diff --git a/.dev_scripts/gather_models.py b/.dev_scripts/gather_models.py
index ba5039c2..f05e2b5b 100644
--- a/.dev_scripts/gather_models.py
+++ b/.dev_scripts/gather_models.py
@@ -108,6 +108,7 @@ def get_dataset_name(config):
name_map = dict(
CityscapesDataset='Cityscapes',
CocoDataset='COCO',
+ PoseCocoDataset='COCO Person',
YOLOv5CocoDataset='COCO',
CocoPanopticDataset='COCO',
YOLOv5DOTADataset='DOTA 1.0',
diff --git a/configs/_base_/pose/coco.py b/configs/_base_/pose/coco.py
new file mode 100644
index 00000000..865a95bc
--- /dev/null
+++ b/configs/_base_/pose/coco.py
@@ -0,0 +1,181 @@
+dataset_info = dict(
+ dataset_name='coco',
+ paper_info=dict(
+ author='Lin, Tsung-Yi and Maire, Michael and '
+ 'Belongie, Serge and Hays, James and '
+ 'Perona, Pietro and Ramanan, Deva and '
+ r'Doll{\'a}r, Piotr and Zitnick, C Lawrence',
+ title='Microsoft coco: Common objects in context',
+ container='European conference on computer vision',
+ year='2014',
+ homepage='http://cocodataset.org/',
+ ),
+ keypoint_info={
+ 0:
+ dict(name='nose', id=0, color=[51, 153, 255], type='upper', swap=''),
+ 1:
+ dict(
+ name='left_eye',
+ id=1,
+ color=[51, 153, 255],
+ type='upper',
+ swap='right_eye'),
+ 2:
+ dict(
+ name='right_eye',
+ id=2,
+ color=[51, 153, 255],
+ type='upper',
+ swap='left_eye'),
+ 3:
+ dict(
+ name='left_ear',
+ id=3,
+ color=[51, 153, 255],
+ type='upper',
+ swap='right_ear'),
+ 4:
+ dict(
+ name='right_ear',
+ id=4,
+ color=[51, 153, 255],
+ type='upper',
+ swap='left_ear'),
+ 5:
+ dict(
+ name='left_shoulder',
+ id=5,
+ color=[0, 255, 0],
+ type='upper',
+ swap='right_shoulder'),
+ 6:
+ dict(
+ name='right_shoulder',
+ id=6,
+ color=[255, 128, 0],
+ type='upper',
+ swap='left_shoulder'),
+ 7:
+ dict(
+ name='left_elbow',
+ id=7,
+ color=[0, 255, 0],
+ type='upper',
+ swap='right_elbow'),
+ 8:
+ dict(
+ name='right_elbow',
+ id=8,
+ color=[255, 128, 0],
+ type='upper',
+ swap='left_elbow'),
+ 9:
+ dict(
+ name='left_wrist',
+ id=9,
+ color=[0, 255, 0],
+ type='upper',
+ swap='right_wrist'),
+ 10:
+ dict(
+ name='right_wrist',
+ id=10,
+ color=[255, 128, 0],
+ type='upper',
+ swap='left_wrist'),
+ 11:
+ dict(
+ name='left_hip',
+ id=11,
+ color=[0, 255, 0],
+ type='lower',
+ swap='right_hip'),
+ 12:
+ dict(
+ name='right_hip',
+ id=12,
+ color=[255, 128, 0],
+ type='lower',
+ swap='left_hip'),
+ 13:
+ dict(
+ name='left_knee',
+ id=13,
+ color=[0, 255, 0],
+ type='lower',
+ swap='right_knee'),
+ 14:
+ dict(
+ name='right_knee',
+ id=14,
+ color=[255, 128, 0],
+ type='lower',
+ swap='left_knee'),
+ 15:
+ dict(
+ name='left_ankle',
+ id=15,
+ color=[0, 255, 0],
+ type='lower',
+ swap='right_ankle'),
+ 16:
+ dict(
+ name='right_ankle',
+ id=16,
+ color=[255, 128, 0],
+ type='lower',
+ swap='left_ankle')
+ },
+ skeleton_info={
+ 0:
+ dict(link=('left_ankle', 'left_knee'), id=0, color=[0, 255, 0]),
+ 1:
+ dict(link=('left_knee', 'left_hip'), id=1, color=[0, 255, 0]),
+ 2:
+ dict(link=('right_ankle', 'right_knee'), id=2, color=[255, 128, 0]),
+ 3:
+ dict(link=('right_knee', 'right_hip'), id=3, color=[255, 128, 0]),
+ 4:
+ dict(link=('left_hip', 'right_hip'), id=4, color=[51, 153, 255]),
+ 5:
+ dict(link=('left_shoulder', 'left_hip'), id=5, color=[51, 153, 255]),
+ 6:
+ dict(link=('right_shoulder', 'right_hip'), id=6, color=[51, 153, 255]),
+ 7:
+ dict(
+ link=('left_shoulder', 'right_shoulder'),
+ id=7,
+ color=[51, 153, 255]),
+ 8:
+ dict(link=('left_shoulder', 'left_elbow'), id=8, color=[0, 255, 0]),
+ 9:
+ dict(
+ link=('right_shoulder', 'right_elbow'), id=9, color=[255, 128, 0]),
+ 10:
+ dict(link=('left_elbow', 'left_wrist'), id=10, color=[0, 255, 0]),
+ 11:
+ dict(link=('right_elbow', 'right_wrist'), id=11, color=[255, 128, 0]),
+ 12:
+ dict(link=('left_eye', 'right_eye'), id=12, color=[51, 153, 255]),
+ 13:
+ dict(link=('nose', 'left_eye'), id=13, color=[51, 153, 255]),
+ 14:
+ dict(link=('nose', 'right_eye'), id=14, color=[51, 153, 255]),
+ 15:
+ dict(link=('left_eye', 'left_ear'), id=15, color=[51, 153, 255]),
+ 16:
+ dict(link=('right_eye', 'right_ear'), id=16, color=[51, 153, 255]),
+ 17:
+ dict(link=('left_ear', 'left_shoulder'), id=17, color=[51, 153, 255]),
+ 18:
+ dict(
+ link=('right_ear', 'right_shoulder'), id=18, color=[51, 153, 255])
+ },
+ joint_weights=[
+ 1., 1., 1., 1., 1., 1., 1., 1.2, 1.2, 1.5, 1.5, 1., 1., 1.2, 1.2, 1.5,
+ 1.5
+ ],
+ sigmas=[
+ 0.026, 0.025, 0.025, 0.035, 0.035, 0.079, 0.079, 0.072, 0.072, 0.062,
+ 0.062, 0.107, 0.107, 0.087, 0.087, 0.089, 0.089
+ ])
diff --git a/configs/yolox/README.md b/configs/yolox/README.md
index e646dd20..7d5dc683 100644
--- a/configs/yolox/README.md
+++ b/configs/yolox/README.md
@@ -45,6 +45,35 @@ The modified training parameters are as follows:
1. The test score threshold is 0.001.
2. Due to the need for pre-training weights, we cannot reproduce the performance of the `yolox-nano` model. Please refer to https://github.com/Megvii-BaseDetection/YOLOX/issues/674 for more information.
+## YOLOX-Pose
+
+Based on [MMPose](https://github.com/open-mmlab/mmpose/blob/main/projects/yolox-pose/README.md), we have implemented a YOLOX-based human pose estimator, utilizing the approach outlined in **YOLO-Pose: Enhancing YOLO for Multi Person Pose Estimation Using Object Keypoint Similarity Loss (CVPRW 2022)**. This pose estimator is lightweight and quick, making it well-suited for crowded scenes.
+
+
+
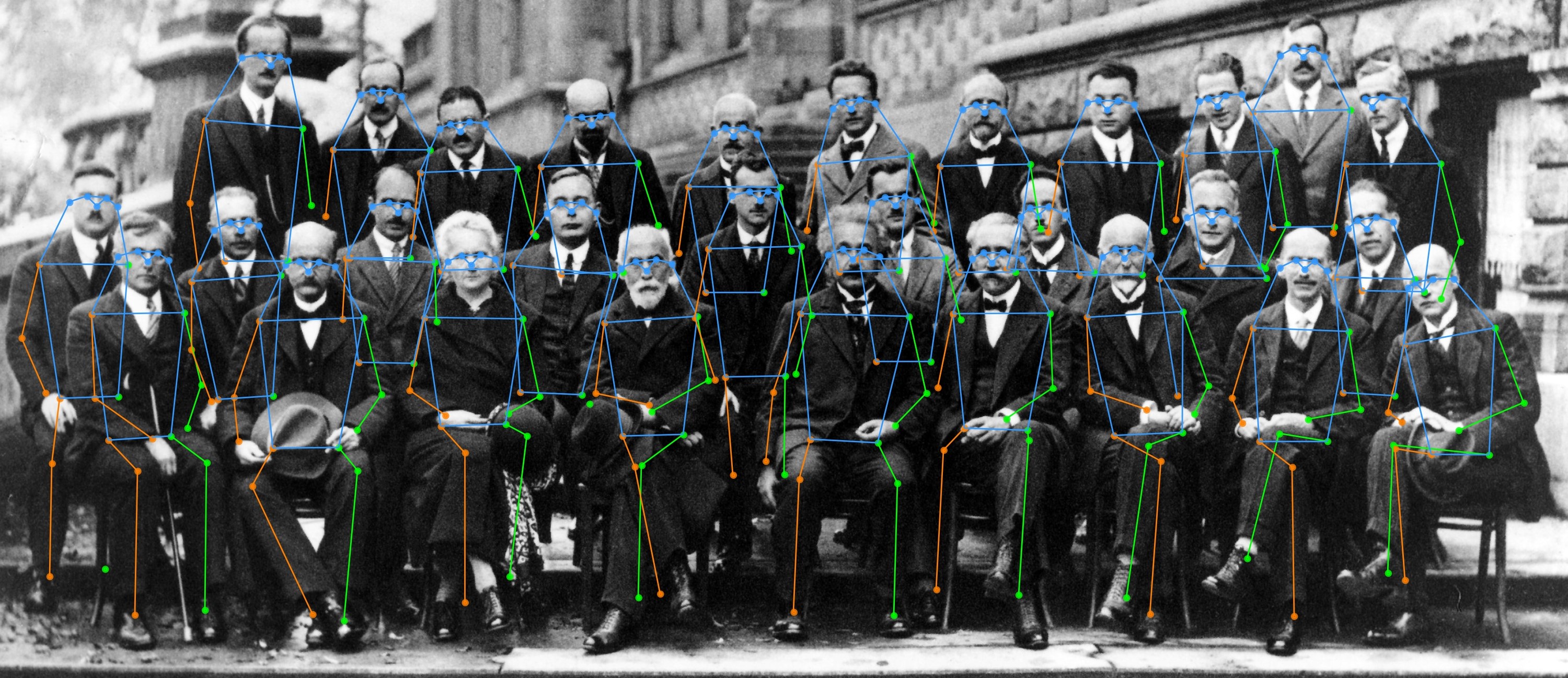
+
+
+### Results
+
+| Backbone | Size | Batch Size | AMP | RTMDet-Hyp | Mem (GB) | AP | Config | Download |
+| :--------: | :--: | :--------: | :-: | :--------: | :------: | :--: | :------------------------------------------------------------: | :----------------------------------------------------------------------------------------------------------------------------------------------------------------------------------------------------------------------------------------------------------------------------------------------------------------------------------------------------------: |
+| YOLOX-tiny | 416 | 8xb32 | Yes | Yes | 5.3 | 52.8 | [config](./pose/yolox-pose_tiny_8xb32-300e-rtmdet-hyp_coco.py) | [model](https://download.openmmlab.com/mmyolo/v0/yolox/pose/yolox-pose_tiny_8xb32-300e-rtmdet-hyp_coco/yolox-pose_tiny_8xb32-300e-rtmdet-hyp_coco_20230427_080351-2117af67.pth) \| [log](https://download.openmmlab.com/mmyolo/v0/yolox/pose/yolox-pose_tiny_8xb32-300e-rtmdet-hyp_coco/yolox-pose_tiny_8xb32-300e-rtmdet-hyp_coco_20230427_080351.log.json) |
+| YOLOX-s | 640 | 8xb32 | Yes | Yes | 10.7 | 63.7 | [config](./pose/yolox-pose_s_8xb32-300e-rtmdet-hyp_coco.py) | [model](https://download.openmmlab.com/mmyolo/v0/yolox/pose/yolox-pose_s_8xb32-300e-rtmdet-hyp_coco/yolox-pose_s_8xb32-300e-rtmdet-hyp_coco_20230427_005150-e87d843a.pth) \| [log](https://download.openmmlab.com/mmyolo/v0/yolox/pose/yolox-pose_s_8xb32-300e-rtmdet-hyp_coco/yolox-pose_s_8xb32-300e-rtmdet-hyp_coco_20230427_005150.log.json) |
+| YOLOX-m | 640 | 8xb32 | Yes | Yes | 19.2 | 69.3 | [config](./pose/yolox-pose_m_8xb32-300e-rtmdet-hyp_coco.py) | [model](https://download.openmmlab.com/mmyolo/v0/yolox/pose/yolox-pose_m_8xb32-300e-rtmdet-hyp_coco/yolox-pose_m_8xb32-300e-rtmdet-hyp_coco_20230427_094024-bbeacc1c.pth) \| [log](https://download.openmmlab.com/mmyolo/v0/yolox/pose/yolox-pose_m_8xb32-300e-rtmdet-hyp_coco/yolox-pose_m_8xb32-300e-rtmdet-hyp_coco_20230427_094024.log.json) |
+| YOLOX-l | 640 | 8xb32 | Yes | Yes | 30.3 | 71.1 | [config](./pose/yolox-pose_l_8xb32-300e-rtmdet-hyp_coco.py) | [model](https://download.openmmlab.com/mmyolo/v0/yolox/pose/yolox-pose_l_8xb32-300e-rtmdet-hyp_coco/yolox-pose_l_8xb32-300e-rtmdet-hyp_coco_20230427_041140-82d65ac8.pth) \| [log](https://download.openmmlab.com/mmyolo/v0/yolox/pose/yolox-pose_l_8xb32-300e-rtmdet-hyp_coco/yolox-pose_l_8xb32-300e-rtmdet-hyp_coco_20230427_041140.log.json) |
+
+**Note**
+
+1. The performance is unstable and may fluctuate and the highest performance weight in `COCO` training may not be the last epoch. The performance shown above is the best model.
