mirror of https://github.com/open-mmlab/mmyolo.git
[WIP][Doc] Add pipeline doc of rtmdet_description.md (#102)
* [Feature] Make docker image smaller (#67) * [Feature] Make docker image smaller * Improve doc * Improve doc * Improve doc * Improve doc * Use Compose directly in BaseMixImageTransform (#71) * Use 'Compose' directly Use 'Compose' directly * avoids some unnecessary calculations avoids some unnecessary calculations. * remove whitespace remove whitespace * add pipeline doc * update * update * update * update * update * update * update * update * update * update * update * update * update * update Co-authored-by: HinGwenWoong <peterhuang0323@qq.com> Co-authored-by: jason_w <wongzheng@126.com>pull/137/head
parent
fa487140b6
commit
7dfc474a28
|
@ -27,6 +27,124 @@ RTMDet 由 tiny/s/m/l/x 一系列不同大小的模型组成,为不同的应
|
|||
<img alt="RTMDet_精度图" src="https://user-images.githubusercontent.com/12907710/192182907-f9a671d6-89cb-4d73-abd8-c2b9dada3c66.png"/>
|
||||
</div>
|
||||
|
||||
## 数据增强模块
|
||||
|
||||
RTMDet 采用了多种数据增强的方式来增加模型的性能,主要包括单图数据增强:
|
||||
|
||||
- **RandomResize 随机尺度变换**
|
||||
- **RandomCrop 随机裁剪**
|
||||
- **HSVRandomAug 颜色空间增强**
|
||||
- **RandomFlip 随机水平翻转**
|
||||
|
||||
以及混合类数据增强:
|
||||
|
||||
- **Mosaic 马赛克**
|
||||
- **MixUp 图像混合**
|
||||
|
||||
数据增强流程如下:
|
||||
|
||||
<center>
|
||||
<img src="https://user-images.githubusercontent.com/33799979/192940322-3864fac4-cbbc-4f80-a8d3-0f84a2c40e27.png" width=800 />
|
||||
</center>
|
||||
|
||||
其中 RandomResize 这个在 大模型 M,L,X 和 小模型 s, tiny 上是不一样的,大模型由于参数较多,可以使用 large scale jitter 策略即参数为 (0.1,2.0),而小模型采用 stand scale jitter 策略即 (0.5, 2.0) 策略。
|
||||
MMDetection 开源库中已经对单图数据增强进行了封装,用户通过简单的修改配置即可使用库中提供的任何数据增强功能,且都是属于比较常规的数据增强,不需要特殊介绍。下面将具体介绍混合类数据增强的具体实现。
|
||||
|
||||
与 YOLOv5 不同的是,YOLOv5认为在 s 和 nano 模型上使用 MixUp 是过剩的,小模型不需要这么强的数据增强。而 RTMDet 在 s 和 tiny 上也使用了 MixUp,这是因为 RTMDet 在最后 20 epoch 会切换为正常的 aug, 并通过训练证明这个操作是有效的。 并且 RTMDet 为混合类数据增强引入了 Cache 方案,有效地减少了图像处理的时间,和引入了可调超参 max_cached_images ,当使用较小的 cache 时,其效果类似 repeated augmentation。具体介绍如下:
