mirror of https://github.com/open-mmlab/mmyolo.git
[Docs] Deployment translation (#289)
* translate deployment docs * translate deployment docs part 2 * Update docs/zh_cn/deploy/yolov5_deployment.md Co-authored-by: Range King <RangeKingHZ@gmail.com> * Update docs/zh_cn/deploy/yolov5_deployment.md Co-authored-by: Range King <RangeKingHZ@gmail.com> * Update docs/zh_cn/deploy/yolov5_deployment.md Co-authored-by: Range King <RangeKingHZ@gmail.com> * Update docs/zh_cn/deploy/yolov5_deployment.md Co-authored-by: Range King <RangeKingHZ@gmail.com> * Update docs/zh_cn/deploy/yolov5_deployment.md Co-authored-by: Range King <RangeKingHZ@gmail.com> * Update docs/zh_cn/deploy/yolov5_deployment.md Co-authored-by: Range King <RangeKingHZ@gmail.com> * Update docs/zh_cn/deploy/yolov5_deployment.md Co-authored-by: Range King <RangeKingHZ@gmail.com> * Update docs/zh_cn/deploy/yolov5_deployment.md Co-authored-by: Range King <RangeKingHZ@gmail.com> * Update docs/en/deploy/basic_deployment_guide.md Co-authored-by: HinGwenWoong <peterhuang0323@qq.com> * Update docs/en/deploy/basic_deployment_guide.md Co-authored-by: HinGwenWoong <peterhuang0323@qq.com> * Update docs/en/deploy/basic_deployment_guide.md Co-authored-by: HinGwenWoong <peterhuang0323@qq.com> * debug link representation in readthedocs * debug link representation in readthedocs * debug link representation in readthedocs Co-authored-by: Range King <RangeKingHZ@gmail.com> Co-authored-by: HinGwenWoong <peterhuang0323@qq.com>pull/286/head
parent
daae790075
commit
7440a68e51
|
@ -1 +1,287 @@
|
|||
# Basic Deployment Guide
|
||||
|
||||
## Introduction of MMDeploy
|
||||
|
||||
MMDeploy is an open-source deep learning model deployment toolset. It is a part of the [OpenMMLab](https://openmmlab.com/) project, and provides **a unified experience of exporting different models** to various platforms and devices of the OpenMMLab series libraries. Using MMDeploy, developers can easily export the specific compiled SDK they need from the training result, which saves a lot of effort.
|
||||
|
||||
More detailed introduction and guides can be found [here](https://github.com/open-mmlab/mmdeploy/blob/dev-1.x/docs/en/get_started.md)
|
||||
|
||||
## Supported Algorithms
|
||||
|
||||
Currently our deployment kit supports on the following models and backends:
|
||||
|
||||
| Model | Task | OnnxRuntime | TensorRT | Model config |
|
||||
| :----- | :-------------- | :---------: | :------: | :---------------------------------------------------------------------: |
|
||||
| YOLOv5 | ObjectDetection | Y | Y | [config](https://github.com/open-mmlab/mmyolo/tree/main/configs/yolov5) |
|
||||
| YOLOv6 | ObjectDetection | Y | Y | [config](https://github.com/open-mmlab/mmyolo/tree/main/configs/yolov6) |
|
||||
| YOLOX | ObjectDetection | Y | Y | [config](https://github.com/open-mmlab/mmyolo/tree/main/configs/yolox) |
|
||||
| RTMDet | ObjectDetection | Y | Y | [config](https://github.com/open-mmlab/mmyolo/tree/main/configs/rtmdet) |
|
||||
|
||||
Note: ncnn and other inference backends support are coming soon.
|
||||
|
||||
## How to Write Config for MMYOLO
|
||||
|
||||
All config files related to the deployment are located at [`configs/deploy`](../../../configs/deploy/).
|
||||
|
||||
You only need to change the relative data processing part in the model config file to support either static or dynamic input for your model. Besides, MMDeploy integrates the post-processing parts as customized ops, you can modify the strategy in `post_processing` parameter in `codebase_config`.
|
||||
|
||||
Here is the detail description:
|
||||
|
||||
```python
|
||||
codebase_config = dict(
|
||||
type='mmyolo',
|
||||
task='ObjectDetection',
|
||||
model_type='end2end',
|
||||
post_processing=dict(
|
||||
score_threshold=0.05,
|
||||
confidence_threshold=0.005,
|
||||
iou_threshold=0.5,
|
||||
max_output_boxes_per_class=200,
|
||||
pre_top_k=5000,
|
||||
keep_top_k=100,
|
||||
background_label_id=-1),
|
||||
module=['mmyolo.deploy'])
|
||||
```
|
||||
|
||||
- `score_threshold`: set the score threshold to filter candidate bboxes before `nms`
|
||||
- `confidence_threshold`: set the confidence threshold to filter candidate bboxes before `nms`
|
||||
- `iou_threshold`: set the `iou` threshold for removing duplicates in `nums`
|
||||
- `max_output_boxes_per_class`: set the maximum number of bboxes for each class
|
||||
- `pre_top_k`: set the number of fixedcandidate bboxes before `nms`, sorted by scores
|
||||
- `keep_top_k`: set the number of output candidate bboxs after `nms`
|
||||
- `background_label_id`: set to `-1` as MMYOLO has no background class information
|
||||
|
||||
### Configuration for Static Inputs
|
||||
|
||||
#### 1. Model Config
|
||||
|
||||
Taking `YOLOv5` of MMYOLO as an example, here are the details:
|
||||
|
||||
```python
|
||||
_base_ = '../../yolov5/yolov5_s-v61_syncbn_8xb16-300e_coco.py'
|
||||
|
||||
test_pipeline = [
|
||||
dict(type='LoadImageFromFile', file_client_args=_base_.file_client_args),
|
||||
dict(
|
||||
type='LetterResize',
|
||||
scale=_base_.img_scale,
|
||||
allow_scale_up=False,
|
||||
use_mini_pad=False,
|
||||
),
|
||||
dict(type='LoadAnnotations', with_bbox=True, _scope_='mmdet'),
|
||||
dict(
|
||||
type='mmdet.PackDetInputs',
|
||||
meta_keys=('img_id', 'img_path', 'ori_shape', 'img_shape',
|
||||
'scale_factor', 'pad_param'))
|
||||
]
|
||||
|
||||
test_dataloader = dict(
|
||||
dataset=dict(pipeline=test_pipeline, batch_shapes_cfg=None))
|
||||
```
|
||||
|
||||
`_base_ = '../../yolov5/yolov5_s-v61_syncbn_8xb16-300e_coco.py'` inherits the model config in the training stage.
|
||||
|
||||
`test_pipeline` adds the data processing piple for the deployment, `LetterResize` controls the size of the input images and the input for the converted model
|
||||
|
||||
`test_dataloader` adds the dataloader config for the deployment, `batch_shapes_cfg` decides whether to use the `batch_shapes` strategy. More details can be found at [yolov5 configs](../user_guides/config.md)
|
||||
|
||||
#### 2. Deployment Config
|
||||
|
||||
Here we still use the `YOLOv5` in MMYOLO as the example. We can use [`detection_onnxruntime_static.py`](https://github.com/open-mmlab/mmyolo/blob/main/configs/deploy/detection_onnxruntime_static.py) as the config to deploy \`YOLOv5\` to \`ONNXRuntim\` with static inputs.
|
||||
|
||||
```python
|
||||
_base_ = ['./base_static.py']
|
||||
codebase_config = dict(
|
||||
type='mmyolo',
|
||||
task='ObjectDetection',
|
||||
model_type='end2end',
|
||||
post_processing=dict(
|
||||
score_threshold=0.05,
|
||||
confidence_threshold=0.005,
|
||||
iou_threshold=0.5,
|
||||
max_output_boxes_per_class=200,
|
||||
pre_top_k=5000,
|
||||
keep_top_k=100,
|
||||
background_label_id=-1),
|
||||
module=['mmyolo.deploy'])
|
||||
backend_config = dict(type='onnxruntime')
|
||||
```
|
||||
|
||||
`backend_config` indicates the deployment backend with `type='onnxruntime'`, other information can be referred from the third section.