+
+### Installation
+
+Install MMPose
+
+```
+mim install -r requirements/mmpose.txt
+```
+
## Citation
```latex
diff --git a/configs/yolox/metafile.yml b/configs/yolox/metafile.yml
index 0926519e..78ede704 100644
--- a/configs/yolox/metafile.yml
+++ b/configs/yolox/metafile.yml
@@ -116,3 +116,51 @@ Models:
Metrics:
box AP: 47.5
Weights: https://download.openmmlab.com/mmyolo/v0/yolox/yolox_m_fast_8xb32-300e-rtmdet-hyp_coco/yolox_m_fast_8xb32-300e-rtmdet-hyp_coco_20230210_144328-e657e182.pth
+ - Name: yolox-pose_tiny_8xb32-300e-rtmdet-hyp_coco
+ In Collection: YOLOX
+ Config: yolox-pose_tiny_8xb32-300e-rtmdet-hyp_coco.py
+ Metadata:
+ Training Memory (GB): 5.3
+ Epochs: 300
+ Results:
+ - Task: Human Pose Estimation
+ Dataset: COCO
+ Metrics:
+ AP: 52.8
+ Weights: https://download.openmmlab.com/mmyolo/v0/yolox/pose/yolox-pose_tiny_8xb32-300e-rtmdet-hyp_coco/yolox-pose_tiny_8xb32-300e-rtmdet-hyp_coco_20230427_080351-2117af67.pth
+ - Name: yolox-pose_s_8xb32-300e-rtmdet-hyp_coco
+ In Collection: YOLOX
+ Config: yolox-pose_s_8xb32-300e-rtmdet-hyp_coco.py
+ Metadata:
+ Training Memory (GB): 10.7
+ Epochs: 300
+ Results:
+ - Task: Human Pose Estimation
+ Dataset: COCO
+ Metrics:
+ AP: 63.7
+ Weights: https://download.openmmlab.com/mmyolo/v0/yolox/pose/yolox-pose_s_8xb32-300e-rtmdet-hyp_coco/yolox-pose_s_8xb32-300e-rtmdet-hyp_coco_20230427_005150-e87d843a.pth
+ - Name: yolox-pose_m_8xb32-300e-rtmdet-hyp_coco
+ In Collection: YOLOX
+ Config: yolox-pose_m_8xb32-300e-rtmdet-hyp_coco.py
+ Metadata:
+ Training Memory (GB): 19.2
+ Epochs: 300
+ Results:
+ - Task: Human Pose Estimation
+ Dataset: COCO
+ Metrics:
+ AP: 69.3
+ Weights: https://download.openmmlab.com/mmyolo/v0/yolox/pose/yolox-pose_m_8xb32-300e-rtmdet-hyp_coco/yolox-pose_m_8xb32-300e-rtmdet-hyp_coco_20230427_094024-bbeacc1c.pth
+ - Name: yolox-pose_l_8xb32-300e-rtmdet-hyp_coco
+ In Collection: YOLOX
+ Config: yolox-pose_l_8xb32-300e-rtmdet-hyp_coco.py
+ Metadata:
+ Training Memory (GB): 30.3
+ Epochs: 300
+ Results:
+ - Task: Human Pose Estimation
+ Dataset: COCO
+ Metrics:
+ AP: 71.1
+ Weights: https://download.openmmlab.com/mmyolo/v0/yolox/pose/yolox-pose_l_8xb32-300e-rtmdet-hyp_coco/yolox-pose_l_8xb32-300e-rtmdet-hyp_coco_20230427_041140-82d65ac8.pth
diff --git a/configs/yolox/pose/yolox-pose_l_8xb32-300e-rtmdet-hyp_coco.py b/configs/yolox/pose/yolox-pose_l_8xb32-300e-rtmdet-hyp_coco.py
new file mode 100644
index 00000000..96de5e98
--- /dev/null
+++ b/configs/yolox/pose/yolox-pose_l_8xb32-300e-rtmdet-hyp_coco.py
@@ -0,0 +1,14 @@
+_base_ = ['./yolox-pose_m_8xb32-300e-rtmdet-hyp_coco.py']
+
+load_from = 'https://download.openmmlab.com/mmyolo/v0/yolox/yolox_l_fast_8xb8-300e_coco/yolox_l_fast_8xb8-300e_coco_20230213_160715-c731eb1c.pth' # noqa
+
+# ========================modified parameters======================
+deepen_factor = 1.0
+widen_factor = 1.0
+
+# =======================Unmodified in most cases==================
+# model settings
+model = dict(
+ backbone=dict(deepen_factor=deepen_factor, widen_factor=widen_factor),
+ neck=dict(deepen_factor=deepen_factor, widen_factor=widen_factor),
+ bbox_head=dict(head_module=dict(widen_factor=widen_factor)))
diff --git a/configs/yolox/pose/yolox-pose_m_8xb32-300e-rtmdet-hyp_coco.py b/configs/yolox/pose/yolox-pose_m_8xb32-300e-rtmdet-hyp_coco.py
new file mode 100644
index 00000000..f78d6a3a
--- /dev/null
+++ b/configs/yolox/pose/yolox-pose_m_8xb32-300e-rtmdet-hyp_coco.py
@@ -0,0 +1,14 @@
+_base_ = ['./yolox-pose_s_8xb32-300e-rtmdet-hyp_coco.py']
+
+load_from = 'https://download.openmmlab.com/mmyolo/v0/yolox/yolox_m_fast_8xb32-300e-rtmdet-hyp_coco/yolox_m_fast_8xb32-300e-rtmdet-hyp_coco_20230210_144328-e657e182.pth' # noqa
+
+# ========================modified parameters======================
+deepen_factor = 0.67
+widen_factor = 0.75
+
+# =======================Unmodified in most cases==================
+# model settings
+model = dict(
+ backbone=dict(deepen_factor=deepen_factor, widen_factor=widen_factor),
+ neck=dict(deepen_factor=deepen_factor, widen_factor=widen_factor),
+ bbox_head=dict(head_module=dict(widen_factor=widen_factor)))
diff --git a/configs/yolox/pose/yolox-pose_s_8xb32-300e-rtmdet-hyp_coco.py b/configs/yolox/pose/yolox-pose_s_8xb32-300e-rtmdet-hyp_coco.py
new file mode 100644
index 00000000..8fa2172c
--- /dev/null
+++ b/configs/yolox/pose/yolox-pose_s_8xb32-300e-rtmdet-hyp_coco.py
@@ -0,0 +1,136 @@
+_base_ = '../yolox_s_fast_8xb32-300e-rtmdet-hyp_coco.py'
+
+load_from = 'https://download.openmmlab.com/mmyolo/v0/yolox/yolox_s_fast_8xb32-300e-rtmdet-hyp_coco/yolox_s_fast_8xb32-300e-rtmdet-hyp_coco_20230210_134645-3a8dfbd7.pth' # noqa
+
+num_keypoints = 17
+scaling_ratio_range = (0.75, 1.0)
+mixup_ratio_range = (0.8, 1.6)
+num_last_epochs = 20
+
+# model settings
+model = dict(
+ bbox_head=dict(
+ type='YOLOXPoseHead',
+ head_module=dict(
+ type='YOLOXPoseHeadModule',
+ num_classes=1,
+ num_keypoints=num_keypoints,
+ ),
+ loss_pose=dict(
+ type='OksLoss',
+ metainfo='configs/_base_/pose/coco.py',
+ loss_weight=30.0)),
+ train_cfg=dict(
+ assigner=dict(
+ type='PoseSimOTAAssigner',
+ center_radius=2.5,
+ oks_weight=3.0,
+ iou_calculator=dict(type='mmdet.BboxOverlaps2D'),
+ oks_calculator=dict(
+ type='OksLoss', metainfo='configs/_base_/pose/coco.py'))),
+ test_cfg=dict(score_thr=0.01))
+
+# pipelines
+pre_transform = [
+ dict(type='LoadImageFromFile', backend_args=_base_.backend_args),
+ dict(type='LoadAnnotations', with_keypoints=True)
+]
+
+img_scale = _base_.img_scale
+
+train_pipeline_stage1 = [
+ *pre_transform,
+ dict(
+ type='Mosaic',
+ img_scale=img_scale,
+ pad_val=114.0,
+ pre_transform=pre_transform),
+ dict(
+ type='RandomAffine',
+ scaling_ratio_range=scaling_ratio_range,
+ border=(-img_scale[0] // 2, -img_scale[1] // 2)),
+ dict(
+ type='YOLOXMixUp',
+ img_scale=img_scale,
+ ratio_range=mixup_ratio_range,
+ pad_val=114.0,
+ pre_transform=pre_transform),
+ dict(type='mmdet.YOLOXHSVRandomAug'),
+ dict(type='RandomFlip', prob=0.5),
+ dict(type='FilterAnnotations', by_keypoints=True, keep_empty=False),
+ dict(
+ type='PackDetInputs',
+ meta_keys=('img_id', 'img_path', 'ori_shape', 'img_shape'))
+]
+
+train_pipeline_stage2 = [
+ *pre_transform,
+ dict(type='Resize', scale=img_scale, keep_ratio=True),
+ dict(
+ type='mmdet.Pad',
+ pad_to_square=True,
+ pad_val=dict(img=(114.0, 114.0, 114.0))),
+ dict(type='mmdet.YOLOXHSVRandomAug'),
+ dict(type='RandomFlip', prob=0.5),
+ dict(type='FilterAnnotations', by_keypoints=True, keep_empty=False),
+ dict(type='PackDetInputs')
+]
+
+test_pipeline = [
+ *pre_transform,
+ dict(type='Resize', scale=img_scale, keep_ratio=True),
+ dict(
+ type='mmdet.Pad',
+ pad_to_square=True,
+ pad_val=dict(img=(114.0, 114.0, 114.0))),
+ dict(
+ type='PackDetInputs',
+ meta_keys=('id', 'img_id', 'img_path', 'ori_shape', 'img_shape',
+ 'scale_factor', 'flip_indices'))
+]
+
+# dataset settings
+dataset_type = 'PoseCocoDataset'
+
+train_dataloader = dict(
+ dataset=dict(
+ type=dataset_type,
+ data_mode='bottomup',
+ ann_file='annotations/person_keypoints_train2017.json',
+ pipeline=train_pipeline_stage1))
+
+val_dataloader = dict(
+ dataset=dict(
+ type=dataset_type,
+ data_mode='bottomup',
+ ann_file='annotations/person_keypoints_val2017.json',
+ pipeline=test_pipeline))
+test_dataloader = val_dataloader
+
+# evaluators
+val_evaluator = dict(
+ _delete_=True,
+ type='mmpose.CocoMetric',
+ ann_file=_base_.data_root + 'annotations/person_keypoints_val2017.json',
+ score_mode='bbox')
+test_evaluator = val_evaluator
+
+default_hooks = dict(checkpoint=dict(save_best='coco/AP', rule='greater'))
+
+visualizer = dict(type='mmpose.PoseLocalVisualizer')
+
+custom_hooks = [
+ dict(
+ type='YOLOXModeSwitchHook',
+ num_last_epochs=num_last_epochs,
+ new_train_pipeline=train_pipeline_stage2,
+ priority=48),
+ dict(type='mmdet.SyncNormHook', priority=48),
+ dict(
+ type='EMAHook',
+ ema_type='ExpMomentumEMA',
+ momentum=0.0002,
+ update_buffers=True,
+ strict_load=False,
+ priority=49)
+]
diff --git a/configs/yolox/pose/yolox-pose_tiny_8xb32-300e-rtmdet-hyp_coco.py b/configs/yolox/pose/yolox-pose_tiny_8xb32-300e-rtmdet-hyp_coco.py
new file mode 100644
index 00000000..a7399065
--- /dev/null
+++ b/configs/yolox/pose/yolox-pose_tiny_8xb32-300e-rtmdet-hyp_coco.py
@@ -0,0 +1,70 @@
+_base_ = './yolox-pose_s_8xb32-300e-rtmdet-hyp_coco.py'
+
+load_from = 'https://download.openmmlab.com/mmyolo/v0/yolox/yolox_tiny_fast_8xb32-300e-rtmdet-hyp_coco/yolox_tiny_fast_8xb32-300e-rtmdet-hyp_coco_20230210_143637-4c338102.pth' # noqa
+
+deepen_factor = 0.33
+widen_factor = 0.375
+scaling_ratio_range = (0.75, 1.0)
+
+# model settings
+model = dict(
+ data_preprocessor=dict(batch_augments=[
+ dict(
+ type='YOLOXBatchSyncRandomResize',
+ random_size_range=(320, 640),
+ size_divisor=32,
+ interval=1)
+ ]),
+ backbone=dict(
+ deepen_factor=deepen_factor,
+ widen_factor=widen_factor,
+ ),
+ neck=dict(
+ deepen_factor=deepen_factor,
+ widen_factor=widen_factor,
+ ),
+ bbox_head=dict(head_module=dict(widen_factor=widen_factor)))
+
+# data settings
+img_scale = _base_.img_scale
+pre_transform = _base_.pre_transform
+
+train_pipeline_stage1 = [
+ *pre_transform,
+ dict(
+ type='Mosaic',
+ img_scale=img_scale,
+ pad_val=114.0,
+ pre_transform=pre_transform),
+ dict(
+ type='RandomAffine',
+ scaling_ratio_range=scaling_ratio_range,
+ border=(-img_scale[0] // 2, -img_scale[1] // 2)),
+ dict(type='mmdet.YOLOXHSVRandomAug'),
+ dict(type='RandomFlip', prob=0.5),
+ dict(
+ type='FilterAnnotations',
+ by_keypoints=True,
+ min_gt_bbox_wh=(1, 1),
+ keep_empty=False),
+ dict(
+ type='PackDetInputs',
+ meta_keys=('img_id', 'img_path', 'ori_shape', 'img_shape'))
+]
+
+test_pipeline = [
+ *pre_transform,
+ dict(type='Resize', scale=(416, 416), keep_ratio=True),
+ dict(
+ type='mmdet.Pad',
+ pad_to_square=True,
+ pad_val=dict(img=(114.0, 114.0, 114.0))),
+ dict(
+ type='PackDetInputs',
+ meta_keys=('id', 'img_id', 'img_path', 'ori_shape', 'img_shape',
+ 'scale_factor', 'flip_indices'))
+]
+
+train_dataloader = dict(dataset=dict(pipeline=train_pipeline_stage1))
+val_dataloader = dict(dataset=dict(pipeline=test_pipeline))
+test_dataloader = val_dataloader
diff --git a/mmyolo/datasets/__init__.py b/mmyolo/datasets/__init__.py
index b3b6b971..9db43904 100644
--- a/mmyolo/datasets/__init__.py
+++ b/mmyolo/datasets/__init__.py
@@ -1,4 +1,5 @@
# Copyright (c) OpenMMLab. All rights reserved.
+from .pose_coco import PoseCocoDataset
from .transforms import * # noqa: F401,F403
from .utils import BatchShapePolicy, yolov5_collate
from .yolov5_coco import YOLOv5CocoDataset
@@ -8,5 +9,6 @@ from .yolov5_voc import YOLOv5VOCDataset
__all__ = [
'YOLOv5CocoDataset', 'YOLOv5VOCDataset', 'BatchShapePolicy',
- 'yolov5_collate', 'YOLOv5CrowdHumanDataset', 'YOLOv5DOTADataset'
+ 'yolov5_collate', 'YOLOv5CrowdHumanDataset', 'YOLOv5DOTADataset',
+ 'PoseCocoDataset'
]
diff --git a/mmyolo/datasets/pose_coco.py b/mmyolo/datasets/pose_coco.py
new file mode 100644
index 00000000..85041f14
--- /dev/null
+++ b/mmyolo/datasets/pose_coco.py
@@ -0,0 +1,24 @@
+# Copyright (c) OpenMMLab. All rights reserved.