|
||||
|
||||
### 为图像混合数据增强引入Cache
|
||||
|
||||
Mosaic&MixUp 涉及到多张图片的混合,它们的耗时会是普通数据增强的K倍(K为混入图片的数量)。 如在YOLOv5中,每次做 Mosaic 时, 4张图片的信息都需要从硬盘中重新加载。 而 RTMDet 只需要重新载入当前的一张图片,其余参与混合增强的图片则从缓存队列中获取,通过牺牲一定内存空间的方式大幅提升了效率。 另外,通过调整 cache 的大小以及 pop 的方式,也可以调整增强的强度。
|
||||
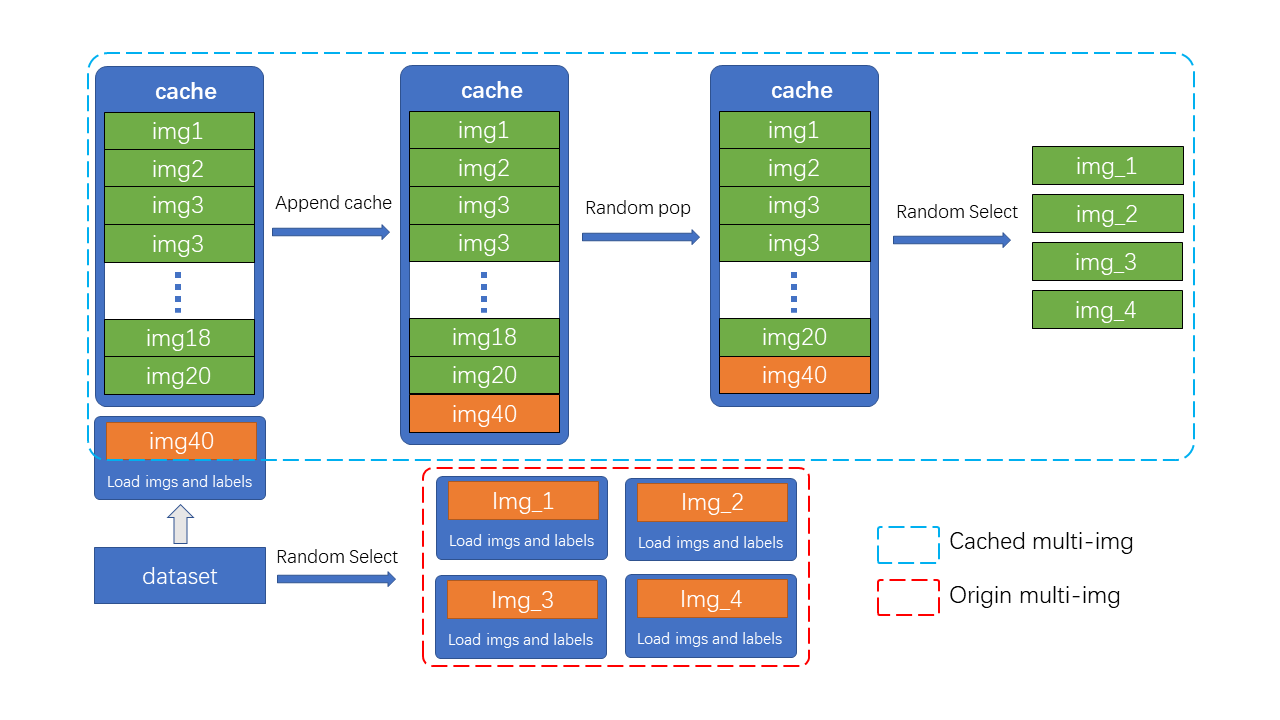
|
||||
如图所示,cache 队列中预先储存了N张已加载的图像与标签数据,每一个训练 step 中只需加载一张新的图片及其标签数据并更新到 cache 队列中(cache 队列中的图像可重复,如图中出现两次 img3),同时如果 cache 队列长度超过预设长度,则随机 pop 一张图(为了 tiny 模型训练更稳定,在 tiny 模型中不采用随机 pop 的方式, 而是移除最先加入的图片。),当需要进行混合数据增强时,只需要从 cache 中随机选择需要的图像进行拼接等处理,而不需要全部从硬盘中加载,节省了图像加载的时间。
|
||||
|
||||
> cache 队列的最大长度 N 为可调整参数,根据经验性的原则,当为每一张需要混合的图片提供十个缓存时,可以认为提供了足够的随机性,而 Mosaic 增强是四张图混合,因此 cache 数量默认 N=40, 同理 MixUp 的 cache 数量默认为20, tiny 模型需要更稳定的训练条件,因此其 cache 数量也为其余规格模型的一半( MixUp 为10,Mosaic 为20)
|
||||
|
||||
在具体实现中,MMYOLO 设计了`BaseMiximageTransform`类来支持多张图像混合数据增强:
|
||||
|
||||
```python
|
||||
if self.use_cached:
|
||||
# Be careful: deep copying can be very time-consuming
|
||||
# if results includes dataset.
|
||||
dataset = results.pop('dataset', None)
|
||||
self.results_cache.append(copy.deepcopy(results)) # 将当前加载的图片数据缓存到 cache 中
|
||||
if len(self.results_cache) > self.max_cached_images:
|
||||
if self.random_pop: # 除了tiny模型,self.random_pop=True
|
||||
index = random.randint(0, len(self.results_cache) - 1)
|
||||
else:
|
||||
index = 0
|
||||
self.results_cache.pop(index)
|
||||
|
||||
if len(self.results_cache) <= 4:
|
||||
return results
|
||||
else:
|
||||
assert 'dataset' in results
|
||||
# Be careful: deep copying can be very time-consuming
|
||||
# if results includes dataset.
|
||||
dataset = results.pop('dataset', None)
|
||||
```
|
||||
|
||||
### Mosaic
|
||||
|
||||
Mosaic 是将 4 张图拼接为 1 张大图,相当于变相的增加了 batch size,具体步骤为:
|
||||
|
||||
1. 根据索引随机从自定义数据集中再采样3个图像,可能重复
|
||||
|
||||
```python
|
||||
def get_indexes(self, dataset: Union[BaseDataset, list]) -> list:
|
||||
"""Call function to collect indexes.
