ClearML experiment tracking integration (#8620)
* Add titles to matplotlib plots * Add ClearML Experiment Tracking integration. * Add ClearML Data Version Management automatic download when requested * Add ClearML Hyperparameter Optimization * ClearML save period integration * Fix wandb breaking when used with ClearML dataset * Fix wandb breaking when used with ClearML resume and dataset * Add ClearML documentation * fixed small bug in clearml integration that misreports epoch number * Final ClearMl additions before refactor * Add correct epoch reporting * Add remote execution and autoscaling docs for ClearML integration * Added images to clearml integration docs * fixed logo alignment bug and added hpo screenshot clearml * Fixed small epoch number bug in clearml integration * Remove saved model flush clearml * Cleanup clearml readme section * Cleaned up clearml logger docstring * Remove resume readme section clearml * Clearml integration cleanup * Updated ClearML documentation * Added dark vs light icons ClearML Readme * Clearml Readme styling * Add better gifs * Fixed gif file size * Add better images in tutorial notebook * [pre-commit.ci] auto fixes from pre-commit.com hooks for more information, see https://pre-commit.ci * Addressed comments in PR #8620 * Fixed circular import * Fixed circular import * Update tutorial.ipynb * Update tutorial.ipynb * Inline comment * Restructured tutorial notebook * Add correct ClearML link to README * Update tutorial.ipynb * Update general.py * Update __init__.py * Update __init__.py * [pre-commit.ci] auto fixes from pre-commit.com hooks for more information, see https://pre-commit.ci * Update __init__.py * [pre-commit.ci] auto fixes from pre-commit.com hooks for more information, see https://pre-commit.ci * Update __init__.py * Update README.md * Update __init__.py * [pre-commit.ci] auto fixes from pre-commit.com hooks for more information, see https://pre-commit.ci * spelling * Update tutorial.ipynb * notebook cutt.ly links * Update README.md * Update README.md * cutt.ly links in tutorial * Removed labels as they show up on last subplot only Co-authored-by: pre-commit-ci[bot] <66853113+pre-commit-ci[bot]@users.noreply.github.com> Co-authored-by: Glenn Jocher <glenn.jocher@ultralytics.com>pull/8887/head
parent
2794483e09
commit
378bde4bba
21
README.md
21
README.md
|
@ -151,7 +151,8 @@ python train.py --data coco.yaml --cfg yolov5n.yaml --weights '' --batch-size 12
|
|||
- [Train Custom Data](https://github.com/ultralytics/yolov5/wiki/Train-Custom-Data) 🚀 RECOMMENDED
|
||||
- [Tips for Best Training Results](https://github.com/ultralytics/yolov5/wiki/Tips-for-Best-Training-Results) ☘️
|
||||
RECOMMENDED
|
||||
- [Weights & Biases Logging](https://github.com/ultralytics/yolov5/issues/1289) 🌟 NEW
|
||||
- [ClearML Logging](https://github.com/ultralytics/yolov5/tree/master/utils/loggers/clearml) 🌟 NEW
|
||||
- [Weights & Biases Logging](https://github.com/ultralytics/yolov5/issues/1289)
|
||||
- [Roboflow for Datasets, Labeling, and Active Learning](https://github.com/ultralytics/yolov5/issues/4975) 🌟 NEW
|
||||
- [Multi-GPU Training](https://github.com/ultralytics/yolov5/issues/475)
|
||||
- [PyTorch Hub](https://github.com/ultralytics/yolov5/issues/36) ⭐ NEW
|
||||
|
@ -190,17 +191,23 @@ Get started in seconds with our verified environments. Click each icon below for
|
|||
## <div align="center">Integrations</div>
|
||||
|
||||
<div align="center">
|
||||
<a href="https://wandb.ai/site?utm_campaign=repo_yolo_readme">
|
||||
<img src="https://github.com/ultralytics/yolov5/releases/download/v1.0/logo-wb-long.png" width="49%"/>
|
||||
<a href="https://cutt.ly/yolov5-readme-clearml#gh-light-mode-only">
|
||||
<img src="https://github.com/thepycoder/clearml_screenshots/raw/main/banner_github.png#gh-light-mode-only" width="32%" />
|
||||
</a>
|
||||
<a href="https://cutt.ly/yolov5-readme-clearml#gh-dark-mode-only">
|
||||
<img src="https://github.com/thepycoder/clearml_screenshots/raw/main/banner_github_light.png#gh-dark-mode-only" width="32%" />
|
||||
</a>
|
||||
<a href="https://roboflow.com/?ref=ultralytics">
|
||||
<img src="https://github.com/ultralytics/yolov5/releases/download/v1.0/logo-roboflow-long.png" width="49%"/>
|
||||
<img src="https://github.com/ultralytics/yolov5/releases/download/v1.0/logo-roboflow-long.png" width="33%"/>
|
||||
</a>
|
||||
<a href="https://wandb.ai/site?utm_campaign=repo_yolo_readme">
|
||||
<img src="https://github.com/ultralytics/yolov5/releases/download/v1.0/logo-wb-long.png" width="33%"/>
|
||||
</a>
|
||||
</div>
|
||||
|
||||
|Weights and Biases|Roboflow ⭐ NEW|
|
||||
|:-:|:-:|
|
||||
|Automatically track and visualize all your YOLOv5 training runs in the cloud with [Weights & Biases](https://wandb.ai/site?utm_campaign=repo_yolo_readme)|Label and export your custom datasets directly to YOLOv5 for training with [Roboflow](https://roboflow.com/?ref=ultralytics) |
|
||||
|ClearML ⭐ NEW|Roboflow|Weights and Biases
|
||||
|:-:|:-:|:-:|
|
||||
|Automatically track, visualize and even remotely train YOLOv5 using [ClearML](https://cutt.ly/yolov5-readme-clearml) (open-source!)|Label and export your custom datasets directly to YOLOv5 for training with [Roboflow](https://roboflow.com/?ref=ultralytics) |Automatically track and visualize all your YOLOv5 training runs in the cloud with [Weights & Biases](https://wandb.ai/site?utm_campaign=repo_yolo_readme)
|
||||
|
||||
<!-- ## <div align="center">Compete and Win</div>
|
||||
|
||||
|
|
|
@ -17,6 +17,7 @@ protobuf<=3.20.1 # https://github.com/ultralytics/yolov5/issues/8012
|
|||
# Logging -------------------------------------
|
||||
tensorboard>=2.4.1
|
||||
# wandb
|
||||