+from typing import Any
+
+from mmengine.dataset import force_full_init
+
+try:
+ from mmpose.datasets import CocoDataset as MMPoseCocoDataset
+except ImportError:
+ raise ImportError('Please run "mim install -r requirements/mmpose.txt" '
+ 'to install mmpose first for rotated detection.')
+
+from ..registry import DATASETS
+
+
+@DATASETS.register_module()
+class PoseCocoDataset(MMPoseCocoDataset):
+
+ METAINFO: dict = dict(from_file='configs/_base_/pose/coco.py')
+
+ @force_full_init
+ def prepare_data(self, idx) -> Any:
+ data_info = self.get_data_info(idx)
+ data_info['dataset'] = self
+ return self.pipeline(data_info)
diff --git a/mmyolo/datasets/transforms/__init__.py b/mmyolo/datasets/transforms/__init__.py
index 6719ac33..7cdcf862 100644
--- a/mmyolo/datasets/transforms/__init__.py
+++ b/mmyolo/datasets/transforms/__init__.py
@@ -1,16 +1,18 @@
# Copyright (c) OpenMMLab. All rights reserved.
from .formatting import PackDetInputs
from .mix_img_transforms import Mosaic, Mosaic9, YOLOv5MixUp, YOLOXMixUp
-from .transforms import (LetterResize, LoadAnnotations, Polygon2Mask,
- PPYOLOERandomCrop, PPYOLOERandomDistort,
- RegularizeRotatedBox, RemoveDataElement,
- YOLOv5CopyPaste, YOLOv5HSVRandomAug,
- YOLOv5KeepRatioResize, YOLOv5RandomAffine)
+from .transforms import (FilterAnnotations, LetterResize, LoadAnnotations,
+ Polygon2Mask, PPYOLOERandomCrop, PPYOLOERandomDistort,
+ RandomAffine, RandomFlip, RegularizeRotatedBox,
+ RemoveDataElement, Resize, YOLOv5CopyPaste,
+ YOLOv5HSVRandomAug, YOLOv5KeepRatioResize,
+ YOLOv5RandomAffine)
__all__ = [
'YOLOv5KeepRatioResize', 'LetterResize', 'Mosaic', 'YOLOXMixUp',
'YOLOv5MixUp', 'YOLOv5HSVRandomAug', 'LoadAnnotations',
'YOLOv5RandomAffine', 'PPYOLOERandomDistort', 'PPYOLOERandomCrop',
'Mosaic9', 'YOLOv5CopyPaste', 'RemoveDataElement', 'RegularizeRotatedBox',
- 'Polygon2Mask', 'PackDetInputs'
+ 'Polygon2Mask', 'PackDetInputs', 'RandomAffine', 'RandomFlip', 'Resize',
+ 'FilterAnnotations'
]
diff --git a/mmyolo/datasets/transforms/formatting.py b/mmyolo/datasets/transforms/formatting.py
index 0185d78c..07eb0121 100644
--- a/mmyolo/datasets/transforms/formatting.py
+++ b/mmyolo/datasets/transforms/formatting.py
@@ -16,6 +16,13 @@ class PackDetInputs(MMDET_PackDetInputs):
Compared to mmdet, we just add the `gt_panoptic_seg` field and logic.
"""
+ mapping_table = {
+ 'gt_bboxes': 'bboxes',
+ 'gt_bboxes_labels': 'labels',
+ 'gt_masks': 'masks',
+ 'gt_keypoints': 'keypoints',
+ 'gt_keypoints_visible': 'keypoints_visible'
+ }
def transform(self, results: dict) -> dict:
"""Method to pack the input data.
@@ -50,6 +57,10 @@ class PackDetInputs(MMDET_PackDetInputs):
if 'gt_ignore_flags' in results:
valid_idx = np.where(results['gt_ignore_flags'] == 0)[0]
ignore_idx = np.where(results['gt_ignore_flags'] == 1)[0]
+ if 'gt_keypoints' in results:
+ results['gt_keypoints_visible'] = results[
+ 'gt_keypoints'].keypoints_visible
+ results['gt_keypoints'] = results['gt_keypoints'].keypoints
data_sample = DetDataSample()
instance_data = InstanceData()
diff --git a/mmyolo/datasets/transforms/keypoint_structure.py b/mmyolo/datasets/transforms/keypoint_structure.py
new file mode 100644
index 00000000..7b8402be
--- /dev/null
+++ b/mmyolo/datasets/transforms/keypoint_structure.py
@@ -0,0 +1,248 @@
+# Copyright (c) OpenMMLab. All rights reserved.
+from abc import ABCMeta
+from copy import deepcopy
+from typing import List, Optional, Sequence, Tuple, Type, TypeVar, Union
+
+import numpy as np
+import torch
+from torch import Tensor
+
+DeviceType = Union[str, torch.device]
+T = TypeVar('T')
+IndexType = Union[slice, int, list, torch.LongTensor, torch.cuda.LongTensor,
+ torch.BoolTensor, torch.cuda.BoolTensor, np.ndarray]
+
+
+class Keypoints(metaclass=ABCMeta):
+ """The Keypoints class is for keypoints representation.
+
+ Args:
+ keypoints (Tensor or np.ndarray): The keypoint data with shape of
+ (N, K, 2).
+ keypoints_visible (Tensor or np.ndarray): The visibility of keypoints
+ with shape of (N, K).
+ device (str or torch.device, Optional): device of keypoints.
+ Default to None.
+ clone (bool): Whether clone ``keypoints`` or not. Defaults to True.
+ flip_indices (list, Optional): The indices of keypoints when the
+ images is flipped. Defaults to None.
+
+ Notes:
+ N: the number of instances.
+ K: the number of keypoints.
+ """
+
+ def __init__(self,
+ keypoints: Union[Tensor, np.ndarray],
+ keypoints_visible: Union[Tensor, np.ndarray],
+ device: Optional[DeviceType] = None,
+ clone: bool = True,
+ flip_indices: Optional[List] = None) -> None:
+
+ assert len(keypoints_visible) == len(keypoints)
+ assert keypoints.ndim == 3
+ assert keypoints_visible.ndim == 2
+
+ keypoints = torch.as_tensor(keypoints)
+ keypoints_visible = torch.as_tensor(keypoints_visible)
+
+ if device is not None:
+ keypoints = keypoints.to(device=device)
+ keypoints_visible = keypoints_visible.to(device=device)
+
+ if clone:
+ keypoints = keypoints.clone()
+ keypoints_visible = keypoints_visible.clone()
+
+ self.keypoints = keypoints
+ self.keypoints_visible = keypoints_visible
+ self.flip_indices = flip_indices
+
+ def flip_(self,
+ img_shape: Tuple[int, int],
+ direction: str = 'horizontal') -> None:
+ """Flip boxes & kpts horizontally in-place.
+
+ Args:
+ img_shape (Tuple[int, int]): A tuple of image height and width.
+ direction (str): Flip direction, options are "horizontal",
+ "vertical" and "diagonal". Defaults to "horizontal"
+ """
+ assert direction == 'horizontal'
+ self.keypoints[..., 0] = img_shape[1] - self.keypoints[..., 0]
+ self.keypoints = self.keypoints[:, self.flip_indices]
+ self.keypoints_visible = self.keypoints_visible[:, self.flip_indices]
+
+ def translate_(self, distances: Tuple[float, float]) -> None:
+ """Translate boxes and keypoints in-place.
+
+ Args:
+ distances (Tuple[float, float]): translate distances. The first
+ is horizontal distance and the second is vertical distance.
+ """
+ assert len(distances) == 2
+ distances = self.keypoints.new_tensor(distances).reshape(1, 1, 2)
+ self.keypoints = self.keypoints + distances
+
+ def rescale_(self, scale_factor: Tuple[float, float]) -> None:
+ """Rescale boxes & keypoints w.r.t. rescale_factor in-place.
+
+ Note:
+ Both ``rescale_`` and ``resize_`` will enlarge or shrink boxes
+ w.r.t ``scale_facotr``. The difference is that ``resize_`` only
+ changes the width and the height of boxes, but ``rescale_`` also
+ rescales the box centers simultaneously.
+
+ Args:
+ scale_factor (Tuple[float, float]): factors for scaling boxes.
+ The length should be 2.
+ """
+ assert len(scale_factor) == 2
+
+ scale_factor = self.keypoints.new_tensor(scale_factor).reshape(1, 1, 2)
+ self.keypoints = self.keypoints * scale_factor
+
+ def clip_(self, img_shape: Tuple[int, int]) -> None:
+ """Clip bounding boxes and set invisible keypoints outside the image
+ boundary in-place.
+
+ Args:
+ img_shape (Tuple[int, int]): A tuple of image height and width.
+ """
+
+ kpt_outside = torch.logical_or(
+ torch.logical_or(self.keypoints[..., 0] < 0,
+ self.keypoints[..., 1] < 0),
+ torch.logical_or(self.keypoints[..., 0] > img_shape[1],
+ self.keypoints[..., 1] > img_shape[0]))
+ self.keypoints_visible[kpt_outside] *= 0
+
+ def project_(self, homography_matrix: Union[Tensor, np.ndarray]) -> None:
+ """Geometrically transform bounding boxes and keypoints in-place using
+ a homography matrix.
+
+ Args:
+ homography_matrix (Tensor or np.ndarray): A 3x3 tensor or ndarray
+ representing the homography matrix for the transformation.
+ """
+ keypoints = self.keypoints
+ if isinstance(homography_matrix, np.ndarray):
+ homography_matrix = keypoints.new_tensor(homography_matrix)
+
+ # Convert keypoints to homogeneous coordinates
+ keypoints = torch.cat([
+ self.keypoints,
+ self.keypoints.new_ones(*self.keypoints.shape[:-1], 1)
+ ],
+ dim=-1)
+
+ # Transpose keypoints for matrix multiplication
+ keypoints_T = torch.transpose(keypoints, -1, 0).contiguous().flatten(1)
+
+ # Apply homography matrix to corners and keypoints
+ keypoints_T = torch.matmul(homography_matrix, keypoints_T)
+
+ # Transpose back to original shape
+ keypoints_T = keypoints_T.reshape(3, self.keypoints.shape[1], -1)
+ keypoints = torch.transpose(keypoints_T, -1, 0).contiguous()
+
+ # Convert corners and keypoints back to non-homogeneous coordinates
+ keypoints = keypoints[..., :2] / keypoints[..., 2:3]
+
+ # Convert corners back to bounding boxes and update object attributes
+ self.keypoints = keypoints
+
+ @classmethod
+ def cat(cls: Type[T], kps_list: Sequence[T], dim: int = 0) -> T:
+ """Cancatenates an instance list into one single instance. Similar to
+ ``torch.cat``.
+
+ Args:
+ box_list (Sequence[T]): A sequence of instances.
+ dim (int): The dimension over which the box and keypoint are
+ concatenated. Defaults to 0.
+
+ Returns:
+ T: Concatenated instance.
+ """
+ assert isinstance(kps_list, Sequence)
+ if len(kps_list) == 0:
+ raise ValueError('kps_list should not be a empty list.')
+
+ assert dim == 0
+ assert all(isinstance(keypoints, cls) for keypoints in kps_list)
+
+ th_kpt_list = torch.cat(
+ [keypoints.keypoints for keypoints in kps_list], dim=dim)
+ th_kpt_vis_list = torch.cat(
+ [keypoints.keypoints_visible for keypoints in kps_list], dim=dim)
+ flip_indices = kps_list[0].flip_indices
+ return cls(
+ th_kpt_list,
+ th_kpt_vis_list,
+ clone=False,
+ flip_indices=flip_indices)
+
+ def __getitem__(self: T, index: IndexType) -> T:
+ """Rewrite getitem to protect the last dimension shape."""
+ if isinstance(index, np.ndarray):
+ index = torch.as_tensor(index, device=self.device)
+ if isinstance(index, Tensor) and index.dtype == torch.bool:
+ assert index.dim() < self.keypoints.dim() - 1
+ elif isinstance(index, tuple):
+ assert len(index) < self.keypoints.dim() - 1
+ # `Ellipsis`(...) is commonly used in index like [None, ...].
+ # When `Ellipsis` is in index, it must be the last item.
+ if Ellipsis in index:
+ assert index[-1] is Ellipsis
+
+ keypoints = self.keypoints[index]
+ keypoints_visible = self.keypoints_visible[index]
+ if self.keypoints.dim() == 2:
+ keypoints = keypoints.reshape(1, -1, 2)
+ keypoints_visible = keypoints_visible.reshape(1, -1)
+ return type(self)(
+ keypoints,
+ keypoints_visible,
+ flip_indices=self.flip_indices,
+ clone=False)
+
+ def __repr__(self) -> str:
+ """Return a strings that describes the object."""
+ return self.__class__.__name__ + '(\n' + str(self.keypoints) + ')'
+
+ @property
+ def num_keypoints(self) -> Tensor:
+ """Compute the number of visible keypoints for each object."""
+ return self.keypoints_visible.sum(dim=1).int()
+
+ def __deepcopy__(self, memo):
+ """Only clone the tensors when applying deepcopy."""
+ cls = self.__class__
+ other = cls.__new__(cls)
+ memo[id(self)] = other
+ other.keypoints = self.keypoints.clone()
+ other.keypoints_visible = self.keypoints_visible.clone()
+ other.flip_indices = deepcopy(self.flip_indices)
+ return other
+
+ def clone(self: T) -> T:
+ """Reload ``clone`` for tensors."""
+ return type(self)(
+ self.keypoints,
+ self.keypoints_visible,
+ flip_indices=self.flip_indices,
+ clone=True)
+
+ def to(self: T, *args, **kwargs) -> T:
+ """Reload ``to`` for tensors."""
+ return type(self)(
+ self.keypoints.to(*args, **kwargs),
+ self.keypoints_visible.to(*args, **kwargs),
+ flip_indices=self.flip_indices,
+ clone=False)
+
+ @property
+ def device(self) -> torch.device:
+ """Reload ``device`` from self.tensor."""
