* add * reproduce map * add typehint and doc * format code * replace key * add ut * format * format * format code * fix ut * fix ut * fix comment * fix comment * fix comment * [WIP][Feature] Support yolov5-Ins training * fix comment * change data flow and fix loss_mask compute * align the data pipeline * remove albu gt mask key * support yolov5 ins inference * fix multi gpu test * align the post_process with v8 * support training * support training * code formatting * code formatting * Support pad_param type (#672) * add half_pad_param * fix default fast_test * fix loss weight compute * add models * add dataset1 * add dataset2 * add dataset3 * add configs * re commit __init__ * re commit __init__ * re commit * del local * add typo * del PoseToDetConverter and BBoxKeypoints * del local changes * fix mask rescale, add segment merge, fix segment2bbox * fix pipeline * add dataset * fix typo * add resize in mmyolo * fix typo * del local * del local changes * del local changes * fix dir name * fix dir name * add FilterAnnotations * fix typo * new config for yolox-pose * fix typo * fix typo * fix clip and fix mask init * del pose dataset changes * fix YOLOv5DetDataPreprocessor * del local file * fix typo * del init_cfg * simplify config * fix batch size * fix batch size * fix typo * code formatting * code formatting * code formatting * code formatting * fix bug for FilterAnnotations * simpler way for FilterAnnotations * update config * [Fix] fix load image from file * shorten eval time * fix typo * add large model * [Add] Add docs and more config * [Fix] config type and test_formatting * [Fix] fix yolov5-ins_m packdetinputs * hand rebase from yolov5-ins * use new PackDetInputs * rebase fix typo * add mapping table * fix typo * add weight * del typo * del typo * add results * install mmpose, Keypoints note, context manager, predict, ota rename * fix test * add unittest for pose_sim_ota_assigner and yolox_head * add unittest for pose_sim_ota_assigner and yolox_head * fix typo --------- Co-authored-by: Nioolek <379319054@qq.com> Co-authored-by: josonchan <josonchan1998@163.com> Co-authored-by: Nioolek <40284075+Nioolek@users.noreply.github.com> Co-authored-by: huanghaian <huanghaian@sensetime.com> |
||
---|---|---|
.. | ||
pose | ||
README.md | ||
metafile.yml | ||
yolox_l_fast_8xb8-300e_coco.py | ||
yolox_m_fast_8xb8-300e_coco.py | ||
yolox_m_fast_8xb32-300e-rtmdet-hyp_coco.py | ||
yolox_nano_fast_8xb8-300e_coco.py | ||
yolox_nano_fast_8xb32-300e-rtmdet-hyp_coco.py | ||
yolox_p5_tta.py | ||
yolox_s_fast_1xb12-40e-rtmdet-hyp_cat.py | ||
yolox_s_fast_8xb8-300e_coco.py | ||
yolox_s_fast_8xb32-300e-rtmdet-hyp_coco.py | ||
yolox_tiny_fast_8xb8-300e_coco.py | ||
yolox_tiny_fast_8xb32-300e-rtmdet-hyp_coco.py | ||
yolox_x_fast_8xb8-300e_coco.py |
README.md
YOLOX
Abstract
In this report, we present some experienced improvements to YOLO series, forming a new high-performance detector -- YOLOX. We switch the YOLO detector to an anchor-free manner and conduct other advanced detection techniques, i.e., a decoupled head and the leading label assignment strategy SimOTA to achieve state-of-the-art results across a large scale range of models: For YOLO-Nano with only 0.91M parameters and 1.08G FLOPs, we get 25.3% AP on COCO, surpassing NanoDet by 1.8% AP; for YOLOv3, one of the most widely used detectors in industry, we boost it to 47.3% AP on COCO, outperforming the current best practice by 3.0% AP; for YOLOX-L with roughly the same amount of parameters as YOLOv4-CSP, YOLOv5-L, we achieve 50.0% AP on COCO at a speed of 68.9 FPS on Tesla V100, exceeding YOLOv5-L by 1.8% AP. Further, we won the 1st Place on Streaming Perception Challenge (Workshop on Autonomous Driving at CVPR 2021) using a single YOLOX-L model. We hope this report can provide useful experience for developers and researchers in practical scenes, and we also provide deploy versions with ONNX, TensorRT, NCNN, and Openvino supported.