# clearml
|
||||
|
||||
# Plotting ------------------------------------
|
||||
pandas>=1.1.4
|
||||
|
|
2
train.py
2
train.py
|
@ -90,6 +90,8 @@ def train(hyp, opt, device, callbacks): # hyp is path/to/hyp.yaml or hyp dictio
|
|||
data_dict = None
|
||||
if RANK in {-1, 0}:
|
||||
loggers = Loggers(save_dir, weights, opt, hyp, LOGGER) # loggers instance
|
||||
if loggers.clearml:
|
||||
data_dict = loggers.clearml.data_dict # None if no ClearML dataset or filled in by ClearML
|
||||
if loggers.wandb:
|
||||
data_dict = loggers.wandb.data_dict
|
||||
if resume:
|
||||
|
|
|
@ -7,6 +7,7 @@
|
|||
"provenance": [],
|
||||
"collapsed_sections": [],
|
||||
"machine_shape": "hm",
|
||||
"toc_visible": true,
|
||||
"include_colab_link": true
|
||||
},
|
||||
"kernelspec": {
|
||||
|
@ -913,6 +914,30 @@
|
|||
"# 4. Visualize"
|
||||
]
|
||||
},
|
||||
{
|
||||
"cell_type": "markdown",
|
||||
"source": [
|
||||
"## ClearML Logging and Automation 🌟 NEW\n",
|
||||
"\n",
|
||||
"[ClearML](https://cutt.ly/yolov5-notebook-clearml) is completely integrated into YOLOv5 to track your experimentation, manage dataset versions and even remotely execute training runs.\n",
|
||||
"\n",
|
||||
"To enable ClearML (Check cells above):\n",
|
||||
"- `pip install clearml`\n",
|
||||
"- run `clearml-init` to connect to a ClearML server (**deploy your own open-source server [here](https://github.com/allegroai/clearml-server)**, or use our free hosted server [here](https://cutt.ly/yolov5-notebook-clearml))\n",
|
||||
"\n",
|
||||
"You'll get all the great expected features from an experiment manager: live updates, model upload, experiment comparison etc. but ClearML also tracks uncommitted changes and installed packages for example. Thanks to that ClearML Tasks (which is what we call experiments) are also reproducible on different machines! With only 1 extra line, we can schedule a YOLOv5 training task on a queue to be executed by any number of ClearML Agents (workers).\n",
|
||||
"\n",
|
||||
"You can use ClearML Data to version your dataset and then pass it to YOLOv5 simply using its unique ID. This will help you keep track of your data without adding extra hassle. \n",
|
||||
"\n",
|
||||
"Explore the [ClearML Tutorial](https://github.com/ultralytics/yolov5/tree/master/utils/loggers/clearml) for more info!\n",
|
||||
"\n",
|
||||
"<a href=\"https://cutt.ly/yolov5-notebook-clearml\">\n",
|
||||
"<img alt=\"ClearML Experiment Management UI\" src=\"https://github.com/thepycoder/clearml_screenshots/raw/main/scalars.jpg\" width=\"1280\"/></a>"
|
||||
],
|
||||
"metadata": {
|
||||
"id": "Lay2WsTjNJzP"
|
||||
}
|
||||
},
|
||||
{
|
||||
"cell_type": "markdown",
|
||||
"metadata": {
|
||||
|
@ -1105,4 +1130,4 @@
|
|||
"outputs": []
|
||||
}
|
||||
]
|
||||
}
|
||||
}
|
||||
|
|
|
@ -14,6 +14,7 @@ import random
|
|||
import re
|
||||
import shutil
|
||||
import signal
|
||||
import sys
|
||||
import threading
|
||||
import time
|
||||
import urllib
|
||||
|
@ -449,6 +450,9 @@ def check_file(file, suffix=''):
|
|||
torch.hub.download_url_to_file(url, file)
|
||||
assert Path(file).exists() and Path(file).stat().st_size > 0, f'File download failed: {url}' # check
|
||||
return file
|
||||
elif file.startswith('clearml://'): # ClearML Dataset ID
|
||||
assert 'clearml' in sys.modules, "ClearML is not installed, so cannot use ClearML dataset. Try running 'pip install clearml'."
|
||||
return file
|
||||
else: # search
|
||||
files = []
|
||||
for d in 'data', 'models', 'utils': # search directories
|
||||
|
|
|
@ -11,11 +11,12 @@ import torch
|
|||
from torch.utils.tensorboard import SummaryWriter
|
||||
|
||||
from utils.general import colorstr, cv2, emojis
|
||||
from utils.loggers.clearml.clearml_utils import ClearmlLogger
|
||||
from utils.loggers.wandb.wandb_utils import WandbLogger
|
||||
from utils.plots import plot_images, plot_results
|
||||
from utils.torch_utils import de_parallel
|
||||
|
||||
LOGGERS = ('csv', 'tb', 'wandb') # text-file, TensorBoard, Weights & Biases
|
||||
LOGGERS = ('csv', 'tb', 'wandb', 'clearml') # *.csv, TensorBoard, Weights & Biases, ClearML
|
||||
RANK = int(os.getenv('RANK', -1))
|
||||
|
||||
try:
|
||||
|
@ -32,6 +33,13 @@ try:
|
|||
except (ImportError, AssertionError):
|
||||
wandb = None
|
||||
|
||||
try:
|
||||
import clearml
|
||||
|
||||
assert hasattr(clearml, '__version__') # verify package import not local dir
|
||||
except (ImportError, AssertionError):
|
||||
clearml = None
|
||||
|
||||
|
||||
class Loggers():
|
||||
# YOLOv5 Loggers class
|
||||
|
@ -61,10 +69,14 @@ class Loggers():
|
|||
setattr(self, k, None) # init empty logger dictionary
|
||||
self.csv = True # always log to csv
|
||||
|
||||
# Message
|
||||
# Messages
|
||||
if not wandb:
|
||||
prefix = colorstr('Weights & Biases: ')
|
||||
s = f"{prefix}run 'pip install wandb' to automatically track and visualize YOLOv5 🚀 runs (RECOMMENDED)"
|
||||
s = f"{prefix}run 'pip install wandb' to automatically track and visualize YOLOv5 🚀 runs in Weights & Biases"
|
||||
self.logger.info(emojis(s))
|
||||
if not clearml:
|
||||
prefix = colorstr('ClearML: ')
|
||||
s = f"{prefix}run 'pip install clearml' to automatically track, visualize and remotely train YOLOv5 🚀 runs in ClearML"
|
||||
self.logger.info(emojis(s))
|
||||
|
||||
# TensorBoard
|
||||
|
@ -82,12 +94,17 @@ class Loggers():
|
|||
self.wandb = WandbLogger(self.opt, run_id)
|
||||
# temp warn. because nested artifacts not supported after 0.12.10
|
||||
if pkg.parse_version(wandb.__version__) >= pkg.parse_version('0.12.11'):
|
||||
self.logger.warning(
|
||||
"YOLOv5 temporarily requires wandb version 0.12.10 or below. Some features may not work as expected."