|
||||
|
||||
Args:
|
||||
dataset (:obj:`Dataset` or list): The dataset or cached list.
|
||||
|
||||
Returns:
|
||||
list: indexes.
|
||||
"""
|
||||
indexes = [random.randint(0, len(dataset)) for _ in range(3)]
|
||||
return indexes
|
||||
```
|
||||
|
||||
2. 随机选出 4 幅图像相交的中点。
|
||||
|
||||
```python
|
||||
# mosaic center x, y
|
||||
center_x = int(
|
||||
random.uniform(*self.center_ratio_range) * self.img_scale[1])
|
||||
center_y = int(
|
||||
random.uniform(*self.center_ratio_range) * self.img_scale[0])
|
||||
center_position = (center_x, center_y)
|
||||
```
|
||||
|
||||
3. 根据采样的 index 读取图片并拼接, 拼接前会先进行 keep-ratio 的 resize 图片(即为最大边一定是 640)。
|
||||
|
||||
```python
|
||||
# keep_ratio resize
|
||||
scale_ratio_i = min(self.img_scale[0] / h_i,
|
||||
self.img_scale[1] / w_i)
|
||||
img_i = mmcv.imresize(
|
||||
img_i, (int(w_i * scale_ratio_i), int(h_i * scale_ratio_i)))
|
||||
```
|
||||
|
||||
4. 拼接后,把 bbox 和 label 全部拼接起来,然后对 bbox 进行裁剪但是不过滤(可能出现一些无效框)
|
||||
|
||||
```python
|
||||
mosaic_bboxes.clip_([2 * self.img_scale[0], 2 * self.img_scale[1]])
|
||||
```
|
||||
|
||||
更多的关于 Mosaic 原理的详情可以参考 [YOLOv5 原理和实现全解析](./yolov5_description.md) 中的 Mosaic 原理分析。
|
||||
|
||||
### MixUp
|
||||
|
||||
RTMDet 的 MixUp 实现方式与 YOLOX 中一样,只不过增加了类似上文中提到的 [cache](#31-为图像混合数据增强引入cache) 功能。
|
||||
|
||||
更多的关于 MixUp 原理的详情也可以参考 [YOLOv5 原理和实现全解析](./yolov5_description.md) 中的 MixUp 原理分析。
|
||||
|
||||
### 强弱两阶段训练
|
||||
|
||||
Mosaic+MixUp 失真度比较高,持续用太强的数据增强对模型并不一定有益。YOLOX 中率先使用了强弱两阶段的训川练方式,但由于引入了旋转,切片导致box标注产生误差,需要在第二阶段引入额外的L1oss来纠正回归分支的性能。
|
||||
|
||||
为了使数据增强的方式更为通用,RTMDet 在前 280 epoch 使用不带旋转的 Mosaic+MixUp, 且通过混入8张图片来提升强度以及正样本数。后 20 epoch 使用比较小的学习率在比较弱的 Random Crop 下进行微调,同时在EMA的作用下将参数缓慢更新至模型,能够得到比较大的提升。
|
||||
|
||||
## 模型结构
|
||||
|
||||
RTMDet 模型整体结构和 [YOLOX](https://arxiv.org/abs/2107.08430) 几乎一致,由 `CSPNeXt` + `CSPNeXtPAFPN` + `共享卷积权重但分别计算 BN 的 SepBNHead` 构成。内部核心模块也是 `CSPLayer`,但对其中的 `Basic Block` 进行了改进,提出了 `CSPNeXt Block`。
|
||||
|
|
|
@ -586,7 +586,7 @@ max_per_img 表示最终保留的最大检测框数目,通常设置为 300。
|
|||
以 COCO 80 类为例,假设输入图片大小为 640x640
|
||||
|
||||
<div align=center >
|
||||
<img alt="image" src="https://user-images.githubusercontent.com/40284075/190550471-a71ecf04-f427-4ba6-b62f-71f4fdd2c8c7.png"/>
|
||||
<img alt="image" src="https://user-images.githubusercontent.com/17425982/192942249-96b0fcfb-059f-48fe-862f-7d526a3a06d7.png"/>
|
||||
</div>
|
||||
|
||||
其推理和后处理过程为:
|
||||
|
|
Loading…
Reference in New Issue