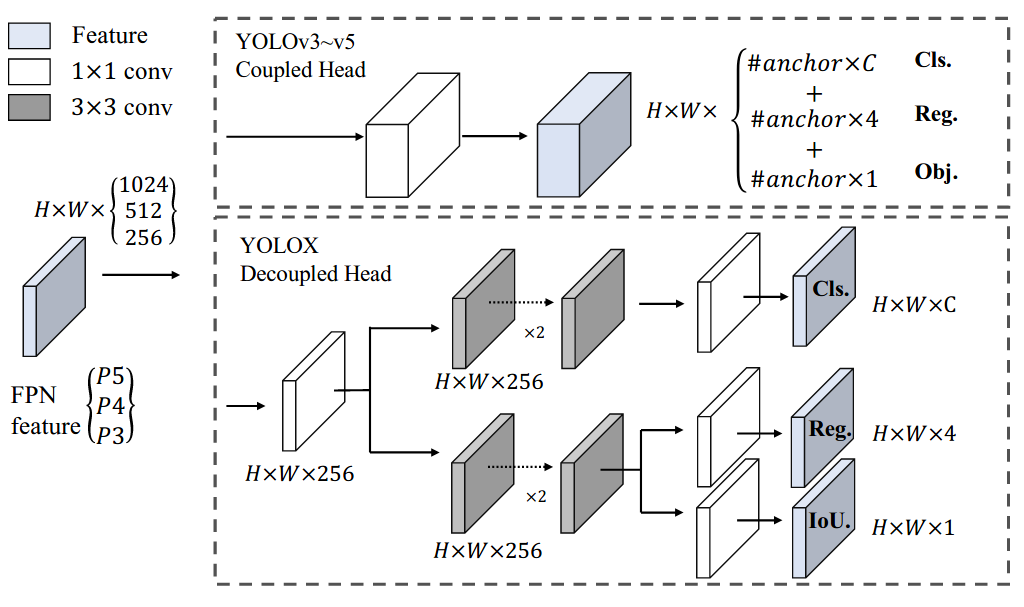
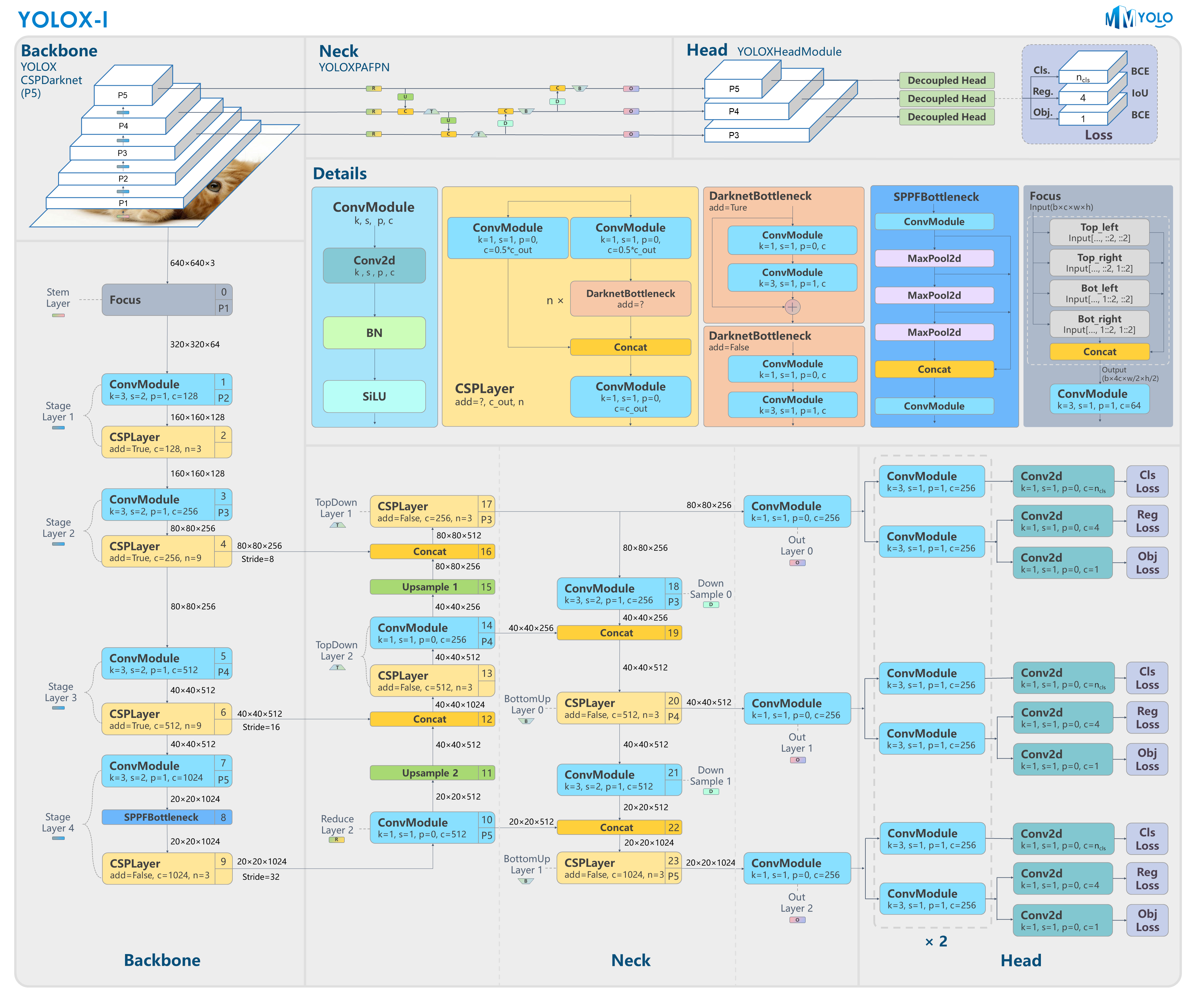
🥳 🚀 Results and Models
Backbone | Size | Batch Size | AMP | RTMDet-Hyp | Mem (GB) | Box AP | Config | Download |
---|---|---|---|---|---|---|---|---|
YOLOX-tiny | 416 | 8xb8 | No | No | 2.8 | 32.7 | config | model | log |
YOLOX-tiny | 416 | 8xb32 | Yes | Yes | 4.9 | 34.3 (+1.6) | config | model | log |
YOLOX-s | 640 | 8xb8 | Yes | No | 2.9 | 40.7 | config | model | log |
YOLOX-s | 640 | 8xb32 | Yes | Yes | 9.8 | 41.9 (+1.2) | config | model | log |
YOLOX-m | 640 | 8xb8 | Yes | No | 4.9 | 46.9 | config | model | log |
YOLOX-m | 640 | 8xb32 | Yes | Yes | 17.6 | 47.5 (+0.6) | config | model | log |
YOLOX-l | 640 | 8xb8 | Yes | No | 8.0 | 50.1 | config | model | log |
YOLOX-x | 640 | 8xb8 | Yes | No | 9.8 | 51.4 | config | model | log |
YOLOX uses a default training configuration of 8xbs8
which results in a long training time, we expect it to use 8xbs32
to speed up the training and not cause a decrease in mAP. We modified train_batch_size_per_gpu
from 8 to 32, batch_augments_interval
from 10 to 1 and base_lr
from 0.01 to 0.04 under YOLOX-s default configuration based on the linear scaling rule, which resulted in mAP degradation. Finally, I found that using RTMDet's training hyperparameter can improve performance in YOLOX Tiny/S/M, which also validates the superiority of RTMDet's training hyperparameter.
The modified training parameters are as follows:
- train_batch_size_per_gpu: 8 -> 32
- batch_augments_interval: 10 -> 1
- num_last_epochs: 15 -> 20
- optim cfg: SGD -> AdamW, base_lr 0.01 -> 0.004, weight_decay 0.0005 -> 0.05
- ema momentum: 0.0001 -> 0.0002
Note:
- The test score threshold is 0.001.
- Due to the need for pre-training weights, we cannot reproduce the performance of the
yolox-nano
model. Please refer to https://github.com/Megvii-BaseDetection/YOLOX/issues/674 for more information.
YOLOX-Pose
Based on MMPose, we have implemented a YOLOX-based human pose estimator, utilizing the approach outlined in YOLO-Pose: Enhancing YOLO for Multi Person Pose Estimation Using Object Keypoint Similarity Loss (CVPRW 2022). This pose estimator is lightweight and quick, making it well-suited for crowded scenes.