+ return self.keypoints.device
diff --git a/mmyolo/datasets/transforms/mix_img_transforms.py b/mmyolo/datasets/transforms/mix_img_transforms.py
index 4753ecc3..29e4a405 100644
--- a/mmyolo/datasets/transforms/mix_img_transforms.py
+++ b/mmyolo/datasets/transforms/mix_img_transforms.py
@@ -318,7 +318,9 @@ class Mosaic(BaseMixImageTransform):
mosaic_bboxes_labels = []
mosaic_ignore_flags = []
mosaic_masks = []
+ mosaic_kps = []
with_mask = True if 'gt_masks' in results else False
+ with_kps = True if 'gt_keypoints' in results else False
# self.img_scale is wh format
img_scale_w, img_scale_h = self.img_scale
@@ -386,6 +388,12 @@ class Mosaic(BaseMixImageTransform):
offset=padh,
direction='vertical')
mosaic_masks.append(gt_masks_i)
+ if with_kps and results_patch.get('gt_keypoints',
+ None) is not None:
+ gt_kps_i = results_patch['gt_keypoints']
+ gt_kps_i.rescale_([scale_ratio_i, scale_ratio_i])
+ gt_kps_i.translate_([padw, padh])
+ mosaic_kps.append(gt_kps_i)
mosaic_bboxes = mosaic_bboxes[0].cat(mosaic_bboxes, 0)
mosaic_bboxes_labels = np.concatenate(mosaic_bboxes_labels, 0)
@@ -396,6 +404,10 @@ class Mosaic(BaseMixImageTransform):
if with_mask:
mosaic_masks = mosaic_masks[0].cat(mosaic_masks)
results['gt_masks'] = mosaic_masks
+ if with_kps:
+ mosaic_kps = mosaic_kps[0].cat(mosaic_kps, 0)
+ mosaic_kps.clip_([2 * img_scale_h, 2 * img_scale_w])
+ results['gt_keypoints'] = mosaic_kps
else:
# remove outside bboxes
inside_inds = mosaic_bboxes.is_inside(
@@ -406,6 +418,10 @@ class Mosaic(BaseMixImageTransform):
if with_mask:
mosaic_masks = mosaic_masks[0].cat(mosaic_masks)[inside_inds]
results['gt_masks'] = mosaic_masks
+ if with_kps:
+ mosaic_kps = mosaic_kps[0].cat(mosaic_kps, 0)
+ mosaic_kps = mosaic_kps[inside_inds]
+ results['gt_keypoints'] = mosaic_kps
results['img'] = mosaic_img
results['img_shape'] = mosaic_img.shape
@@ -1131,6 +1147,31 @@ class YOLOXMixUp(BaseMixImageTransform):
mixup_gt_bboxes_labels = mixup_gt_bboxes_labels[inside_inds]
mixup_gt_ignore_flags = mixup_gt_ignore_flags[inside_inds]
+ if 'gt_keypoints' in results:
+ # adjust kps
+ retrieve_gt_keypoints = retrieve_results['gt_keypoints']
+ retrieve_gt_keypoints.rescale_([scale_ratio, scale_ratio])
+ if self.bbox_clip_border:
+ retrieve_gt_keypoints.clip_([origin_h, origin_w])
+
+ if is_filp:
+ retrieve_gt_keypoints.flip_([origin_h, origin_w],
+ direction='horizontal')
+
+ # filter
+ cp_retrieve_gt_keypoints = retrieve_gt_keypoints.clone()
+ cp_retrieve_gt_keypoints.translate_([-x_offset, -y_offset])
+ if self.bbox_clip_border:
+ cp_retrieve_gt_keypoints.clip_([target_h, target_w])
+
+ # mixup
+ mixup_gt_keypoints = cp_retrieve_gt_keypoints.cat(
+ (results['gt_keypoints'], cp_retrieve_gt_keypoints), dim=0)
+ if not self.bbox_clip_border:
+ # remove outside bbox
+ mixup_gt_keypoints = mixup_gt_keypoints[inside_inds]
+ results['gt_keypoints'] = mixup_gt_keypoints
+
results['img'] = mixup_img.astype(np.uint8)
results['img_shape'] = mixup_img.shape
results['gt_bboxes'] = mixup_gt_bboxes
diff --git a/mmyolo/datasets/transforms/transforms.py b/mmyolo/datasets/transforms/transforms.py
index 30dfdb3f..12d15c96 100644
--- a/mmyolo/datasets/transforms/transforms.py
+++ b/mmyolo/datasets/transforms/transforms.py
@@ -7,9 +7,13 @@ import cv2
import mmcv
import numpy as np
import torch
+from mmcv.image.geometric import _scale_size
from mmcv.transforms import BaseTransform, Compose
from mmcv.transforms.utils import cache_randomness
+from mmdet.datasets.transforms import FilterAnnotations as FilterDetAnnotations
from mmdet.datasets.transforms import LoadAnnotations as MMDET_LoadAnnotations
+from mmdet.datasets.transforms import RandomAffine as MMDET_RandomAffine
+from mmdet.datasets.transforms import RandomFlip as MMDET_RandomFlip
from mmdet.datasets.transforms import Resize as MMDET_Resize
from mmdet.structures.bbox import (HorizontalBoxes, autocast_box_type,
get_box_type)
@@ -17,6 +21,7 @@ from mmdet.structures.mask import PolygonMasks, polygon_to_bitmap
from numpy import random
from mmyolo.registry import TRANSFORMS
+from .keypoint_structure import Keypoints
# TODO: Waiting for MMCV support
TRANSFORMS.register_module(module=Compose, force=True)
@@ -435,6 +440,11 @@ class LoadAnnotations(MMDET_LoadAnnotations):
self._update_mask_ignore_data(results)
gt_bboxes = results['gt_masks'].get_bboxes(dst_type='hbox')
results['gt_bboxes'] = gt_bboxes
+ elif self.with_keypoints:
+ self._load_kps(results)
+ _, box_type_cls = get_box_type(self.box_type)
+ results['gt_bboxes'] = box_type_cls(
+ results.get('bbox', []), dtype=torch.float32)
else:
results = super().transform(results)
self._update_mask_ignore_data(results)
@@ -611,6 +621,36 @@ class LoadAnnotations(MMDET_LoadAnnotations):
dis = ((arr1[:, None, :] - arr2[None, :, :])**2).sum(-1)
return np.unravel_index(np.argmin(dis, axis=None), dis.shape)
+ def _load_kps(self, results: dict) -> None:
+ """Private function to load keypoints annotations.
+
+ Args:
+ results (dict): Result dict from
+ :class:`mmengine.dataset.BaseDataset`.
+
+ Returns:
+ dict: The dict contains loaded keypoints annotations.
+ """
+ results['height'] = results['img_shape'][0]
+ results['width'] = results['img_shape'][1]
+ num_instances = len(results.get('bbox', []))
+
+ if num_instances == 0:
+ results['keypoints'] = np.empty(
+ (0, len(results['flip_indices']), 2), dtype=np.float32)
+ results['keypoints_visible'] = np.empty(
+ (0, len(results['flip_indices'])), dtype=np.int32)
+ results['category_id'] = []
+
+ results['gt_keypoints'] = Keypoints(
+ keypoints=results['keypoints'],
+ keypoints_visible=results['keypoints_visible'],
+ flip_indices=results['flip_indices'],
+ )
+
+ results['gt_ignore_flags'] = np.array([False] * num_instances)
+ results['gt_bboxes_labels'] = np.array(results['category_id']) - 1
+
def __repr__(self) -> str:
repr_str = self.__class__.__name__
repr_str += f'(with_bbox={self.with_bbox}, '
@@ -1872,3 +1912,192 @@ class Polygon2Mask(BaseTransform):
# Consistent logic with mmdet
results['gt_masks'] = masks
return results
+
+
+@TRANSFORMS.register_module()
+class FilterAnnotations(FilterDetAnnotations):
+ """Filter invalid annotations.
+
+ In addition to the conditions checked by ``FilterDetAnnotations``, this
+ filter adds a new condition requiring instances to have at least one
+ visible keypoints.
+ """
+
+ def __init__(self, by_keypoints: bool = False, **kwargs) -> None:
+ # TODO: add more filter options
+ super().__init__(**kwargs)
+ self.by_keypoints = by_keypoints
+
+ @autocast_box_type()
+ def transform(self, results: dict) -> Union[dict, None]:
+ """Transform function to filter annotations.
+
+ Args:
+ results (dict): Result dict.
+ Returns:
+ dict: Updated result dict.
+ """
+ assert 'gt_bboxes' in results
+ gt_bboxes = results['gt_bboxes']
+ if gt_bboxes.shape[0] == 0:
+ return results
+
+ tests = []
+ if self.by_box:
+ tests.append(
+ ((gt_bboxes.widths > self.min_gt_bbox_wh[0]) &
+ (gt_bboxes.heights > self.min_gt_bbox_wh[1])).numpy())
+
+ if self.by_mask:
+ assert 'gt_masks' in results
+ gt_masks = results['gt_masks']
+ tests.append(gt_masks.areas >= self.min_gt_mask_area)
+
+ if self.by_keypoints:
+ assert 'gt_keypoints' in results
+ num_keypoints = results['gt_keypoints'].num_keypoints
+ tests.append((num_keypoints > 0).numpy())
+
+ keep = tests[0]
+ for t in tests[1:]:
+ keep = keep & t
+
+ if not keep.any():
+ if self.keep_empty:
+ return None
+
+ keys = ('gt_bboxes', 'gt_bboxes_labels', 'gt_masks', 'gt_ignore_flags',
+ 'gt_keypoints')
+ for key in keys:
+ if key in results:
+ results[key] = results[key][keep]
+
+ return results
+
+
+# TODO: Check if it can be merged with mmdet.YOLOXHSVRandomAug
+@TRANSFORMS.register_module()
+class RandomAffine(MMDET_RandomAffine):
+
+ def __init__(self, **kwargs) -> None:
+ super().__init__(**kwargs)
+
+ @autocast_box_type()
+ def transform(self, results: dict) -> dict:
+ img = results['img']
+ height = img.shape[0] + self.border[1] * 2
+ width = img.shape[1] + self.border[0] * 2
+
+ warp_matrix = self._get_random_homography_matrix(height, width)
+
+ img = cv2.warpPerspective(
+ img,
+ warp_matrix,
+ dsize=(width, height),
+ borderValue=self.border_val)
+ results['img'] = img
+ results['img_shape'] = img.shape
+
+ bboxes = results['gt_bboxes']
+ num_bboxes = len(bboxes)
+ if num_bboxes:
+ bboxes.project_(warp_matrix)
+ if self.bbox_clip_border:
+ bboxes.clip_([height, width])
+ # remove outside bbox
+ valid_index = bboxes.is_inside([height, width]).numpy()
+ results['gt_bboxes'] = bboxes[valid_index]
+ results['gt_bboxes_labels'] = results['gt_bboxes_labels'][
+ valid_index]
+ results['gt_ignore_flags'] = results['gt_ignore_flags'][
+ valid_index]
+
+ if 'gt_masks' in results:
+ raise NotImplementedError('RandomAffine only supports bbox.')
+
+ if 'gt_keypoints' in results:
+ keypoints = results['gt_keypoints']
+ keypoints.project_(warp_matrix)
+ if self.bbox_clip_border:
+ keypoints.clip_([height, width])
+ results['gt_keypoints'] = keypoints[valid_index]
+
+ return results
+
+ def __repr__(self) -> str:
+ repr_str = self.__class__.__name__
+ repr_str += f'(hue_delta={self.hue_delta}, '
+ repr_str += f'saturation_delta={self.saturation_delta}, '
+ repr_str += f'value_delta={self.value_delta})'
+ return repr_str
+
+
+# TODO: Check if it can be merged with mmdet.YOLOXHSVRandomAug
+@TRANSFORMS.register_module()
+class RandomFlip(MMDET_RandomFlip):
+
+ @autocast_box_type()
+ def _flip(self, results: dict) -> None:
+ """Flip images, bounding boxes, and semantic segmentation map."""
+ # flip image
+ results['img'] = mmcv.imflip(
+ results['img'], direction=results['flip_direction'])
+
+ img_shape = results['img'].shape[:2]
+
+ # flip bboxes
+ if results.get('gt_bboxes', None) is not None:
+ results['gt_bboxes'].flip_(img_shape, results['flip_direction'])
+
+ # flip keypoints
+ if results.get('gt_keypoints', None) is not None:
+ results['gt_keypoints'].flip_(img_shape, results['flip_direction'])
+
+ # flip masks
+ if results.get('gt_masks', None) is not None:
+ results['gt_masks'] = results['gt_masks'].flip(
+ results['flip_direction'])
+
+ # flip segs
+ if results.get('gt_seg_map', None) is not None:
+ results['gt_seg_map'] = mmcv.imflip(
+ results['gt_seg_map'], direction=results['flip_direction'])
+
+ # record homography matrix for flip
+ self._record_homography_matrix(results)
+
+
+@TRANSFORMS.register_module()
+class Resize(MMDET_Resize):
+
+ def _resize_keypoints(self, results: dict) -> None:
+ """Resize bounding boxes with ``results['scale_factor']``."""
+ if results.get('gt_keypoints', None) is not None:
+ results['gt_keypoints'].rescale_(results['scale_factor'])
+ if self.clip_object_border:
+ results['gt_keypoints'].clip_(results['img_shape'])
+
+ @autocast_box_type()
+ def transform(self, results: dict) -> dict:
+ """Transform function to resize images, bounding boxes and semantic
+ segmentation map.
+
+ Args:
+ results (dict): Result dict from loading pipeline.
+ Returns:
+ dict: Resized results, 'img', 'gt_bboxes', 'gt_seg_map',
+ 'scale', 'scale_factor', 'height', 'width', and 'keep_ratio' keys
+ are updated in result dict.