|
||||
|
||||
To deploy the `YOLOv5` to `TensorRT`, please refer to the [`detection_tensorrt_static-640x640.py`](https://github.com/open-mmlab/mmyolo/blob/main/configs/deploy/detection_tensorrt_static-640x640.py) as follows.
|
||||
|
||||
```python
|
||||
_base_ = ['./base_static.py']
|
||||
onnx_config = dict(input_shape=(640, 640))
|
||||
backend_config = dict(
|
||||
type='tensorrt',
|
||||
common_config=dict(fp16_mode=False, max_workspace_size=1 << 30),
|
||||
model_inputs=[
|
||||
dict(
|
||||
input_shapes=dict(
|
||||
input=dict(
|
||||
min_shape=[1, 3, 640, 640],
|
||||
opt_shape=[1, 3, 640, 640],
|
||||
max_shape=[1, 3, 640, 640])))
|
||||
])
|
||||
use_efficientnms = False
|
||||
```
|
||||
|
||||
`backend_config` indices the backend with `type=‘tensorrt’`.
|
||||
|
||||
Different from `ONNXRuntime` deployment configuration, `TensorRT` needs to specify the input image size and the parameters required to build the engine file, including:
|
||||
|
||||
- `onnx_config` specifies the input shape as `input_shape=(640, 640)`
|
||||
- `fp16_mode=False` and `max_workspace_size=1 << 30` in `backend_config['common_config']` indicates whether to build the engine in the parameter format of `fp16`, and the maximum video memory for the current `gpu` device, respectively. The unit is in `GB`. For detailed configuration of `fp16`, please refer to the [`detection_tensorrt-fp16_static-640x640.py`](https://github.com/open-mmlab/mmyolo/blob/main/configs/deploy/detection_tensorrt-fp16_static-640x640.py)
|
||||
- The `min_shape`/`opt_shape`/`max_shape` in `backend_config['model_inputs']['input_shapes']['input']` should remain the same under static input, the default is `[1, 3, 640, 640]`.
|
||||
|
||||
`use_efficientnms` is a new configuration introduced by the `MMYOLO` series, indicating whether to enable `Efficient NMS Plugin` to replace `TRTBatchedNMS plugin` in `MMDeploy` when exporting `onnx`.
|
||||
|
||||
You can refer to the official [efficient NMS plugins](https://github.com/NVIDIA/TensorRT/blob/main/plugin/efficientNMSPlugin/README.md) by `TensorRT` for more details.
|
||||
|
||||
Note: this out-of-box feature is **only available in TensorRT>=8.0**, no need to compile it by yourself.
|
||||
|
||||
### Configuration for Dynamic Inputs
|
||||
|
||||
#### 1. Model Config
|
||||
|
||||
When you deploy a dynamic input model, you don't need to modify any model configuration files but the deployment configuration files.
|
||||
|
||||
#### 2. Deployment Config
|
||||
|
||||
To deploy the `YOLOv5` in MMYOLO to `ONNXRuntime`, please refer to the [`detection_onnxruntime_dynamic.py`](https://github.com/open-mmlab/mmyolo/blob/main/configs/deploy/detection_onnxruntime_dynamic.py).
|
||||
|
||||
```python
|
||||
_base_ = ['./base_dynamic.py']
|
||||
codebase_config = dict(
|
||||
type='mmyolo',
|
||||
task='ObjectDetection',
|
||||
model_type='end2end',
|
||||
post_processing=dict(
|
||||
score_threshold=0.05,
|
||||
confidence_threshold=0.005,
|
||||
iou_threshold=0.5,
|
||||
max_output_boxes_per_class=200,
|
||||
pre_top_k=5000,
|
||||
keep_top_k=100,
|
||||
background_label_id=-1),
|
||||
module=['mmyolo.deploy'])
|
||||
backend_config = dict(type='onnxruntime')
|
||||
```
|
||||
|
||||
`backend_config` indicates the backend with `type='onnxruntime'`. Other parameters stay the same as the static input section.
|
||||
|
||||
To deploy the `YOLOv5` to `TensorRT`, please refer to the [`detection_tensorrt_dynamic-192x192-960x960.py`](https://github.com/open-mmlab/mmyolo/blob/main/configs/deploy/detection_tensorrt_dynamic-192x192-960x960.py).
|
||||
|
||||
```python
|
||||
_base_ = ['./base_dynamic.py']
|
||||
backend_config = dict(
|
||||
type='tensorrt',
|
||||
common_config=dict(fp16_mode=False, max_workspace_size=1 << 30),
|
||||
model_inputs=[
|
||||
dict(
|
||||
input_shapes=dict(
|
||||
input=dict(
|
||||
min_shape=[1, 3, 192, 192],
|
||||
opt_shape=[1, 3, 640, 640],
|
||||
max_shape=[1, 3, 960, 960])))
|
||||
])
|
||||
use_efficientnms = False
|
||||
```
|
||||
|
||||
`backend_config` indicates the backend with `type='tensorrt'`. Since the dynamic and static inputs are different in `TensorRT`, please check the details at [TensorRT dynamic input official introduction](https://docs.nvidia.com/deeplearning/tensorrt/archives/tensorrt-843/developer-guide/index.html#work_dynamic_shapes).
|
||||
|
||||
`TensorRT` deployment requires you to specify `min_shape`, `opt_shape` , and `max_shape`. `TensorRT` limits the size of the input image between `min_shape` and `max_shape`.
|
||||
|
||||
`min_shape` is the minimum size of the input image. `opt_shape` is the common size of the input image, inference performance is best under this size. `max_shape` is the maximum size of the input image.
|
||||
|
||||
`use_efficientnms` configuration is the same as the `TensorRT` static input configuration in the previous section.
|
||||
|
||||
### INT8 Quantization Support
|
||||
|
||||
Note: Int8 quantization support will soon be released.
|
||||
|
||||
## How to Convert Model
|
||||
|
||||
### Usage
|
||||
|
||||
Set the root directory of `MMDeploy` as an env parameter `MMDEPLOY_DIR` using `export MMDEPLOY_DIR=/the/root/path/of/MMDeploy` command.
|
||||
|
||||
```shell
|
||||
python3 ${MMDEPLOY_DIR}/tools/deploy.py \
|
||||
${DEPLOY_CFG_PATH} \
|
||||
${MODEL_CFG_PATH} \
|
||||
${MODEL_CHECKPOINT_PATH} \
|
||||
${INPUT_IMG} \
|
||||
--test-img ${TEST_IMG} \
|
||||
--work-dir ${WORK_DIR} \
|
||||
--calib-dataset-cfg ${CALIB_DATA_CFG} \
|
||||
--device ${DEVICE} \
|
||||
--log-level INFO \
|
||||
--show \
|
||||
--dump-info
|
||||
```
|
||||
|
||||
### Parameter Description
|
||||
|
||||
- `deploy_cfg`: set the deployment config path of MMDeploy for the model, including the type of inference framework, whether quantize, whether the input shape is dynamic, etc. There may be a reference relationship between configuration files, e.g. `configs/deploy/detection_onnxruntime_static.py`
|
||||
- `model_cfg`: set the MMYOLO model config path, e.g. `configs/deploy/model/yolov5_s-deploy.py`, regardless of the path to MMDeploy
|
||||
- `checkpoint`: set the torch model path. It can start with `http/https`, more details are available in `mmengine.fileio` apis
|
||||
- `img`: set the path to the image or point cloud file used for testing during model conversion
|
||||
- `--test-img`: set the image file that used to test model. If not specified, it will be set to `None`
|
||||
- `--work-dir`: set the work directory that used to save logs and models
|
||||
- `--calib-dataset-cfg`: use for calibration only for INT8 mode. If not specified, it will be set to None and use “val” dataset in model config for calibration
|
||||
- `--device`: set the device used for model conversion. The default is `cpu`, for TensorRT used `cuda:0`
|
||||
- `--log-level`: set log level which in `'CRITICAL', 'FATAL', 'ERROR', 'WARN', 'WARNING', 'INFO', 'DEBUG', 'NOTSET'`. If not specified, it will be set to `INFO`
|
||||
- `--show`: show the result on screen or not
|
||||
- `--dump-info`: output SDK information or not
|
||||
|
||||
## How to Evaluate Model
|
||||
|
||||
### Usage
|
||||
|
||||
After the model is converted to your backend, you can use `${MMDEPLOY_DIR}/tools/test.py` to evaluate the performance.