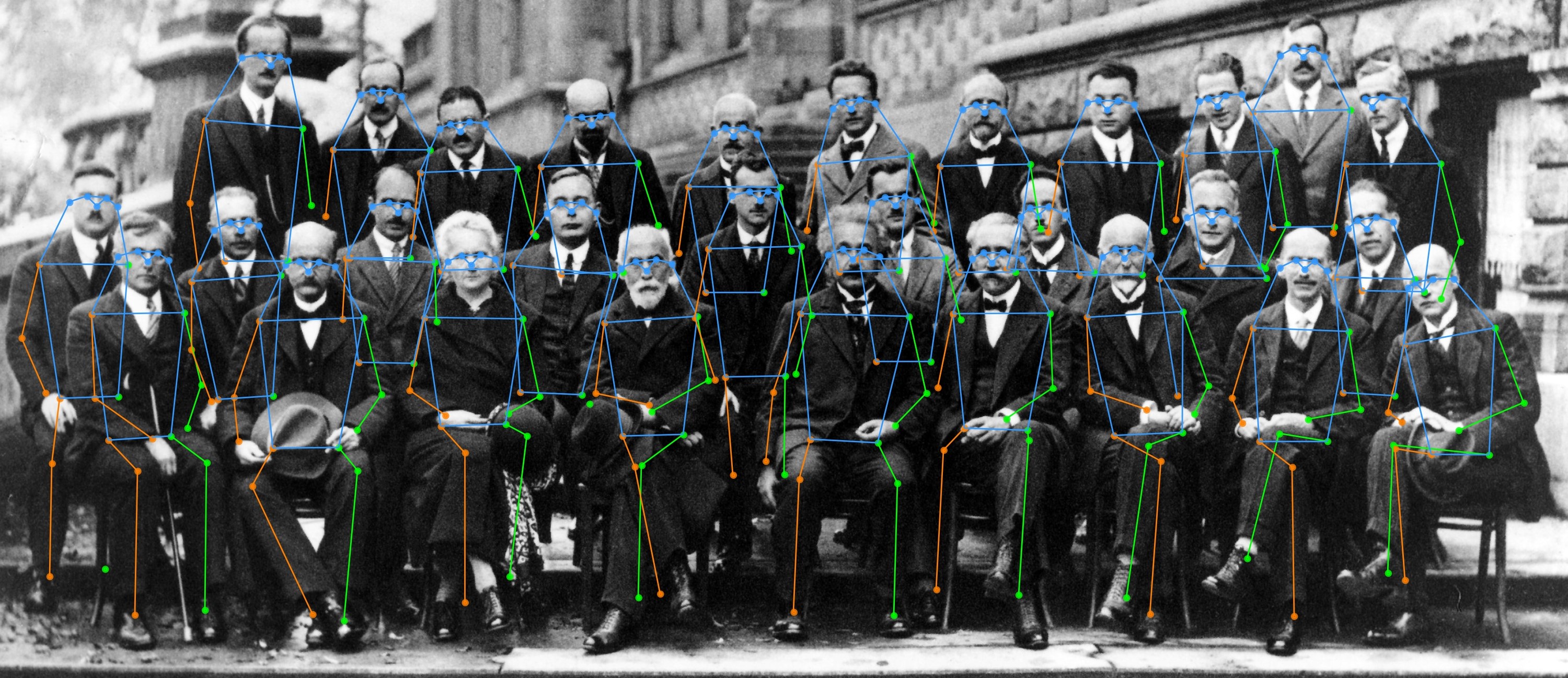
Results
Backbone | Size | Batch Size | AMP | RTMDet-Hyp | Mem (GB) | AP | Config | Download |
---|---|---|---|---|---|---|---|---|
YOLOX-tiny | 416 | 8xb32 | Yes | Yes | 5.3 | 52.8 | config | model | log |
YOLOX-s | 640 | 8xb32 | Yes | Yes | 10.7 | 63.7 | config | model | log |
YOLOX-m | 640 | 8xb32 | Yes | Yes | 19.2 | 69.3 | config | model | log |
YOLOX-l | 640 | 8xb32 | Yes | Yes | 30.3 | 71.1 | config | model | log |
Note
- The performance is unstable and may fluctuate and the highest performance weight in
COCO
training may not be the last epoch. The performance shown above is the best model.
Installation
Install MMPose
mim install -r requirements/mmpose.txt
Citation
@article{yolox2021,
title={{YOLOX}: Exceeding YOLO Series in 2021},
author={Ge, Zheng and Liu, Songtao and Wang, Feng and Li, Zeming and Sun, Jian},
journal={arXiv preprint arXiv:2107.08430},
year={2021}
}