+ """
+ if self.scale:
+ results['scale'] = self.scale
+ else:
+ img_shape = results['img'].shape[:2]
+ results['scale'] = _scale_size(img_shape[::-1], self.scale_factor)
+ self._resize_img(results)
+ self._resize_bboxes(results)
+ self._resize_keypoints(results)
+ self._resize_masks(results)
+ self._resize_seg(results)
+ self._record_homography_matrix(results)
+ return results
diff --git a/mmyolo/datasets/utils.py b/mmyolo/datasets/utils.py
index d50207c8..efa2ff5e 100644
--- a/mmyolo/datasets/utils.py
+++ b/mmyolo/datasets/utils.py
@@ -21,6 +21,8 @@ def yolov5_collate(data_batch: Sequence,
batch_imgs = []
batch_bboxes_labels = []
batch_masks = []
+ batch_keyponits = []
+ batch_keypoints_visible = []
for i in range(len(data_batch)):
datasamples = data_batch[i]['data_samples']
inputs = data_batch[i]['inputs']
@@ -33,11 +35,16 @@ def yolov5_collate(data_batch: Sequence,
batch_masks.append(masks)
if 'gt_panoptic_seg' in datasamples:
batch_masks.append(datasamples.gt_panoptic_seg.pan_seg)
+ if 'keypoints' in datasamples.gt_instances:
+ keypoints = datasamples.gt_instances.keypoints
+ keypoints_visible = datasamples.gt_instances.keypoints_visible
+ batch_keyponits.append(keypoints)
+ batch_keypoints_visible.append(keypoints_visible)
+
batch_idx = gt_labels.new_full((len(gt_labels), 1), i)
bboxes_labels = torch.cat((batch_idx, gt_labels[:, None], gt_bboxes),
dim=1)
batch_bboxes_labels.append(bboxes_labels)
-
collated_results = {
'data_samples': {
'bboxes_labels': torch.cat(batch_bboxes_labels, 0)
@@ -46,6 +53,12 @@ def yolov5_collate(data_batch: Sequence,
if len(batch_masks) > 0:
collated_results['data_samples']['masks'] = torch.cat(batch_masks, 0)
+ if len(batch_keyponits) > 0:
+ collated_results['data_samples']['keypoints'] = torch.cat(
+ batch_keyponits, 0)
+ collated_results['data_samples']['keypoints_visible'] = torch.cat(
+ batch_keypoints_visible, 0)
+
if use_ms_training:
collated_results['inputs'] = batch_imgs
else:
diff --git a/mmyolo/models/data_preprocessors/data_preprocessor.py b/mmyolo/models/data_preprocessors/data_preprocessor.py
index f09fd8e7..a29b9084 100644
--- a/mmyolo/models/data_preprocessors/data_preprocessor.py
+++ b/mmyolo/models/data_preprocessors/data_preprocessor.py
@@ -49,6 +49,10 @@ class YOLOXBatchSyncRandomResize(BatchSyncRandomResize):
data_samples['bboxes_labels'][:, 2::2] *= scale_x
data_samples['bboxes_labels'][:, 3::2] *= scale_y
+ if 'keypoints' in data_samples:
+ data_samples['keypoints'][..., 0] *= scale_x
+ data_samples['keypoints'][..., 1] *= scale_y
+
message_hub = MessageHub.get_current_instance()
if (message_hub.get_info('iter') + 1) % self._interval == 0:
self._input_size = self._get_random_size(
@@ -102,6 +106,10 @@ class YOLOv5DetDataPreprocessor(DetDataPreprocessor):
}
if 'masks' in data_samples:
data_samples_output['masks'] = data_samples['masks']
+ if 'keypoints' in data_samples:
+ data_samples_output['keypoints'] = data_samples['keypoints']
+ data_samples_output['keypoints_visible'] = data_samples[
+ 'keypoints_visible']
return {'inputs': inputs, 'data_samples': data_samples_output}
diff --git a/mmyolo/models/dense_heads/__init__.py b/mmyolo/models/dense_heads/__init__.py
index ac65c42e..90587c3f 100644
--- a/mmyolo/models/dense_heads/__init__.py
+++ b/mmyolo/models/dense_heads/__init__.py
@@ -10,6 +10,7 @@ from .yolov6_head import YOLOv6Head, YOLOv6HeadModule
from .yolov7_head import YOLOv7Head, YOLOv7HeadModule, YOLOv7p6HeadModule
from .yolov8_head import YOLOv8Head, YOLOv8HeadModule
from .yolox_head import YOLOXHead, YOLOXHeadModule
+from .yolox_pose_head import YOLOXPoseHead, YOLOXPoseHeadModule
__all__ = [
'YOLOv5Head', 'YOLOv6Head', 'YOLOXHead', 'YOLOv5HeadModule',
@@ -17,5 +18,6 @@ __all__ = [
'RTMDetSepBNHeadModule', 'YOLOv7Head', 'PPYOLOEHead', 'PPYOLOEHeadModule',
'YOLOv7HeadModule', 'YOLOv7p6HeadModule', 'YOLOv8Head', 'YOLOv8HeadModule',
'RTMDetRotatedHead', 'RTMDetRotatedSepBNHeadModule', 'RTMDetInsSepBNHead',
- 'RTMDetInsSepBNHeadModule', 'YOLOv5InsHead', 'YOLOv5InsHeadModule'
+ 'RTMDetInsSepBNHeadModule', 'YOLOv5InsHead', 'YOLOv5InsHeadModule',
+ 'YOLOXPoseHead', 'YOLOXPoseHeadModule'
]
diff --git a/mmyolo/models/dense_heads/yolox_pose_head.py b/mmyolo/models/dense_heads/yolox_pose_head.py
new file mode 100644
index 00000000..96264e55
--- /dev/null
+++ b/mmyolo/models/dense_heads/yolox_pose_head.py
@@ -0,0 +1,409 @@
+# Copyright (c) OpenMMLab. All rights reserved.
+from collections import defaultdict
+from typing import List, Optional, Sequence, Tuple, Union
+
+import torch
+import torch.nn as nn
+from mmcv.ops import batched_nms
+from mmdet.models.utils import filter_scores_and_topk
+from mmdet.utils import ConfigType, OptInstanceList
+from mmengine.config import ConfigDict
+from mmengine.model import ModuleList, bias_init_with_prob
+from mmengine.structures import InstanceData
+from torch import Tensor
+
+from mmyolo.registry import MODELS
+from ..utils import OutputSaveFunctionWrapper, OutputSaveObjectWrapper
+from .yolox_head import YOLOXHead, YOLOXHeadModule
+
+
+@MODELS.register_module()
+class YOLOXPoseHeadModule(YOLOXHeadModule):
+ """YOLOXPoseHeadModule serves as a head module for `YOLOX-Pose`.
+
+ In comparison to `YOLOXHeadModule`, this module introduces branches for
+ keypoint prediction.
+ """
+
+ def __init__(self, num_keypoints: int, *args, **kwargs):
+ self.num_keypoints = num_keypoints
+ super().__init__(*args, **kwargs)
+
+ def _init_layers(self):
+ """Initializes the layers in the head module."""
+ super()._init_layers()
+
+ # The pose branch requires additional layers for precise regression
+ self.stacked_convs *= 2
+
+ # Create separate layers for each level of feature maps
+ pose_convs, offsets_preds, vis_preds = [], [], []
+ for _ in self.featmap_strides:
+ pose_convs.append(self._build_stacked_convs())
+ offsets_preds.append(
+ nn.Conv2d(self.feat_channels, self.num_keypoints * 2, 1))
+ vis_preds.append(
+ nn.Conv2d(self.feat_channels, self.num_keypoints, 1))
+
+ self.multi_level_pose_convs = ModuleList(pose_convs)
+ self.multi_level_conv_offsets = ModuleList(offsets_preds)
+ self.multi_level_conv_vis = ModuleList(vis_preds)
+
+ def init_weights(self):
+ """Initialize weights of the head."""
+ super().init_weights()
+
+ # Use prior in model initialization to improve stability
+ bias_init = bias_init_with_prob(0.01)
+ for conv_vis in self.multi_level_conv_vis:
+ conv_vis.bias.data.fill_(bias_init)
+
+ def forward(self, x: Tuple[Tensor]) -> Tuple[List]:
+ """Forward features from the upstream network."""
+ offsets_pred, vis_pred = [], []
+ for i in range(len(x)):
+ pose_feat = self.multi_level_pose_convs[i](x[i])
+ offsets_pred.append(self.multi_level_conv_offsets[i](pose_feat))
+ vis_pred.append(self.multi_level_conv_vis[i](pose_feat))
+ return (*super().forward(x), offsets_pred, vis_pred)
+
+
+@MODELS.register_module()
+class YOLOXPoseHead(YOLOXHead):
+ """YOLOXPoseHead head used in `YOLO-Pose.
+
+ `_.
+ Args:
+ loss_pose (ConfigDict, optional): Config of keypoint OKS loss.
+ """
+
+ def __init__(
+ self,
+ loss_pose: Optional[ConfigType] = None,
+ *args,
+ **kwargs,
+ ):
+ super().__init__(*args, **kwargs)
+ self.loss_pose = MODELS.build(loss_pose)
+ self.num_keypoints = self.head_module.num_keypoints
+
+ # set up buffers to save variables generated in methods of
+ # the class's base class.
+ self._log = defaultdict(list)
+ self.sampler = OutputSaveObjectWrapper(self.sampler)
+
+ # ensure that the `sigmas` in self.assigner.oks_calculator
+ # is on the same device as the model
+ if hasattr(self.assigner, 'oks_calculator'):
+ self.add_module('assigner_oks_calculator',
+ self.assigner.oks_calculator)
+
+ def _clear(self):
+ """Clear variable buffers."""
+ self.sampler.clear()
+ self._log.clear()
+
+ def loss(self, x: Tuple[Tensor], batch_data_samples: Union[list,
+ dict]) -> dict:
+
+ if isinstance(batch_data_samples, list):
+ losses = super().loss(x, batch_data_samples)
+ else:
+ outs = self(x)
+ # Fast version
+ loss_inputs = outs + (batch_data_samples['bboxes_labels'],
+ batch_data_samples['keypoints'],
+ batch_data_samples['keypoints_visible'],
+ batch_data_samples['img_metas'])
+ losses = self.loss_by_feat(*loss_inputs)
+
+ return losses
+
+ def loss_by_feat(
+ self,
+ cls_scores: Sequence[Tensor],
+ bbox_preds: Sequence[Tensor],
+ objectnesses: Sequence[Tensor],
+ kpt_preds: Sequence[Tensor],
+ vis_preds: Sequence[Tensor],
+ batch_gt_instances: Tensor,
+ batch_gt_keypoints: Tensor,
+ batch_gt_keypoints_visible: Tensor,
+ batch_img_metas: Sequence[dict],
+ batch_gt_instances_ignore: OptInstanceList = None) -> dict:
+ """Calculate the loss based on the features extracted by the detection
+ head.
+
+ In addition to the base class method, keypoint losses are also
+ calculated in this method.
+ """
+
+ self._clear()
+ batch_gt_instances = self.gt_kps_instances_preprocess(
+ batch_gt_instances, batch_gt_keypoints, batch_gt_keypoints_visible,
+ len(batch_img_metas))
+
+ # collect keypoints coordinates and visibility from model predictions
+ kpt_preds = torch.cat([
+ kpt_pred.flatten(2).permute(0, 2, 1).contiguous()
+ for kpt_pred in kpt_preds
+ ],
+ dim=1)
+
+ featmap_sizes = [cls_score.shape[2:] for cls_score in cls_scores]
+ mlvl_priors = self.prior_generator.grid_priors(
+ featmap_sizes,
+ dtype=cls_scores[0].dtype,
+ device=cls_scores[0].device,
+ with_stride=True)
+ grid_priors = torch.cat(mlvl_priors)
+
+ flatten_kpts = self.decode_pose(grid_priors[..., :2], kpt_preds,
+ grid_priors[..., 2])
+
+ vis_preds = torch.cat([
+ vis_pred.flatten(2).permute(0, 2, 1).contiguous()
+ for vis_pred in vis_preds
+ ],
+ dim=1)
+
+ # compute detection losses and collect targets for keypoints
+ # predictions simultaneously
+ self._log['pred_keypoints'] = list(flatten_kpts.detach().split(
+ 1, dim=0))
+ self._log['pred_keypoints_vis'] = list(vis_preds.detach().split(
+ 1, dim=0))
+
+ losses = super().loss_by_feat(cls_scores, bbox_preds, objectnesses,
+ batch_gt_instances, batch_img_metas,
+ batch_gt_instances_ignore)
+
+ kpt_targets, vis_targets = [], []
+ sampling_results = self.sampler.log['sample']
+ sampling_result_idx = 0
+ for gt_instances in batch_gt_instances:
+ if len(gt_instances) > 0:
+ sampling_result = sampling_results[sampling_result_idx]
+ kpt_target = gt_instances['keypoints'][
+ sampling_result.pos_assigned_gt_inds]
+ vis_target = gt_instances['keypoints_visible'][
+ sampling_result.pos_assigned_gt_inds]
+ sampling_result_idx += 1
+ kpt_targets.append(kpt_target)
+ vis_targets.append(vis_target)
+
+ if len(kpt_targets) > 0:
+ kpt_targets = torch.cat(kpt_targets, 0)
+ vis_targets = torch.cat(vis_targets, 0)
+
+ # compute keypoint losses
+ if len(kpt_targets) > 0:
+ vis_targets = (vis_targets > 0).float()
+ pos_masks = torch.cat(self._log['foreground_mask'], 0)
+ bbox_targets = torch.cat(self._log['bbox_target'], 0)
+ loss_kpt = self.loss_pose(
+ flatten_kpts.view(-1, self.num_keypoints, 2)[pos_masks],
+ kpt_targets, vis_targets, bbox_targets)
+ loss_vis = self.loss_cls(
+ vis_preds.view(-1, self.num_keypoints)[pos_masks],
+ vis_targets) / vis_targets.sum()
+ else:
+ loss_kpt = kpt_preds.sum() * 0
+ loss_vis = vis_preds.sum() * 0
+
+ losses.update(dict(loss_kpt=loss_kpt, loss_vis=loss_vis))
+
+ self._clear()
+ return losses
+
+ @torch.no_grad()
+ def _get_targets_single(
+ self,
+ priors: Tensor,
+ cls_preds: Tensor,
+ decoded_bboxes: Tensor,
+ objectness: Tensor,
+ gt_instances: InstanceData,
+ img_meta: dict,
+ gt_instances_ignore: Optional[InstanceData] = None) -> tuple:
+ """Calculates targets for a single image, and saves them to the log.
+
+ This method is similar to the _get_targets_single method in the base
+ class, but additionally saves the foreground mask and bbox targets to
+ the log.
+ """
+
+ # Construct a combined representation of bboxes and keypoints to
+ # ensure keypoints are also involved in the positive sample
+ # assignment process
+ kpt = self._log['pred_keypoints'].pop(0).squeeze(0)
+ kpt_vis = self._log['pred_keypoints_vis'].pop(0).squeeze(0)
+ kpt = torch.cat((kpt, kpt_vis.unsqueeze(-1)), dim=-1)
+ decoded_bboxes = torch.cat((decoded_bboxes, kpt.flatten(1)), dim=1)
+
+ targets = super()._get_targets_single(priors, cls_preds,
+ decoded_bboxes, objectness,
+ gt_instances, img_meta,
+ gt_instances_ignore)
+ self._log['foreground_mask'].append(targets[0])
+ self._log['bbox_target'].append(targets[3])
+ return targets
+
+ def predict_by_feat(self,
+ cls_scores: List[Tensor],
+ bbox_preds: List[Tensor],
+ objectnesses: Optional[List[Tensor]] = None,
+ kpt_preds: Optional[List[Tensor]] = None,
+ vis_preds: Optional[List[Tensor]] = None,
+ batch_img_metas: Optional[List[dict]] = None,
+ cfg: Optional[ConfigDict] = None,
+ rescale: bool = True,
+ with_nms: bool = True) -> List[InstanceData]:
+ """Transform a batch of output features extracted by the head into bbox
+ and keypoint results.
+
+ In addition to the base class method, keypoint predictions are also
+ calculated in this method.
+ """
+ """calculate predicted bboxes and get the kept instances indices.