|
||||
)
|
||||
s = "YOLOv5 temporarily requires wandb version 0.12.10 or below. Some features may not work as expected."
|
||||
self.logger.warning(s)
|
||||
else:
|
||||
self.wandb = None
|
||||
|
||||
# ClearML
|
||||
if clearml and 'clearml' in self.include:
|
||||
self.clearml = ClearmlLogger(self.opt, self.hyp)
|
||||
else:
|
||||
self.clearml = None
|
||||
|
||||
def on_train_start(self):
|
||||
# Callback runs on train start
|
||||
pass
|
||||
|
@ -97,9 +114,12 @@ class Loggers():
|
|||
paths = self.save_dir.glob('*labels*.jpg') # training labels
|
||||
if self.wandb:
|
||||
self.wandb.log({"Labels": [wandb.Image(str(x), caption=x.name) for x in paths]})
|
||||
if self.clearml:
|
||||
pass # ClearML saves these images automatically using hooks
|
||||
|
||||
def on_train_batch_end(self, ni, model, imgs, targets, paths, plots):
|
||||
# Callback runs on train batch end
|
||||
# ni: number integrated batches (since train start)
|
||||
if plots:
|
||||
if ni == 0:
|
||||
if self.tb and not self.opt.sync_bn: # --sync known issue https://github.com/ultralytics/yolov5/issues/3754
|
||||
|
@ -109,9 +129,12 @@ class Loggers():
|
|||
if ni < 3:
|
||||
f = self.save_dir / f'train_batch{ni}.jpg' # filename
|
||||
plot_images(imgs, targets, paths, f)
|
||||
if self.wandb and ni == 10:
|
||||
if (self.wandb or self.clearml) and ni == 10:
|
||||
files = sorted(self.save_dir.glob('train*.jpg'))
|
||||
self.wandb.log({'Mosaics': [wandb.Image(str(f), caption=f.name) for f in files if f.exists()]})
|
||||
if self.wandb:
|
||||
self.wandb.log({'Mosaics': [wandb.Image(str(f), caption=f.name) for f in files if f.exists()]})
|
||||
if self.clearml:
|
||||
self.clearml.log_debug_samples(files, title='Mosaics')
|
||||
|
||||
def on_train_epoch_end(self, epoch):
|
||||
# Callback runs on train epoch end
|
||||
|
@ -122,12 +145,17 @@ class Loggers():
|
|||
# Callback runs on val image end
|
||||
if self.wandb:
|
||||
self.wandb.val_one_image(pred, predn, path, names, im)
|
||||
if self.clearml:
|
||||
self.clearml.log_image_with_boxes(path, pred, names, im)
|
||||
|
||||
def on_val_end(self):
|
||||
# Callback runs on val end
|
||||
if self.wandb:
|
||||
if self.wandb or self.clearml:
|
||||
files = sorted(self.save_dir.glob('val*.jpg'))
|
||||
self.wandb.log({"Validation": [wandb.Image(str(f), caption=f.name) for f in files]})
|
||||
if self.wandb:
|
||||
self.wandb.log({"Validation": [wandb.Image(str(f), caption=f.name) for f in files]})
|
||||
if self.clearml:
|
||||
self.clearml.log_debug_samples(files, title='Validation')
|
||||
|
||||
def on_fit_epoch_end(self, vals, epoch, best_fitness, fi):
|
||||
# Callback runs at the end of each fit (train+val) epoch
|
||||
|
@ -142,6 +170,10 @@ class Loggers():
|
|||
if self.tb:
|
||||
for k, v in x.items():
|
||||
self.tb.add_scalar(k, v, epoch)
|
||||
elif self.clearml: # log to ClearML if TensorBoard not used
|
||||
for k, v in x.items():
|
||||
title, series = k.split('/')
|
||||
self.clearml.task.get_logger().report_scalar(title, series, v, epoch)
|
||||
|
||||
if self.wandb:
|
||||
if best_fitness == fi:
|
||||
|
@ -151,12 +183,22 @@ class Loggers():
|
|||
self.wandb.log(x)
|
||||
self.wandb.end_epoch(best_result=best_fitness == fi)
|
||||
|
||||
if self.clearml:
|
||||
self.clearml.current_epoch_logged_images = set() # reset epoch image limit
|
||||
self.clearml.current_epoch += 1
|
||||
|
||||
def on_model_save(self, last, epoch, final_epoch, best_fitness, fi):
|
||||
# Callback runs on model save event
|
||||
if self.wandb:
|
||||
if ((epoch + 1) % self.opt.save_period == 0 and not final_epoch) and self.opt.save_period != -1:
|
||||
self.wandb.log_model(last.parent, self.opt, epoch, fi, best_model=best_fitness == fi)
|
||||
|
||||
if self.clearml:
|
||||
if ((epoch + 1) % self.opt.save_period == 0 and not final_epoch) and self.opt.save_period != -1:
|
||||
self.clearml.task.update_output_model(model_path=str(last),
|
||||
model_name='Latest Model',
|
||||
auto_delete_file=False)
|
||||
|
||||
def on_train_end(self, last, best, plots, epoch, results):
|
||||
# Callback runs on training end
|
||||
if plots:
|
||||
|
@ -165,7 +207,7 @@ class Loggers():
|
|||
files = [(self.save_dir / f) for f in files if (self.save_dir / f).exists()] # filter
|
||||
self.logger.info(f"Results saved to {colorstr('bold', self.save_dir)}")
|
||||
|
||||
if self.tb:
|
||||
if self.tb and not self.clearml: # These images are already captured by ClearML by now, we don't want doubles
|
||||
for f in files:
|
||||
self.tb.add_image(f.stem, cv2.imread(str(f))[..., ::-1], epoch, dataformats='HWC')
|
||||
|
||||
|
@ -180,6 +222,12 @@ class Loggers():
|
|||
aliases=['latest', 'best', 'stripped'])
|
||||
self.wandb.finish_run()
|
||||
|
||||
if self.clearml:
|
||||
# Save the best model here
|
||||
if not self.opt.evolve:
|
||||
self.clearml.task.update_output_model(model_path=str(best if best.exists() else last),
|
||||
name='Best Model')
|
||||
|
||||
def on_params_update(self, params):
|
||||
# Update hyperparams or configs of the experiment
|
||||
# params: A dict containing {param: value} pairs
|
||||
|
|
|
@ -0,0 +1,222 @@
|
|||
# ClearML Integration
|
||||
|
||||
<img align="center" src="https://github.com/thepycoder/clearml_screenshots/raw/main/logos_dark.png#gh-light-mode-only" alt="Clear|ML"><img align="center" src="https://github.com/thepycoder/clearml_screenshots/raw/main/logos_light.png#gh-dark-mode-only" alt="Clear|ML">
|
||||
|
||||
## About ClearML
|
||||
|
||||
[ClearML](https://cutt.ly/yolov5-tutorial-clearml) is an [open-source](https://github.com/allegroai/clearml) toolbox designed to save you time ⏱️.
