ cls_scores: List[Tensor],
+ bbox_preds: List[Tensor],
+ objectnesses: Optional[List[Tensor]] = None,
+ batch_img_metas: Optional[List[dict]] = None,
+ cfg: Optional[ConfigDict] = None,
+ rescale: bool = True,
+ with_nms: bool = True) -> List[InstanceData]:
+ """Transform a batch of output features extracted by the head into
+ bbox results.
+ Args:
+ cls_scores (list[Tensor]): Classification scores for all
+ scale levels, each is a 4D-tensor, has shape
+ (batch_size, num_priors * num_classes, H, W).
+ bbox_preds (list[Tensor]): Box energies / deltas for all
+ scale levels, each is a 4D-tensor, has shape
+ (batch_size, num_priors * 4, H, W).
+ objectnesses (list[Tensor], Optional): Score factor for
+ all scale level, each is a 4D-tensor, has shape
+ (batch_size, 1, H, W).
+ batch_img_metas (list[dict], Optional): Batch image meta info.
+ Defaults to None.
+ cfg (ConfigDict, optional): Test / postprocessing
+ configuration, if None, test_cfg would be used.
+ Defaults to None.
+ rescale (bool): If True, return boxes in original image space.
+ Defaults to False.
+ with_nms (bool): If True, do nms before return boxes.
+ Defaults to True.
+
+ Returns:
+ list[:obj:`InstanceData`]: Object detection results of each image
+ after the post process. Each item usually contains following keys.
+
+ - scores (Tensor): Classification scores, has a shape
+ (num_instance, )
+ - labels (Tensor): Labels of bboxes, has a shape
+ (num_instances, ).
+ - bboxes (Tensor): Has a shape (num_instances, 4),
+ the last dimension 4 arrange as (x1, y1, x2, y2).
+ """
+ assert len(cls_scores) == len(bbox_preds)
+ if objectnesses is None:
+ with_objectnesses = False
+ else:
+ with_objectnesses = True
+ assert len(cls_scores) == len(objectnesses)
+
+ cfg = self.test_cfg if cfg is None else cfg
+ cfg = copy.deepcopy(cfg)
+
+ multi_label = cfg.multi_label
+ multi_label &= self.num_classes > 1
+ cfg.multi_label = multi_label
+
+ num_imgs = len(batch_img_metas)
+ featmap_sizes = [cls_score.shape[2:] for cls_score in cls_scores]
+
+ # If the shape does not change, use the previous mlvl_priors
+ if featmap_sizes != self.featmap_sizes:
+ self.mlvl_priors = self.prior_generator.grid_priors(
+ featmap_sizes,
+ dtype=cls_scores[0].dtype,
+ device=cls_scores[0].device)
+ self.featmap_sizes = featmap_sizes
+ flatten_priors = torch.cat(self.mlvl_priors)
+
+ mlvl_strides = [
+ flatten_priors.new_full(
+ (featmap_size.numel() * self.num_base_priors, ), stride) for
+ featmap_size, stride in zip(featmap_sizes, self.featmap_strides)
+ ]
+ flatten_stride = torch.cat(mlvl_strides)
+
+ # flatten cls_scores, bbox_preds and objectness
+ flatten_cls_scores = [
+ cls_score.permute(0, 2, 3, 1).reshape(num_imgs, -1,
+ self.num_classes)
+ for cls_score in cls_scores
+ ]
+ flatten_bbox_preds = [
+ bbox_pred.permute(0, 2, 3, 1).reshape(num_imgs, -1, 4)
+ for bbox_pred in bbox_preds
+ ]
+
+ flatten_cls_scores = torch.cat(flatten_cls_scores, dim=1).sigmoid()
+ flatten_bbox_preds = torch.cat(flatten_bbox_preds, dim=1)
+ flatten_decoded_bboxes = self.bbox_coder.decode(
+ flatten_priors[None], flatten_bbox_preds, flatten_stride)
+
+ if with_objectnesses:
+ flatten_objectness = [
+ objectness.permute(0, 2, 3, 1).reshape(num_imgs, -1)
+ for objectness in objectnesses
+ ]
+ flatten_objectness = torch.cat(flatten_objectness, dim=1).sigmoid()
+ else:
+ flatten_objectness = [None for _ in range(len(featmap_sizes))]
+
+ results_list = []
+ for (bboxes, scores, objectness,
+ img_meta) in zip(flatten_decoded_bboxes, flatten_cls_scores,
+ flatten_objectness, batch_img_metas):
+ ori_shape = img_meta['ori_shape']