|
||||
|
||||
```shell
|
||||
python3 ${MMDEPLOY_DIR}/tools/test.py \
|
||||
${DEPLOY_CFG} \
|
||||
${MODEL_CFG} \
|
||||
--model ${BACKEND_MODEL_FILES} \
|
||||
[--out ${OUTPUT_PKL_FILE}] \
|
||||
[--format-only] \
|
||||
[--metrics ${METRICS}] \
|
||||
[--show] \
|
||||
[--show-dir ${OUTPUT_IMAGE_DIR}] \
|
||||
[--show-score-thr ${SHOW_SCORE_THR}] \
|
||||
--device ${DEVICE} \
|
||||
[--cfg-options ${CFG_OPTIONS}] \
|
||||
[--metric-options ${METRIC_OPTIONS}]
|
||||
[--log2file work_dirs/output.txt]
|
||||
[--batch-size ${BATCH_SIZE}]
|
||||
[--speed-test] \
|
||||
[--warmup ${WARM_UP}] \
|
||||
[--log-interval ${LOG_INTERVERL}]
|
||||
```
|
||||
|
||||
### Parameter Description
|
||||
|
||||
- `deploy_cfg`: set the deployment config file path
|
||||
- `model_cfg`: set the MMYOLO model config file path
|
||||
- `--model`: set the converted model. For example, if we exported a TensorRT model, we need to pass in the file path with the suffix ".engine"
|
||||
- `--out`: save the output result in pickle format, use only when you need it
|
||||
- `--format-only`: format the output without evaluating it. It is useful when you want to format the result into a specific format and submit it to a test server
|
||||
- `--metrics`: use the specific metric supported in MMYOLO to evaluate, such as "proposal" in COCO format data.
|
||||
- `--show`: show the evaluation result on screen or not
|
||||
- `--show-dir`: save the evaluation result to this directory, valid only when specified
|
||||
- `--show-score-thr`: show the threshold for the detected bboxes or not
|
||||
- `--device`: indicate the device to run the model. Note that some backends limit the running devices. For example, TensorRT must run on CUDA
|
||||
- `--cfg-options`: pass in additional configs, which will override the current deployment configs
|
||||
- `--metric-options`: add custom options for metrics. The key-value pair format in xxx=yyy will be the kwargs of the dataset.evaluate() method
|
||||
- `--log2file`: save the evaluation results (with the speed) to a file
|
||||
- `--batch-size`: set the batch size for inference, which will override the `samples_per_gpu` in data config. The default value is `1`, however, not every model supports `batch_size > 1`
|
||||
- `--speed-test`: test the inference speed or not
|
||||
- `--warmup`: warm up before speed test or not, works only when `speed-test` is specified
|
||||
- `--log-interval`: set the interval between each log, works only when `speed-test` is specified
|
||||
|
||||
Note: other parameters in `${MMDEPLOY_DIR}/tools/test.py` are used for speed test, they will not affect the evaluation results.
|
||||
|
|
|
@ -1 +1,432 @@
|
|||
# YOLOv5 Deployment
|
||||
|
||||
Please check the [basic_deployment_guide](basic_deployment_guide.md) to get familiar with the configurations.
|
||||
|
||||
## Model Training and Validation
|
||||
|
||||
The details of training and validation can be found at [yolov5_tutorial](../user_guides/yolov5_tutorial.md).
|
||||
|
||||
## MMDeploy Environment Setup
|
||||
|
||||
Please check the installation document of `MMDeploy` at [build_from_source](https://github.com/open-mmlab/mmdeploy/blob/dev-1.x/docs/en/01-how-to-build/build_from_source.md). Please build both `MMDeploy` and the customized Ops to your specific platform.
|
||||
|
||||
Note: please check at `MMDeploy` [FAQ](https://github.com/open-mmlab/mmdeploy/blob/dev-1.x/docs/en/faq.md) or create new issues in `MMDeploy` when you come across any problems.
|
||||
|
||||
## How to Prepare Configuration File
|
||||
|
||||
This deployment guide uses the `YOLOv5` model trained on `COCO` dataset in MMYOLO to illustrate the whole process, including both static and dynamic inputs and different procedures for `TensorRT` and `ONNXRuntime`.
|
||||
|
||||
### For Static Input
|
||||
|
||||
#### 1. Model Config
|
||||
|
||||
To deploy the model with static inputs, you need to ensure that the model inputs are in fixed size, e.g. the input size is set to `640x640` while uploading data in the test pipeline and test dataloader.
|
||||
|
||||
Here is a example in [`yolov5_s-static.py`](https://github.com/open-mmlab/mmyolo/tree/main/configs/deploy/model/yolov5_s-static.py)
|
||||
|
||||
```python
|
||||
_base_ = '../../yolov5/yolov5_s-v61_syncbn_8xb16-300e_coco.py'
|
||||
|
||||
test_pipeline = [
|
||||
dict(type='LoadImageFromFile', file_client_args=_base_.file_client_args),
|
||||
dict(
|
||||
type='LetterResize',
|
||||
scale=_base_.img_scale,
|
||||
allow_scale_up=False,
|
||||
use_mini_pad=False,
|
||||
),
|
||||
dict(type='LoadAnnotations', with_bbox=True, _scope_='mmdet'),
|
||||
dict(
|
||||
type='mmdet.PackDetInputs',
|
||||
meta_keys=('img_id', 'img_path', 'ori_shape', 'img_shape',
|
||||
'scale_factor', 'pad_param'))
|
||||
]
|
||||
|
||||
test_dataloader = dict(
|
||||
dataset=dict(pipeline=test_pipeline, batch_shapes_cfg=None))
|
||||
```
|
||||
|
||||
As the `YOLOv5` will turn on `allow_scale_up` and `use_mini_pad` during the test to change the size of the input image in order to achieve higher accuracy. However, it will cause the input size mismatch problem when deploying in the static input model.
|
||||
|
||||
Compared with the original configuration file, this configuration has been modified as follows:
|
||||
|
||||
- turn off the settings related to reshaping the image in `test_pipeline`, e.g. setting `allow_scale_up=False` and `use_mini_pad=False` in `LetterResize`
|
||||
- turn off the `batch_shapes` in `test_dataloader` as `batch_shapes_cfg=None`.
|
||||
|
||||
#### 2. Deployment Cofnig
|
||||
|
||||
To deploy the model to `ONNXRuntime`, please refer to the [`detection_onnxruntime_static.py`](https://github.com/open-mmlab/mmyolo/tree/main/configs/deploy/detection_onnxruntime_static.py) as follows:
|
||||
|
||||
```python
|
||||
_base_ = ['./base_static.py']
|
||||
codebase_config = dict(
|
||||
type='mmyolo',
|
||||
task='ObjectDetection',
|
||||
model_type='end2end',
|
||||
post_processing=dict(
|
||||
score_threshold=0.05,
|
||||
confidence_threshold=0.005,
|
||||
iou_threshold=0.5,
|
||||
max_output_boxes_per_class=200,
|
||||
pre_top_k=5000,
|
||||
keep_top_k=100,
|
||||
background_label_id=-1),
|
||||
module=['mmyolo.deploy'])
|
||||
backend_config = dict(type='onnxruntime')
|
||||
```
|
||||
|
||||
The `post_processing` in the default configuration aligns the accuracy of the current model with the trained `pytorch` model. If you need to modify the relevant parameters, you can refer to the detailed introduction of [dasic_deployment_guide](basic_deployment_guide.md).
|
||||
|
||||
To deploy the model to `TensorRT`, please refer to the [`detection_tensorrt_static-640x640.py`](https://github.com/open-mmlab/mmyolo/tree/main/configs/deploy/detection_tensorrt_static-640x640.p).