|
||||
|
||||
🔨 Track every YOLOv5 training run in the <b>experiment manager</b>
|
||||
|
||||
🔧 Version and easily access your custom training data with the integrated ClearML <b>Data Versioning Tool</b>
|
||||
|
||||
🔦 <b>Remotely train and monitor</b> your YOLOv5 training runs using ClearML Agent
|
||||
|
||||
🔬 Get the very best mAP using ClearML <b>Hyperparameter Optimization</b>
|
||||
|
||||
🔭 Turn your newly trained <b>YOLOv5 model into an API</b> with just a few commands using ClearML Serving
|
||||
|
||||
<br />
|
||||
And so much more. It's up to you how many of these tools you want to use, you can stick to the experiment manager, or chain them all together into an impressive pipeline!
|
||||
<br />
|
||||
<br />
|
||||
|
||||

|
||||
|
||||
|
||||
<br />
|
||||
<br />
|
||||
|
||||
## 🦾 Setting Things Up
|
||||
|
||||
To keep track of your experiments and/or data, ClearML needs to communicate to a server. You have 2 options to get one:
|
||||
|
||||
Either sign up for free to the [ClearML Hosted Service](https://cutt.ly/yolov5-tutorial-clearml) or you can set up your own server, see [here](https://clear.ml/docs/latest/docs/deploying_clearml/clearml_server). Even the server is open-source, so even if you're dealing with sensitive data, you should be good to go!
|
||||
|
||||
1. Install the `clearml` python package:
|
||||
|
||||
```bash
|
||||
pip install clearml
|
||||
```
|
||||
|
||||
1. Connect the ClearML SDK to the server by [creating credentials](https://app.clear.ml/settings/workspace-configuration) (go right top to Settings -> Workspace -> Create new credentials), then execute the command below and follow the instructions:
|
||||
|
||||
```bash
|
||||
clearml-init
|
||||
```
|
||||
|
||||
That's it! You're done 😎
|
||||
|
||||
<br />
|
||||
|
||||
## 🚀 Training YOLOv5 With ClearML
|
||||
|
||||
To enable ClearML experiment tracking, simply install the ClearML pip package.
|
||||
|
||||
```bash
|
||||
pip install clearml
|
||||
```
|
||||
|
||||
This will enable integration with the YOLOv5 training script. Every training run from now on, will be captured and stored by the ClearML experiment manager. If you want to change the `project_name` or `task_name`, head over to our custom logger, where you can change it: `utils/loggers/clearml/clearml_utils.py`
|
||||
|
||||
```bash
|
||||
python train.py --img 640 --batch 16 --epochs 3 --data coco128.yaml --weights yolov5s.pt --cache
|
||||
```
|
||||
|
||||
This will capture:
|
||||
- Source code + uncommitted changes
|
||||
- Installed packages
|
||||
- (Hyper)parameters
|
||||
- Model files (use `--save-period n` to save a checkpoint every n epochs)
|
||||
- Console output
|
||||
- Scalars (mAP_0.5, mAP_0.5:0.95, precision, recall, losses, learning rates, ...)
|
||||
- General info such as machine details, runtime, creation date etc.
|
||||
- All produced plots such as label correlogram and confusion matrix
|
||||
- Images with bounding boxes per epoch
|
||||
- Mosaic per epoch
|
||||
- Validation images per epoch
|
||||
- ...
|
||||
|
||||
That's a lot right? 🤯
|
||||
Now, we can visualize all of this information in the ClearML UI to get an overview of our training progress. Add custom columns to the table view (such as e.g. mAP_0.5) so you can easily sort on the best performing model. Or select multiple experiments and directly compare them!
|
||||
|
||||
There even more we can do with all of this information, like hyperparameter optimization and remote execution, so keep reading if you want to see how that works!
|
||||
|
||||
<br />
|
||||
|
||||
## 🔗 Dataset Version Management
|
||||
|
||||
Versioning your data separately from your code is generally a good idea and makes it easy to aqcuire the latest version too. This repository supports supplying a dataset version ID and it will make sure to get the data if it's not there yet. Next to that, this workflow also saves the used dataset ID as part of the task parameters, so you will always know for sure which data was used in which experiment!
|
||||
|
||||

|
||||
|
||||
### Prepare Your Dataset
|
||||
|
||||
The YOLOv5 repository supports a number of different datasets by using yaml files containing their information. By default datasets are downloaded to the `../datasets` folder in relation to the repository root folder. So if you downloaded the `coco128` dataset using the link in the yaml or with the scripts provided by yolov5, you get this folder structure:
|
||||
|
||||
```
|
||||
..