+ scale_factor = img_meta['scale_factor']
+ if 'pad_param' in img_meta:
+ pad_param = img_meta['pad_param']
+ else:
+ pad_param = None
+
+ score_thr = cfg.get('score_thr', -1)
+ # yolox_style does not require the following operations
+ if objectness is not None and score_thr > 0 and not cfg.get(
+ 'yolox_style', False):
+ conf_inds = objectness > score_thr
+ bboxes = bboxes[conf_inds, :]
+ scores = scores[conf_inds, :]
+ objectness = objectness[conf_inds]
+
+ if objectness is not None:
+ # conf = obj_conf * cls_conf
+ scores *= objectness[:, None]
+
+ if scores.shape[0] == 0:
+ empty_results = InstanceData()
+ empty_results.bboxes = bboxes
+ empty_results.scores = scores[:, 0]
+ empty_results.labels = scores[:, 0].int()
+ results_list.append(empty_results)
+ continue
+
+ nms_pre = cfg.get('nms_pre', 100000)
+ if cfg.multi_label is False:
+ scores, labels = scores.max(1, keepdim=True)
+ scores, _, keep_idxs, results = filter_scores_and_topk(
+ scores,
+ score_thr,
+ nms_pre,
+ results=dict(labels=labels[:, 0]))
+ labels = results['labels']
+ else:
+ scores, labels, keep_idxs, _ = filter_scores_and_topk(
+ scores, score_thr, nms_pre)
+
+ results = InstanceData(
+ scores=scores, labels=labels, bboxes=bboxes[keep_idxs])
+
+ if rescale:
+ if pad_param is not None:
+ results.bboxes -= results.bboxes.new_tensor([
+ pad_param[2], pad_param[0], pad_param[2], pad_param[0]
+ ])
+ results.bboxes /= results.bboxes.new_tensor(
+ scale_factor).repeat((1, 2))
+
+ if cfg.get('yolox_style', False):
+ # do not need max_per_img
+ cfg.max_per_img = len(results)
+
+ results = self._bbox_post_process(
+ results=results,
+ cfg=cfg,
+ rescale=False,
+ with_nms=with_nms,
+ img_meta=img_meta)
+ results.bboxes[:, 0::2].clamp_(0, ori_shape[1])
+ results.bboxes[:, 1::2].clamp_(0, ori_shape[0])
+
+ results_list.append(results)
+ return results_list
+
+ def loss(self, x: Tuple[Tensor], batch_data_samples: Union[list,
+ dict]) -> dict:
+ """Perform forward propagation and loss calculation of the detection
+ head on the features of the upstream network.
+
+ Args:
+ x (tuple[Tensor]): Features from the upstream network, each is
+ a 4D-tensor.
+ batch_data_samples (List[:obj:`DetDataSample`], dict): The Data
+ Samples. It usually includes information such as
+ `gt_instance`, `gt_panoptic_seg` and `gt_sem_seg`.
+
+ Returns:
+ dict: A dictionary of loss components.
+ """
+ outs = self(x)
+
+ if isinstance(batch_data_samples, list):
+ losses = super().loss(x, batch_data_samples)
+ else:
+ # Fast version
+ loss_inputs = outs + (batch_data_samples['bboxes_labels'],
+ batch_data_samples['img_metas'])
+ losses = self.loss_by_feat(*loss_inputs)
+
+ return losses
+
+ def loss_by_feat(
+ self,
+ cls_scores: Sequence[Tensor],
+ bbox_preds: Sequence[Tensor],
+ objectnesses: Sequence[Tensor],
+ batch_gt_instances: Sequence[InstanceData],
+ batch_img_metas: Sequence[dict],
+ batch_gt_instances_ignore: OptInstanceList = None) -> dict:
+ """Calculate the loss based on the features extracted by the detection
+ head.
+
+ Args:
+ cls_scores (Sequence[Tensor]): Box scores for each scale level,
+ each is a 4D-tensor, the channel number is
+ num_priors * num_classes.
+ bbox_preds (Sequence[Tensor]): Box energies / deltas for each scale
+ level, each is a 4D-tensor, the channel number is
+ num_priors * 4.
+ objectnesses (Sequence[Tensor]): Score factor for
+ all scale level, each is a 4D-tensor, has shape
+ (batch_size, 1, H, W).
+ batch_gt_instances (Sequence[InstanceData]): Batch of
+ gt_instance. It usually includes ``bboxes`` and ``labels``
+ attributes.