|
||||
|
||||
```python
|
||||
_base_ = ['./base_static.py']
|
||||
onnx_config = dict(input_shape=(640, 640))
|
||||
backend_config = dict(
|
||||
type='tensorrt',
|
||||
common_config=dict(fp16_mode=False, max_workspace_size=1 << 30),
|
||||
model_inputs=[
|
||||
dict(
|
||||
input_shapes=dict(
|
||||
input=dict(
|
||||
min_shape=[1, 3, 640, 640],
|
||||
opt_shape=[1, 3, 640, 640],
|
||||
max_shape=[1, 3, 640, 640])))
|
||||
])
|
||||
use_efficientnms = False
|
||||
```
|
||||
|
||||
In this guide, we use the default settings such as `input_shape=(640, 640)` and `fp16_mode=False` to build in network in `fp32` mode. Moreover, we set `max_workspace_size=1 << 30` for the gpu memory which allows `TensorRT` to build the engine with maximum `1GB` memory.
|
||||
|
||||
### For Dynamic Input
|
||||
|
||||
#### 1. Model Confige
|
||||
|
||||
As `TensorRT` limits the minimum and maximum input size, we can use any size for the inputs when deploy the model in dynamic mode. In this way, we can keep the default settings in [`yolov5_s-v61_syncbn_8xb16-300e_coco.py`](https://github.com/open-mmlab/mmyolo/tree/main/configs/yolov5/yolov5_s-v61_syncbn_8xb16-300e_coco.py). The data processing and dataloader parts are as follows.
|
||||
|
||||
```python
|
||||
batch_shapes_cfg = dict(
|
||||
type='BatchShapePolicy',
|
||||
batch_size=val_batch_size_per_gpu,
|
||||
img_size=img_scale[0],
|
||||
size_divisor=32,
|
||||
extra_pad_ratio=0.5)
|
||||
|
||||
test_pipeline = [
|
||||
dict(type='LoadImageFromFile', file_client_args=_base_.file_client_args),
|
||||
dict(type='YOLOv5KeepRatioResize', scale=img_scale),
|
||||
dict(
|
||||
type='LetterResize',
|
||||
scale=img_scale,
|
||||
allow_scale_up=False,
|
||||
pad_val=dict(img=114)),
|
||||
dict(type='LoadAnnotations', with_bbox=True, _scope_='mmdet'),
|
||||
dict(
|
||||
type='mmdet.PackDetInputs',
|
||||
meta_keys=('img_id', 'img_path', 'ori_shape', 'img_shape',
|
||||
'scale_factor', 'pad_param'))
|
||||
]
|
||||
|
||||
val_dataloader = dict(
|
||||
batch_size=val_batch_size_per_gpu,
|
||||
num_workers=val_num_workers,
|
||||
persistent_workers=persistent_workers,
|
||||
pin_memory=True,
|
||||
drop_last=False,
|
||||
sampler=dict(type='DefaultSampler', shuffle=False),
|
||||
dataset=dict(
|
||||
type=dataset_type,
|
||||
data_root=data_root,
|
||||
test_mode=True,
|
||||
data_prefix=dict(img='val2017/'),
|
||||
ann_file='annotations/instances_val2017.json',
|
||||
pipeline=test_pipeline,
|
||||
batch_shapes_cfg=batch_shapes_cfg))
|
||||
```
|
||||
|
||||
We use `allow_scale_up=False` to control when the input small images will be upsampled or not in the initialization of `LetterResize`. At the same time, the default `use_mini_pad=False` turns off the minimum padding strategy of the image, and `val_dataloader['dataset']` is passed in` batch_shapes_cfg=batch_shapes_cfg` to ensure that the minimum padding is performed according to the input size in `batch`. These configs will change the dimensions of the input image, so the converted model can support dynamic inputs according to the above dataset loader when testing.
|
||||
|
||||
#### 2. Deployment Cofnig
|
||||
|
||||
To deploy the model to `ONNXRuntime`, please refer to the [`detection_onnxruntime_dynamic.py`](https://github.com/open-mmlab/mmyolo/blob/main/configs/deploy/detection_onnxruntime_dynamic.py) for more details.
|
||||
|
||||
```python
|
||||
_base_ = ['./base_dynamic.py']
|
||||
codebase_config = dict(
|
||||
type='mmyolo',
|
||||
task='ObjectDetection',
|
||||
model_type='end2end',
|
||||
post_processing=dict(
|
||||
score_threshold=0.05,
|
||||
confidence_threshold=0.005,
|
||||
iou_threshold=0.5,
|
||||
max_output_boxes_per_class=200,
|
||||
pre_top_k=5000,
|
||||
keep_top_k=100,
|
||||
background_label_id=-1),
|
||||
module=['mmyolo.deploy'])
|
||||
backend_config = dict(type='onnxruntime')
|
||||
```
|
||||
|
||||
Differs from the static input config we introduced in previous section, dynamic input config additionally inherits the `dynamic_axes`. The rest of the configuration stays the same as the static inputs.
|
||||
|
||||
To deploy the model to `TensorRT`, please refer to the [`detection_tensorrt_dynamic-192x192-960x960.py`](https://github.com/open-mmlab/mmyolo/tree/main/configs/deploy/detection_tensorrt_dynamic-192x192-960x960.py) for more details.
|
||||
|
||||
```python
|
||||
_base_ = ['./base_dynamic.py']
|
||||
backend_config = dict(
|
||||
type='tensorrt',
|
||||
common_config=dict(fp16_mode=False, max_workspace_size=1 << 30),
|
||||
model_inputs=[
|
||||
dict(
|
||||
input_shapes=dict(
|
||||
input=dict(
|
||||
min_shape=[1, 3, 192, 192],
|
||||
opt_shape=[1, 3, 640, 640],
|
||||
max_shape=[1, 3, 960, 960])))
|
||||
])
|
||||
use_efficientnms = False
|
||||
```
|
||||
|
||||
In our example, the network is built in `fp32` mode as `fp16_mode=False`, and the maximum graphic memory is `1GB` for building the `TensorRT` engine as `max_workspace_size=1 << 30`.
|
||||
|
||||
At the same time, `min_shape=[1, 3, 192, 192]`, `opt_shape=[1, 3, 640, 640]`, and `max_shape=[1, 3, 960, 960]` in the default setting set the model with minimum input size to `192x192`, the maximum size to `960x960`, and the most common size to `640x640`.
|
||||
|
||||
When you deploy the model, it can adopt to the input image dimensions automatically.
|
||||
|
||||
## How to Convert Model
|
||||
|
||||
Note: The `MMDeploy` root directory used in this guide is `/home/openmmlab/dev/mmdeploy`, please modify it to your `MMDeploy` directory.
|
||||
|
||||
Use the following command to download the pretrained YOLOv5 weight and save it to your device:
|
||||
|
||||
```shell
|
||||
wget https://download.openmmlab.com/mmyolo/v0/yolov5/yolov5_s-v61_syncbn_fast_8xb16-300e_coco/yolov5_s-v61_syncbn_fast_8xb16-300e_coco_20220918_084700-86e02187.pth -O /home/openmmlab/dev/mmdeploy/yolov5s.pth
|
||||
```
|
||||
|
||||
Set the relevant env parameters using the following command as well:
|
||||
|
||||
```shell
|
||||
export MMDEPLOY_DIR=/home/openmmlab/dev/mmdeploy
|
||||
export PATH_TO_CHECKPOINTS=/home/openmmlab/dev/mmdeploy/yolov5s.pth
|
||||
```
|
||||
|
||||
### YOLOv5 Static Model Deployment
|
||||
|
||||
#### ONNXRuntime
|
||||
|
||||
```shell
|
||||
python3 ${MMDEPLOY_DIR}/tools/deploy.py \
|
||||
configs/deploy/detection_onnxruntime_static.py \
|
||||
configs/deploy/model/yolov5_s-static.py \
|
||||
${PATH_TO_CHECKPOINTS} \
|
||||
demo/demo.jpg \
|
||||
--work-dir work_dir \
|
||||
--show \
|
||||
--device cpu
|
||||
```
|
||||
|
||||
#### TensorRT
|
||||
|
||||
```shell
|
||||
python3 ${MMDEPLOY_DIR}/tools/deploy.py \
|
||||
configs/deploy/detection_tensorrt_static-640x640.py \
|
||||
configs/deploy/model/yolov5_s-static.py \
|
||||
${PATH_TO_CHECKPOINTS} \
|
||||
demo/demo.jpg \
|
||||
--work-dir work_dir \
|
||||
--show \
|
||||
--device cuda:0
|
||||
```
|
||||
|
||||
### YOLOv5 Dynamic Model Deployment
|
||||
|
||||
#### ONNXRuntime
|
||||
|
||||
```shell
|
||||
python3 ${MMDEPLOY_DIR}/tools/deploy.py \
|
||||
configs/deploy/detection_onnxruntime_dynamic.py \
|
||||
configs/yolov5/yolov5_s-v61_syncbn_8xb16-300e_coco.py \
|
||||
${PATH_TO_CHECKPOINTS} \
|
||||
demo/demo.jpg \
|
||||
--work-dir work_dir \
|
||||
--show \
|
||||
--device cpu
|
||||
```
|
||||
|
||||
#### TensorRT
|
||||
|
||||
```shell
|
||||
python3 ${MMDEPLOY_DIR}/tools/deploy.py \
|
||||
configs/deploy/detection_tensorrt_dynamic-192x192-960x960.py \
|
||||
configs/yolov5/yolov5_s-v61_syncbn_8xb16-300e_coco.py \
|
||||
${PATH_TO_CHECKPOINTS} \
|
||||
demo/demo.jpg \
|
||||
--work-dir work_dir \
|
||||
--show \
|
||||
--device cuda:0
|
||||
```
|
||||
|
||||
When convert the model using the above commands, you will find the following files under the `work_dir` folder:
|
||||
|
||||
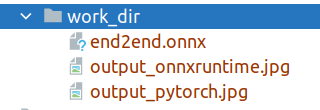
|
||||
|
||||
or
|
||||
|
||||
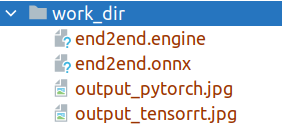
|
||||
|
||||
After exporting to `onnxruntime`, you will get three files as shown in Figure 1, where `end2end.onnx` represents the exported `onnxruntime` model.