|
||||
|_ yolov5
|
||||
|_ datasets
|
||||
|_ coco128
|
||||
|_ images
|
||||
|_ labels
|
||||
|_ LICENSE
|
||||
|_ README.txt
|
||||
```
|
||||
But this can be any dataset you wish. Feel free to use your own, as long as you keep to this folder structure.
|
||||
|
||||
Next, ⚠️**copy the corresponding yaml file to the root of the dataset folder**⚠️. This yaml files contains the information ClearML will need to properly use the dataset. You can make this yourself too, of course, just follow the structure of the example yamls.
|
||||
|
||||
Basically we need the following keys: `path`, `train`, `test`, `val`, `nc`, `names`.
|
||||
|
||||
```
|
||||
..
|
||||
|_ yolov5
|
||||
|_ datasets
|
||||
|_ coco128
|
||||
|_ images
|
||||
|_ labels
|
||||
|_ coco128.yaml # <---- HERE!
|
||||
|_ LICENSE
|
||||
|_ README.txt
|
||||
```
|
||||
|
||||
### Upload Your Dataset
|
||||
|
||||
To get this dataset into ClearML as a versionned dataset, go to the dataset root folder and run the following command:
|
||||
```bash
|
||||
cd coco128
|
||||
clearml-data sync --project YOLOv5 --name coco128 --folder .
|
||||
```
|
||||
|
||||
The command `clearml-data sync` is actually a shorthand command. You could also run these commands one after the other:
|
||||
```bash
|
||||
# Optionally add --parent <parent_dataset_id> if you want to base
|
||||
# this version on another dataset version, so no duplicate files are uploaded!
|
||||
clearml-data create --name coco128 --project YOLOv5
|
||||
clearml-data add --files .
|
||||
clearml-data close
|
||||
```
|
||||
|
||||
### Run Training Using A ClearML Dataset
|
||||
|
||||
Now that you have a ClearML dataset, you can very simply use it to train custom YOLOv5 🚀 models!
|
||||
|
||||
```bash
|
||||
python train.py --img 640 --batch 16 --epochs 3 --data clearml://<your_dataset_id> --weights yolov5s.pt --cache
|
||||
```
|
||||
|
||||
<br />
|
||||
|
||||
## 👀 Hyperparameter Optimization
|
||||
|
||||
Now that we have our experiments and data versioned, it's time to take a look at what we can build on top!
|
||||
|
||||
Using the code information, installed packages and environment details, the experiment itself is now **completely reproducible**. In fact, ClearML allows you to clone an experiment and even change its parameters. We can then just rerun it with these new parameters automatically, this is basically what HPO does!
|
||||
|
||||
To **run hyperparameter optimization locally**, we've included a pre-made script for you. Just make sure a training task has been run at least once, so it is in the ClearML experiment manager, we will essentially clone it and change its hyperparameters.
|
||||
|
||||
You'll need to fill in the ID of this `template task` in the script found at `utils/loggers/clearml/hpo.py` and then just run it :) You can change `task.execute_locally()` to `task.execute()` to put it in a ClearML queue and have a remote agent work on it instead.
|
||||
|
||||
```bash
|
||||
# To use optuna, install it first, otherwise you can change the optimizer to just be RandomSearch
|
||||
pip install optuna
|
||||
python utils/loggers/clearml/hpo.py
|
||||
```
|
||||
|
||||

|
||||
|
||||
## 🤯 Remote Execution (advanced)
|
||||
|
||||
Running HPO locally is really handy, but what if we want to run our experiments on a remote machine instead? Maybe you have access to a very powerful GPU machine on-site or you have some budget to use cloud GPUs.
|
||||
This is where the ClearML Agent comes into play. Check out what the agent can do here:
|
||||
|
||||
- [Youtube video](https://youtu.be/MX3BrXnaULs)
|
||||
- [Documentation](https://clear.ml/docs/latest/docs/clearml_agent)
|
||||
|
||||
In short: every experiment tracked by the experiment manager contains enough information to reproduce it on a different machine (installed packages, uncommitted changes etc.). So a ClearML agent does just that: it listens to a queue for incoming tasks and when it finds one, it recreates the environment and runs it while still reporting scalars, plots etc. to the experiment manager.
|
||||
|
||||
You can turn any machine (a cloud VM, a local GPU machine, your own laptop ... ) into a ClearML agent by simply running:
|
||||
```bash
|
||||
clearml-agent daemon --queue <queues_to_listen_to> [--docker]
|
||||
```
|
||||
|
||||
### Cloning, Editing And Enqueuing
|
||||
|
||||
With our agent running, we can give it some work. Remember from the HPO section that we can clone a task and edit the hyperparameters? We can do that from the interface too!
|
||||
|
||||
🪄 Clone the experiment by right clicking it
|
||||
|
||||
🎯 Edit the hyperparameters to what you wish them to be
|
||||
|
||||
⏳ Enqueue the task to any of the queues by right clicking it
|
||||
|
||||

|
||||
|
||||
### Executing A Task Remotely
|
||||
|
||||
Now you can clone a task like we explained above, or simply mark your current script by adding `task.execute_remotely()` and on execution it will be put into a queue, for the agent to start working on!
|
||||
|
||||
To run the YOLOv5 training script remotely, all you have to do is add this line to the training.py script after the clearml logger has been instatiated:
|
||||
```python
|
||||
# ...
|
||||
# Loggers
|
||||
data_dict = None
|
||||
if RANK in {-1, 0}:
|
||||
loggers = Loggers(save_dir, weights, opt, hyp, LOGGER) # loggers instance
|
||||
if loggers.clearml:
|
||||
loggers.clearml.task.execute_remotely(queue='my_queue') # <------ ADD THIS LINE
|
||||
# Data_dict is either None is user did not choose for ClearML dataset or is filled in by ClearML
|
||||
data_dict = loggers.clearml.data_dict
|
||||
# ...
|
||||
```
|
||||
When running the training script after this change, python will run the script up until that line, after which it will package the code and send it to the queue instead!