+ batch_img_metas (Sequence[dict]): Meta information of each image,
+ e.g., image size, scaling factor, etc.
+ batch_gt_instances_ignore (list[:obj:`InstanceData`], optional):
+ Batch of gt_instances_ignore. It includes ``bboxes`` attribute
+ data that is ignored during training and testing.
+ Defaults to None.
+ Returns:
+ dict[str, Tensor]: A dictionary of losses.
+ """
+ # 1. Convert gt to norm format
+ batch_targets_normed = self._convert_gt_to_norm_format(
+ batch_gt_instances, batch_img_metas)
+
+ device = cls_scores[0].device
+ loss_cls = torch.zeros(1, device=device)
+ loss_box = torch.zeros(1, device=device)
+ loss_obj = torch.zeros(1, device=device)
+ scaled_factor = torch.ones(7, device=device)
+
+ for i in range(self.num_levels):
+ batch_size, _, h, w = bbox_preds[i].shape
+ target_obj = torch.zeros_like(objectnesses[i])
+
+ # empty gt bboxes
+ if batch_targets_normed.shape[1] == 0:
+ loss_box += bbox_preds[i].sum() * 0
+ loss_cls += cls_scores[i].sum() * 0
+ loss_obj += self.loss_obj(
+ objectnesses[i], target_obj) * self.obj_level_weights[i]
+ continue
+
+ priors_base_sizes_i = self.priors_base_sizes[i]
+ # feature map scale whwh
+ scaled_factor[2:6] = torch.tensor(
+ bbox_preds[i].shape)[[3, 2, 3, 2]]
+ # Scale batch_targets from range 0-1 to range 0-features_maps size.
+ # (num_base_priors, num_bboxes, 7)
+ batch_targets_scaled = batch_targets_normed * scaled_factor
+
+ # 2. Shape match
+ wh_ratio = batch_targets_scaled[...,
+ 4:6] / priors_base_sizes_i[:, None]
+ match_inds = torch.max(
+ wh_ratio, 1 / wh_ratio).max(2)[0] < self.prior_match_thr
+ batch_targets_scaled = batch_targets_scaled[match_inds]
+
+ # no gt bbox matches anchor
+ if batch_targets_scaled.shape[0] == 0:
+ loss_box += bbox_preds[i].sum() * 0
+ loss_cls += cls_scores[i].sum() * 0
+ loss_obj += self.loss_obj(
+ objectnesses[i], target_obj) * self.obj_level_weights[i]
+ continue
+
+ # 3. Positive samples with additional neighbors
+
+ # check the left, up, right, bottom sides of the
+ # targets grid, and determine whether assigned
+ # them as positive samples as well.
+ batch_targets_cxcy = batch_targets_scaled[:, 2:4]
+ grid_xy = scaled_factor[[2, 3]] - batch_targets_cxcy
+ left, up = ((batch_targets_cxcy % 1 < 0.5) &
+ (batch_targets_cxcy > 1)).T
+ right, bottom = ((grid_xy % 1 < 0.5) & (grid_xy > 1)).T
+ offset_inds = torch.stack(
+ (torch.ones_like(left), left, up, right, bottom))
+
+ batch_targets_scaled = batch_targets_scaled.repeat(
+ (5, 1, 1))[offset_inds]
+ retained_offsets = self.grid_offset.repeat(1, offset_inds.shape[1],
+ 1)[offset_inds]
+
+ # prepare pred results and positive sample indexes to
+ # calculate class loss and bbox lo
+ _chunk_targets = batch_targets_scaled.chunk(4, 1)
+ img_class_inds, grid_xy, grid_wh, priors_inds = _chunk_targets
+ priors_inds, (img_inds, class_inds) = priors_inds.long().view(
+ -1), img_class_inds.long().T
+
+ grid_xy_long = (grid_xy - retained_offsets).long()
+ grid_x_inds, grid_y_inds = grid_xy_long.T
+ bboxes_targets = torch.cat((grid_xy - grid_xy_long, grid_wh), 1)
+
+ # 4. Calculate loss
+ # bbox loss
+ retained_bbox_pred = bbox_preds[i].reshape(
+ batch_size, self.num_base_priors, -1, h,
+ w)[img_inds, priors_inds, :, grid_y_inds, grid_x_inds]
+ priors_base_sizes_i = priors_base_sizes_i[priors_inds]
+ decoded_bbox_pred = self._decode_bbox_to_xywh(
+ retained_bbox_pred, priors_base_sizes_i)
+ loss_box_i, iou = self.loss_bbox(decoded_bbox_pred, bboxes_targets)
+ loss_box += loss_box_i
+
+ # obj loss
+ iou = iou.detach().clamp(0)
+ target_obj[img_inds, priors_inds, grid_y_inds,
+ grid_x_inds] = iou.type(target_obj.dtype)
+ loss_obj += self.loss_obj(objectnesses[i],
+ target_obj) * self.obj_level_weights[i]
+
+ # cls loss
+ if self.num_classes > 1:
+ pred_cls_scores = cls_scores[i].reshape(
+ batch_size, self.num_base_priors, -1, h,
+ w)[img_inds, priors_inds, :, grid_y_inds, grid_x_inds]
+
+ target_class = torch.full_like(pred_cls_scores, 0.)