+
+ use OutputSaveFunctionWrapper as context manager to obtain
+ intermediate output from a parent class without copying a
+ arge block of code
+ """
+ with OutputSaveFunctionWrapper(
+ filter_scores_and_topk,
+ super().predict_by_feat.__globals__) as outputs_1:
+ with OutputSaveFunctionWrapper(
+ batched_nms,
+ super()._bbox_post_process.__globals__) as outputs_2:
+ results_list = super().predict_by_feat(cls_scores, bbox_preds,
+ objectnesses,
+ batch_img_metas, cfg,
+ rescale, with_nms)
+ keep_indices_topk = [
+ out[2][:cfg.max_per_img] for out in outputs_1
+ ]
+ keep_indices_nms = [
+ out[1][:cfg.max_per_img] for out in outputs_2
+ ]
+
+ num_imgs = len(batch_img_metas)
+
+ # recover keypoints coordinates from model predictions
+ featmap_sizes = [vis_pred.shape[2:] for vis_pred in vis_preds]
+ priors = torch.cat(self.mlvl_priors)
+ strides = [
+ priors.new_full((featmap_size.numel() * self.num_base_priors, ),
+ stride) for featmap_size, stride in zip(
+ featmap_sizes, self.featmap_strides)
+ ]
+ strides = torch.cat(strides)
+ kpt_preds = torch.cat([
+ kpt_pred.permute(0, 2, 3, 1).reshape(
+ num_imgs, -1, self.num_keypoints * 2) for kpt_pred in kpt_preds
+ ],
+ dim=1)
+ flatten_decoded_kpts = self.decode_pose(priors, kpt_preds, strides)
+
+ vis_preds = torch.cat([
+ vis_pred.permute(0, 2, 3, 1).reshape(
+ num_imgs, -1, self.num_keypoints) for vis_pred in vis_preds
+ ],
+ dim=1).sigmoid()
+
+ # select keypoints predictions according to bbox scores and nms result
+ keep_indices_nms_idx = 0
+ for pred_instances, kpts, kpts_vis, img_meta, keep_idxs \
+ in zip(
+ results_list, flatten_decoded_kpts, vis_preds,
+ batch_img_metas, keep_indices_topk):
+
+ pred_instances.bbox_scores = pred_instances.scores
+
+ if len(pred_instances) == 0:
+ pred_instances.keypoints = kpts[:0]
+ pred_instances.keypoint_scores = kpts_vis[:0]
+ continue
+
+ kpts = kpts[keep_idxs]
+ kpts_vis = kpts_vis[keep_idxs]
+
+ if rescale:
+ pad_param = img_meta.get('img_meta', None)
+ scale_factor = img_meta['scale_factor']
+ if pad_param is not None:
+ kpts -= kpts.new_tensor([pad_param[2], pad_param[0]])
+ kpts /= kpts.new_tensor(scale_factor).repeat(
+ (1, self.num_keypoints, 1))
+
+ keep_idxs_nms = keep_indices_nms[keep_indices_nms_idx]
+ kpts = kpts[keep_idxs_nms]
+ kpts_vis = kpts_vis[keep_idxs_nms]
+ keep_indices_nms_idx += 1
+
+ pred_instances.keypoints = kpts
+ pred_instances.keypoint_scores = kpts_vis
+
+ results_list = [r.numpy() for r in results_list]
+ return results_list
+
+ def decode_pose(self, grids: torch.Tensor, offsets: torch.Tensor,
+ strides: Union[torch.Tensor, int]) -> torch.Tensor:
+ """Decode regression offsets to keypoints.
+
+ Args:
+ grids (torch.Tensor): The coordinates of the feature map grids.
+ offsets (torch.Tensor): The predicted offset of each keypoint
+ relative to its corresponding grid.
+ strides (torch.Tensor | int): The stride of the feature map for
+ each instance.
+ Returns:
+ torch.Tensor: The decoded keypoints coordinates.
+ """
+
+ if isinstance(strides, int):
+ strides = torch.tensor([strides]).to(offsets)
+
+ strides = strides.reshape(1, -1, 1, 1)
+ offsets = offsets.reshape(*offsets.shape[:2], -1, 2)
+ xy_coordinates = (offsets[..., :2] * strides) + grids.unsqueeze(1)
+ return xy_coordinates
+
+ @staticmethod
+ def gt_kps_instances_preprocess(batch_gt_instances: Tensor,
+ batch_gt_keypoints,
+ batch_gt_keypoints_visible,
+ batch_size: int) -> List[InstanceData]:
+ """Split batch_gt_instances with batch size.
+
+ Args:
+ batch_gt_instances (Tensor): Ground truth
+ a 2D-Tensor for whole batch, shape [all_gt_bboxes, 6]
+ batch_size (int): Batch size.
+
+ Returns:
+ List: batch gt instances data, shape [batch_size, InstanceData]
+ """
+ # faster version
+ batch_instance_list = []
+ for i in range(batch_size):
+ batch_gt_instance_ = InstanceData()
+ single_batch_instance = \
+ batch_gt_instances[batch_gt_instances[:, 0] == i, :]
+ keypoints = \
+ batch_gt_keypoints[batch_gt_instances[:, 0] == i, :]
+ keypoints_visible = \
+ batch_gt_keypoints_visible[batch_gt_instances[:, 0] == i, :]
+ batch_gt_instance_.bboxes = single_batch_instance[:, 2:]
+ batch_gt_instance_.labels = single_batch_instance[:, 1]
+ batch_gt_instance_.keypoints = keypoints
+ batch_gt_instance_.keypoints_visible = keypoints_visible
+ batch_instance_list.append(batch_gt_instance_)
+
+ return batch_instance_list
+
+ @staticmethod
+ def gt_instances_preprocess(batch_gt_instances: List[InstanceData], *args,
+ **kwargs) -> List[InstanceData]:
+ return batch_gt_instances
diff --git a/mmyolo/models/losses/__init__.py b/mmyolo/models/losses/__init__.py
index ee192921..c89fe4dc 100644
--- a/mmyolo/models/losses/__init__.py
+++ b/mmyolo/models/losses/__init__.py
@@ -1,4 +1,5 @@
# Copyright (c) OpenMMLab. All rights reserved.
from .iou_loss import IoULoss, bbox_overlaps
+from .oks_loss import OksLoss
-__all__ = ['IoULoss', 'bbox_overlaps']
+__all__ = ['IoULoss', 'bbox_overlaps', 'OksLoss']
diff --git a/mmyolo/models/losses/oks_loss.py b/mmyolo/models/losses/oks_loss.py
new file mode 100644
index 00000000..8440f06e
--- /dev/null
+++ b/mmyolo/models/losses/oks_loss.py
@@ -0,0 +1,88 @@
+# Copyright (c) OpenMMLab. All rights reserved.
+from typing import Optional
+
+import torch
+import torch.nn as nn
+from torch import Tensor
+
+from mmyolo.registry import MODELS
+
+try:
+ from mmpose.datasets.datasets.utils import parse_pose_metainfo
+except ImportError:
+ raise ImportError('Please run "mim install -r requirements/mmpose.txt" '
+ 'to install mmpose first for rotated detection.')
+
+
+@MODELS.register_module()
+class OksLoss(nn.Module):
+ """A PyTorch implementation of the Object Keypoint Similarity (OKS) loss as
+ described in the paper "YOLO-Pose: Enhancing YOLO for Multi Person Pose
+ Estimation Using Object Keypoint Similarity Loss" by Debapriya et al.
+
+ (2022).
+ The OKS loss is used for keypoint-based object recognition and consists
+ of a measure of the similarity between predicted and ground truth
+ keypoint locations, adjusted by the size of the object in the image.
+ The loss function takes as input the predicted keypoint locations, the
+ ground truth keypoint locations, a mask indicating which keypoints are
+ valid, and bounding boxes for the objects.
+ Args:
+ metainfo (Optional[str]): Path to a JSON file containing information
+ about the dataset's annotations.
+ loss_weight (float): Weight for the loss.
+ """
+
+ def __init__(self,
+ metainfo: Optional[str] = None,
+ loss_weight: float = 1.0):
+ super().__init__()
+
+ if metainfo is not None:
+ metainfo = parse_pose_metainfo(dict(from_file=metainfo))
+ sigmas = metainfo.get('sigmas', None)
+ if sigmas is not None:
+ self.register_buffer('sigmas', torch.as_tensor(sigmas))
+ self.loss_weight = loss_weight
+
+ def forward(self,
+ output: Tensor,
+ target: Tensor,
+ target_weights: Tensor,
+ bboxes: Optional[Tensor] = None) -> Tensor:
+ oks = self.compute_oks(output, target, target_weights, bboxes)
+ loss = 1 - oks
+ return loss * self.loss_weight
+
+ def compute_oks(self,
+ output: Tensor,
+ target: Tensor,
+ target_weights: Tensor,
+ bboxes: Optional[Tensor] = None) -> Tensor:
+ """Calculates the OKS loss.
+
+ Args:
+ output (Tensor): Predicted keypoints in shape N x k x 2, where N
+ is batch size, k is the number of keypoints, and 2 are the
+ xy coordinates.
+ target (Tensor): Ground truth keypoints in the same shape as
+ output.
+ target_weights (Tensor): Mask of valid keypoints in shape N x k,
+ with 1 for valid and 0 for invalid.
+ bboxes (Optional[Tensor]): Bounding boxes in shape N x 4,
+ where 4 are the xyxy coordinates.
+ Returns:
+ Tensor: The calculated OKS loss.
+ """
+
+ dist = torch.norm(output - target, dim=-1)
+
+ if hasattr(self, 'sigmas'):
+ sigmas = self.sigmas.reshape(*((1, ) * (dist.ndim - 1)), -1)
+ dist = dist / sigmas
+ if bboxes is not None:
+ area = torch.norm(bboxes[..., 2:] - bboxes[..., :2], dim=-1)
+ dist = dist / area.clip(min=1e-8).unsqueeze(-1)
+
+ return (torch.exp(-dist.pow(2) / 2) * target_weights).sum(
+ dim=-1) / target_weights.sum(dim=-1).clip(min=1e-8)
diff --git a/mmyolo/models/task_modules/assigners/__init__.py b/mmyolo/models/task_modules/assigners/__init__.py
index e74ab728..7b2e2e69 100644
--- a/mmyolo/models/task_modules/assigners/__init__.py
+++ b/mmyolo/models/task_modules/assigners/__init__.py
@@ -2,11 +2,13 @@
from .batch_atss_assigner import BatchATSSAssigner
from .batch_dsl_assigner import BatchDynamicSoftLabelAssigner
from .batch_task_aligned_assigner import BatchTaskAlignedAssigner
+from .pose_sim_ota_assigner import PoseSimOTAAssigner
from .utils import (select_candidates_in_gts, select_highest_overlaps,
yolov6_iou_calculator)
__all__ = [
'BatchATSSAssigner', 'BatchTaskAlignedAssigner',
'select_candidates_in_gts', 'select_highest_overlaps',
- 'yolov6_iou_calculator', 'BatchDynamicSoftLabelAssigner'
+ 'yolov6_iou_calculator', 'BatchDynamicSoftLabelAssigner',
+ 'PoseSimOTAAssigner'
]
diff --git a/mmyolo/models/task_modules/assigners/pose_sim_ota_assigner.py b/mmyolo/models/task_modules/assigners/pose_sim_ota_assigner.py
new file mode 100644
index 00000000..e66a9bf1
--- /dev/null
+++ b/mmyolo/models/task_modules/assigners/pose_sim_ota_assigner.py
@@ -0,0 +1,210 @@
+# Copyright (c) OpenMMLab. All rights reserved.
+from typing import Optional, Tuple
+
+import torch
+import torch.nn.functional as F
+from mmdet.models.task_modules.assigners import AssignResult, SimOTAAssigner
+from mmdet.utils import ConfigType
+from mmengine.structures import InstanceData
+from torch import Tensor
+
+from mmyolo.registry import MODELS, TASK_UTILS
+
+INF = 100000.0
+EPS = 1.0e-7
+
+
+@TASK_UTILS.register_module()
+class PoseSimOTAAssigner(SimOTAAssigner):
+
+ def __init__(self,
+ center_radius: float = 2.5,
+ candidate_topk: int = 10,
+ iou_weight: float = 3.0,
+ cls_weight: float = 1.0,
+ oks_weight: float = 0.0,
+ vis_weight: float = 0.0,
+ iou_calculator: ConfigType = dict(type='BboxOverlaps2D'),
+ oks_calculator: ConfigType = dict(type='OksLoss')):
+
+ self.center_radius = center_radius
+ self.candidate_topk = candidate_topk
+ self.iou_weight = iou_weight
+ self.cls_weight = cls_weight
+ self.oks_weight = oks_weight
+ self.vis_weight = vis_weight
+
+ self.iou_calculator = TASK_UTILS.build(iou_calculator)
+ self.oks_calculator = MODELS.build(oks_calculator)
+
+ def assign(self,
+ pred_instances: InstanceData,
+ gt_instances: InstanceData,
+ gt_instances_ignore: Optional[InstanceData] = None,
+ **kwargs) -> AssignResult:
+ """Assign gt to priors using SimOTA.
+
+ Args:
+ pred_instances (:obj:`InstanceData`): Instances of model
+ predictions. It includes ``priors``, and the priors can
+ be anchors or points, or the bboxes predicted by the
+ previous stage, has shape (n, 4). The bboxes predicted by
+ the current model or stage will be named ``bboxes``,
+ ``labels``, and ``scores``, the same as the ``InstanceData``
+ in other places.
+ gt_instances (:obj:`InstanceData`): Ground truth of instance
+ annotations. It usually includes ``bboxes``, with shape (k, 4),
+ and ``labels``, with shape (k, ).
+ gt_instances_ignore (:obj:`InstanceData`, optional): Instances
+ to be ignored during training. It includes ``bboxes``
+ attribute data that is ignored during training and testing.
+ Defaults to None.
+ Returns:
+ obj:`AssignResult`: The assigned result.