|
||||
|
||||
### Autoscaling workers
|
||||
|
||||
ClearML comes with autoscalers too! This tool will automatically spin up new remote machines in the cloud of your choice (AWS, GCP, Azure) and turn them into ClearML agents for you whenever there are experiments detected in the queue. Once the tasks are processed, the autoscaler will automatically shut down the remote machines and you stop paying!
|
||||
|
||||
Check out the autoscalers getting started video below.
|
||||
|
||||
[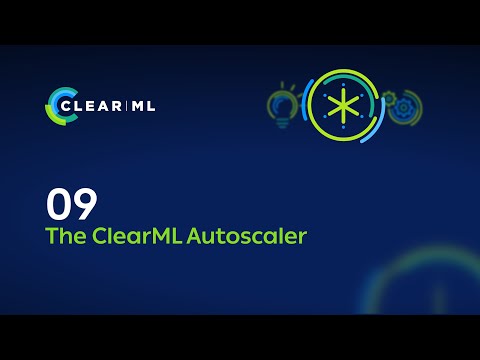](https://youtu.be/j4XVMAaUt3E)
|
|
@ -0,0 +1,150 @@
|
|||
"""Main Logger class for ClearML experiment tracking."""
|
||||
import glob
|
||||
import re
|
||||
from pathlib import Path
|
||||
|
||||
import yaml
|
||||
from torchvision.transforms import ToPILImage
|
||||
from torchvision.utils import draw_bounding_boxes
|
||||
|
||||
try:
|
||||
import clearml
|
||||
from clearml import Dataset, Task
|
||||
|
||||
assert hasattr(clearml, '__version__') # verify package import not local dir
|
||||
except (ImportError, AssertionError):
|
||||
clearml = None
|
||||
|
||||
|
||||
def construct_dataset(clearml_info_string):
|
||||
dataset_id = clearml_info_string.replace('clearml://', '')
|
||||
dataset = Dataset.get(dataset_id=dataset_id)
|
||||
dataset_root_path = Path(dataset.get_local_copy())
|
||||
|
||||
# We'll search for the yaml file definition in the dataset
|
||||
yaml_filenames = list(glob.glob(str(dataset_root_path / "*.yaml")) + glob.glob(str(dataset_root_path / "*.yml")))
|
||||
if len(yaml_filenames) > 1:
|
||||
raise ValueError('More than one yaml file was found in the dataset root, cannot determine which one contains '
|
||||
'the dataset definition this way.')
|
||||
elif len(yaml_filenames) == 0:
|
||||
raise ValueError('No yaml definition found in dataset root path, check that there is a correct yaml file '
|
||||
'inside the dataset root path.')
|
||||
with open(yaml_filenames[0]) as f:
|
||||
dataset_definition = yaml.safe_load(f)
|
||||
|
||||
assert set(dataset_definition.keys()).issuperset(
|
||||
{'train', 'test', 'val', 'nc', 'names'}
|
||||
), "The right keys were not found in the yaml file, make sure it at least has the following keys: ('train', 'test', 'val', 'nc', 'names')"
|
||||
|
||||
data_dict = dict()
|
||||
data_dict['train'] = str(
|
||||
(dataset_root_path / dataset_definition['train']).resolve()) if dataset_definition['train'] else None
|
||||
data_dict['test'] = str(
|
||||
(dataset_root_path / dataset_definition['test']).resolve()) if dataset_definition['test'] else None
|
||||
data_dict['val'] = str(
|
||||
(dataset_root_path / dataset_definition['val']).resolve()) if dataset_definition['val'] else None
|
||||
data_dict['nc'] = dataset_definition['nc']
|
||||
data_dict['names'] = dataset_definition['names']
|
||||
|
||||
return data_dict
|
||||
|
||||
|
||||
class ClearmlLogger:
|
||||
"""Log training runs, datasets, models, and predictions to ClearML.
|
||||
|
||||
This logger sends information to ClearML at app.clear.ml or to your own hosted server. By default,
|
||||
this information includes hyperparameters, system configuration and metrics, model metrics, code information and
|
||||
basic data metrics and analyses.
|
||||
|
||||
By providing additional command line arguments to train.py, datasets,
|
||||
models and predictions can also be logged.
|
||||
"""
|
||||
|
||||
def __init__(self, opt, hyp):
|
||||
"""
|
||||
- Initialize ClearML Task, this object will capture the experiment
|
||||
- Upload dataset version to ClearML Data if opt.upload_dataset is True
|
||||
|
||||
arguments:
|
||||
opt (namespace) -- Commandline arguments for this run
|
||||
hyp (dict) -- Hyperparameters for this run
|
||||
|
||||
"""
|
||||
self.current_epoch = 0
|
||||
# Keep tracked of amount of logged images to enforce a limit
|
||||
self.current_epoch_logged_images = set()
|
||||
# Maximum number of images to log to clearML per epoch
|
||||
self.max_imgs_to_log_per_epoch = 16
|
||||
# Get the interval of epochs when bounding box images should be logged
|
||||
self.bbox_interval = opt.bbox_interval
|
||||
self.clearml = clearml
|
||||
self.task = None
|
||||
self.data_dict = None
|
||||
if self.clearml:
|
||||
self.task = Task.init(
|
||||
project_name='YOLOv5',
|
||||
task_name='training',
|
||||
tags=['YOLOv5'],
|
||||
output_uri=True,
|
||||
auto_connect_frameworks={'pytorch': False}
|
||||
# We disconnect pytorch auto-detection, because we added manual model save points in the code
|
||||
)
|
||||
# ClearML's hooks will already grab all general parameters
|
||||
# Only the hyperparameters coming from the yaml config file
|
||||
# will have to be added manually!
|
||||
self.task.connect(hyp, name='Hyperparameters')
|
||||
|
||||
# Get ClearML Dataset Version if requested
|
||||
if opt.data.startswith('clearml://'):
|
||||
# data_dict should have the following keys:
|
||||
# names, nc (number of classes), test, train, val (all three relative paths to ../datasets)
|
||||
self.data_dict = construct_dataset(opt.data)
|
||||
# Set data to data_dict because wandb will crash without this information and opt is the best way
|
||||
# to give it to them
|
||||
opt.data = self.data_dict
|
||||
|
||||
def log_debug_samples(self, files, title='Debug Samples'):
|
||||
"""
|
||||
Log files (images) as debug samples in the ClearML task.