+ target_class[range(batch_targets_scaled.shape[0]),
+ class_inds] = 1.
+ loss_cls += self.loss_cls(pred_cls_scores, target_class)
+ else:
+ loss_cls += cls_scores[i].sum() * 0
+
+ _, world_size = get_dist_info()
+ return dict(
+ loss_cls=loss_cls * batch_size * world_size,
+ loss_obj=loss_obj * batch_size * world_size,
+ loss_bbox=loss_box * batch_size * world_size)
+
+ def _convert_gt_to_norm_format(self,
+ batch_gt_instances: Sequence[InstanceData],
+ batch_img_metas: Sequence[dict]) -> Tensor:
+ if isinstance(batch_gt_instances, torch.Tensor):
+ # fast version
+ img_shape = batch_img_metas[0]['batch_input_shape']
+ gt_bboxes_xyxy = batch_gt_instances[:, 2:]
+ xy1, xy2 = gt_bboxes_xyxy.split((2, 2), dim=-1)
+ gt_bboxes_xywh = torch.cat([(xy2 + xy1) / 2, (xy2 - xy1)], dim=-1)
+ gt_bboxes_xywh[:, 1::2] /= img_shape[0]
+ gt_bboxes_xywh[:, 0::2] /= img_shape[1]
+ batch_gt_instances[:, 2:] = gt_bboxes_xywh
+
+ # (num_base_priors, num_bboxes, 6)
+ batch_targets_normed = batch_gt_instances.repeat(
+ self.num_base_priors, 1, 1)
+ else:
+ batch_target_list = []
+ # Convert xyxy bbox to yolo format.
+ for i, gt_instances in enumerate(batch_gt_instances):
+ img_shape = batch_img_metas[i]['batch_input_shape']
+ bboxes = gt_instances.bboxes
+ labels = gt_instances.labels
+
+ xy1, xy2 = bboxes.split((2, 2), dim=-1)
+ bboxes = torch.cat([(xy2 + xy1) / 2, (xy2 - xy1)], dim=-1)
+ # normalized to 0-1
+ bboxes[:, 1::2] /= img_shape[0]
+ bboxes[:, 0::2] /= img_shape[1]
+
+ index = bboxes.new_full((len(bboxes), 1), i)
+ # (batch_idx, label, normed_bbox)
+ target = torch.cat((index, labels[:, None].float(), bboxes),
+ dim=1)
+ batch_target_list.append(target)
+
+ # (num_base_priors, num_bboxes, 6)
+ batch_targets_normed = torch.cat(
+ batch_target_list, dim=0).repeat(self.num_base_priors, 1, 1)
+
+ # (num_base_priors, num_bboxes, 1)
+ batch_targets_prior_inds = self.prior_inds.repeat(
+ 1, batch_targets_normed.shape[1])[..., None]
+ # (num_base_priors, num_bboxes, 7)
+ # (img_ind, labels, bbox_cx, bbox_cy, bbox_w, bbox_h, prior_ind)
+ batch_targets_normed = torch.cat(
+ (batch_targets_normed, batch_targets_prior_inds), 2)
+ return batch_targets_normed
+
+ def _decode_bbox_to_xywh(self, bbox_pred, priors_base_sizes) -> Tensor:
+ bbox_pred = bbox_pred.sigmoid()
+ pred_xy = bbox_pred[:, :2] * 2 - 0.5
+ pred_wh = (bbox_pred[:, 2:] * 2)**2 * priors_base_sizes
+ decoded_bbox_pred = torch.cat((pred_xy, pred_wh), dim=-1)
+ return decoded_bbox_pred