+ """
+ gt_bboxes = gt_instances.bboxes
+ gt_labels = gt_instances.labels
+ gt_keypoints = gt_instances.keypoints
+ gt_keypoints_visible = gt_instances.keypoints_visible
+ num_gt = gt_bboxes.size(0)
+
+ decoded_bboxes = pred_instances.bboxes[..., :4]
+ pred_kpts = pred_instances.bboxes[..., 4:]
+ pred_kpts = pred_kpts.reshape(*pred_kpts.shape[:-1], -1, 3)
+ pred_kpts_vis = pred_kpts[..., -1]
+ pred_kpts = pred_kpts[..., :2]
+ pred_scores = pred_instances.scores
+ priors = pred_instances.priors
+ num_bboxes = decoded_bboxes.size(0)
+
+ # assign 0 by default
+ assigned_gt_inds = decoded_bboxes.new_full((num_bboxes, ),
+ 0,
+ dtype=torch.long)
+ if num_gt == 0 or num_bboxes == 0:
+ # No ground truth or boxes, return empty assignment
+ max_overlaps = decoded_bboxes.new_zeros((num_bboxes, ))
+ assigned_labels = decoded_bboxes.new_full((num_bboxes, ),
+ -1,
+ dtype=torch.long)
+ return AssignResult(
+ num_gt, assigned_gt_inds, max_overlaps, labels=assigned_labels)
+
+ valid_mask, is_in_boxes_and_center = self.get_in_gt_and_in_center_info(
+ priors, gt_bboxes)
+ valid_decoded_bbox = decoded_bboxes[valid_mask]
+ valid_pred_scores = pred_scores[valid_mask]
+ valid_pred_kpts = pred_kpts[valid_mask]
+ valid_pred_kpts_vis = pred_kpts_vis[valid_mask]
+ num_valid = valid_decoded_bbox.size(0)
+ if num_valid == 0:
+ # No valid bboxes, return empty assignment
+ max_overlaps = decoded_bboxes.new_zeros((num_bboxes, ))
+ assigned_labels = decoded_bboxes.new_full((num_bboxes, ),
+ -1,
+ dtype=torch.long)
+ return AssignResult(
+ num_gt, assigned_gt_inds, max_overlaps, labels=assigned_labels)
+
+ cost_matrix = (~is_in_boxes_and_center) * INF
+
+ # calculate iou
+ pairwise_ious = self.iou_calculator(valid_decoded_bbox, gt_bboxes)
+ if self.iou_weight > 0:
+ iou_cost = -torch.log(pairwise_ious + EPS)
+ cost_matrix = cost_matrix + iou_cost * self.iou_weight
+
+ # calculate oks
+ pairwise_oks = self.oks_calculator.compute_oks(
+ valid_pred_kpts.unsqueeze(1), # [num_valid, -1, k, 2]
+ gt_keypoints.unsqueeze(0), # [1, num_gt, k, 2]
+ gt_keypoints_visible.unsqueeze(0), # [1, num_gt, k]
+ bboxes=gt_bboxes.unsqueeze(0), # [1, num_gt, 4]
+ ) # -> [num_valid, num_gt]
+ if self.oks_weight > 0:
+ oks_cost = -torch.log(pairwise_oks + EPS)
+ cost_matrix = cost_matrix + oks_cost * self.oks_weight
+
+ # calculate cls
+ if self.cls_weight > 0:
+ gt_onehot_label = (
+ F.one_hot(gt_labels.to(torch.int64),
+ pred_scores.shape[-1]).float().unsqueeze(0).repeat(
+ num_valid, 1, 1))
+
+ valid_pred_scores = valid_pred_scores.unsqueeze(1).repeat(
+ 1, num_gt, 1)
+ # disable AMP autocast to avoid overflow
+ with torch.cuda.amp.autocast(enabled=False):
+ cls_cost = (
+ F.binary_cross_entropy(
+ valid_pred_scores.to(dtype=torch.float32),
+ gt_onehot_label,
+ reduction='none',
+ ).sum(-1).to(dtype=valid_pred_scores.dtype))
+ cost_matrix = cost_matrix + cls_cost * self.cls_weight
+
+ # calculate vis
+ if self.vis_weight > 0:
+ valid_pred_kpts_vis = valid_pred_kpts_vis.sigmoid().unsqueeze(
+ 1).repeat(1, num_gt, 1) # [num_valid, 1, k]
+ gt_kpt_vis = gt_keypoints_visible.unsqueeze(
+ 0).float() # [1, num_gt, k]
+ with torch.cuda.amp.autocast(enabled=False):
+ vis_cost = (
+ F.binary_cross_entropy(
+ valid_pred_kpts_vis.to(dtype=torch.float32),
+ gt_kpt_vis.repeat(num_valid, 1, 1),
+ reduction='none',
+ ).sum(-1).to(dtype=valid_pred_kpts_vis.dtype))
+ cost_matrix = cost_matrix + vis_cost * self.vis_weight
+
+ # mixed metric
+ pairwise_oks = pairwise_oks.pow(0.5)
+ matched_pred_oks, matched_gt_inds = \
+ self.dynamic_k_matching(
+ cost_matrix, pairwise_ious, pairwise_oks, num_gt, valid_mask)
+
+ # convert to AssignResult format
+ assigned_gt_inds[valid_mask] = matched_gt_inds + 1
+ assigned_labels = assigned_gt_inds.new_full((num_bboxes, ), -1)
+ assigned_labels[valid_mask] = gt_labels[matched_gt_inds].long()
+ max_overlaps = assigned_gt_inds.new_full((num_bboxes, ),
+ -INF,
+ dtype=torch.float32)
+ max_overlaps[valid_mask] = matched_pred_oks
+ return AssignResult(
+ num_gt, assigned_gt_inds, max_overlaps, labels=assigned_labels)
+
+ def dynamic_k_matching(self, cost: Tensor, pairwise_ious: Tensor,
+ pairwise_oks: Tensor, num_gt: int,
+ valid_mask: Tensor) -> Tuple[Tensor, Tensor]:
+ """Use IoU and matching cost to calculate the dynamic top-k positive
+ targets."""
+ matching_matrix = torch.zeros_like(cost, dtype=torch.uint8)
+ # select candidate topk ious for dynamic-k calculation
+ candidate_topk = min(self.candidate_topk, pairwise_ious.size(0))
+ topk_ious, _ = torch.topk(pairwise_ious, candidate_topk, dim=0)
+ # calculate dynamic k for each gt
+ dynamic_ks = torch.clamp(topk_ious.sum(0).int(), min=1)
+ for gt_idx in range(num_gt):
+ _, pos_idx = torch.topk(
+ cost[:, gt_idx], k=dynamic_ks[gt_idx], largest=False)
+ matching_matrix[:, gt_idx][pos_idx] = 1
+
+ del topk_ious, dynamic_ks, pos_idx
+
+ prior_match_gt_mask = matching_matrix.sum(1) > 1
+ if prior_match_gt_mask.sum() > 0:
+ cost_min, cost_argmin = torch.min(
+ cost[prior_match_gt_mask, :], dim=1)
+ matching_matrix[prior_match_gt_mask, :] *= 0
+ matching_matrix[prior_match_gt_mask, cost_argmin] = 1
+ # get foreground mask inside box and center prior
+ fg_mask_inboxes = matching_matrix.sum(1) > 0
+ valid_mask[valid_mask.clone()] = fg_mask_inboxes
+
+ matched_gt_inds = matching_matrix[fg_mask_inboxes, :].argmax(1)
+ matched_pred_oks = (matching_matrix *
+ pairwise_oks).sum(1)[fg_mask_inboxes]
+ return matched_pred_oks, matched_gt_inds
diff --git a/mmyolo/models/utils/__init__.py b/mmyolo/models/utils/__init__.py
index cdfeaaf0..d62ff80e 100644
--- a/mmyolo/models/utils/__init__.py
+++ b/mmyolo/models/utils/__init__.py
@@ -1,4 +1,8 @@
# Copyright (c) OpenMMLab. All rights reserved.
-from .misc import gt_instances_preprocess, make_divisible, make_round
+from .misc import (OutputSaveFunctionWrapper, OutputSaveObjectWrapper,
+ gt_instances_preprocess, make_divisible, make_round)
-__all__ = ['make_divisible', 'make_round', 'gt_instances_preprocess']
+__all__ = [
+ 'make_divisible', 'make_round', 'gt_instances_preprocess',
+ 'OutputSaveFunctionWrapper', 'OutputSaveObjectWrapper'
+]
diff --git a/mmyolo/models/utils/misc.py b/mmyolo/models/utils/misc.py
index 531558b6..96cd1195 100644
--- a/mmyolo/models/utils/misc.py
+++ b/mmyolo/models/utils/misc.py
@@ -1,6 +1,8 @@
# Copyright (c) OpenMMLab. All rights reserved.
import math
-from typing import Sequence, Union
+from collections import defaultdict
+from copy import deepcopy
+from typing import Any, Callable, Dict, Optional, Sequence, Tuple, Union
import torch
from mmdet.structures.bbox.transforms import get_box_tensor
@@ -95,3 +97,90 @@ def gt_instances_preprocess(batch_gt_instances: Union[Tensor, Sequence],
device=batch_gt_instances.device)
return batch_instance
+
+
+class OutputSaveObjectWrapper:
+ """A wrapper class that saves the output of function calls on an object."""
+
+ def __init__(self, obj: Any) -> None:
+ self.obj = obj
+ self.log = defaultdict(list)
+
+ def __getattr__(self, attr: str) -> Any:
+ """Overrides the default behavior when an attribute is accessed.
+
+ - If the attribute is callable, hooks the attribute and saves the
+ returned value of the function call to the log.
+ - If the attribute is not callable, saves the attribute's value to the
+ log and returns the value.
+ """
+ orig_attr = getattr(self.obj, attr)
+
+ if not callable(orig_attr):
+ self.log[attr].append(orig_attr)
+ return orig_attr
+
+ def hooked(*args: Tuple, **kwargs: Dict) -> Any:
+ """The hooked function that logs the return value of the original
+ function."""
+ result = orig_attr(*args, **kwargs)
+ self.log[attr].append(result)
+ return result
+
+ return hooked
+
+ def clear(self):
+ """Clears the log of function call outputs."""
+ self.log.clear()
+
+ def __deepcopy__(self, memo):
+ """Only copy the object when applying deepcopy."""
+ other = type(self)(deepcopy(self.obj))
+ memo[id(self)] = other
+ return other
+
+
+class OutputSaveFunctionWrapper:
+ """A class that wraps a function and saves its outputs.
+
+ This class can be used to decorate a function to save its outputs. It wraps
+ the function with a `__call__` method that calls the original function and
+ saves the results in a log attribute.
+ Args:
+ func (Callable): A function to wrap.
+ spec (Optional[Dict]): A dictionary of global variables to use as the
+ namespace for the wrapper. If `None`, the global namespace of the
+ original function is used.
+ """
+
+ def __init__(self, func: Callable, spec: Optional[Dict]) -> None:
+ """Initializes the OutputSaveFunctionWrapper instance."""
+ assert callable(func)
+ self.log = []
+ self.func = func
+ self.func_name = func.__name__
+
+ if isinstance(spec, dict):
+ self.spec = spec
+ elif hasattr(func, '__globals__'):
+ self.spec = func.__globals__
+ else:
+ raise ValueError
+
+ def __call__(self, *args, **kwargs) -> Any:
+ """Calls the wrapped function with the given arguments and saves the
+ results in the `log` attribute."""
+ results = self.func(*args, **kwargs)
+ self.log.append(results)
+ return results
+
+ def __enter__(self) -> None:
+ """Enters the context and sets the wrapped function to be a global
+ variable in the specified namespace."""
+ self.spec[self.func_name] = self
+ return self.log
+
+ def __exit__(self, exc_type, exc_val, exc_tb) -> None:
+ """Exits the context and resets the wrapped function to its original
+ value in the specified namespace."""
+ self.spec[self.func_name] = self.func
diff --git a/requirements/mmpose.txt b/requirements/mmpose.txt
new file mode 100644
index 00000000..8e4726e6
--- /dev/null
+++ b/requirements/mmpose.txt
@@ -0,0 +1 @@
+mmpose>=1.0.0
diff --git a/requirements/tests.txt b/requirements/tests.txt
index 55ea3663..285b3f39 100644
--- a/requirements/tests.txt
+++ b/requirements/tests.txt
@@ -5,6 +5,7 @@ isort==4.3.21
kwarray
memory_profiler
mmcls>=1.0.0rc4
+mmpose>=1.0.0
mmrazor>=1.0.0rc2
mmrotate>=1.0.0rc1
parameterized
diff --git a/tests/test_models/test_dense_heads/test_yolox_head.py b/tests/test_models/test_dense_heads/test_yolox_head.py
index 60e0abe9..39099441 100644
--- a/tests/test_models/test_dense_heads/test_yolox_head.py
+++ b/tests/test_models/test_dense_heads/test_yolox_head.py
@@ -6,7 +6,7 @@ from mmengine.config import Config
from mmengine.model import bias_init_with_prob
from mmengine.testing import assert_allclose
-from mmyolo.models.dense_heads import YOLOXHead
+from mmyolo.models.dense_heads import YOLOXHead, YOLOXPoseHead
from mmyolo.utils import register_all_modules
register_all_modules()
@@ -157,3 +157,223 @@ class TestYOLOXHead(TestCase):
'there should be no box loss when gt_bboxes out of bound')
self.assertGreater(empty_obj_loss.item(), 0,
'objectness loss should be non-zero')
+
+
+class TestYOLOXPoseHead(TestCase):
+
+ def setUp(self):
+ self.head_module = dict(
+ type='YOLOXPoseHeadModule',
+ num_classes=1,
+ num_keypoints=17,
+ in_channels=1,
+ stacked_convs=1,
+ )
+ self.train_cfg = Config(
+ dict(
+ assigner=dict(
+ type='PoseSimOTAAssigner',
+ center_radius=2.5,
+ oks_weight=3.0,
+ iou_calculator=dict(type='mmdet.BboxOverlaps2D'),
+ oks_calculator=dict(
+ type='OksLoss',
+ metainfo='configs/_base_/pose/coco.py'))))
+ self.loss_pose = Config(
+ dict(
+ type='OksLoss',
+ metainfo='configs/_base_/pose/coco.py',
+ loss_weight=30.0))
+
+ def test_init_weights(self):
+ head = YOLOXPoseHead(
+ head_module=self.head_module,
+ loss_pose=self.loss_pose,
+ train_cfg=self.train_cfg)
+ head.head_module.init_weights()
+ bias_init = bias_init_with_prob(0.01)
+ for conv_cls, conv_obj, conv_vis in zip(
+ head.head_module.multi_level_conv_cls,
+ head.head_module.multi_level_conv_obj,
+ head.head_module.multi_level_conv_vis):
+ assert_allclose(conv_cls.bias.data,
+ torch.ones_like(conv_cls.bias.data) * bias_init)
+ assert_allclose(conv_obj.bias.data,
+ torch.ones_like(conv_obj.bias.data) * bias_init)
+ assert_allclose(conv_vis.bias.data,
+ torch.ones_like(conv_vis.bias.data) * bias_init)
+
+ def test_predict_by_feat(self):
+ s = 256
+ img_metas = [{
+ 'img_shape': (s, s, 3),
+ 'ori_shape': (s, s, 3),
+ 'scale_factor': (1.0, 1.0),
+ }]
+ test_cfg = Config(
+ dict(
+ multi_label=True,
+ max_per_img=300,
+ score_thr=0.01,
+ nms=dict(type='nms', iou_threshold=0.65)))
+
+ head = YOLOXPoseHead(
+ head_module=self.head_module,
+ loss_pose=self.loss_pose,
+ train_cfg=self.train_cfg,
+ test_cfg=test_cfg)
+ feat = [
+ torch.rand(1, 1, s // feat_size, s // feat_size)
+ for feat_size in [4, 8, 16]
+ ]
+ cls_scores, bbox_preds, objectnesses, \
+ offsets_preds, vis_preds = head.forward(feat)
+ head.predict_by_feat(
+ cls_scores,
+ bbox_preds,
+ objectnesses,
+ offsets_preds,
+ vis_preds,
+ img_metas,
+ cfg=test_cfg,
+ rescale=True,
+ with_nms=True)
+
+ def test_loss_by_feat(self):
+ s = 256
+ img_metas = [{
+ 'img_shape': (s, s, 3),
+ 'scale_factor': 1,
+ }]
+
+ head = YOLOXPoseHead(
+ head_module=self.head_module,
+ loss_pose=self.loss_pose,
+ train_cfg=self.train_cfg)
+ assert not head.use_bbox_aux
+
+ feat = [
+ torch.rand(1, 1, s // feat_size, s // feat_size)
+ for feat_size in [4, 8, 16]
+ ]
+ cls_scores, bbox_preds, objectnesses, \
+ offsets_preds, vis_preds = head.forward(feat)
+
+ # Test that empty ground truth encourages the network to predict
+ # background
+ gt_instances = torch.empty((0, 6))
+ gt_keypoints = torch.empty((0, 17, 2))
+ gt_keypoints_visible = torch.empty((0, 17))
+
+ empty_gt_losses = head.loss_by_feat(cls_scores, bbox_preds,
+ objectnesses, offsets_preds,
+ vis_preds, gt_instances,
+ gt_keypoints, gt_keypoints_visible,
+ img_metas)
+ # When there is no truth, the cls loss should be nonzero but there
+ # should be no box loss.