|
||||
|
||||
arguments:
|
||||
files (List(PosixPath)) a list of file paths in PosixPath format
|
||||
title (str) A title that groups together images with the same values
|
||||
"""
|
||||
for f in files:
|
||||
if f.exists():
|
||||
it = re.search(r'_batch(\d+)', f.name)
|
||||
iteration = int(it.groups()[0]) if it else 0
|
||||
self.task.get_logger().report_image(title=title,
|
||||
series=f.name.replace(it.group(), ''),
|
||||
local_path=str(f),
|
||||
iteration=iteration)
|
||||
|
||||
def log_image_with_boxes(self, image_path, boxes, class_names, image):
|
||||
"""
|
||||
Draw the bounding boxes on a single image and report the result as a ClearML debug sample
|
||||
|
||||
arguments:
|
||||
image_path (PosixPath) the path the original image file
|
||||
boxes (list): list of scaled predictions in the format - [xmin, ymin, xmax, ymax, confidence, class]
|
||||
class_names (dict): dict containing mapping of class int to class name
|
||||
image (Tensor): A torch tensor containing the actual image data
|
||||
"""
|
||||
if len(self.current_epoch_logged_images) < self.max_imgs_to_log_per_epoch and self.current_epoch >= 0:
|
||||
# Log every bbox_interval times and deduplicate for any intermittend extra eval runs
|
||||
if self.current_epoch % self.bbox_interval == 0 and image_path not in self.current_epoch_logged_images:
|
||||
converter = ToPILImage()
|
||||
labels = []
|
||||
for conf, class_nr in zip(boxes[:, 4], boxes[:, 5]):
|
||||
class_name = class_names[int(class_nr)]
|
||||
confidence = round(float(conf) * 100, 2)
|
||||
labels.append(f"{class_name}: {confidence}%")
|
||||
annotated_image = converter(
|
||||
draw_bounding_boxes(image=image.mul(255).clamp(0, 255).byte().cpu(),
|
||||
boxes=boxes[:, :4],
|
||||
labels=labels))
|
||||
self.task.get_logger().report_image(title='Bounding Boxes',
|
||||
series=image_path.name,
|
||||
iteration=self.current_epoch,
|
||||
image=annotated_image)
|
||||
self.current_epoch_logged_images.add(image_path)
|
|
@ -0,0 +1,84 @@
|
|||
from clearml import Task
|
||||
# Connecting ClearML with the current process,
|
||||
# from here on everything is logged automatically
|
||||
from clearml.automation import HyperParameterOptimizer, UniformParameterRange
|
||||
from clearml.automation.optuna import OptimizerOptuna
|
||||
|
||||
task = Task.init(project_name='Hyper-Parameter Optimization',
|
||||
task_name='YOLOv5',
|
||||
task_type=Task.TaskTypes.optimizer,
|
||||
reuse_last_task_id=False)
|
||||
|
||||
# Example use case:
|
||||
optimizer = HyperParameterOptimizer(
|
||||
# This is the experiment we want to optimize
|
||||
base_task_id='<your_template_task_id>',
|
||||
# here we define the hyper-parameters to optimize
|
||||
# Notice: The parameter name should exactly match what you see in the UI: <section_name>/<parameter>
|
||||
# For Example, here we see in the base experiment a section Named: "General"
|
||||
# under it a parameter named "batch_size", this becomes "General/batch_size"
|
||||
# If you have `argparse` for example, then arguments will appear under the "Args" section,
|
||||
# and you should instead pass "Args/batch_size"
|
||||
hyper_parameters=[
|
||||
UniformParameterRange('Hyperparameters/lr0', min_value=1e-5, max_value=1e-1),
|
||||
UniformParameterRange('Hyperparameters/lrf', min_value=0.01, max_value=1.0),
|
||||
UniformParameterRange('Hyperparameters/momentum', min_value=0.6, max_value=0.98),
|
||||
UniformParameterRange('Hyperparameters/weight_decay', min_value=0.0, max_value=0.001),
|
||||
UniformParameterRange('Hyperparameters/warmup_epochs', min_value=0.0, max_value=5.0),
|
||||
UniformParameterRange('Hyperparameters/warmup_momentum', min_value=0.0, max_value=0.95),
|
||||
UniformParameterRange('Hyperparameters/warmup_bias_lr', min_value=0.0, max_value=0.2),
|
||||
UniformParameterRange('Hyperparameters/box', min_value=0.02, max_value=0.2),
|
||||
UniformParameterRange('Hyperparameters/cls', min_value=0.2, max_value=4.0),
|
||||
UniformParameterRange('Hyperparameters/cls_pw', min_value=0.5, max_value=2.0),
|
||||
UniformParameterRange('Hyperparameters/obj', min_value=0.2, max_value=4.0),
|
||||
UniformParameterRange('Hyperparameters/obj_pw', min_value=0.5, max_value=2.0),
|
||||
UniformParameterRange('Hyperparameters/iou_t', min_value=0.1, max_value=0.7),
|
||||
UniformParameterRange('Hyperparameters/anchor_t', min_value=2.0, max_value=8.0),
|
||||
UniformParameterRange('Hyperparameters/fl_gamma', min_value=0.0, max_value=4.0),
|
||||
UniformParameterRange('Hyperparameters/hsv_h', min_value=0.0, max_value=0.1),
|
||||
UniformParameterRange('Hyperparameters/hsv_s', min_value=0.0, max_value=0.9),
|
||||
UniformParameterRange('Hyperparameters/hsv_v', min_value=0.0, max_value=0.9),
|
||||
UniformParameterRange('Hyperparameters/degrees', min_value=0.0, max_value=45.0),
|
||||
UniformParameterRange('Hyperparameters/translate', min_value=0.0, max_value=0.9),
|
||||
UniformParameterRange('Hyperparameters/scale', min_value=0.0, max_value=0.9),
|
||||
UniformParameterRange('Hyperparameters/shear', min_value=0.0, max_value=10.0),
|
||||
UniformParameterRange('Hyperparameters/perspective', min_value=0.0, max_value=0.001),
|
||||
UniformParameterRange('Hyperparameters/flipud', min_value=0.0, max_value=1.0),
|
||||
UniformParameterRange('Hyperparameters/fliplr', min_value=0.0, max_value=1.0),
|
||||
UniformParameterRange('Hyperparameters/mosaic', min_value=0.0, max_value=1.0),
|
||||
UniformParameterRange('Hyperparameters/mixup', min_value=0.0, max_value=1.0),
|
||||
UniformParameterRange('Hyperparameters/copy_paste', min_value=0.0, max_value=1.0)],