|
||||
|
||||
After exporting to `TensorRT`, you will get the four files as shown in Figure 2, where `end2end.onnx` represents the exported intermediate model. `MMDeploy` uses this model to automatically continue to convert the `end2end.engine` model for `TensorRT `Deployment.
|
||||
|
||||
## How to Evaluate Model
|
||||
|
||||
After successfully convert the model, you can use `${MMDEPLOY_DIR}/tools/test.py` to evaluate the converted model. The following part shows how to evaluate the static models of `ONNXRuntime` and `TensorRT`. For dynamic model evaluation, please modify the configuration of the inputs.
|
||||
|
||||
#### ONNXRuntime
|
||||
|
||||
```shell
|
||||
python3 ${MMDEPLOY_DIR}/tools/test.py \
|
||||
configs/deploy/detection_onnxruntime_static.py \
|
||||
configs/deploy/model/yolov5_s-static.py \
|
||||
--model work_dir/end2end.onnx \
|
||||
--device cpu \
|
||||
--work-dir work_dir
|
||||
```
|
||||
|
||||
Once the process is done, you can get the output results as this:
|
||||
|
||||
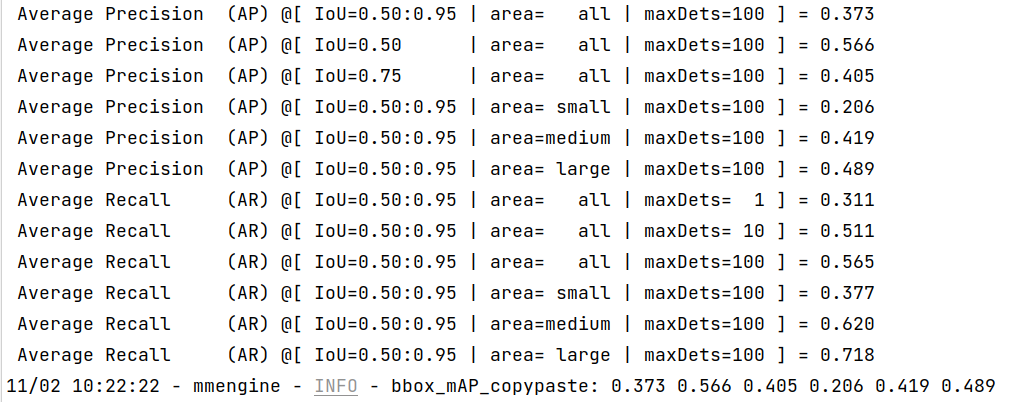
|
||||
|
||||
#### TensorRT
|
||||
|
||||
Note: `TensorRT` must run on `CUDA` devices!
|
||||
|
||||
```shell
|
||||
python3 ${MMDEPLOY_DIR}/tools/test.py \
|
||||
configs/deploy/detection_tensorrt_static-640x640.py \
|
||||
configs/deploy/model/yolov5_s-static.py \
|
||||
--model work_dir/end2end.engine \
|
||||
--device cuda:0 \
|
||||
--work-dir work_dir
|
||||
```
|
||||
|
||||
Once the process is done, you can get the output results as this:
|
||||
|
||||
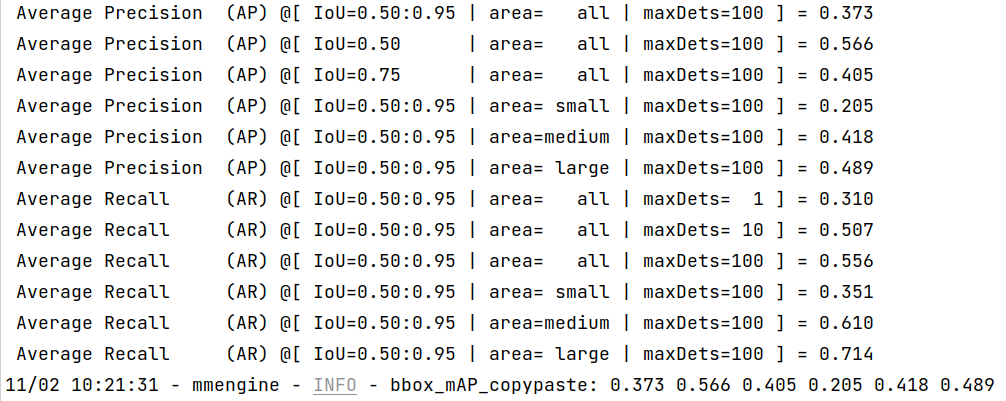
|
||||
|
||||
More useful evaluation tools will be released in the future.
|
||||
|
||||
# Deploy using Docker
|
||||
|
||||
`MMYOLO` provides a deployment [`Dockerfile`](https://github.com/open-mmlab/mmyolo/blob/main/docker/Dockerfile_deployment) for deployment purpose. Please make sure your local docker version is greater than `19.03`.
|
||||
|
||||
Note: users in mainland China can comment out the `Optional` part in the dockerfile for better experience.
|
||||
|
||||
```dockerfile
|
||||
# (Optional)
|
||||
RUN sed -i 's/http:\/\/archive.ubuntu.com\/ubuntu\//http:\/\/mirrors.aliyun.com\/ubuntu\//g' /etc/apt/sources.list && \
|
||||
pip config set global.index-url https://pypi.tuna.tsinghua.edu.cn/simple
|
||||
```
|
||||
|
||||
To build the docker image,
|
||||
|
||||
```bash
|
||||
# build an image with PyTorch 1.12, CUDA 11.6, TensorRT 8.2.4 ONNXRuntime 1.8.1
|
||||
docker build -f docker/Dockerfile_deployment -t mmyolo:v1 .
|
||||
```
|
||||
|
||||
To run the docker image,
|
||||
|
||||
```bash
|
||||
export DATA_DIR=/path/to/your/dataset
|
||||
docker run --gpus all --shm-size=8g -it --name mmyolo -v ${DATA_DIR}:/openmmlab/mmyolo/data/coco mmyolo:v1
|
||||
```
|
||||
|
||||
`DATA_DIR` is the path of your `COCO` dataset.
|
||||
|
||||
We provide a `script.sh` file for you which runs the whole pipeline. Create the script under `/openmmlab/mmyolo` directory in your docker container using the following content.