+ empty_cls_loss = empty_gt_losses['loss_cls'].sum()
+ empty_box_loss = empty_gt_losses['loss_bbox'].sum()
+ empty_obj_loss = empty_gt_losses['loss_obj'].sum()
+ empty_loss_kpt = empty_gt_losses['loss_kpt'].sum()
+ empty_loss_vis = empty_gt_losses['loss_vis'].sum()
+ self.assertEqual(
+ empty_cls_loss.item(), 0,
+ 'there should be no cls loss when there are no true boxes')
+ self.assertEqual(
+ empty_box_loss.item(), 0,
+ 'there should be no box loss when there are no true boxes')
+ self.assertGreater(empty_obj_loss.item(), 0,
+ 'objectness loss should be non-zero')
+ self.assertEqual(
+ empty_loss_kpt.item(), 0,
+ 'there should be no kpt loss when there are no true keypoints')
+ self.assertEqual(
+ empty_loss_vis.item(), 0,
+ 'there should be no vis loss when there are no true keypoints')
+ # When truth is non-empty then both cls and box loss should be nonzero
+ # for random inputs
+ head = YOLOXPoseHead(
+ head_module=self.head_module,
+ loss_pose=self.loss_pose,
+ train_cfg=self.train_cfg)
+ gt_instances = torch.Tensor(
+ [[0, 0, 23.6667, 23.8757, 238.6326, 151.8874]])
+ gt_keypoints = torch.Tensor([[[317.1519,
+ 429.8433], [338.3080, 416.9187],
+ [298.9951,
+ 403.8911], [102.7025, 273.1329],
+ [255.4321,
+ 404.8712], [400.0422, 554.4373],
+ [167.7857,
+ 516.7591], [397.4943, 737.4575],
+ [116.3247,
+ 674.5684], [102.7025, 273.1329],
+ [66.0319,
+ 808.6383], [102.7025, 273.1329],
+ [157.6150,
+ 819.1249], [102.7025, 273.1329],
+ [102.7025,
+ 273.1329], [102.7025, 273.1329],
+ [102.7025, 273.1329]]])
+ gt_keypoints_visible = torch.Tensor([[
+ 1., 1., 1., 0., 1., 1., 1., 0., 0., 0., 0., 0., 0., 0., 0., 0., 0.
+ ]])
+
+ one_gt_losses = head.loss_by_feat(cls_scores, bbox_preds, objectnesses,
+ offsets_preds, vis_preds,
+ gt_instances, gt_keypoints,
+ gt_keypoints_visible, img_metas)
+ onegt_cls_loss = one_gt_losses['loss_cls'].sum()
+ onegt_box_loss = one_gt_losses['loss_bbox'].sum()
+ onegt_obj_loss = one_gt_losses['loss_obj'].sum()
+ onegt_loss_kpt = one_gt_losses['loss_kpt'].sum()
+ onegt_loss_vis = one_gt_losses['loss_vis'].sum()
+
+ self.assertGreater(onegt_cls_loss.item(), 0,
+ 'cls loss should be non-zero')
+ self.assertGreater(onegt_box_loss.item(), 0,
+ 'box loss should be non-zero')
+ self.assertGreater(onegt_obj_loss.item(), 0,
+ 'obj loss should be non-zero')
+ self.assertGreater(onegt_loss_kpt.item(), 0,
+ 'kpt loss should be non-zero')
+ self.assertGreater(onegt_loss_vis.item(), 0,
+ 'vis loss should be non-zero')
+
+ # Test groud truth out of bound
+ gt_instances = torch.Tensor(
+ [[0, 2, s * 4, s * 4, s * 4 + 10, s * 4 + 10]])
+ gt_keypoints = torch.Tensor([[[s * 4, s * 4 + 10], [s * 4, s * 4 + 10],
+ [s * 4, s * 4 + 10], [s * 4, s * 4 + 10],
+ [s * 4, s * 4 + 10], [s * 4, s * 4 + 10],
+ [s * 4, s * 4 + 10], [s * 4, s * 4 + 10],
+ [s * 4, s * 4 + 10], [s * 4, s * 4 + 10],
+ [s * 4, s * 4 + 10], [s * 4, s * 4 + 10],
+ [s * 4, s * 4 + 10], [s * 4, s * 4 + 10],
+ [s * 4, s * 4 + 10], [s * 4, s * 4 + 10],
+ [s * 4, s * 4 + 10]]])
+ empty_gt_losses = head.loss_by_feat(cls_scores, bbox_preds,
+ objectnesses, offsets_preds,
+ vis_preds, gt_instances,
+ gt_keypoints, gt_keypoints_visible,
+ img_metas)
+ # When gt_bboxes out of bound, the assign results should be empty,
+ # so the cls and bbox loss should be zero.
+ empty_cls_loss = empty_gt_losses['loss_cls'].sum()
+ empty_box_loss = empty_gt_losses['loss_bbox'].sum()
+ empty_obj_loss = empty_gt_losses['loss_obj'].sum()
+ empty_kpt_loss = empty_gt_losses['loss_kpt'].sum()
+ empty_vis_loss = empty_gt_losses['loss_vis'].sum()
+ self.assertEqual(
+ empty_cls_loss.item(), 0,
+ 'there should be no cls loss when gt_bboxes out of bound')
+ self.assertEqual(
+ empty_box_loss.item(), 0,
+ 'there should be no box loss when gt_bboxes out of bound')
+ self.assertGreater(empty_obj_loss.item(), 0,
+ 'objectness loss should be non-zero')
+ self.assertEqual(empty_kpt_loss.item(), 0,
+ 'kps loss should be non-zero')
+ self.assertEqual(empty_vis_loss.item(), 0,
+ 'vis loss should be non-zero')
diff --git a/tests/test_models/test_task_modules/test_assigners/test_pose_sim_ota_assigner.py b/tests/test_models/test_task_modules/test_assigners/test_pose_sim_ota_assigner.py
new file mode 100644
index 00000000..fb4793f7
--- /dev/null
+++ b/tests/test_models/test_task_modules/test_assigners/test_pose_sim_ota_assigner.py
@@ -0,0 +1,85 @@
+# Copyright (c) OpenMMLab. All rights reserved.
+from unittest import TestCase
+
+import torch
+from mmengine.structures import InstanceData
+from mmengine.testing import assert_allclose
+
+from mmyolo.models.task_modules.assigners import PoseSimOTAAssigner
+
+
+class TestPoseSimOTAAssigner(TestCase):
+
+ def test_assign(self):
+ assigner = PoseSimOTAAssigner(
+ center_radius=2.5,
+ candidate_topk=1,
+ iou_weight=3.0,
+ cls_weight=1.0,
+ iou_calculator=dict(type='mmdet.BboxOverlaps2D'))
+ pred_instances = InstanceData(
+ bboxes=torch.Tensor([[23, 23, 43, 43] + [1] * 51,
+ [4, 5, 6, 7] + [1] * 51]),
+ scores=torch.FloatTensor([[0.2], [0.8]]),
+ priors=torch.Tensor([[30, 30, 8, 8], [4, 5, 6, 7]]))
+ gt_instances = InstanceData(
+ bboxes=torch.Tensor([[23, 23, 43, 43]]),
+ labels=torch.LongTensor([0]),
+ keypoints_visible=torch.Tensor([[
+ 1., 1., 1., 1., 1., 1., 1., 1., 0., 1., 1., 0., 0., 0., 0., 0.,
+ 0.
+ ]]),
+ keypoints=torch.Tensor([[[30, 30], [30, 30], [30, 30], [30, 30],
+ [30, 30], [30, 30], [30, 30], [30, 30],
+ [30, 30], [30, 30], [30, 30], [30, 30],
+ [30, 30], [30, 30], [30, 30], [30, 30],
+ [30, 30]]]))
+ assign_result = assigner.assign(
+ pred_instances=pred_instances, gt_instances=gt_instances)
+
+ expected_gt_inds = torch.LongTensor([1, 0])
+ assert_allclose(assign_result.gt_inds, expected_gt_inds)
+
+ def test_assign_with_no_valid_bboxes(self):
+ assigner = PoseSimOTAAssigner(
+ center_radius=2.5,
+ candidate_topk=1,
+ iou_weight=3.0,
+ cls_weight=1.0,
+ iou_calculator=dict(type='mmdet.BboxOverlaps2D'))
+ pred_instances = InstanceData(
+ bboxes=torch.Tensor([[123, 123, 143, 143], [114, 151, 161, 171]]),
+ scores=torch.FloatTensor([[0.2], [0.8]]),
+ priors=torch.Tensor([[30, 30, 8, 8], [55, 55, 8, 8]]))
+ gt_instances = InstanceData(
+ bboxes=torch.Tensor([[0, 0, 1, 1]]),
+ labels=torch.LongTensor([0]),
+ keypoints_visible=torch.zeros((1, 17)),
+ keypoints=torch.zeros((1, 17, 2)))
+ assign_result = assigner.assign(
+ pred_instances=pred_instances, gt_instances=gt_instances)
+
+ expected_gt_inds = torch.LongTensor([0, 0])
+ assert_allclose(assign_result.gt_inds, expected_gt_inds)
+
+ def test_assign_with_empty_gt(self):
+ assigner = PoseSimOTAAssigner(
+ center_radius=2.5,
+ candidate_topk=1,
+ iou_weight=3.0,
+ cls_weight=1.0,
+ iou_calculator=dict(type='mmdet.BboxOverlaps2D'))
+ pred_instances = InstanceData(
+ bboxes=torch.Tensor([[[30, 40, 50, 60]], [[4, 5, 6, 7]]]),
+ scores=torch.FloatTensor([[0.2], [0.8]]),
+ priors=torch.Tensor([[0, 12, 23, 34], [4, 5, 6, 7]]))
+ gt_instances = InstanceData(
+ bboxes=torch.empty(0, 4),
+ labels=torch.empty(0),
+ keypoints_visible=torch.empty(0, 17),
+ keypoints=torch.empty(0, 17, 2))
+
+ assign_result = assigner.assign(
+ pred_instances=pred_instances, gt_instances=gt_instances)
+ expected_gt_inds = torch.LongTensor([0, 0])
+ assert_allclose(assign_result.gt_inds, expected_gt_inds)
diff --git a/tools/analysis_tools/browse_dataset.py b/tools/analysis_tools/browse_dataset.py
index 42bcade3..21a1d709 100644
--- a/tools/analysis_tools/browse_dataset.py
+++ b/tools/analysis_tools/browse_dataset.py
@@ -19,6 +19,7 @@ from mmyolo.registry import DATASETS, VISUALIZERS
# TODO: Support for printing the change in key of results
+# TODO: Some bug. If you meet some bug, please use the original
def parse_args():
parser = argparse.ArgumentParser(description='Browse a dataset')
parser.add_argument('config', help='train config file path')
diff --git a/tools/analysis_tools/browse_dataset_simple.py b/tools/analysis_tools/browse_dataset_simple.py
new file mode 100644
index 00000000..ebacbde3
--- /dev/null
+++ b/tools/analysis_tools/browse_dataset_simple.py
@@ -0,0 +1,89 @@
+# Copyright (c) OpenMMLab. All rights reserved.
+import argparse
+import os.path as osp
+
+from mmdet.models.utils import mask2ndarray
+from mmdet.structures.bbox import BaseBoxes
+from mmengine.config import Config, DictAction
+from mmengine.registry import init_default_scope
+from mmengine.utils import ProgressBar
+
+from mmyolo.registry import DATASETS, VISUALIZERS
+
+
+def parse_args():
+ parser = argparse.ArgumentParser(description='Browse a dataset')
+ parser.add_argument('config', help='train config file path')
+ parser.add_argument(
+ '--output-dir',
+ default=None,
+ type=str,
+ help='If there is no display interface, you can save it')
+ parser.add_argument('--not-show', default=False, action='store_true')
+ parser.add_argument(
+ '--show-interval',
+ type=float,
+ default=0,
+ help='the interval of show (s)')
+ parser.add_argument(
+ '--cfg-options',
+ nargs='+',
+ action=DictAction,
+ help='override some settings in the used config, the key-value pair '
+ 'in xxx=yyy format will be merged into config file. If the value to '
+ 'be overwritten is a list, it should be like key="[a,b]" or key=a,b '
+ 'It also allows nested list/tuple values, e.g. key="[(a,b),(c,d)]" '
+ 'Note that the quotation marks are necessary and that no white space '
+ 'is allowed.')
+ args = parser.parse_args()
+ return args
+
+
+def main():
+ args = parse_args()
+ cfg = Config.fromfile(args.config)
+ if args.cfg_options is not None:
+ cfg.merge_from_dict(args.cfg_options)
+
+ # register all modules in mmdet into the registries
+ init_default_scope(cfg.get('default_scope', 'mmyolo'))
+
+ dataset = DATASETS.build(cfg.train_dataloader.dataset)
+ visualizer = VISUALIZERS.build(cfg.visualizer)
+ visualizer.dataset_meta = dataset.metainfo
+
+ progress_bar = ProgressBar(len(dataset))
+ for item in dataset:
+ img = item['inputs'].permute(1, 2, 0).numpy()
+ data_sample = item['data_samples'].numpy()
+ gt_instances = data_sample.gt_instances
+ img_path = osp.basename(item['data_samples'].img_path)
+
+ out_file = osp.join(
+ args.output_dir,
+ osp.basename(img_path)) if args.output_dir is not None else None
+
+ img = img[..., [2, 1, 0]] # bgr to rgb
+ gt_bboxes = gt_instances.get('bboxes', None)
+ if gt_bboxes is not None and isinstance(gt_bboxes, BaseBoxes):
+ gt_instances.bboxes = gt_bboxes.tensor
+ gt_masks = gt_instances.get('masks', None)
+ if gt_masks is not None:
+ masks = mask2ndarray(gt_masks)
+ gt_instances.masks = masks.astype(bool)
+ data_sample.gt_instances = gt_instances
+
+ visualizer.add_datasample(
+ osp.basename(img_path),
+ img,
+ data_sample,
+ draw_pred=False,
+ show=not args.not_show,
+ wait_time=args.show_interval,
+ out_file=out_file)
+
+ progress_bar.update()
+
+
+if __name__ == '__main__':
+ main()