|
||||
# this is the objective metric we want to maximize/minimize
|
||||
objective_metric_title='metrics',
|
||||
objective_metric_series='mAP_0.5',
|
||||
# now we decide if we want to maximize it or minimize it (accuracy we maximize)
|
||||
objective_metric_sign='max',
|
||||
# let us limit the number of concurrent experiments,
|
||||
# this in turn will make sure we do dont bombard the scheduler with experiments.
|
||||
# if we have an auto-scaler connected, this, by proxy, will limit the number of machine
|
||||
max_number_of_concurrent_tasks=1,
|
||||
# this is the optimizer class (actually doing the optimization)
|
||||
# Currently, we can choose from GridSearch, RandomSearch or OptimizerBOHB (Bayesian optimization Hyper-Band)
|
||||
optimizer_class=OptimizerOptuna,
|
||||
# If specified only the top K performing Tasks will be kept, the others will be automatically archived
|
||||
save_top_k_tasks_only=5, # 5,
|
||||
compute_time_limit=None,
|
||||
total_max_jobs=20,
|
||||
min_iteration_per_job=None,
|
||||
max_iteration_per_job=None,
|
||||
)
|
||||
|
||||
# report every 10 seconds, this is way too often, but we are testing here
|
||||
optimizer.set_report_period(10)
|
||||
# You can also use the line below instead to run all the optimizer tasks locally, without using queues or agent
|
||||
# an_optimizer.start_locally(job_complete_callback=job_complete_callback)
|
||||
# set the time limit for the optimization process (2 hours)
|
||||
optimizer.set_time_limit(in_minutes=120.0)
|
||||
# Start the optimization process in the local environment
|
||||
optimizer.start_locally()
|
||||
# wait until process is done (notice we are controlling the optimization process in the background)
|
||||
optimizer.wait()
|
||||
# make sure background optimization stopped
|
||||
optimizer.stop()
|
||||
|
||||
print('We are done, good bye')
|
|
@ -43,6 +43,9 @@ def check_wandb_config_file(data_config_file):
|
|||
def check_wandb_dataset(data_file):
|
||||
is_trainset_wandb_artifact = False
|
||||
is_valset_wandb_artifact = False
|
||||
if isinstance(data_file, dict):
|
||||
# In that case another dataset manager has already processed it and we don't have to
|
||||
return data_file
|
||||
if check_file(data_file) and data_file.endswith('.yaml'):
|
||||
with open(data_file, errors='ignore') as f:
|
||||
data_dict = yaml.safe_load(f)
|
||||
|
@ -121,7 +124,7 @@ class WandbLogger():
|
|||
"""
|
||||
- Initialize WandbLogger instance
|
||||
- Upload dataset if opt.upload_dataset is True
|
||||
- Setup trainig processes if job_type is 'Training'
|
||||
- Setup training processes if job_type is 'Training'
|
||||
|
||||
arguments:
|
||||
opt (namespace) -- Commandline arguments for this run
|
||||
|
@ -170,7 +173,11 @@ class WandbLogger():
|
|||
if not opt.resume:
|
||||
self.wandb_artifact_data_dict = self.check_and_upload_dataset(opt)
|
||||
|
||||
if opt.resume:
|
||||
if isinstance(opt.data, dict):
|
||||
# This means another dataset manager has already processed the dataset info (e.g. ClearML)
|
||||
# and they will have stored the already processed dict in opt.data
|
||||
self.data_dict = opt.data
|
||||
elif opt.resume:
|
||||
# resume from artifact
|
||||
if isinstance(opt.resume, str) and opt.resume.startswith(WANDB_ARTIFACT_PREFIX):
|
||||
self.data_dict = dict(self.wandb_run.config.data_dict)
|
||||
|
|
|
@ -209,6 +209,7 @@ class ConfusionMatrix:
|
|||
yticklabels=names + ['background FN'] if labels else "auto").set_facecolor((1, 1, 1))
|
||||
fig.axes[0].set_xlabel('True')
|
||||
fig.axes[0].set_ylabel('Predicted')
|
||||
plt.title('Confusion Matrix')
|
||||
fig.savefig(Path(save_dir) / 'confusion_matrix.png', dpi=250)
|
||||
plt.close()
|
||||
except Exception as e:
|
||||
|
@ -336,6 +337,7 @@ def plot_pr_curve(px, py, ap, save_dir=Path('pr_curve.png'), names=()):
|
|||
ax.set_xlim(0, 1)
|
||||
ax.set_ylim(0, 1)
|
||||
plt.legend(bbox_to_anchor=(1.04, 1), loc="upper left")
|
||||
plt.title('Precision-Recall Curve')
|
||||
fig.savefig(save_dir, dpi=250)
|
||||
plt.close()
|
||||
|
||||
|
@ -357,5 +359,6 @@ def plot_mc_curve(px, py, save_dir=Path('mc_curve.png'), names=(), xlabel='Confi
|
|||
ax.set_xlim(0, 1)
|
||||
ax.set_ylim(0, 1)
|
||||
plt.legend(bbox_to_anchor=(1.04, 1), loc="upper left")
|
||||
plt.title(f'{ylabel}-Confidence Curve')
|
||||
fig.savefig(save_dir, dpi=250)
|
||||
plt.close()
|
||||
|
|
|
@ -148,6 +148,7 @@ def feature_visualization(x, module_type, stage, n=32, save_dir=Path('runs/detec
|
|||
ax[i].axis('off')
|
||||
|
||||
LOGGER.info(f'Saving {f}... ({n}/{channels})')
|
||||
plt.title('Features')
|
||||
plt.savefig(f, dpi=300, bbox_inches='tight')
|
||||
plt.close()
|
||||
np.save(str(f.with_suffix('.npy')), x[0].cpu().numpy()) # npy save
|
||||
|
|
Loading…
Reference in New Issue