|
||||
|
||||
```bash
|
||||
#!/bin/bash
|
||||
wget -q https://download.openmmlab.com/mmyolo/v0/yolov5/yolov5_s-v61_syncbn_fast_8xb16-300e_coco/yolov5_s-v61_syncbn_fast_8xb16-300e_coco_20220918_084700-86e02187.pth \
|
||||
-O yolov5s.pth
|
||||
export MMDEPLOY_DIR=/openmmlab/mmdeploy
|
||||
export PATH_TO_CHECKPOINTS=/openmmlab/mmyolo/yolov5s.pth
|
||||
|
||||
python3 ${MMDEPLOY_DIR}/tools/deploy.py \
|
||||
configs/deploy/detection_tensorrt_static-640x640.py \
|
||||
configs/deploy/model/yolov5_s-static.py \
|
||||
${PATH_TO_CHECKPOINTS} \
|
||||
demo/demo.jpg \
|
||||
--work-dir work_dir_trt \
|
||||
--device cuda:0
|
||||
|
||||
python3 ${MMDEPLOY_DIR}/tools/test.py \
|
||||
configs/deploy/detection_tensorrt_static-640x640.py \
|
||||
configs/deploy/model/yolov5_s-static.py \
|
||||
--model work_dir_trt/end2end.engine \
|
||||
--device cuda:0 \
|
||||
--work-dir work_dir_trt
|
||||
|
||||
python3 ${MMDEPLOY_DIR}/tools/deploy.py \
|
||||
configs/deploy/detection_onnxruntime_static.py \
|
||||
configs/deploy/model/yolov5_s-static.py \
|
||||
${PATH_TO_CHECKPOINTS} \
|
||||
demo/demo.jpg \
|
||||
--work-dir work_dir_ort \
|
||||
--device cpu
|
||||
|
||||
python3 ${MMDEPLOY_DIR}/tools/test.py \
|
||||
configs/deploy/detection_onnxruntime_static.py \
|
||||
configs/deploy/model/yolov5_s-static.py \
|
||||
--model work_dir_ort/end2end.onnx \
|
||||
--device cpu \
|
||||
--work-dir work_dir_ort
|
||||
```
|
||||
|
||||
Then run the script under `/openmmlab/mmyolo`.
|
||||
|
||||
```bash
|
||||
sh script.sh
|
||||
```
|
||||
|
||||
This script automatically downloads the `YOLOv5` pretrained weights in `MMYOLO` and convert the model using `MMDeploy`. You will get the output result as follows.
|
||||
|
||||
- TensorRT:
|
||||
|
||||
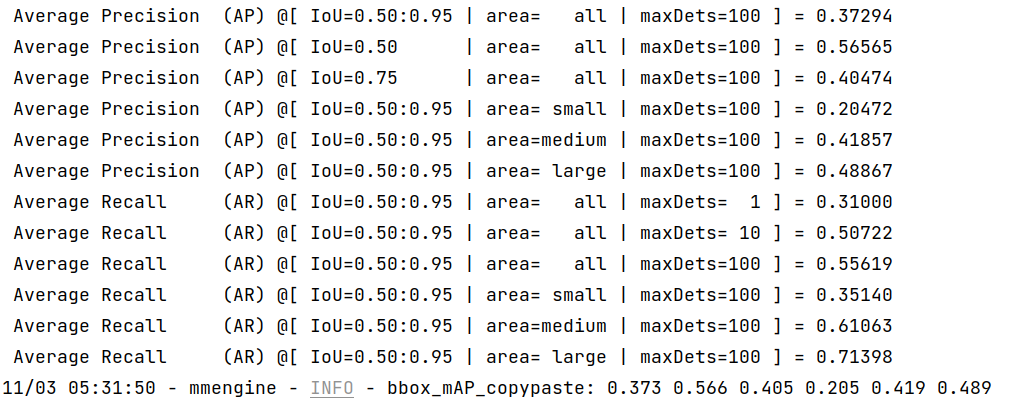
|
||||
|
||||
- ONNXRuntime:
|
||||
|
||||
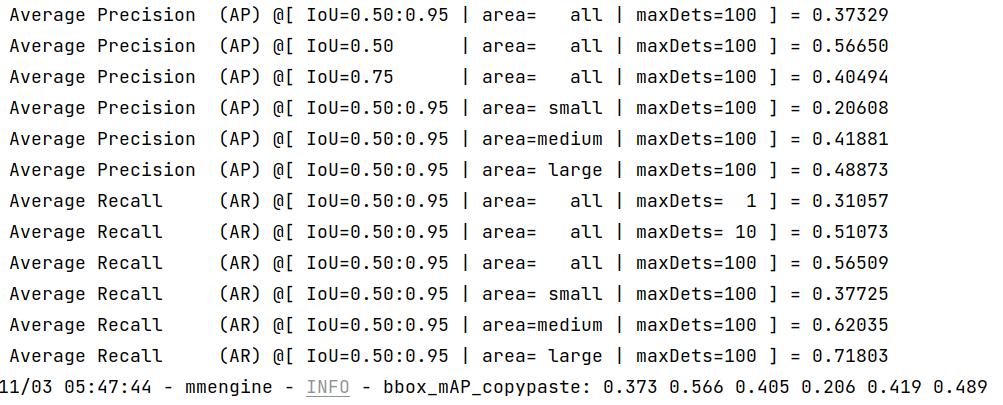
|
||||
|
||||
We can see from the above images that the accuracy of converted models shrink within 1% compared with the pytorch [MMYOLO-YOLOv5](https://github.com/open-mmlab/mmyolo/tree/main/configs/yolov5#results-and-models) models.
|
||||
|
||||
If you need to test the inference speed of the converted model, you can use the following commands.
|
||||
|
||||
- TensorRT
|
||||
|
||||
```shell
|
||||
python3 ${MMDEPLOY_DIR}/tools/profiler.py \
|
||||
configs/deploy/detection_tensorrt_static-640x640.py \
|
||||
configs/deploy/model/yolov5_s-static.py \
|
||||
data/coco/val2017 \
|
||||
--model work_dir_trt/end2end.engine \
|
||||
--device cuda:0
|
||||
```
|
||||
|
||||
- ONNXRuntime
|
||||
|
||||
```shell
|
||||
python3 ${MMDEPLOY_DIR}/tools/profiler.py \
|
||||
configs/deploy/detection_onnxruntime_static.py \
|
||||
configs/deploy/model/yolov5_s-static.py \
|
||||
data/coco/val2017 \
|
||||
--model work_dir_ort/end2end.onnx \
|
||||
--device cpu
|
||||
```
|
||||
|
||||
## Model Inference
|
||||
|
||||
TODO
|
||||
|
|
|
@ -21,7 +21,7 @@ ncnn 和其他后端的支持会在后续支持。
|
|||
|
||||
## MMYOLO 中部署相关配置说明
|
||||
|
||||
所有部署配置文件在 [`configs/deploy`](configs/deploy) 目录下。
|
||||
所有部署配置文件在 [`configs/deploy`](../../../configs/deploy/) 目录下。
|
||||
|
||||
您可以部署静态输入或者动态输入的模型,因此您需要修改模型配置文件中与此相关的数据处理流程。
|
||||
|
||||
|
@ -89,7 +89,7 @@ test_dataloader = dict(
|
|||
|
||||
以 `MMYOLO` 中的 `YOLOv5` 部署配置为例,下面是对配置文件参数说明介绍。
|
||||
|
||||
`ONNXRuntime` 部署 `YOLOv5` 可以使用 [`detection_onnxruntime_static.py`](configs/deploy/detection_onnxruntime_static.py) 配置。
|
||||
`ONNXRuntime` 部署 `YOLOv5` 可以使用 [`detection_onnxruntime_static.py`](https://github.com/open-mmlab/mmyolo/blob/main/configs/deploy/detection_onnxruntime_static.py) 配置。
|
||||
|
||||
```python
|
||||
_base_ = ['./base_static.py']
|
||||
|
@ -111,7 +111,7 @@ backend_config = dict(type='onnxruntime')
|
|||
|
||||
`backend_config` 中指定了部署后端 `type=‘onnxruntime’`,其他信息可参考第三小节。
|
||||
|
||||
`TensorRT` 部署 `YOLOv5` 可以使用 [`detection_tensorrt_static-640x640.py`](config/deploy/detection_tensorrt_static-640x640.py) 配置。
|
||||
`TensorRT` 部署 `YOLOv5` 可以使用 [`detection_tensorrt_static-640x640.py`](https://github.com/open-mmlab/mmyolo/blob/main/configs/deploy/detection_tensorrt_static-640x640.py) 配置。
|
||||
|
||||
```python
|
||||
_base_ = ['./base_static.py']
|
||||
|
@ -135,7 +135,7 @@ use_efficientnms = False
|
|||
与 `ONNXRuntime` 部署配置不同的是,`TensorRT` 需要指定输入图片尺寸和构建引擎文件需要的参数,包括:
|
||||
|
||||
- `onnx_config` 中指定 `input_shape=(640, 640)`
|
||||
- `backend_config['common_config']` 中 `fp16_mode=False` 和 `max_workspace_size=1 << 30`, `fp16_mode` 表示是否以 `fp16` 的参数格式构建引擎,`max_workspace_size` 表示当前 `gpu` 设备最大显存, 单位为 `GB`。`fp16` 的详细配置可以参考 [`detection_tensorrt-fp16_static-640x640.py`](configs/deploy/detection_tensorrt-fp16_static-640x640.py)
|
||||
- `backend_config['common_config']` 中包括 `fp16_mode=False` 和 `max_workspace_size=1 << 30`, `fp16_mode` 表示是否以 `fp16` 的参数格式构建引擎,`max_workspace_size` 表示当前 `gpu` 设备最大显存, 单位为 `GB`。`fp16` 的详细配置可以参考 [`detection_tensorrt-fp16_static-640x640.py`](https://github.com/open-mmlab/mmyolo/blob/main/configs/deploy/detection_tensorrt-fp16_static-640x640.py)
|
||||
- `backend_config['model_inputs']['input_shapes']['input']` 中 `min_shape` /`opt_shape`/`max_shape` 对应的值在静态输入下应该保持相同,即默认均为 `[1, 3, 640, 640]`。
|
||||
|
||||
`use_efficientnms` 是 `MMYOLO` 系列新引入的配置,表示在导出 `onnx` 时是否启用`Efficient NMS Plugin`来替换 `MMDeploy` 中的 `TRTBatchedNMS plugin` 。
|
||||
|
@ -148,11 +148,11 @@ use_efficientnms = False
|
|||
|
||||
#### (1) 模型配置文件介绍
|
||||
|
||||
当您部署静态输入模型时,您无需修改任何模型配置文件,仅需要修改部署配置文件即可。
|
||||
当您部署动态输入模型时,您无需修改任何模型配置文件,仅需要修改部署配置文件即可。
|
||||
|
||||
#### (2) 部署配置文件介绍
|
||||
|
||||
`ONNXRuntime` 部署 `YOLOv5` 可以使用 [`detection_onnxruntime_dynamic.py`](configs/deploy/detection_onnxruntime_dynamic.py) 配置。
|
||||
`ONNXRuntime` 部署 `YOLOv5` 可以使用 [`detection_onnxruntime_dynamic.py`](https://github.com/open-mmlab/mmyolo/blob/main/configs/deploy/detection_onnxruntime_dynamic.py) 配置。
|
||||
|
||||
```python
|
||||
_base_ = ['./base_dynamic.py']
|
||||
|
@ -174,7 +174,7 @@ backend_config = dict(type='onnxruntime')
|
|||
|
||||
`backend_config` 中指定了后端 `type='onnxruntime'`,其他配置与上一节在 ONNXRuntime 部署静态输入模型相同。
|
||||
|
||||
`TensorRT` 部署 `YOLOv5` 可以使用 [`detection_tensorrt_dynamic-192x192-960x960.py`](config/deploy/detection_tensorrt_dynamic-192x192-960x960.py) 配置。
|
||||
`TensorRT` 部署 `YOLOv5` 可以使用 [`detection_tensorrt_dynamic-192x192-960x960.py`](https://github.com/open-mmlab/mmyolo/blob/main/configs/deploy/detection_tensorrt_dynamic-192x192-960x960.py) 配置。
|
||||
|
||||
```python
|
||||
_base_ = ['./base_dynamic.py']
|
||||
|
@ -228,8 +228,8 @@ python3 ${MMDEPLOY_DIR}/tools/deploy.py \
|
|||
### 参数描述
|
||||
|
||||
- `deploy_cfg` : mmdeploy 针对此模型的部署配置,包含推理框架类型、是否量化、输入 shape 是否动态等。配置文件之间可能有引用关系,`configs/deploy/detection_onnxruntime_static.py` 是一个示例。
|
||||
- `model_cfg` : MMYOLO 算法库的模型配置,例如 `configs/deploy/model/yolov5_s-deploy.py`, 与 mmdeploy 的路径无关.
|
||||
- `checkpoint` : torch 模型路径。可以 http/https 开头,详见 `mmcv.FileClient` 的实现。.
|
||||
- `model_cfg` : MMYOLO 算法库的模型配置,例如 `configs/deploy/model/yolov5_s-deploy.py`, 与 mmdeploy 的路径无关。
|
||||
- `checkpoint` : torch 模型路径。可以 http/https 开头,详见 `mmengine.fileio` 的实现。
|
||||
- `img` : 模型转换时,用做测试的图像文件路径。
|
||||
- `--test-img` : 用于测试模型的图像文件路径。默认设置成`None`。
|
||||
- `--work-dir` : 工作目录,用来保存日志和模型文件。
|
||||
|
|
|
@ -1,10 +1,10 @@
|
|||
# YOLOv5 部署全流程说明
|
||||
|
||||
请先参考 [`部署必备指南`](./部署必备指南.md) 了解部署配置文件等相关信息。
|
||||
请先参考 [`部署必备指南`](basic_deployment_guide.md) 了解部署配置文件等相关信息。
|
||||
|
||||
## 模型训练和测试
|
||||
|
||||
模型训练和测试请参考 [`YOLOv5 从入门到部署全流程`](docs/zh_cn/user_guides/yolov5_tutorial.md) 。
|
||||
模型训练和测试请参考 [YOLOv5 从入门到部署全流程](../user_guides/yolov5_tutorial.md) 。
|
||||
|
||||
## 准备 MMDeploy 运行环境
|
||||
|
||||
|
@ -22,7 +22,7 @@
|
|||
|
||||
当您需要部署静态输入模型时,您应该确保模型的输入尺寸是固定的,比如在测试流程或测试数据集加载时输入尺寸为 `640x640`。
|
||||
|
||||
您可以查看 [`yolov5_s-deploy.py`](configs/deploy/model/yolov5_s-deploy.py) 中测试流程或测试数据集加载部分,如下所示:
|
||||
您可以查看 [`yolov5_s-static.py`](https://github.com/open-mmlab/mmyolo/tree/main/configs/deploy/model/yolov5_s-static.py) 中测试流程或测试数据集加载部分,如下所示:
|
||||
|
||||
```python
|
||||
_base_ = '../../yolov5/yolov5_s-v61_syncbn_8xb16-300e_coco.py'
|
||||
|
@ -55,7 +55,7 @@ test_dataloader = dict(
|
|||
|
||||
#### (2) 部署配置文件
|
||||
|
||||
当您部署在 `ONNXRuntime` 时,您可以查看 [`detection_onnxruntime_static.py`](configs/deploy/detection_onnxruntime_static.py) ,如下所示:
|
||||
当您部署在 `ONNXRuntime` 时,您可以查看 [`detection_onnxruntime_static.py`](https://github.com/open-mmlab/mmyolo/tree/main/configs/deploy/detection_onnxruntime_static.py) ,如下所示:
|
||||
|
||||
```python
|
||||
_base_ = ['./base_static.py']
|
||||
|
@ -75,9 +75,9 @@ codebase_config = dict(
|
|||
backend_config = dict(type='onnxruntime')
|
||||
```
|
||||
|
||||
默认配置中的 `post_processing` 后处理参数是当前模型与 `pytorch` 模型精度对齐的配置,若您需要修改相关参数,可以参考 [`部署必备指南`](./部署必备指南.md) 的详细介绍。
|
||||
默认配置中的 `post_processing` 后处理参数是当前模型与 `pytorch` 模型精度对齐的配置,若您需要修改相关参数,可以参考 [`部署必备指南`](basic_deployment_guide.md) 的详细介绍。
|
||||
|
||||
当您部署在 `TensorRT` 时,您可以查看 [`detection_tensorrt_static-640x640.py`](config/deploy/detection_tensorrt_static-640x640.py) ,如下所示:
|
||||
当您部署在 `TensorRT` 时,您可以查看 [`detection_tensorrt_static-640x640.py`](https://github.com/open-mmlab/mmyolo/tree/main/configs/deploy/detection_tensorrt_static-640x640.py) ,如下所示:
|
||||
|
||||
```python
|
||||
_base_ = ['./base_static.py']
|
||||
|
@ -102,7 +102,7 @@ use_efficientnms = False
|
|||
|
||||
#### (1) 模型配置文件
|
||||
|
||||
当您需要部署动态输入模型时,模型的输入可以为任意尺寸(`TensorRT` 会限制最小和最大输入尺寸),因此使用默认的 [`yolov5_s-v61_syncbn_8xb16-300e_coco.py`](configs/yolov5/yolov5_s-v61_syncbn_8xb16-300e_coco.py) 模型配置文件即可,其中数据处理和数据集加载器部分如下所示:
|
||||
当您需要部署动态输入模型时,模型的输入可以为任意尺寸(`TensorRT` 会限制最小和最大输入尺寸),因此使用默认的 [`yolov5_s-v61_syncbn_8xb16-300e_coco.py`](https://github.com/open-mmlab/mmyolo/tree/main/configs/yolov5/yolov5_s-v61_syncbn_8xb16-300e_coco.py) 模型配置文件即可,其中数据处理和数据集加载器部分如下所示:
|
||||
|
||||
```python
|
||||
batch_shapes_cfg = dict(
|
||||
|
@ -148,7 +148,7 @@ val_dataloader = dict(
|
|||
|
||||
#### (2) 部署配置文件
|
||||
|
||||
当您部署在 `ONNXRuntime` 时,您可以查看 [`detection_onnxruntime_dynamic.py`](configs/deploy/detection_onnxruntime_dynamic.py) ,如下所示:
|
||||
当您部署在 `ONNXRuntime` 时,您可以查看 [`detection_onnxruntime_dynamic.py`](https://github.com/open-mmlab/mmyolo/blob/main/configs/deploy/detection_onnxruntime_dynamic.py) ,如下所示:
|
||||
|
||||
```python
|
||||
_base_ = ['./base_dynamic.py']
|
||||
|
@ -170,7 +170,7 @@ backend_config = dict(type='onnxruntime')
|
|||
|
||||
与静态输入配置仅有 `_base_ = ['./base_dynamic.py']` 不同,动态输入会额外继承 `dynamic_axes` 属性。其他配置与静态输入配置相同。
|
||||
|
||||
当您部署在 `TensorRT` 时,您可以查看 [`detection_tensorrt_dynamic-192x192-960x960.py`](config/deploy/detection_tensorrt_dynamic-192x192-960x960.py) ,如下所示:
|
||||
当您部署在 `TensorRT` 时,您可以查看 [`detection_tensorrt_dynamic-192x192-960x960.py`](https://github.com/open-mmlab/mmyolo/tree/main/configs/deploy/detection_tensorrt_dynamic-192x192-960x960.py) ,如下所示:
|
||||
|
||||
```python
|
||||
_base_ = ['./base_dynamic.py']
|
||||
|
@ -227,7 +227,7 @@ python3 ${MMDEPLOY_DIR}/tools/deploy.py \
|
|||
|
||||
#### TensorRT
|
||||
|
||||
```bash
|
||||
```shell
|
||||
python3 ${MMDEPLOY_DIR}/tools/deploy.py \
|
||||
configs/deploy/detection_tensorrt_static-640x640.py \
|
||||
configs/deploy/model/yolov5_s-static.py \
|
||||
|
@ -318,9 +318,9 @@ python3 ${MMDEPLOY_DIR}/tools/test.py \
|
|||
|
||||
# 使用 Docker 部署测试
|
||||
|
||||
`MMYOLO` 提供了一个 [`Dockerfile`](docker/Dockerfile_deployment) 用于构建镜像。请确保您的 `docker` 版本大于等于 `19.03`。
|
||||
`MMYOLO` 提供了一个 [`Dockerfile`](https://github.com/open-mmlab/mmyolo/blob/main/docker/Dockerfile_deployment) 用于构建镜像。请确保您的 `docker` 版本大于等于 `19.03`。
|
||||
|
||||
温馨提示;国内用户建议取消掉 [`Dockerfile`](docker/Dockerfile_deployment) 里面 `Optional` 后两行的注释,可以获得火箭一般的下载提速:
|
||||
温馨提示;国内用户建议取消掉 [`Dockerfile`](https://github.com/open-mmlab/mmyolo/blob/main/docker/Dockerfile_deployment) 里面 `Optional` 后两行的注释,可以获得火箭一般的下载提速:
|
||||
|
||||
```dockerfile
|
||||
# (Optional)
|
||||
|
@ -330,14 +330,14 @@ RUN sed -i 's/http:\/\/archive.ubuntu.com\/ubuntu\//http:\/\/mirrors.aliyun.com\
|
|||
|
||||
构建命令:
|
||||
|
||||
```shell
|
||||
```bash
|
||||
# build an image with PyTorch 1.12, CUDA 11.6, TensorRT 8.2.4 ONNXRuntime 1.8.1
|
||||
docker build -f docker/Dockerfile_deployment -t mmyolo:v1 .
|
||||
```
|
||||
|
||||
用以下命令运行 Docker 镜像:
|
||||
|
||||
```shell
|
||||
```bash
|
||||
export DATA_DIR=/path/to/your/dataset
|
||||
docker run --gpus all --shm-size=8g -it --name mmyolo -v ${DATA_DIR}:/openmmlab/mmyolo/data/coco mmyolo:v1
|
||||
```
|
||||
|
@ -386,7 +386,7 @@ python3 ${MMDEPLOY_DIR}/tools/test.py \
|
|||
|
||||
在 `/openmmlab/mmyolo` 下运行:
|
||||
|
||||
```shell
|
||||
```bash
|
||||
sh script.sh
|
||||
```
|
||||
|
||||
|
@ -400,7 +400,7 @@ sh script.sh
|
|||
|
||||
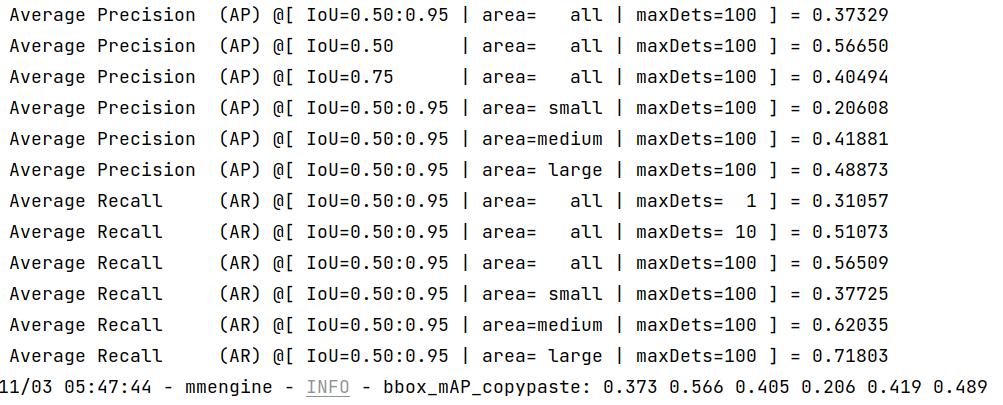
|
||||
|
||||
可以看到,经过 `MMDeploy` 部署的模型与 [MMYOLO-YOLOv5](`https://github.com/open-mmlab/mmyolo/tree/main/configs/yolov5`) 的 mAP-37.7 差距在 1% 以内。
|
||||
可以看到,经过 `MMDeploy` 部署的模型与 [MMYOLO-YOLOv5](https://github.com/open-mmlab/mmyolo/tree/main/configs/yolov5#results-and-models) 的 mAP-37.7 差距在 1% 以内。
|
||||
|
||||
如果您需要测试您的模型推理速度,可以使用以下命令:
|
||||
|
||||
|
|
Loading…
Reference in New Issue